

Prediction of drought and salt stress-related genes in rice based on multi-platform gene expression data
LIU Ya-Wen

通讯作者: * 张红燕, E-mail:hongyan_zhang@hunau.edu.cn
收稿日期:2020-11-30接受日期:2021-04-26网络出版日期:2021-06-02
基金资助: |
Corresponding authors: * E-mail:hongyan_zhang@hunau.edu.cn
Received:2020-11-30Accepted:2021-04-26Published online:2021-06-02
Fund supported: |
作者简介 About authors
E-mail:lyw20201022@163.com

摘要
基于多平台基因表达数据挖掘水稻胁迫相关基因, 可增加关键基因预测的可靠性, 获得更具普适意义的结果。本研究从NCBI数据库中收集了与水稻非生物胁迫相关的94份affymetrix基因芯片数据和42份RNA-seq转录组数据。首先对同一类型同一胁迫相关的多个数据集以数据转换法融合, 得到干旱胁迫相关的affymetrix数据集D_affy和RNA-seq数据集D_rnaseq, 盐胁迫相关的affymetrix数据集S_affy和RNA-seq数据集S_rnaseq; 接着对4个数据集分别基于Pearson线性相关系数的经典WGCNA法和基于MIC非线性相关系数的改进WGCNA法进行基因共表达网络分析, 共获取胁迫相关的8个Hub基因集; 进一步, 对同一胁迫相关的Hub基因进行整合分析, 得到最终的水稻干旱胁迫相关Hub基因1936个、盐胁迫相关的Hub基因1504个。最后, 从预测性能、富集分析、文献报道、STRING在线互作分析和Cytoscape可视化分析等多角度解析Hub基因的生物学意义。结果显示: Hub基因整体预测性能较优, 且大多富集到了与干旱/盐胁迫相关的通路上, 其中有文献已报道的干旱胁迫响应基因31个和盐胁迫响应基因22个。此外, 通过对Hub基因的互作分析, 预测得到11个干旱胁迫候选基因和5个盐胁迫候选基因。本研究为“高维度、小样本”的农作物基因测序数据的有效分析提供了新思路, 实验结果为抗逆水稻品种研究提供了参考。
关键词:
Abstract
Mining stress-related genes based on multi-platform gene expression data in rice can increase the reliability of key genes prediction and obtain more universally meaningful results. In this study, 94 affymetrix microarray data and 42 RNA-seq transcriptome data related to rice abiotic stress were collected from NCBI databases. First, multiple datasets related to the same stress on the same type were fused by data conversion method to obtain the affymetrix data set D_affy and RNA-seq data set D_rnaseq related to drought stress, and the affymetrix data set S_affy and the RNA-seq data set S_rnaseq related to salt stress. Then, the four datasets were analyzed by the classical WGCNA method based on Pearson's linear correlation coefficient and the improved WGCNA method based on the MIC nonlinear correlation coefficient respectively, and the eight Hub gene sets related to stress were obtained. Further, the integration analysis of stress-related Hub genes yielded the final 1936 drought stress-related Hub genes and 1504 salt stress-related Hub genes. Finally, the biological significance of Hub gene was analyzed from multiple perspectives, including prediction performance, enrichment analysis, literature report, STRING online interaction analysis, and Cytoscape visualization analysis. The results revealed that the overall prediction performance of Hub genes was better, and most of them were enriched in the pathways related to drought/salt stress. Among them, there were 31 drought stress response genes and 22 salt stress response genes reported in the literatures. In addition, 11 drought stress candidate genes and 5 salt stress candidate genes were predicted using the interaction analysis of Hub genes. In conclusion, This study provides a new idea for the effective analysis of “high-dimensional, small-sample” crop gene sequencing data, and the experimental results provide a reference for the study of stress-resistant rice varieties.
Keywords:
PDF (3476KB)元数据多维度评价相关文章导出EndNote|Ris|Bibtex收藏本文
本文引用格式
刘亚文, 张红燕, 曹丹, 李兰芝. 基于多平台基因表达数据的水稻干旱和盐胁迫相关基因预测. 作物学报, 2021, 47(12): 2423-2439 DOI:10.3724/SP.J.1006.2021.02084
LIU Ya-Wen, ZHANG Hong-Yan, CAO Dan, LI Lan-Zhi.
水稻在生长发育的过程中受到干旱、高盐等非生物逆境因素胁迫时, 易导致大面积的减产、品质下降甚至坏死[1], 提高其逆境抗性将增加农业产量并扩大适宜耕种面积, 缓解人口压力。水稻的逆境抗性受多基因控制, 基于基因组学数据挖掘水稻非生物胁迫相关基因, 对培育抗逆水稻新品种具有重大意义。近年来, 随着大规模基因表达水平测量技术的发展, 基于杂交原理的基因芯片技术[2]和基于高通量测序技术的RNA-seq[3], 被众多****用于植物胁迫响应基因的挖掘研究中[4]。
然而, 大多实验组测序样本有限, 仅单一地从与水稻某非生物胁迫相关的单个实验组测序数据来挖掘胁迫相关基因, 结果不稳定, 也很难让人信服[5]。当前公共数据库中积累的大量水稻胁迫相关基因芯片和RNA-seq表达数据, 为多平台数据分析提供了研究空间。研究表明, 融合多平台数据能够提高基因表达分析的准确性和可靠性, 多平台表达数据的整合分析成为水稻非生物胁迫相关基因预测研究的趋势[6]。当前, 多平台基因表达数据的融合通常可分为两类: (1) 基于输出层面融合的元分析法。它通过对多个研究结果进行合并汇总, 增大样本总量, 提高检测准确率和统计分析结果的一致性[7]。(2) 基于原始数据融合的数据转换法。它先通过把不同平台基因表达数据按一定规则转换到同一个数据范围内, 再将转换后的多个平台实验数据直接合并成一个表达数据矩阵, 以此来增加样本数目缓解“高维数、小样本”维数灾难问题[8]。综合考虑单个实验组水稻测序的小样本, 及水稻基因芯片数据与RNA-seq数据之间尺度与维度的差异, 本研究首先对同一胁迫相关的多个基因芯片数据或多个RNA-seq数据分别采取数据转换法融合, 再分别基于融合后的基因芯片表达数据集和RNA-seq表达数据集进行胁迫相关基因挖掘, 最后将二者的结果实施元分析, 获取最终的胁迫响应基因。
