全文HTML
--> --> -->提高单光子探测器性能指标, 尤其是光子数分辨能力方面, 近年来主要关注于光敏材料的优化及其器件的制备工艺等探测器硬件本身, 包括: 寻找对单光子响应更灵敏的光敏材料、设计并制备具有快响应时间和噪声特性更好的器件及单光子响应信号读取线路等. 例如, 2008年Lita等[11]采用钨研制出的TES在1556 nm波长的探测效率达到了95%, 能量分辨达到了0.29 eV; 2013年, Lolli等[12]用Ti/Au合金制备的TES单光子探测器实现了最高可分辨29个光子, 能量分辨达到了0.113 eV. 由此, 具有较高光子数分辨水平的TES在量子光学实验中得到了推广应用. 在国内, 中国科学院上海微系统与信息技术研究所和南京大学等实现了高性能超导纳米线单光子探测器的研发和应用[13,14]; 中国科学院南京紫金山天文台等单位也实现了针对天文观测应用的TES单光子探测器[15]. 2017年, 通过和美国国家标准与技术研究院(NIST)合作, 西南交通大学课题组研发了光通信波段可分辨7个光子的光子数可分辨MKID探测器, 其能量分辨最高可达0.22 eV[16], 并实现了阵列探测器的集成[17]. 一般来说, 弱信号探测器件的性能主要取决于探测材料和器件制备工艺等硬件优化, 但与噪声背景中的信号提取也有很大关系. 本文将讨论在探测器芯片、测试线路等硬件条件一定的情况下, 如何通过探测数据中的噪声信号特性分析、建立更有效数据处理方法等, 进一步研究提高批量制备MIKD单光子探测器能量分辨的方法.
本文第2节简要介绍MKID单光子探测的工作原理、结合所测试的弱相干光脉冲特性阐明实验中数据的采集和处理方法. 将实验测量数据作为前置滤波信号, 在第3节中对噪声信号处理方法进行了改进, 针对理想化的白噪声模型和实测噪声的白化处理, 实现了噪声信号最优滤波算法处理, 将批量制备MKID单光子探测器的主要性能指标-能量分辨提升了15%左右. 第4节总结.
2.1.实验测量系统
图1为实验所采用的测量系统简单示意图. 其中弱光脉冲由1550 nm激光二极管输出, 经衰减器后由光纤导入对准10 mK环境中由1/4波长超导谐振器构成的探测器芯片, 照射到探测器芯片上的光子能量拆散超导库珀对成为准粒子, 导致谐振器频率可检测的变化. 测试信号由微波信号产生器输入后一路作为本地信号, 另一路作为探测器的探测信号; 在输出端的IQ混频器实现本地信号和探测信号的混频, 经低通滤波器滤波、AD模数转换后由数据采集卡进行收集.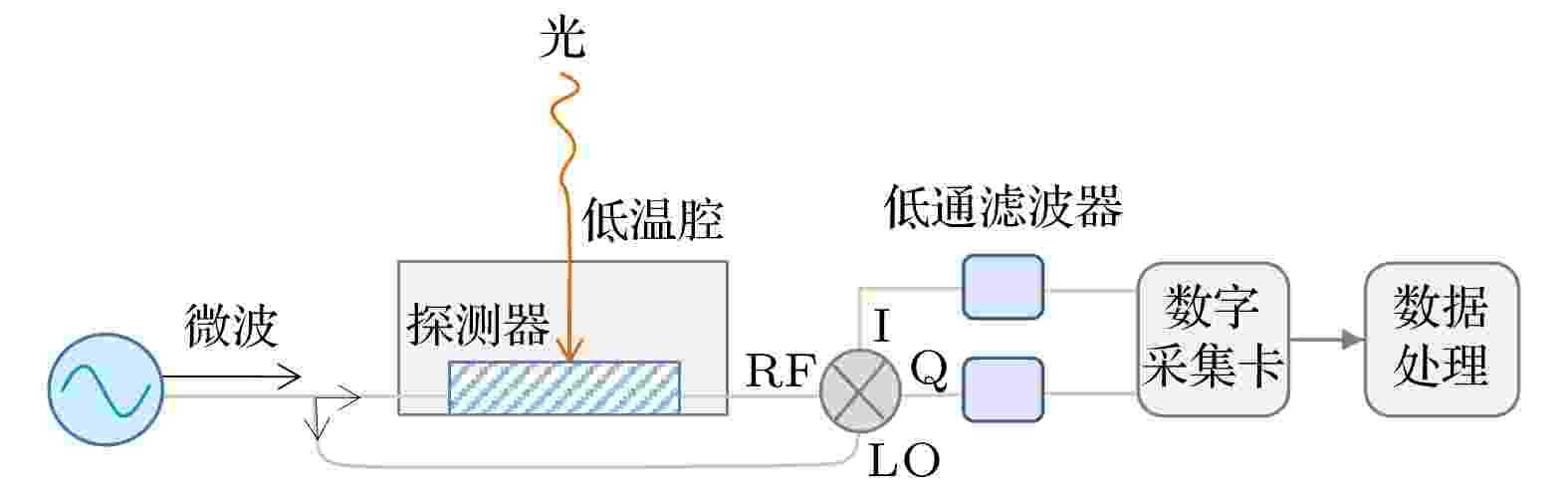
Figure1. Schematic diagram of the experimental system for single-photon detection.
图2为探测器的弱光信号的响应示意图. 其中, 蓝色的圆代表由频率扫描得到的无光照射时的IQ信号谐振圆; 红色箭头表示脉冲导致的信号上升沿, 该响应可以投影到沿谐振环切向的频率响应和沿谐振环法向的噪声响应; 绿色是实际测量中所得到的一个脉冲信号的整个响应. 在实际测量中信号主要沿频率方向响应且恢复至平衡点的时间更长, 因此主要对频率信号进行分析.