为了有效利用多平台基因表达数据, 本文选用加权基因共表达网络分析(Weighted Gene Co-expression Network Analysis, WGCNA)法来挖掘关键基因。WGCNA利用基因表达数据构造协同表达的基因模块, 并根据基因模块与表型的关联性以及基因模块的内连性来鉴定关键基因[9], 其基本假定是“表达模式相似的基因功能相似”。它可将表达模式相似的基因进行聚类, 并分析模块与特定性状或表型之间的关联关系, 因此在作物的干旱胁迫、盐胁迫等非生物胁迫相关基因的挖掘研究中被广泛应用。例如李旭凯等[10]利用WGCNA挖掘到2599个与水稻冷胁迫、干旱胁迫和盐胁迫都相关的基因, 并预测出25个抗逆关键基因; Zhu等[11]通过对转录组数据进行WGCNA分析, 确定了水稻盐胁迫响应核心差异基因和模块; Lv等[12]以转录组数据为基础进行WGCNA分析, 预测了各模块重要的Hub差异基因和调控水稻干旱应答基因表达的主要转录因子; Hopper等[13]使用时间序列转录方法结合WGCNA网络分析, 为葡萄耐旱性研究提供了候选基因; 秦天元等[14]使用WGCNA挖掘马铃薯根系抗旱核心基因, 并进一步利用RT-qPCR验证出挖掘到的核心基因确实响应干旱胁迫。经典的WGCNA 以Pearson相关系数度量2个基因表达量间的线性相似性(记为WGCNA-P), 但无法捕获基因间可能广泛存在的非线性关联。Reshef等[15]****基于信息论中的互信息理论提出了一种可度量两变量非线性相关性的普适性测度最大信息系数(Maximal Information Coefficient, MIC), 论文提出以MIC作为相似性度量替代WGCNA中的Pearson相关系数来构建基因共表达网络(记为WGCNA-MIC), 以捕捉基因间的非线性关联。同时, 考虑到特定线性情形下MIC的统计功效[16]不如Pearson相关系数, 所以本研究对同一数据集分别基于WGCNA-P和WGCNA-MIC两种方法来构建基因共表达网络, 并对各自获取的Hub基因集进行整合分析。
综上, 本研究以多平台水稻非生物胁迫(以干旱和盐胁迫为代表)相关的基因芯片数据和RNA-seq数据为研究对象, 分别以WGCNA-P和WGCNA- MIC挖掘胁迫相关Hub基因, 进而对同一胁迫不同平台数据使用以上2种网络分析法得到的Hub基因进行整合分析, 得到最终的胁迫相关Hub基因集。最后, 从预测性能、基因功能富集分析、文献报道和互作网络分析等多角度解析了Hub基因的生物学意义。
1 材料与方法
1.1 数据的获取及预处理
1.1.1 水稻基因芯片数据的获取及预处理 水稻的基因芯片数据来源于NCBI的GEO (gene expression omnibus)数据库(GPL2025平台)。芯片数据的预处理利用R (v3.5.1)软件完成, 其过程如图1所示。首先利用arrayQualityMetrics包对数据进行质量控制; 然后利用affy包的RMA算法(背景处理、归一化处理、汇总)计算芯片表达水平; 随后再利用biomaRt包[17,18]进行探针号注释, 当多个探针注释到同一基因时, 取多探针表达量的平均值作为该基因表达量。分别合并与干旱胁迫相关的4个数据集GSE6901、GSE21651、GSE23211、GSE26280获62个样本, 与盐胁迫相关的3个数据集GSE6901、GSE14403、GSE16108获32个样本(详见附表1)。用limma包的removeBatchEffect函数去除批次效应, 且对低表达基因进行了过滤用于后续分析。图1
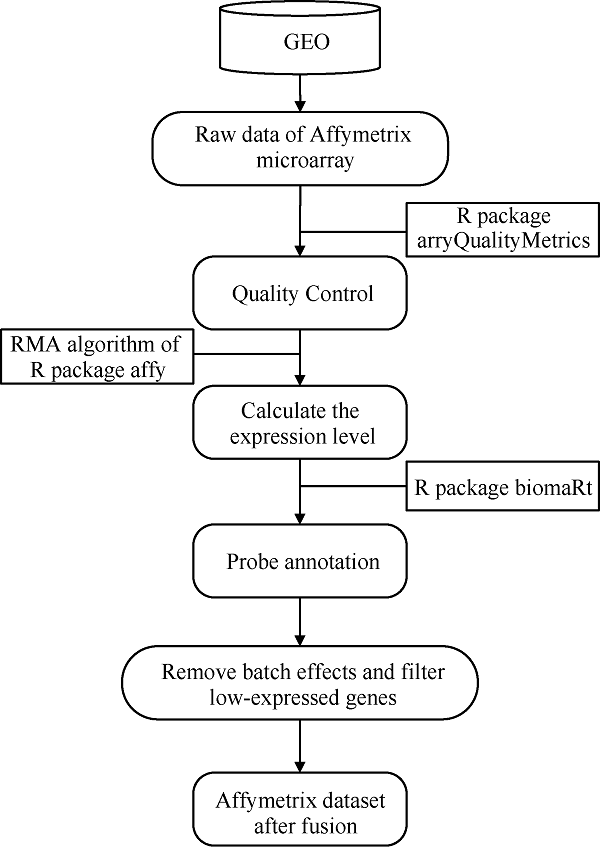
图1水稻affy数据处理流程
Fig. 1Process of rice affy data processing
Table S1
附表1
附表1来源于NCBI的Affymetrix基因芯片数据数据
Table S1
胁迫 Stress | GEO关联 GEO accession | 测序平台 Platform | 样本数(对照组/胁迫组) Samples (control/stress) |
---|---|---|---|
干旱 Drought | GSE6901 | Affymetrix Rice Genome Array (GPL2025) | N = 6 (3/3 drought) |
GSE21651 | Affymetrix Rice Genome Array (GPL2025) | N = 8 (4/4 drought) | |
GSE23211 | Affymetrix Rice Genome Array (GPL2025) | N = 12 (6/6 drought) | |
GSE26280 | Affymetrix Rice Genome Array (GPL2025) | N = 36 (18/18 drought) | |
盐 Salt | GSE6901 | Affymetrix Rice Genome Array (GPL2025) | N = 6 (3/3 salt) |
GSE14403 | Affymetrix Rice Genome Array (GPL2025) | N = 18 (9/9 salt) | |
GSE16108 | Affymetrix Rice Genome Array (GPL2025) | N = 8 (4/4 salt) |
新窗口打开|下载CSV
1.1.2 水稻转录组数据的获取及预处理 水稻转录组RNA-seq数据来源于NCBI的SRA (sequence read archive)数据库(Illumina平台), 干旱胁迫相关有SRR7054176-83、SRR3051740-45、SRR3051752- 57共20个runs, 盐胁迫相关有ERR266221-38、SRR3647326-31共24个runs (选用李旭凯等[10]所用数据, 详见附表2)。数据预处理过程如图2所示。首先利用fasterq-dump (v2.10.7)工具将下载的SRA格式数据转换为fastq格式序列文件, 并利用FastQC (v0.11.9)[19]软件对原始测序数据进行质量评估; 接着利用fastp (0.20.1)[20]软件做质量控制, 得到clean data; 然后根据MSU Rice Genome Annotation Project数据库(
Table S2
附表2
附表2来源于NCBI的RNA-seq数据(RNA-seq)
Table S2
样品编号 Library name | 样品描述 Library description | 样品类型 Library type | 单/双端 Library layout | 水稻品种 Rice genotype |
---|---|---|---|---|
SRR3647331 | 1-leaf_34_day_Salt | RNAseq | Single | Nipponbare |
SRR3647329 | 1-leaf_34_day_Salt | RNAseq | Single | Nipponbare |
SRR3647327 | 1-leaf_34_day_Salt | RNAseq | Single | Nipponbare |
SRR3647330 | 1-leaf_34_day_Control | RNAseq | Single | Nipponbare |
SRR3647328 | 1-leaf_34_day_Control | RNAseq | Single | Nipponbare |
SRR3647326 | 1-leaf_34_day_Control | RNAseq | Single | Nipponbare |
ERR266228 | 1-Seedling_shoots_2_weeks_1h_Control | RNAseq | Single | Nipponbare |
ERR266233 | 1-Seedling_shoots_2_weeks_1h_Control | RNAseq | Single | Nipponbare |
ERR266230 | 1-Seedling_shoots_2_weeks_1h_Control | RNAseq | Single | Nipponbare |
ERR266225 | 1-Seedling_shoots_2_weeks_24h_Control | RNAseq | Single | Nipponbare |
ERR266234 | 1-Seedling_shoots_2_weeks_24h_Control | RNAseq | Single | Nipponbare |
ERR266232 | 1-Seedling_shoots_2_weeks_24h_Control | RNAseq | Single | Nipponbare |
ERR266229 | 1-Seedling_shoots_2_weeks_5h_Control | RNAseq | Single | Nipponbare |
ERR266223 | 1-Seedling_shoots_2_weeks_5h_Control | RNAseq | Single | Nipponbare |
ERR266222 | 1-Seedling_shoots_2_weeks_5h_Control | RNAseq | Single | Nipponbare |
ERR266237 | 1-Seedling_shoots_2_weeks_1h_Salt | RNAseq | Single | Nipponbare |
ERR266236 | 1-Seedling_shoots_2_weeks_1h_Salt | RNAseq | Single | Nipponbare |
ERR266235 | 1-Seedling_shoots_2_weeks_1h_Salt | RNAseq | Single | Nipponbare |
ERR266238 | 1-Seedling_shoots_2_weeks_24h_Salt | RNAseq | Single | Nipponbare |
ERR266226 | 1-Seedling_shoots_2_weeks_24h_Salt | RNAseq | Single | Nipponbare |
ERR266231 | 1-Seedling_shoots_2_weeks_24h_Salt | RNAseq | Single | Nipponbare |
ERR266227 | 1-Seedling_shoots_2_weeks_5h_Salt | RNAseq | Single | Nipponbare |
ERR266224 | 1-Seedling_shoots_2_weeks_5h_Salt | RNAseq | Single | Nipponbare |
ERR266221 | 1-Seedling_shoots_2_weeks_5h_Salt | RNAseq | Single | Nipponbare |
SRR7054183 | 2-Inflorescence_Control | RNAseq | Paired | Nipponbare |
SRR7054182 | 2-Inflorescence_Control | RNAseq | Paired | Nipponbare |
SRR7054181 | 2-Inflorescence_Control | RNAseq | Paired | Nipponbare |
SRR7054180 | 2-Inflorescence_Control | RNAseq | Paired | Nipponbare |
SRR7054179 | 2-Inflorescence_Drought | RNAseq | Paired | Nipponbare |
SRR7054178 | 2-Inflorescence_Drought | RNAseq | Paired | Nipponbare |
SRR7054177 | 2-Inflorescence_Drought | RNAseq | Paired | Nipponbare |
SRR7054176 | 2-Inflorescence_Drought | RNAseq | Paired | Nipponbare |
SRR3051752 | Drought stress_rep1 | RNAseq | Paired | Nipponbare |
SRR3051753 | Drought stress_rep2 | RNAseq | Paired | Nipponbare |
SRR3051754 | Drought stress_rep3 | RNAseq | Paired | Nipponbare |
SRR3051755 | Well-water_rep1 | RNAseq | Paired | Nipponbare |
SRR3051756 | Well-water_rep2 | RNAseq | Paired | Nipponbare |
SRR3051757 | Well-water_rep3 | RNAseq | Paired | Nipponbare |
SRR3051740 | Well-water_rep1 | RNAseq | Paired | Nipponbare |
SRR3051741 | Well-water_rep2 | RNAseq | Paired | Nipponbare |
SRR3051742 | Well-water_rep3 | RNAseq | Paired | Nipponbare |
SRR3051743 | Drought stress_rep1 | RNAseq | Paired | Nipponbare |
SRR3051744 | Drought stress_rep2 | RNAseq | Paired | Nipponbare |
SRR3051745 | Drought stress_rep3 | RNAseq | Paired | Nipponbare |
新窗口打开|下载CSV
图2
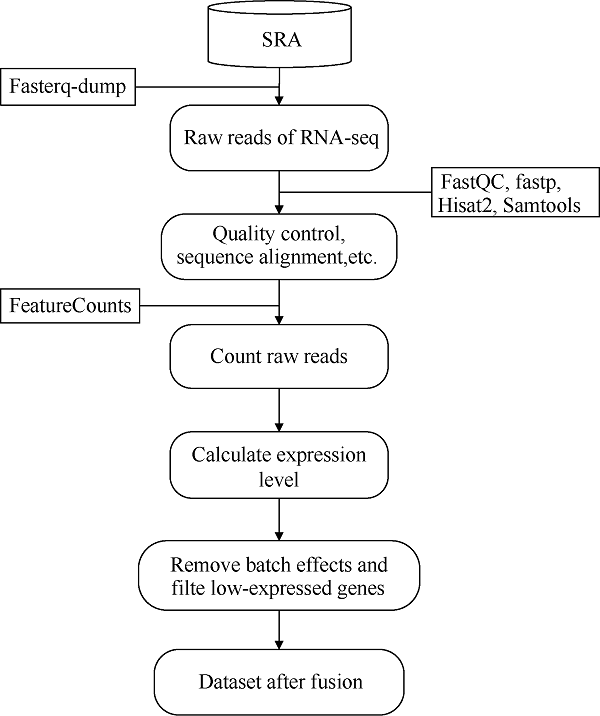
图2水稻RNA-seq数据处理流程
Fig. 2Process of rice RNA-seq data processing
经过上述对同一平台同一胁迫相关的多个数据集的数据融合, 共获4个水稻数据集: 干旱胁迫相关的基因芯片数据集D_affy和RNA-seq数据集D_rnaseq, 盐胁迫相关的基因芯片数据集S_affy和RNA-seq数据集S_rnaseq, 数据详见表1。
Table 1
表1
表1水稻数据集
Table 1
数据集 Data set | 基因数 No. of genes | 总样本数 Total samples | 对照组样本数 Control samples | 胁迫组样本数 Stress samples |
---|---|---|---|---|
干旱芯片数据 Drought stress-related affymetrix dataset (D_affy) | 27,344 | 62 | 31 | 31 |
盐芯片数据 Salt stress-related affymetrix dataset (S_affy) | 27,344 | 32 | 16 | 16 |
干旱RNA-seq数据 Drought stress-related RNA-seq dataset (D_rnaseq) | 29,828 | 20 | 10 | 10 |
盐RNA-seq数据 Salt stress-related RNA-seq dataset (S_rnaseq) | 28,425 | 22 | 11 | 11 |
新窗口打开|下载CSV
1.2 基于WGCNA-P和WGCNA-MIC的共表达网络分析
数据经预处理后仍然包含2万多个基因(表1), 考虑到直接进行共表达网络分析计算量过大, 本研究采用前文提及的最大信息系数MIC进行基因初筛。分别计算各数据中基因与表型之间的MIC值, MIC值越高, 意味着该基因与表型相关性越大, 我们选取MIC值较高的前30%基因用于后续的共表达网络分析。本研究中, 经典的加权基因共表达网络WGCNA-P构建直接利用R语言中的WGCNA包提供的一系列函数实现, 而改进的WGCNA-MIC法则基于WGCNA包中的相关函数自编代码实现(代码见附件)。二者构建的主要步骤如下:
(1)计算相似矩阵${{\left( {{S}_{ij}} \right)}^{\text{unsigned}}}=\left| \text{cor}\left( i,j \right) \right|$基于WGCNA-P方法中相似矩阵中的元素由基因i和基因j之间的Pearson线性相关系数组成; 而基于WGCNA-MIC方法中相似矩阵中的元素由基因i和基因j之间的MIC非线性相关系数组成, 即Sij=MIC(i, j);
(2)定义邻接矩阵${{\text{a}}_{ij}}={{\left| {{S}_{ij}} \right|}^{\beta }}$, 即对相似性进行幂律运算, 且为使得网络中基因间的连接服从无尺度分布, 根据无尺度网络模型指数${{R}^{2}}$选择软阈值$\beta $;
(3)构建拓扑重叠矩阵$\text{TO}{{\text{M}}_{ij}}$;
(4)计算距离矩阵$\text{disTOM}=1-\text{TO}{{\text{M}}_{ij}}$, 构建层次聚类树, 并利用动态剪枝算法获得基因模块, 模块最小基因数设为30。接着对相似模块进行合并, 合并阈值为0.2 (cutHeight=0.2)。
1.3 表型相关基因模块识别
为识别网络中的与表型相关的显著模块, 通常有以下2种方法:(1)计算基因模块特征基因(module eigengenes, MEs)与表型的相关系数, 设为ME, 其中某一模块的第一主成分被定义为该模块的特征基因。