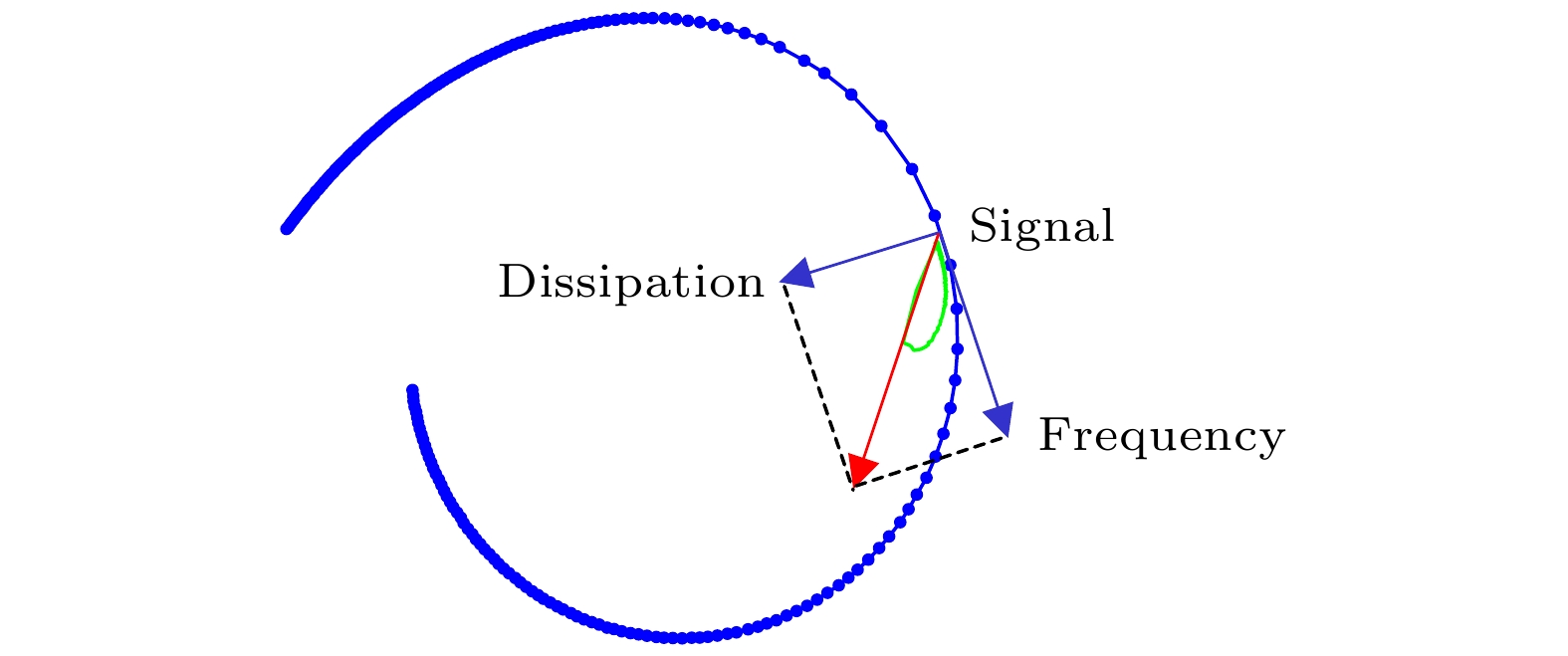
Figure2. Pulse response diagram.
图3简单表示了一个数据的采集时间操作序列. 其中, 蓝色为IQ信号输出; 红色代表200 ns宽的光脉冲; 黄色为信号产生器的120 Hz触发信号并同步至模数转换AD采集卡, 其采样率为2.5 MHz. 每次实验在触发模式下采集20000个脉冲, 并对脉冲前后各5000个(2 ms), 共10000个(4 ms)数据点进行记录分析. 在数据处理过程中, 取前5000个数据点用作噪声分析, 取2501—7500这5000个数据点进行幅值估计.
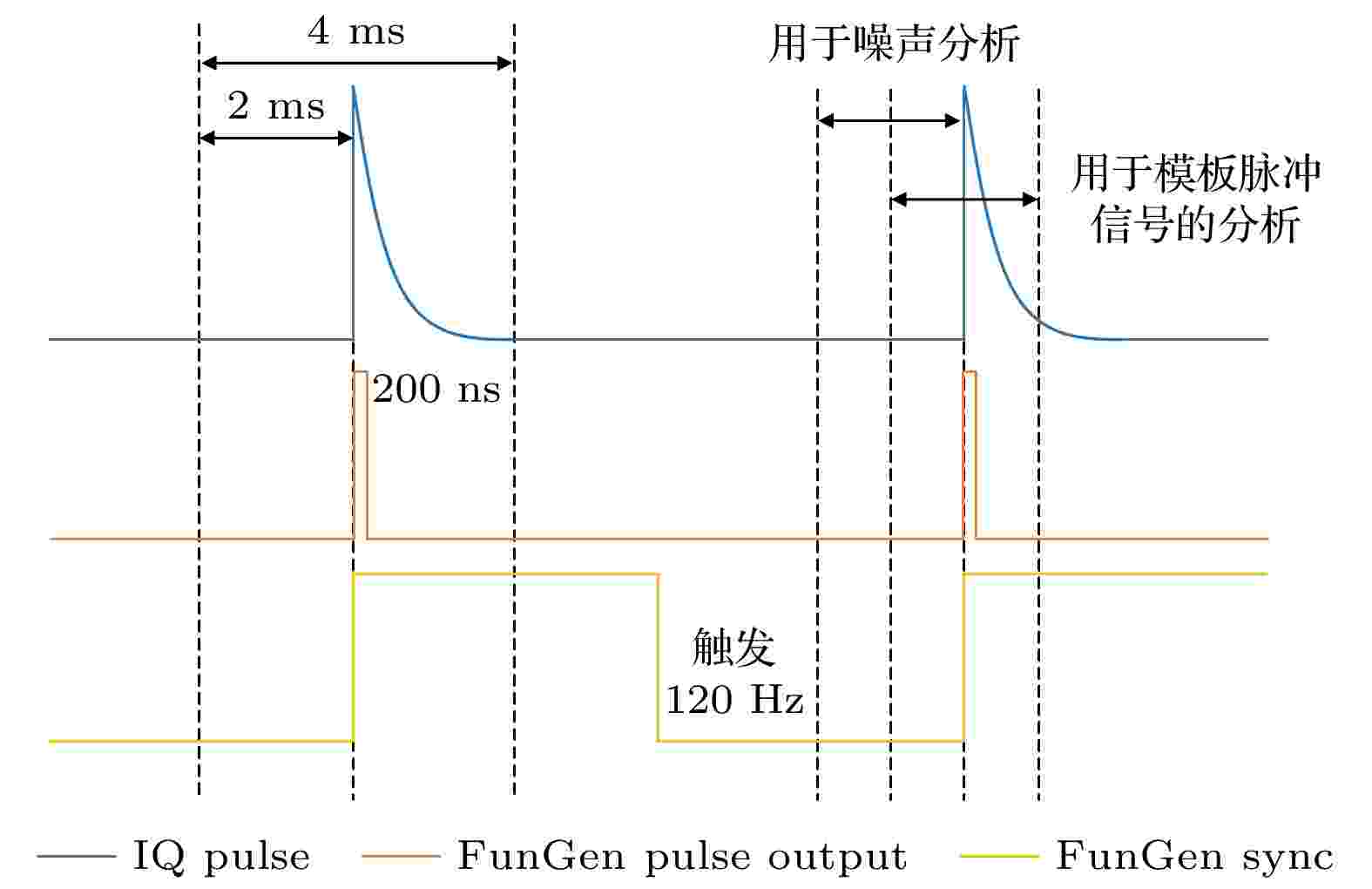
Figure3. Schematic diagram of the signal triggers and records in time domain.