(2)计算模块的显著性系数(module significance, MS), 模块显著性MS是该模块内所有基因的显著性(Gene Significance, GS)的均值[9], GS为基因与表型性状的相关系数绝对值。某模块的ME和MS值越大, 与表型越相关。本研究中, WGCNA-P方法所有涉及相关系数的计算均采用皮尔逊相关系数, 而WGCNA-MIC方法中则均用最大信息系数MIC。综合考虑ME、MS值, 模块数及所选模块的代表性, 本文对模块数小于10的选择1个显著模块、大于等于10且小于15的选择2个显著模块、大于15的选择4个显著模块。
1.4 Hub基因选择
利用网络中连接度高的枢纽节点来确定基因的优先级, 是一种理解和解释网络和整体生物复杂性的简便方法[23]。Hub基因是依据基因与表型性状之间的相关性GS值、基因与其所在模块特征基因间的相关性MM值来选取。对同一胁迫的2个不同平台数据分别基于WGCNA-P和WGCNA-MIC可获得该胁迫相关的4个Hub基因子数据集, 对其进行元分析, 取并集, 可获得该胁迫相关的Hub基因总集。1.5 Hub基因的预测性能
支持向量机(support vector machine, SVM)提供了一种高效分两类或两类以上数据的方法[24], 为验证Hub基因选择的合理性, 本研究基于干旱胁迫和盐胁迫的8个Hub基因子集及最终的2个Hub基因总集依次构建SVM模型对表型进行分类预测。通过5次5折交叉验证进行测试, 最终以平均精度作为最后的预测结果。1.6 Hub基因功能分析
1.6.1 GO富集及文献报道分析 利用AgriGo (1.6.2 蛋白质互作网络构建与分析 利用STRING和Cytoscape工具构建Hub基因的蛋白互作网络。将Hub基因导入STRING (v11.0)[26]蛋白互作
在线分析工具(
2 结果与分析
2.1 WGCNA-P、WGCNA-MIC及显著模块识别
如图3所示, 干旱胁迫基因芯片数据D_affy基于WGCNA-P进行基因共表达网络分析时, 动态剪切得到35个基因模块, 合并后得到23个模块; 基于WGCNA-MIC方法分析, 30个模块合并后得到10个模块。纵坐标的不同颜色代表不同的模块, 各模块与干旱胁迫之间的相关性及模块显著性详见图3, 基于WGCNA-P识别的显著模块及模块内基因数分别为brown (833)、red (383)、darkgrey (106)、purple (260) 四个模块共1582个基因, 而基于WGCNA- MIC识别的模块为darkturquoise (1114)和midnightblue (265)两个模块共1379个基因。图3
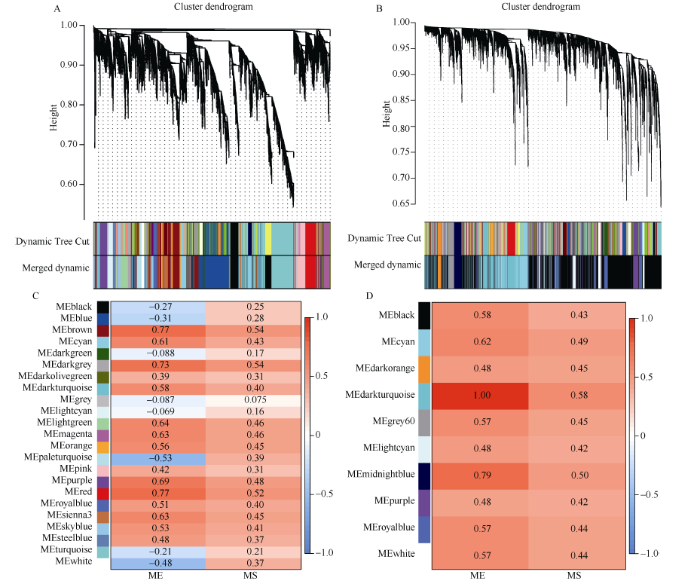
图3D_affy数据WGCNA网络分析结果
A、B: 分别基于WGCNA-P和WGCNA-MIC方法的基因聚类树和模块划分, Dynamic Tree Cut表示由原始计算划分的模块, Merged dynamic表示合并后的结果; C、D: 分别基于WGCNA-P和WGCNA-MIC方法的各模块的ME和MS值, 红色表示模块与干旱胁迫正相关, 蓝色表示模块与干旱胁迫负相关。
Fig. 3WGCNA network analysis of D_affy data
A, B: the gene clustering tree and module division based on WGCNA-P and WGCNA-MIC methods, respectively; the Dynamic Tree Cut represents the module divided by the original calculation, the Merged dynamic represents the merged result; C, D: the ME and MS values of each module based on WGCNA-P and WGCNA-MIC methods, respectively; red block indicates that the module is positively correlated with drought stress, and blue indicates that the module is negatively correlated with drought stress.
如图4所示, 干旱胁迫RNA_seq数据D_rnaseq基于WGCNA-P和WGCNA-MIC方法分别得到13个和7个模块, 且前者分别选取了saddlebrown (983)、darkorange (395)两个模块共1378个基因, 而后者则选取magenta (4089)模块以用于后续分析。
图4
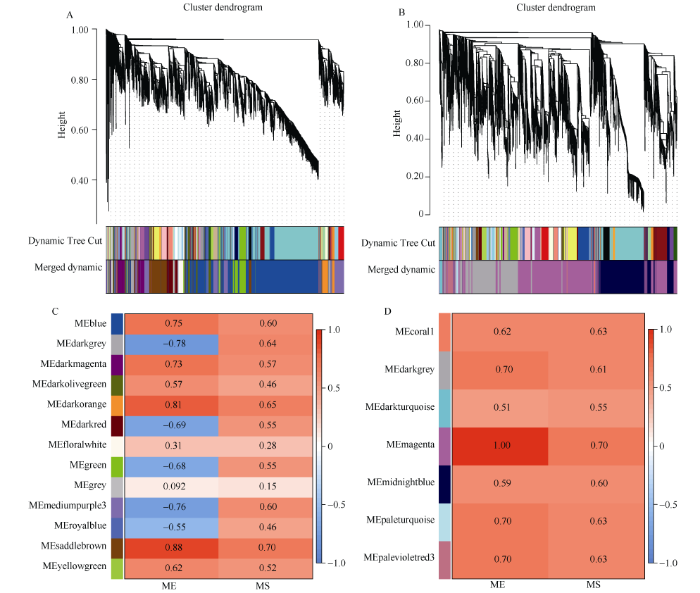
图4D_rnaseq数据WGCNA网络分析结果
A、B: 分别基于WGCNA-P和WGCNA-MIC方法的基因聚类树和模块划分, Dynamic Tree Cut表示由原始计算划分的模块, Merged dynamic表示合并后的结果; C、D: 分别基于WGCNA-P和WGCNA-MIC方法的各模块的ME和MS值, 红色表示模块与干旱胁迫正相关, 蓝色表示模块与干旱胁迫负相关。
Fig. 4WGCNA network analysis of D_rnaseq data
A, B: the gene clustering tree and module division based on WGCNA-P and WGCNA-MIC methods, respectively; the Dynamic Tree Cut represents the module divided by the original calculation, the Merged dynamic represents the merged result; C, D: the ME and MS values of each module based on WGCNA-P and WGCNA-MIC methods, respectively; red block means that the module is positively correlated with drought stress, and blue indicates that the module is negatively correlated with drought stress.
盐胁迫基因芯片数据(附图1), 使用WGCNA-P方法时, 动态剪切得到20个模块, 经合并后得到17个模块; 使用WGCNA-MIC方法时, 43个模块合后得到40个模块。基于WGCNA-P方法最终选取的模块及模块内基因数分别magenta (213)、red (331)、purple (199)、pink (803)四个模块共1546个基因, 而基于WGCNA-MIC方法则分别选取了turquoise (2818)、darkturquoise (74)、red (210)和brown (409)四个模块共3511个基因以用于后续分析。盐胁迫的RNA-seq数据(附图2), 基于WGCNA-P和WGCNA- MIC网络最终分别得到27个和19个模块, 且前者分别选取了brown (1148)、plum1 (73)、darkgreen (183)、magenta (351)四个模块共1755个基因, 而后者则分别选取了pink (308)、lightcyan (42)、darkgreen (130)和darkturquoise (193)四个模块共673个基因以用于后续分析。
附图1
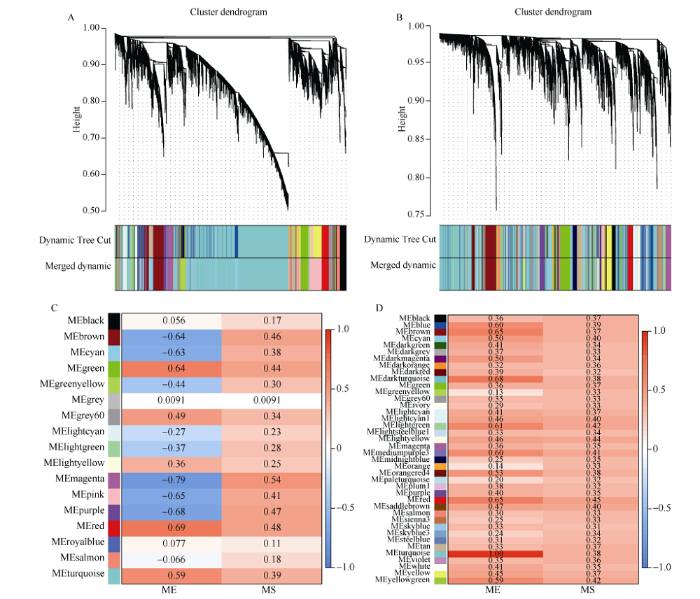
附图1S_affy数据WGCNA网络分析结果
A、B: 分别基于WGCNA-P和WGCNA-MIC方法的基因聚类树和模块划分, Dynamic Tree Cut表示由原始计算划分的模块, Merged dynamic表示合并后的结果; C、D: 分别基于WGCNA-P和WGCNA-MIC方法的各模块的ME和MS值, 红色表示模块与盐胁迫正相关, 蓝色表示模块与盐胁迫负相关。
Fig. S1WGCNA network analysis of S_affy
A, B: gene clustering tree and module division of based on WGCNA-P and WGCNA-MIC methods respectively; the Dynamic Tree Cut represents the module divided by the original calculation, the merged dynamic represents the merged result; C, D: the ME and MS values of each module based on WGCNA-P and WGCNA-MIC methods respectively; red indicates that the module is positively correlated with salt stress, and blue indicates that the module is negatively correlated with salt stress.
附图2
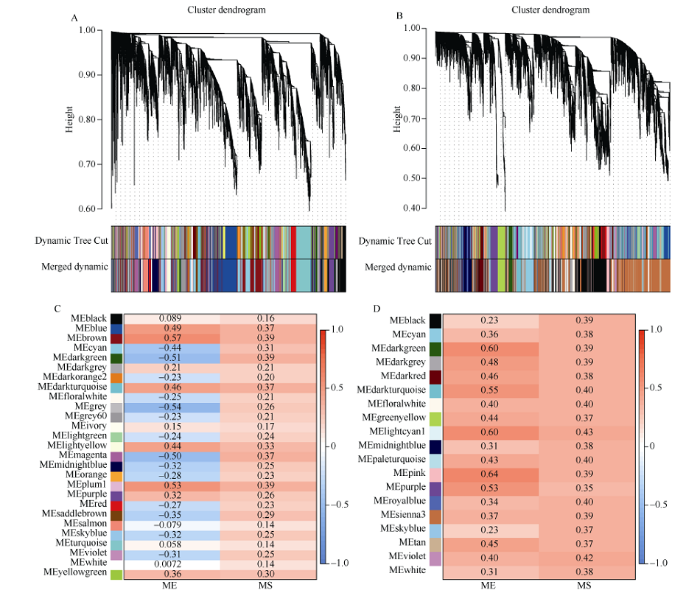
附图2S_rnaseq数据WGCNA网络分析结果
A、B: 分别基于WGCNA-P和WGCNA-MIC法的基因聚类树和模块划分, Dynamic Tree Cut表示由原始计算划分的模块, Merged dynamic表示合并后的结果; C、D: 分别基于WGCNA-P和WGCNA-MIC法的各模块的ME和MS值, 红色表示模块与盐胁迫正相关, 蓝色表示模块与盐胁迫负相关。
Fig. S2WGCNA network analysis of S_rnaseq
A, B: gene clustering tree and module division of based on WGCNA-P and WGCNA-MIC methods respectively; the Dynamic Tree Cut represents the module divided by the original calculation, the merged dynamic represents the merged result; C, D: the ME and MS values of each module based on WGCNA-P and WGCNA-MIC methods respectively; red indicates that the module is positively correlated with salt stress, and blue indicates that the module is negatively correlated with salt stress.
2.2 Hub基因的预测性能
本研究中, 干旱胁迫相关Hub基因挑选阈值设为GS>0.4且MM>0.83, 盐胁迫相关Hub基因筛选阈值设为GS>0.3且MM>0.75。4个数据集分别基于2种网络分析方法共获得8个Hub基因子集(D_affy_ P、D_affy_MIC、D_rnaseq_P、D_rnaseq_MIC和S_affy_P、S_affy_MIC、S_rnaseq_P、S_rnaseq_MIC), 对基因子集元分析后得到干旱胁迫相关Hub基因总集D_meta_hub和盐胁迫相关Hub基因总集S_meta_ hub。基于各Hub基因集对表型的SVM分类精度如表2所示, Hub基因的预测性能整体表现优异, 其中, 基于WGCNA-MIC方法获取的Hub基因, 较之基于WGCNA-P方法获取的Hub基因预测精度略高, 元分析后的Hub基因总集D_meta_hub和S_meta_hub, 在各数据集上的平均预测精度比各Hub基因子集的精度略高。结果表明, Hub基因与表型性状相关性强, WGCNA-MIC方法和元分析有效。Table 2
表2
表2Hub基因的分类精度
Table 2
胁迫 Stress | Hub基因集 Hub gene set | 基因数目 Number of genes | 数据集 Data set | 平均精度 Average accuracy (%) |
---|---|---|---|---|
干旱Drought | D_affy_P | 220 | D_affy | 100 |
D_affy_MIC | 104 | D_affy | 100 | |
D_rnaseq_P | 738 | D_rnaseq | 90.0 | |
D_rnaseq_MIC | 1634 | D_rnaseq | 91.0 | |
D_meta_hub | 1936 | D_affy | 100 | |
D_rnaseq | 96.0 | |||
盐Salt | S_affy_P | 470 | S_affy | 100 |
S_affy_MIC | 293 | S_affy | 100 | |
S_rnaseq_P | 684 | S_rnaseq | 81.0 | |
S_rnaseq_MIC | 331 | S_rnaseq | 84.6 | |
S_meta_hub | 1504 | S_affy | 100 | |
S_rnaseq | 84.6 |
新窗口打开|下载CSV
2.3 GO功能富集分析
利用AgriGO在线功能富集分析工具, 分别对干旱/盐胁迫相关Hub基因集进行基因功能富集分析, 在生物学过程(biological process, BP)、分子功能(molecular function, MF)和细胞组分(cellular component, CC)三大分类中都显著富集到了多个相关GO通路。具体富集结果如表3所示。干旱胁迫相关富集结果显示, 生物学过程中, 显著富集到的通路, 包括应对刺激的通路: 内源性刺激响应(GO:0009719)、激素刺激响应(GO:0009725)和非生物刺激响应(GO:0009628)等; 参与特殊代谢物代谢过程的通路: 萜类化合物代谢过程(GO:0006721)等; 与干旱胁迫较为直接相关的通路: 对水的响应(GO:0009415)和渗透胁迫响应(GO:0006970)等。分子功能中, 显著富集到了与信号传导相关的通路: 受体活性(GO:0004872)、翻译因子活性与核酸结合(GO:0008135)等; 一些参与调控某些蛋白质酶相关的通路: 蛋白质酪氨酸激酶活性(GO:0004713)等。另外, 还有不少显著富集到细胞组分相关的通路: 薄膜(GO:0016020)等。盐胁迫相关富集结果显示, 生物学过程中, 显著富集的通路, 包括参与各种物质代谢过程的通路: 草酸代谢过程(GO:0043436)和有机酸代谢过程(GO:0006082)等; 响应胁迫相关的功能: 内源性激素的响应(GO:0009719)等; 参与光合作用: 光刺激响应(GO:0009416)等。分子功能中, 最显著富集到的通路是受体活性(GO:0004872)。细胞组分中富集到了很多与膜组分相关参与渗透作用的通路, 如薄膜(GO:0016020)和细胞质膜等(GO:0005886); 参与光合作用的组件: 叶绿体(GO:0009507)等。Table 3
表3
表3Hub基因的GO富集部分分析结果
Table 3
胁迫 Stress | GO条目 GO term | 基因数目 Number of genes | 基因本体 Ontology | 描述 Description | P值 P-value |
---|---|---|---|---|---|
干旱 Drought | GO:0010033 | 12 | BP | 对有机物的响应 Response to organic substance | 9.00E-06 |
GO:0009719 | 12 | BP | 内源性刺激响应 Response to endogenous stimulus | 9.00E-06 | |
GO:0009725 | 12 | BP | 激素刺激响应 Response to hormone stimulus | 9.00E-06 | |
GO:0006721 | 8 | BP | 萜类化合物代谢过程 Terpenoid metabolic process | 2.80E-05 | |
GO:0007165 | 37 | BP | 信号传导Signal transduction | 2.20E-05 | |
GO:0009628 | 15 | BP | 对非生物刺激的响应 Response to abiotic stimulus | 0.00054 | |
GO:0006970 | 5 | BP | 渗透胁迫响应 Response to osmotic stress | 0.0036 | |
GO:0009415 | 5 | BP | 对水的响应Response to water | 0.006 | |
GO:0004872 | 22 | MF | 受体活性 Receptor activity | 9.40E-13 | |
GO:0004713 | 21 | MF | 蛋白质酪氨酸激酶活性 Protein tyrosine kinase activity | 9.00E-12 | |
GO:0004722 | 15 | MF | 蛋白丝氨酸/苏氨酸磷酸酶活性 Protein serine/threonine phosphatase activity | 2.70E-05 | |
GO:0008135 | 12 | MF | 翻译因子活性, 核酸结合 Translation factor activity, Nucleic acid binding | 0.0099 | |
GO:0044424 | 1120 | CC | 细胞内成分 Intracellular part | 0 | |
GO:0005737 | 1011 | CC | 细胞质Cytoplasm | 0 | |
GO:0016020 | 278 | CC | 薄膜 Membrane | 3.10E-23 | |
盐 Salt | GO:0070887 | 6 | BP | 细胞对化学刺激的响应 Cellular response to chemical stimulus | 2.00E-06 |
GO:0007275 | 13 | BP | 多细胞有机体的发育 Multicellular organismal development | 5.30E-08 | |
GO:0043436 | 52 | BP | 草酸代谢过程 Oxoacid metabolic process | 4.80E-06 | |
GO:0019752 | 52 | BP | 羧酸代谢过程 Carboxylic acid metabolic process | 4.80E-06 | |
GO:0006082 | 52 | BP | 有机酸代谢过程 Organic acid metabolic process | 5.10E-06 | |
GO:0010033 | 11 | BP | 对有机物的响应 Response to organic substance | 4.70E-06 | |
GO:0009719 | 11 | BP | 内源性刺激响应 Response to endogenous stimulus | 4.70E-06 | |
GO:0009416 | 6 | BP | 光刺激响应 Response to light stimulus | 0.024 | |