2
2.2.MKID探测器的光子探测数据处理
MKID探测数据的处理实际上是一个噪声信号与模板匹配的最优滤波过程[18,19]. 这里模板匹配指的是利用计算机计算探测器信号输出与模板信号之间的互相关程度. 模板信号的最简单提取方法就是多次脉冲信号取平均得到:



对系统实测噪声的特性进行了分析, 如图4所示, 可以发现在极低温环境下, 系统的实测噪声比较接近于白噪声, 其自相关函数比较接近于冲激函数, 功率谱密度函数虽然在一个常数周围波动, 但不够平滑. 这必定会导致平均得到的模板函数与真实的脉冲相似波形存在误差. 尝试用此噪声模型进行滤波处理. 最优滤波算法中最优幅值倍数表达式为[20]
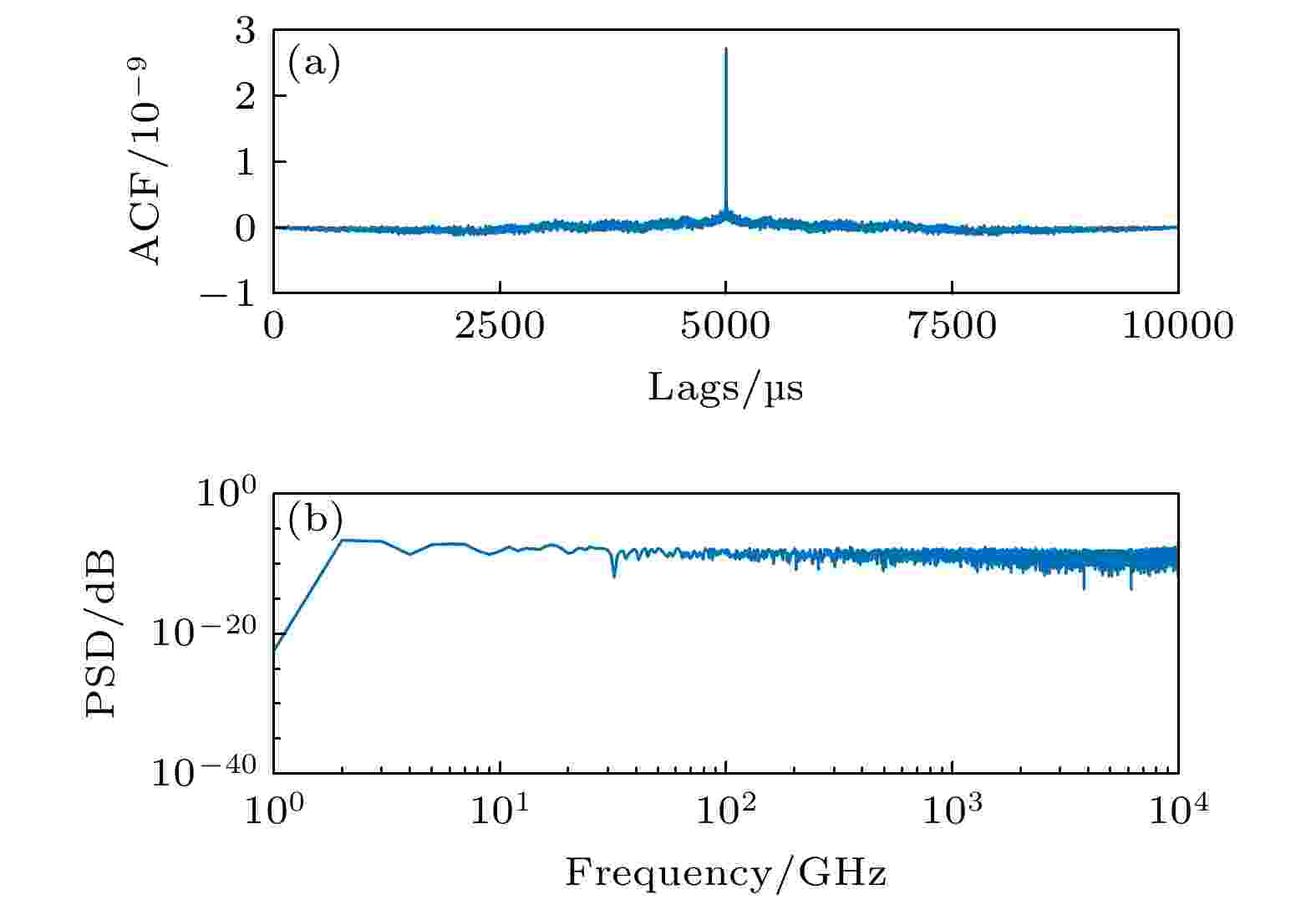

Figure4. Autocorrelation function (a) and power spectral density(b) of detector noise measured experimentally.