GO:0004872 | 14 | MF | 受体活性 Receptor activity | 5.70E-08 | |
GO:0008135 | 18 | MF | 翻译因子活性, 核酸结合 Translation factor activity, nucleic acid binding | 1.00E-06 | |
GO:0003743 | 11 | MF | 翻译起始因子活性 Translation initiation factor activity | 3.00E-05 | |
GO:0016874 | 32 | MF | 连接酶活性 Ligase activity | 0.00064 | |
GO:0016020 | 225 | CC | 薄膜 Membrane | 4.60E-21 | |
GO:0009507 | 20 | CC | 叶绿体 Chloroplast | 4.30E-15 | |
GO:0005886 | 18 | CC | 细胞质膜 Plasma membrane | 1.20E-09 |
新窗口打开|下载CSV
综上, 基于元分析获取的2种胁迫的Hub基因, 均富集到了内源性刺激响应(GO:0009719)、激素刺激响应(GO:0009725)和非生物刺激响应(GO:0009628)等胁迫响应的相关通路上。
2.4 文献报道情况分析
为了验证研究结果的可靠性, 根据从国家水稻数据中心获取到的已报道干旱和盐胁迫相关基因分析所选Hub基因的文献报道情况。本研究所选Hub基因中有已报道干旱胁迫相关基因31个和盐胁迫相关基因22个, 如表4所示。Table 4
表4
表4已报道与胁迫相关的Hub基因
Table 4
胁迫 Stress | 基因编号RAP_locus | 基因符号 Gene symbol | 胁迫 Stress | 基因编号 RAP_locus | 基因符号 Gene symbol |
---|---|---|---|---|---|
干旱 Drought | Os05g0455500 | OsP5CS; OsP5CS1; OsALDH18B1 | 干旱 Drought | Os03g0286900 | OsRCI2-5 |
Os02g0766700 | OsbZIP23 | Os02g0149800 | OsPP18 | ||
Os08g0112700 | OsMADS26 | Os03g0267000 | OsHSP18.0-CI; OsMSR3; OsSHSP1 | ||
Os06g0130100 | OsSIK1 | Os05g0475400 | OsAMTR1 | ||
Os09g0552300 | OsRPK1 | Os08g0408500 | OsERF48; OsDRAP1 | ||
Os01g0867300 | OsABF1; OsbZIP12 | Os04g0676700 | OsMYB6 | ||
Os03g0125100 | DSM2 | Os12g0597500 | OsUAH | ||
Os03g0745000 | OsHsfA2a | Os06g0316000 | Os2H16 | ||
Os05g0542500 | OsLEA3; OsLEA3-1 | Os06g0612800 | OsiSAP8 | ||
Os02g0671100 | MAIF1 | Os04g0572400 | OsDREB1E | ||
Os06g0211200 | OsAREB1; OsbZIP46; OsABF2; ABL1 | Os11g0126900 | OsNAC10; ONAC122 | ||
Os05g0437700 | EDT1; OsbZIP40 | Os11g0707600 | OsGL1-11 | ||
Os05g0569300 | OsbZIP45 | Os03g0805100 | SQS | ||
Os08g0196700 | OsNF-YA7; OsHAP2A | Os05g0213500 | OsPYL/RCAR5; OsPYL5; OsPYL11 | ||
Os03g0230300 | OsSRO1c; BOC1 | Os06g0598800 | WSL1 | ||
Os04g0541700 | Oshox22 | ||||
盐Salt | Os03g0348900 | OsSRFP1; SDEL2 | 盐Salt | Os02g0121300 | OsCYP2; LRT2 |
Os04g0652400 | OsSULTR3; 3;lpa | Os03g0272300 | OsSDIR1 | ||
Os03g0329900 | OsPHR1 | Os07g0129200 | OsPR1a; OsSCP | ||
Os07g0187700 | OsPHF1 | Os05g0437700 | EDT1; OsbZIP40 | ||
Os02g0678200 | OsSPX-MFS2; OsPSS2 | Os08g0557000 | OsPIMT1 | ||
Os02g0325600 | NIGT1 | Os06g0693700 | OsSIDP366 | ||
Os01g0755700 | NBIP1 | Os04g0676700 | OsMYB6 | ||
Os10g0545700 | OsACR2.1 | Os01g0612700 | OsLOL2; OsLOL5 | ||
Os01g0869900 | OsSAPK4; OSPDK | Os01g0948400 | OsP5CR | ||
Os03g0719900 | OsPTR8; OsNPF8.5 | Os03g0319300 | OsCam1-1 | ||
Os09g0434500 | OsBIERF1 | Os05g0584200 | OsLEA5 |
新窗口打开|下载CSV
2.5 Hub基因互作网络构建
利用在线分析工具STRING和Cytoscape软件挖掘Hub基因总集中蛋白互作关系, 重点关注与已报道胁迫相关的Hub基因互作情况。Hub基因总集中, 与2个及2个以上已报道Hub基因有较强关系, 即网络中节点度≥2且STRING中的combined_score≥0.9的Hub基因考虑作为胁迫候选基因可被进一步挖掘。如图5和图6, 图中红色节点表示前文得到的已报道胁迫相关Hub基因(不包括STRING库中未匹配到蛋白质的基因和无相关蛋白的基因), 节点越大, 表示与之相关的基因越多, 线条越粗且颜色越暗, 表示基因之间关系越强。最终找到了与已报道Hub基因存在蛋白互作关系的干旱胁迫候选基因11个(图5中橙色节点), 盐胁迫候选基因5个(图6中橙色节点), 详见表5。图5
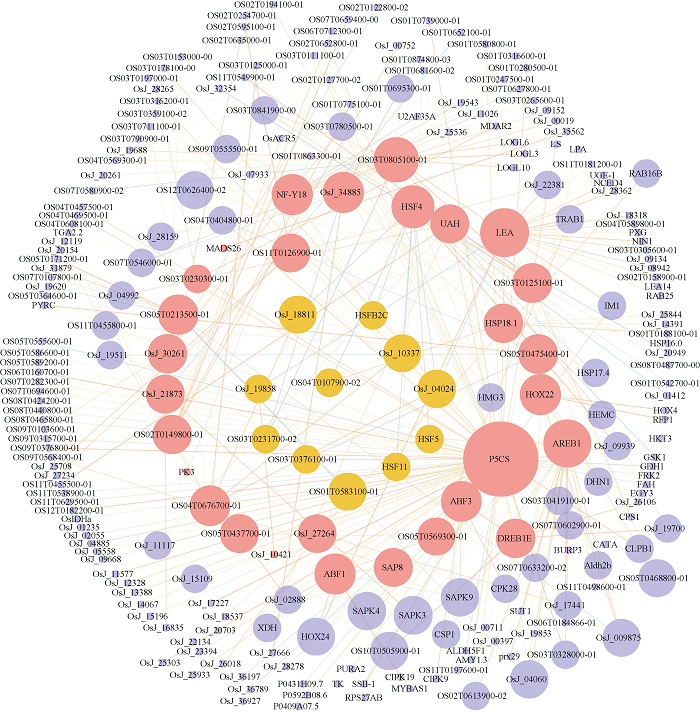
图5干旱胁迫相关基因互作网络
Fig. 5Gene interaction network of drought stress
图6
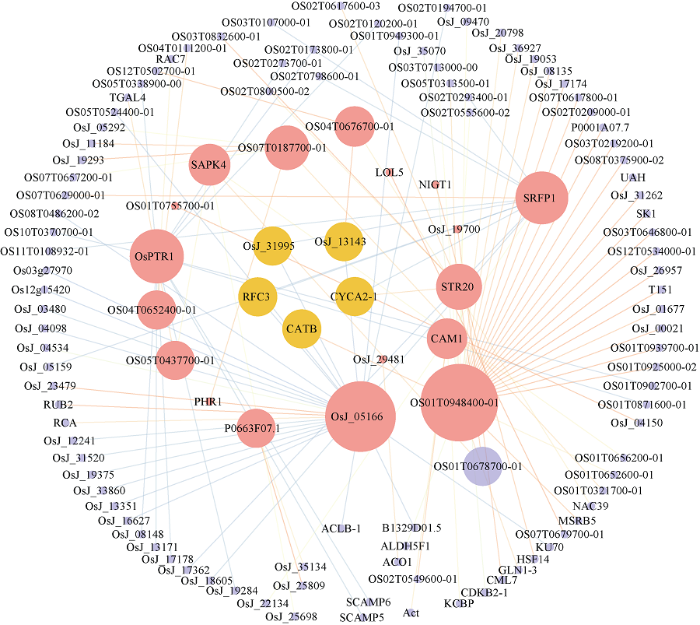
图6盐胁迫相关基因互作网络
Fig. 6Gene interaction network of salt stress
Table 5
表5
表5候选基因在STRING中的注释
Table 5
胁迫 Stress | 候选基因 Candidate gene | STRING中名称 Name in STRING | 注释 Annotation |
---|---|---|---|
干旱Drought | Os01g0733200 | HSF11 | 热应激转录因子C-1b; 与热休克启动子元件(HSE)的DNA特异性结合的转录调控因子。 Heat stress transcription factor C-1b; transcriptional regulator that specifically binds DNA of heat shock promoter elements (HSE). |
Os07g0178600 | HSF5 | 热应激转录因子A-2b; 转录调控因子, 可特异性结合热休克启动子元件(HSE)的DNA; 属于HSF家族, A类亚科。 Heat stress transcription factor A-2b; transcriptional regulator that specifically binds DNA of heat shock promoter elements (HSE); belongs to the HSF family. Class A subfamily. | |
Os09g0526600 | HSFB2C | 热应激转录因子B-2c; 转录调控因子, 可特异性结合热休克启动子元件(HSE)的DNA; 属于HSF家族, B类亚科。 Heat stress transcription factor B-2c; transcriptional regulator that specifically binds DNA of heat shock promoter elements (HSE); belongs to the HSF family. Class B subfamily. | |
Os01g0583100 | OS01T0583100-01 | 可能的蛋白质磷酸酶2C 6; 属于PP2C家族。 Probable protein phosphatase 2C 6; belongs to the PP2C family. | |
Os03g0231700 | OS03T0231700-02 | Os03g0231700蛋白; 角鲨烯单加氧酶, 假定表达; cDNA克隆: J033045D18, 完整插入序列。 Os03g0231700 protein; Squalene monooxygenase, putative, expressed; cDNA clone: J033045D18, full insert sequence. | |
Os03g0376100 | OS03T0376100-01 | Os03g0376100蛋白。 Os03g0376100 protein. | |
胁迫 Stress | 候选基因 Candidate gene | STRING中名称 Name in STRING | 注释 Annotation |
Os04g0107900 | OS04T0107900-02 | Os04g0107900蛋白。 Os04g0107900 protein. | |
Os01g0840100 | OsJ_04024 | cDNA克隆: J100050G20, 完整插入序列; 70 kD热激蛋白; Os01g0840100蛋白; 假定的HSP70; 未表征的蛋白质。 cDNA, clone: J100050G20, full insert sequence; 70 kD heat shock protein; Os01g0840100 protein; Putative HSP70; uncharacterized protein. | |
Os03g0277300 | OsJ_10337 | 热休克同源70 kD蛋白, 假定表达。 Heat shock cognate 70 kD protein, putative, expressed. | |
Os05g0460000 | OsJ_18811 | Os05g0460000蛋白; 推定的hsp70; cDNA克隆: J090096I11, 完整插入序列; 属于热休克蛋白70家族。 Os05g0460000 protein; Putative hsp70; cDNA, clone: J090096I11, full insert sequence; Belongs to the heat shock protein 70 family. | |
Os06g0110200 | OsJ_19858 | cDNA克隆: 002-135-D09, 完整插入序列; Os06g0110200蛋白; 假定的未表征蛋白OSJNBa0004I20.22; 假定的未表征蛋白P0514G12.46。 cDNA clone: 002-135-D09, full insert sequence; Os06g0110200 protein; putative uncharacterized protein OSJNBa0004I20.22; putative uncharacterized protein P0514G12.46. | |
盐 Salt | Os06g0727200 | CATB | 过氧化氢酶同工酶B; 几乎发生在所有有氧呼吸的生物中, 并保护细胞免受过氧化氢的毒性作用。 Catalase isozyme B; occurs in almost all aerobically respiring organisms and serves to protect cells from the toxic effects of hydrogen peroxide. |
Os12g0502300 | CYCA2-1 | Cyclin-A2-1; 属于细胞周期蛋白家族。Cyclin AB亚家族。 Cyclin-A2-1; belongs to the cyclin family. Cyclin AB subfamily. | |
Os03g0821100 | OsJ_13143 | 热休克同源70 kD蛋白2, 假定表达; 热休克蛋白同源物70; Os03g0821100蛋白; cDNA克隆: J023030D03, 完整插入序列。 Heat shock cognate 70 kD protein 2, putative, expressed; heat shock protein cognate 70; Os03g0821100 protein; cDNA clone:J023030D03, full insert sequence. | |
Os10g0491801 | OsJ_31995 | 假定泛素/核糖体蛋白S27a融合蛋白;泛素融合蛋白, 假定表达。 Putative ubiquitin/ribosomal protein S27a fusion protein; ubiquitin fusion protein, putative, expressed. | |
Os02g0775200 | RFC3 | 复制因子C亚基3; 可能参与DNA复制, 从而调节细胞增殖。 Replication factor C subunit 3; may be involved in DNA replication and thus regulate cell proliferation. |
新窗口打开|下载CSV
3 讨论
植物为应对干旱胁迫环境, 在生化、细胞和分子等水平上进化出了很多机制[28], 需要改变基因表达来激活促进耐旱性的代谢过程, 这包括特殊代谢物的合成与积累, 并涉及到物种和基因型特异性的酚类化合物、类黄酮、萜类化合物和含氮化合物的产生[29]。盐胁迫威胁作物生长主要体现在渗透和氧化2个方面, 这不仅会导致叶片脱落、根芽坏死等不良症状的发生, 而且潜在地延迟了光合作用、植物激素功能、代谢途径和基因/蛋白质功能等生理活动[30]。本研究以水稻干旱和盐胁迫相关的Affymetrix基因芯片和RNA-seq两种不同平台的数据为研究对象, 基于WGCNA-P和WGCNA-MIC对其进行了胁迫相关Hub基因的挖掘。从Hub基因预测性能来看, 各Hub基因集的预测精度均达80%以上, 预测性能整体较好。从GO富集分析和文献报道来看, 一方面2种胁迫的Hub基因集都富集到了内源性刺激响应(GO:0009719)、激素刺激响应(GO:0009725)和非生物刺激响应(GO:0009628)等干旱和盐响应相关通路; 另一方面也找到了一些已报道的干旱/盐胁迫相关Hub基因, EDT1 (Os05g0437700)和OsMYB6 (Os04g0 676700)既是已报道干旱胁迫相关Hub基因, 又是已报道盐胁迫相关Hub基因。通过总结部分已报道基因的文献发现, 某个胁迫可能由多个基因协同互作参与调控, 某个基因也可能参与了多个非生物胁迫。比如, 31个已报道干旱胁迫响应基因中, 包括OsP5CS (Os05g0455500)[31]的表达受高盐、干旱、冷胁迫和ABA处理的诱导; OsMADS26 (Os08g0112 700)[32]是水稻响应多种胁迫的调控中心; OsbZIP23 (Os02g0766700)[33]增强了水稻的抗旱耐盐性和对ABA的敏感性; OsSIK1 (Os06g0130100)[34]在水稻耐盐和耐旱过程中起重要作用; OsRPK1 (Os09g0552 300)[35]在盐胁迫下表达水平增加, 其表达也受寒冷、干旱与脱落酸等因素的诱导。22个已报道盐胁迫响应基因中, 包括OsSRFP1 (Os03g0348 900)[36]负向调控水稻的耐盐性和耐低温性; 干旱和盐处理诱导OsPTR8 (Os03g0719900)[37]的表达上调; OsSCP (Os07g0129200)[38]在非生物胁迫应答中通过调控胁迫应答基因而发挥作用; 冷胁迫和盐胁迫会导致OsPIMT1 (Os08g0557000)[39]表达量增加2倍; OsLEA5 (Os05g0584200)[40]与多种非生物胁迫抗性相关等。同时, 与李旭凯等[10]、Zhu等[11]和Lv等[12]的研究结果相比, 我们挖掘到的Hub基因中包含了部分已被广泛报道的水稻干旱胁迫和盐胁迫响应相关的转录因子, 如干旱胁迫相关的bZIP转录因子家族(Os02g0766700、Os06g0211200、Os05g0569300)、MYB转录因子家族(Os04g0676700)、NAC转录因子家族(Os11g0126900)和HSF转录因子家族(Os03g0745000)等; 盐胁迫相关的bZIP转录因子家族(Os05g0437700)和MYB转录因子家族(Os04g06 76700)等。最后, 通过分析Hub基因总集中已报道与胁迫相关的Hub基因及其相关基因之间的互作网络, 进一步挖掘到了与干旱或盐胁迫相关较为紧密的候选基因。
综上, 利用元分析的思路对水稻多平台基因表达数据进行整合分析, 可挖掘到水稻干旱和盐胁迫的关键基因, 对农作物非生物胁迫响应的基因挖掘具有一定的参考价值。STRING分析时, 参数阈值的设置不同, 所获候选基因的数量也会有所不同, 本研究中利用combined_score≥0.9获得的候选基因, 可根据实际情况适当调整阈值, 并有待进一步利用实时荧光定量PCR (RT-qPCR)验证。
4 结论
对多平台数据, 通过加权基因共表达网络分析、元分析和蛋白互作网络分析, 最终获得水稻干旱胁迫和盐胁迫相关的Hub基因分别为1936个和1504个, 其中文献已报道的干旱胁迫和盐胁迫相关Hub基因分别是31个和22个, 预测得到的干旱胁迫和盐胁迫候选基因分别是11个和5个。水稻其他非生物胁迫(如冷胁迫、高温胁迫等)多平台数据数据结构及其实验原理与干旱和盐胁迫类似, 故此方法可推广至其他非生物胁迫相关基因挖掘。本研究为充分利用多平台数据挖掘水稻非生物胁迫相关基因提供了新的思路, 也为进一步研究抗逆性水稻品种提供了参考。参考文献 原文顺序
文献年度倒序
文中引用次数倒序
被引期刊影响因子
[本文引用: 1]
[本文引用: 1]
DOIURL [本文引用: 1]
DOIURL [本文引用: 1]
[本文引用: 1]
[本文引用: 1]
DOIURL [本文引用: 1]
[本文引用: 1]
[本文引用: 1]
DOIURL [本文引用: 2]
[本文引用: 3]
[本文引用: 3]
DOIURL [本文引用: 2]
DOIURL [本文引用: 2]
DOIURL [本文引用: 1]
[本文引用: 1]
[本文引用: 1]
DOIPMID [本文引用: 1]

Identifying interesting relationships between pairs of variables in large data sets is increasingly important. Here, we present a measure of dependence for two-variable relationships: the maximal information coefficient (MIC). MIC captures a wide range of associations both functional and not, and for functional relationships provides a score that roughly equals the coefficient of determination (R(2)) of the data relative to the regression function. MIC belongs to a larger class of maximal information-based nonparametric exploration (MINE) statistics for identifying and classifying relationships. We apply MIC and MINE to data sets in global health, gene expression, major-league baseball, and the human gut microbiota and identify known and novel relationships.