实验中的模板信号是对弱光脉冲照射下谐振频率为6.44 GHz的MKID探测器所探测到的20000次采样信号进行平均而得到的. 利用矩阵分析软件实现了最优滤波算法, 得到图5所示的两组MKID探测器光脉冲响应信号的幅值倍数统计分布及高斯拟合结果. 这里, 高斯拟合的表达式为












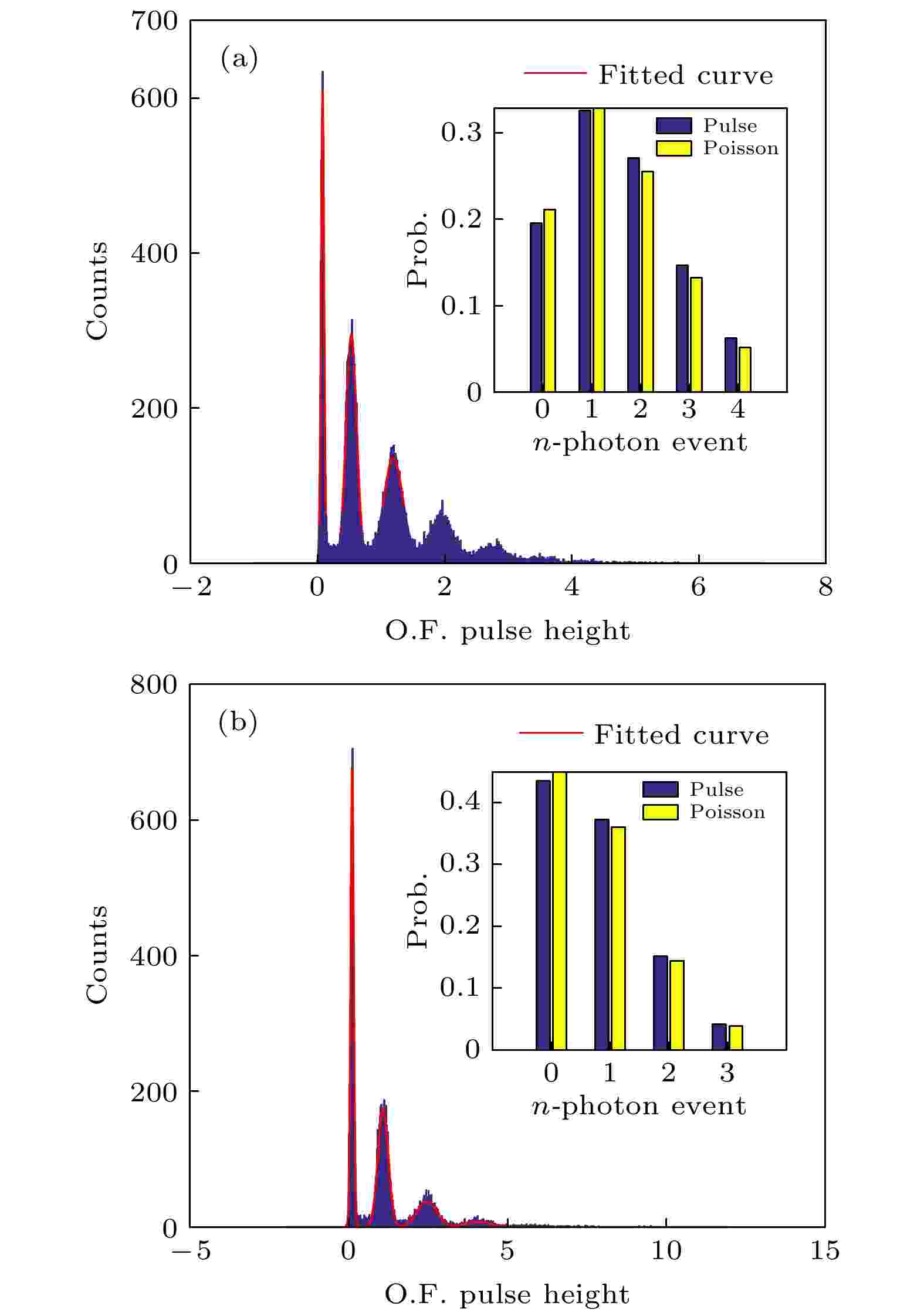
Figure5. Gaussian fitting results of pulse signal amplitudes under optical attenuation of 17 dB (a) and 20 dB (b), respectively, for the experimental noises.
2
2.3.弱光脉冲的特性分析
实验中到达探测器芯片上的是一种通过强衰减所获得的相干光, 它并不是严格的单光子源, 而是一种平均光子数很少的弱相干光源: 每次脉冲所包含的光子数是以平均光子数为均值的一种泊松分布[21]. 因此, 在探测器端所探测到的信号也应该是某种对应的泊松分布. 实际上, 在短时间



















将图5所示弱相干光的光子数标准泊松分布(黄色直方图)与实验数据拟合的高斯分布面积分布图(蓝色直方图)进行比较, 可以看出实测的弱光脉冲信号中的光子数分布与上述理论分析预期的标准泊松分布基本一致, 这说明照射到MKID探测器芯片上的光脉冲确实是一种弱相干信号. 图5中两组数据分别是探测器对光源衰减17 dB和衰减20 dB探测所得到的平均光子数分别为



2
2.4.MKID单光子探测器的能量分辨
单光子探测器的一个重要性能指标是其光子数可分辨性. 由于探测器信号存在噪声, 所以每个光子数峰都不是严格的

























能量分辨 | $ \Delta E_{{0}}{/{\rm{eV}}} $ | $ \Delta E_{{1}}{/{\rm{eV}}} $ | $ \Delta E_{2}{/{\rm{eV}}} $ | $ \Delta E_{3}{/{\rm{eV}}} $ | $ \Delta E_{4}{/{\rm{eV}}} $ |
衰减17 dB 的信号 | 0.1015 | 0.3526 | 0.4360 | 0.4691 | 0.6140 |
衰减20 dB 的信号 | 0.0955 | 0.3200 | 0.4199 | 0.4758 | — |
表1光学衰减17 dB和20 dB下探测器的能量分辨
Table1.Energy resolution of detector under optical attenuation of 17 dB and 20 dB.

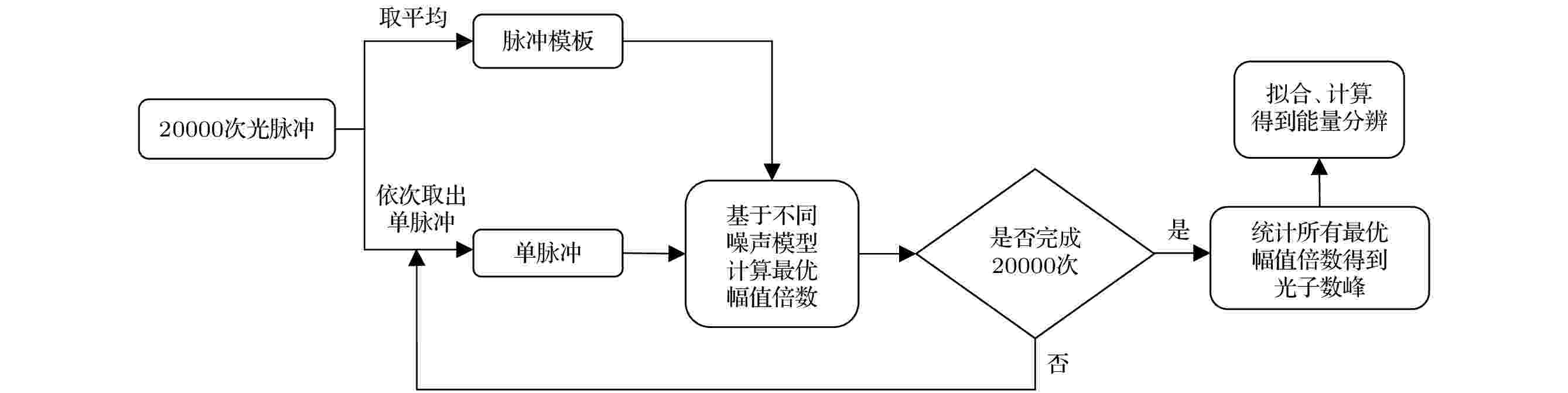
Figure6. Flow diagram of the signal processing.
2
3.1.白噪声模型
从图4所示的实测噪声功率谱密度分布特性中, 我们看到探测器的噪声非常接近于白噪声, 所以我们首先对实测的噪声信号进行高斯白噪声拟合, 如图7所示. 对此拟合拟合的噪声模型, 通常的模板分析方法无疑是最佳的, 从而公式(2)可简化为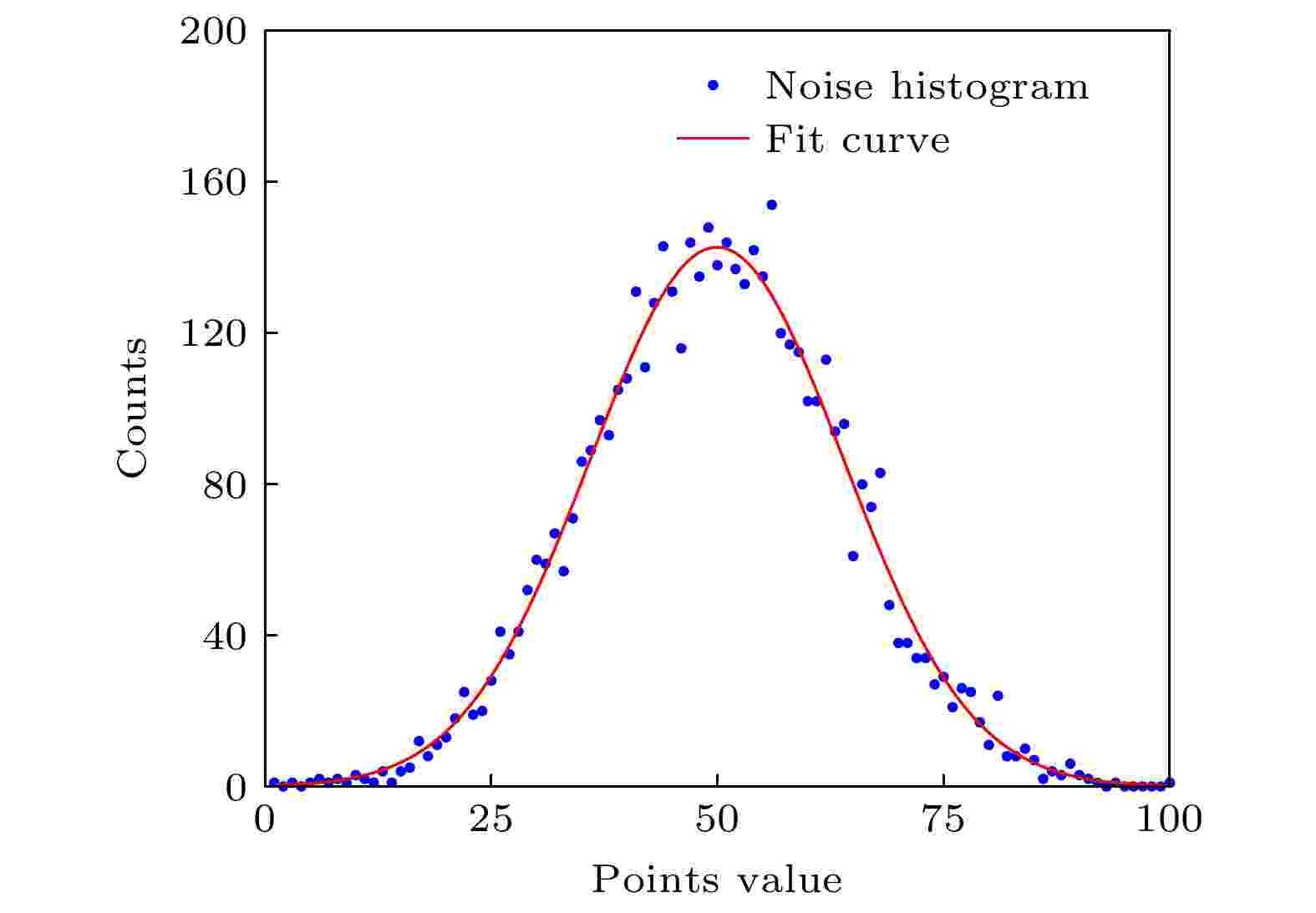
Figure7. Detector noise (point set) and Gaussian distribution function fitting (red solid line).

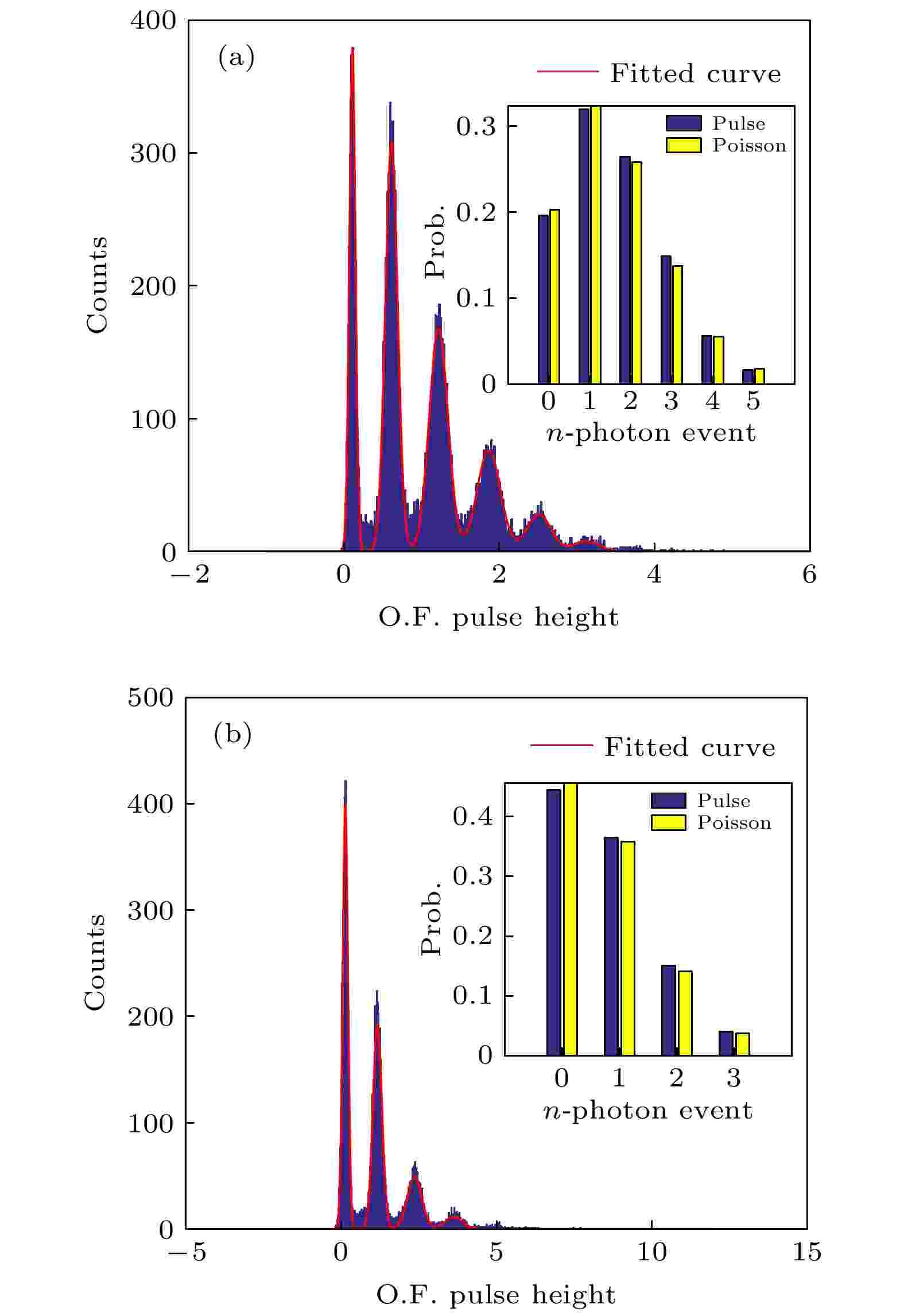
Figure8. Gaussian fitting results of pulse signal amplitudes under optical attenuation of 17 dB (a) and 20 dB (b), respectively, for the ideal white noises.
能量分辨 | $ \Delta E_{{0}}{/{\rm{eV}}} $ | $ \Delta E_{{1}}{/{\rm{eV}}} $ | $ \Delta E_{2}{/{\rm{eV}}} $ | $ \Delta E_{3}{/{\rm{eV}}} $ | $ \Delta E_{4}{/{\rm{eV}}} $ | $ \Delta E_{5}{/{\rm{eV}}} $ |
实测噪声 | 0.1015 | 0.3526 | 0.4360 | 0.4691 | 0.6140 | — |
白噪声模型 | 0.1489 | 0.2992 | 0.3772 | 0.4382 | 0.4448 | 0.5113 |
提高 | –31.83% | +17.85% | +15.59% | +7.05% | +38.04% | — |
表2光学衰减17 dB下使用实测噪声和白噪声模型处理后探测器能量分辨对比
Table2.Comparison of detector energy resolutions after processing with measured noise and white noise model under optical attenuation of 17 dB.
从表2和表3对比可以看出, 除了零光子峰外的其他光子峰的能量分辨都有所提高, 最高提高效率达到了38%. 而且, 从表2第2行数据可以看到, 对噪声模型的修改数据处理后, 可以分辨原来不能分辨的5光子峰响应. 尤其是, 从表3可以看到, 探测器的单光子能量分辨达到0.265 eV, 比之前的数据处理结果(0.32 eV)提高了17%左右.
能量分辨 | $ \Delta E_{{0}}{/{\rm{eV}}} $ | $ \Delta E_{{1}}{/{\rm{eV}}} $ | $ \Delta E_{2}{/{\rm{eV}}} $ | $ \Delta E_{3}{/{\rm{eV}}} $ |
实测噪声 | 0.0955 | 0.3200 | 0.4199 | 0.4758 |
白噪声模型 | 0.1553 | 0.2650 | 0.3748 | 0.4032 |
提高/% | –62.61 | +17.19 | +10.74 | +15.26 |
表3光学衰减20 dB下使用实测噪声和白噪声模型处理后探测器能量分辨对比
Table3.Comparison of detector energy resolutions after processing with measured noise and white noise model under optical attenuation of 20 dB.
2
3.2.噪声白化模型
现实情况下严格的白噪声是不存在的, 所以以上采用白噪声模型来实现最优滤波算法的数据处理过于理想化. 为此, 对实测噪声特性进行白化处理[24], 使之尽量接近最优滤波算法中约定的白噪声模型. 实测噪声的白化处理主要步骤是: 首先, 对噪声数组求自相关得到噪声的自相关函数






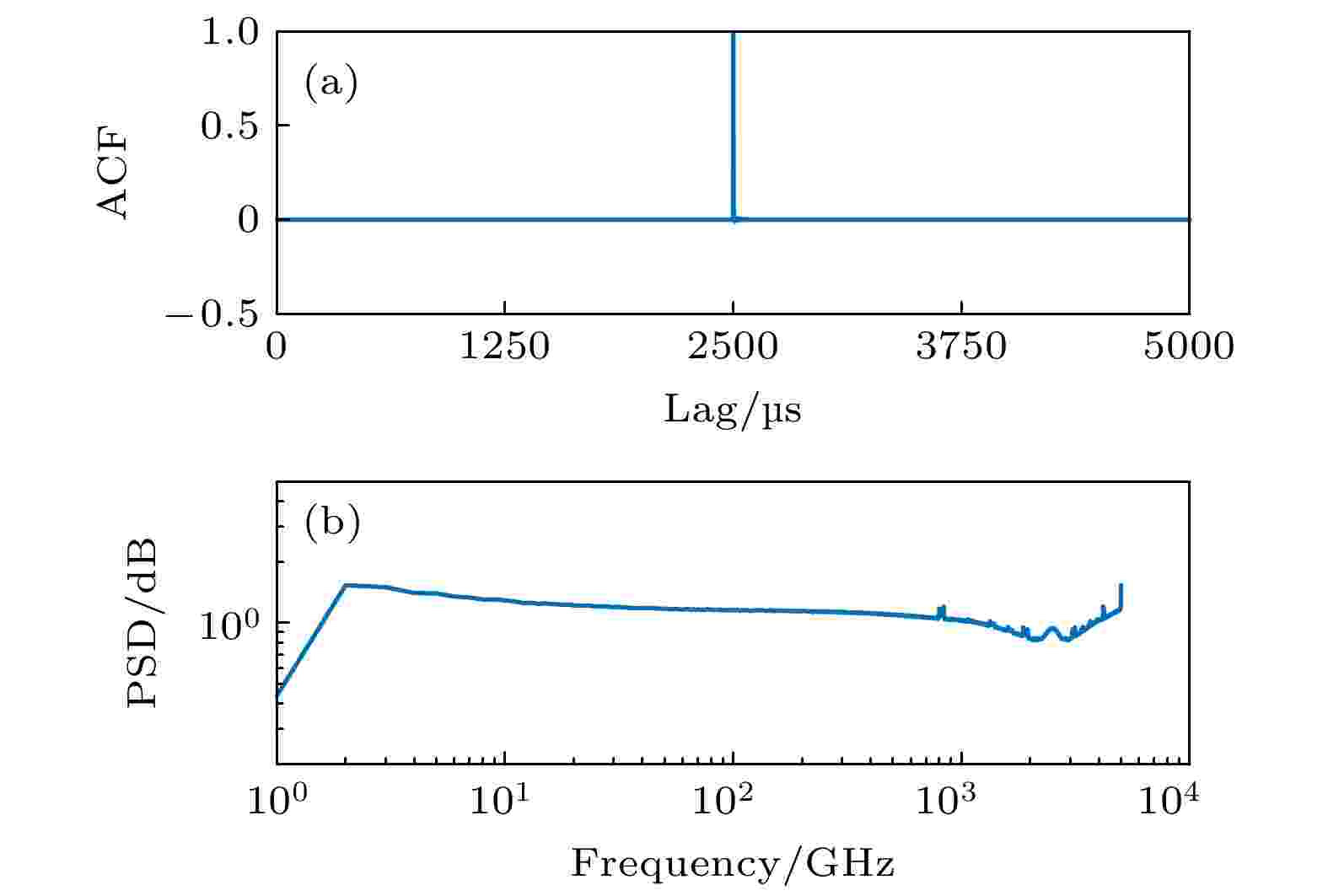

Figure9. Autocorrelation function (a) and Power spectral density (b) of the whitened noise.
可以看出, 白化后的噪声相比于之前系统的实测噪声更加接近白噪. 因此, 相比于图4, 图9的自相关函数更接近于冲激函数, 并且功率谱密度函数更平滑. 将得到的白化后的噪声用于最优滤波幅值估计, 可得最优幅值倍数的矩阵形式[25]:
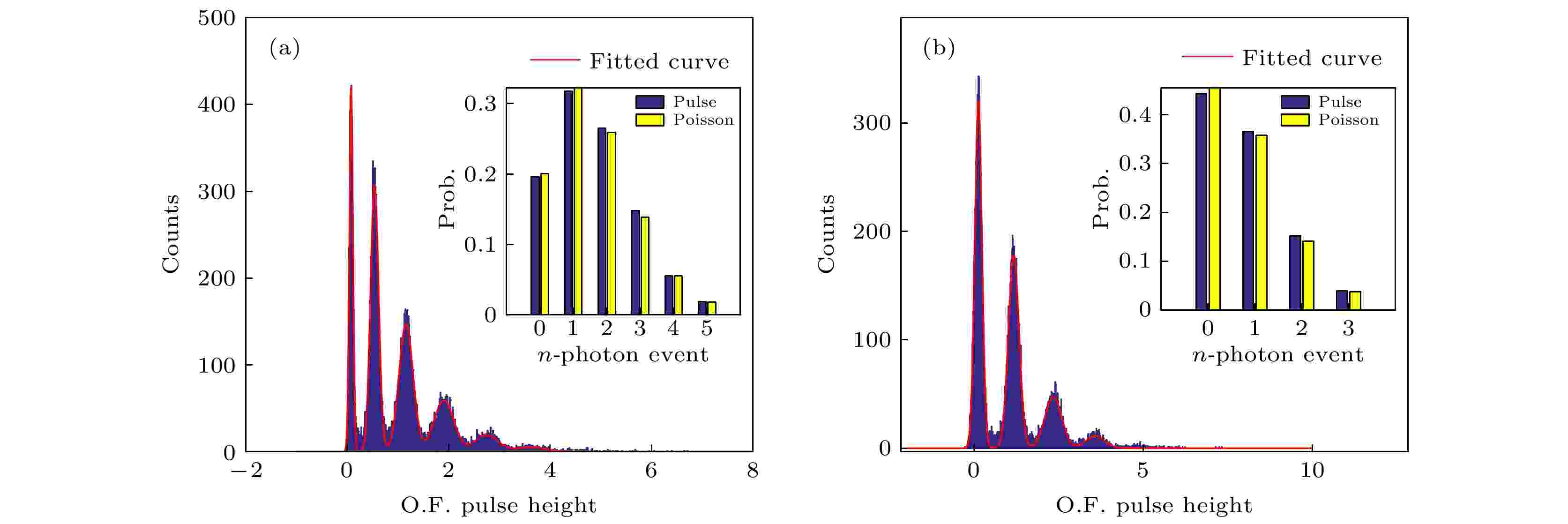
Figure10. Gaussian fitting results of pulse signal amplitudes under optical attenuation of 17 dB (a) and 20 dB (b), respectively, for the whitened noises.
能量分辨 | $ \Delta {{E}}_{{0}}{/{\rm{eV}}} $ | $ \Delta {{E}}_{{1}}{/{\rm{eV}}} $ | $ \Delta {{E}}_{2}{/{\rm{eV}}} $ | $ \Delta {{E}}_{3}{/{\rm{eV}}} $ | $ \Delta {{E}}_{4}{/{\rm{eV}}} $ | $ \Delta {{E}}_{5}{/{\rm{eV}}} $ |
实测噪声 | 0.1015 | 0.3526 | 0.4360 | 0.4691 | 0.6140 | — |
噪声白化 | 0.1469 | 0.3274 | 0.4263 | 0.4897 | 0.5009 | 0.6216 |
提高/% | –44.73 | +7.15 | +2.22 | –4.39 | +18.42 | — |
表4光学衰减17 dB下实测噪声和噪声白化后处理得到的探测器能量分辨对比
Table4.Comparison of energy resolutions for the experimental noises and the whitening ones, where the optical pulse is attenuated 17 dB.
能量分辨 | $ \Delta {{E}}_{{0}}{/{\rm{eV}}} $ | $ \Delta {{E}}_{{1}}{/{\rm{eV}}} $ | $ \Delta {{E}}_{2}{/{\rm{eV}}} $ | $ \Delta {{E}}_{3}{/{\rm{eV}}} $ |
实测噪声 | 0.0955 | 0.3200 | 0.4199 | 0.4758 |
噪声白化 | 0.1932 | 0.2855 | 0.39649 | 0.4196 |
提高/% | –100.02 | +10.78 | +5.60 | +11.81 |
表5光学衰减20 dB下实测噪声和噪声白化后处理得到的探测器能量分辨对比
Table5.Comparison of energy resolutions for the experimental noises and the whitening ones, where the optical pulse is attenuated 20 dB.
从表5可以看出, 通过噪声白化手段处理后, 相比于实测噪声的最优滤波处理, 能量分辨在除0光子峰之外的其他光子也峰略有提高, 但并不比直接使用白噪声模型处理的结果明显. 最后, 为比较方便起见, 表6给出了对光学衰减20 dB的弱相干光, 采用3种噪声模型处理所得到的探测器对1, 2, 和3光子数峰的能量分辨对比.
能量分辨 | $ \Delta {{E}}_{1}{/{\rm{eV}}} $ | $ \Delta {{E}}_{{2}}{/{\rm{eV}}} $ | $ \Delta {{E}}_{3}{/{\rm{eV}}} $ |
实测噪声 | 0.3200 | 0.4199 | 0.4758 |
白噪声模型 | 0.2650 (+17.19%) | 0.3748 (+10.74%) | 0.4032 (+15.26%) |
噪声白化 | 0.2855 (+10.78%) | 0.39649 (+5.60%) | 0.4196 (+11.81%) |
表6光学衰减20 dB下原始滤波和改进噪声模型后探测器能量分辨对比(括号中是改进后相对于实测噪声处理所得到的能量分辨的提高百分比)
Table6.Comparison of energy resolutions of the detector for the experimental noise, white noise and withened noise, respectively. The improvemence is relative to the those for the experimental noise. Here, the optical pulse is attenuated 20 dB.
需要说明的是, 两种改进方法相比于之前使用实测噪声进行最优滤波处理所得的结果都有所提高. 这说明, 基于白噪声模型的最优滤波算法在探测器响应实验数据处理方面, 基本是可行的. 虽然实际噪声并不是白噪声, 但通过噪声白化处理后仍可利用最优滤波算法进行数据处理.
本文工作是在噪声模型近似处理基础上利用最优滤波算法进行探测器噪声信号的处理. 由于最优滤波算法中模板的提取是以白噪声模型为基础的, 所以对实测噪声做白化处理是为了发挥最优滤波算法中模板提取相对容易的优势. 在以后的工作中, 有必要针对实测噪声(一般是有色噪声)利用大数据机器学习的方法来提取与实测噪声特性更为符合的脉冲模板, 再进行噪声滤波, 从而有望在数据处理环节进一步实现MIKD单光子探测器各种性能指标的提升.
感谢美国国家标准与技术研究院(NIST)高湔松博士的讨论和帮助.