[本文引用: 1]
DOIURL [本文引用: 1]
PMID [本文引用: 1]

biomaRt is a new Bioconductor package that integrates BioMart data resources with data analysis software in Bioconductor. It can annotate a wide range of gene or gene product identifiers (e.g. Entrez-Gene and Affymetrix probe identifiers) with information such as gene symbol, chromosomal coordinates, Gene Ontology and OMIM annotation. Furthermore biomaRt enables retrieval of genomic sequences and single nucleotide polymorphism information, which can be used in data analysis. Fast and up-to-date data retrieval is possible as the package executes direct SQL queries to the BioMart databases (e.g. Ensembl). The biomaRt package provides a tight integration of large, public or locally installed BioMart databases with data analysis in Bioconductor creating a powerful environment for biological data mining.
[本文引用: 1]
DOIURL [本文引用: 1]
DOIURL [本文引用: 1]
[本文引用: 1]
DOIURL [本文引用: 1]
DOIPMID [本文引用: 1]

Abiotic and biotic stress responses are traditionally thought to be regulated by discrete signaling mechanisms. Recent experimental evidence revealed a more complex picture where these mechanisms are highly entangled and can have synergistic and antagonistic effects on each other. In this study, we identified shared stress-responsive genes between abiotic and biotic stresses in rice (Oryza sativa) by performing meta-analyses of microarray studies. About 70% of the 1,377 common differentially expressed genes showed conserved expression status, and the majority of the rest were down-regulated in abiotic stresses and up-regulated in biotic stresses. Using dimension reduction techniques, principal component analysis, and partial least squares discriminant analysis, we were able to segregate abiotic and biotic stresses into separate entities. The supervised machine learning model, recursive-support vector machine, could classify abiotic and biotic stresses with 100% accuracy using a subset of differentially expressed genes. Furthermore, using a random forests decision tree model, eight out of 10 stress conditions were classified with high accuracy. Comparison of genes contributing most to the accurate classification by partial least squares discriminant analysis, recursive-support vector machine, and random forests revealed 196 common genes with a dynamic range of expression levels in multiple stresses. Functional enrichment and coexpression network analysis revealed the different roles of transcription factors and genes responding to phytohormones or modulating hormone levels in the regulation of stress responses. We envisage the top-ranked genes identified in this study, which highly discriminate abiotic and biotic stresses, as key components to further our understanding of the inherently complex nature of multiple stress responses in plants.
DOIURL [本文引用: 1]
DOI [本文引用: 1]

Proteins and their functional interactions form the backbone of the cellular machinery. Their connectivity network needs to be considered for the full understanding of biological phenomena, but the available information on protein-protein associations is incomplete and exhibits varying levels of annotation granularity and reliability. The STRING database aims to collect, score and integrate all publicly available sources of protein-protein interaction information, and to complement these with computational predictions. Its goal is to achieve a comprehensive and objective global network, including direct (physical) as well as indirect (functional) interactions. The latest version of STRING (11.0) more than doubles the number of organisms it covers, to 5090. The most important new feature is an option to upload entire, genome-wide datasets as input, allowing users to visualize subsets as interaction networks and to perform gene-set enrichment analysis on the entire input. For the enrichment analysis, STRING implements well-known classification systems such as Gene Ontology and KEGG, but also offers additional, new classification systems based on high-throughput text-mining as well as on a hierarchical clustering of the association network itself. The STRING resource is available online at https://string-db.org/.
DOIURL [本文引用: 1]
[本文引用: 1]
[本文引用: 1]
[本文引用: 1]
DOIURL [本文引用: 1]
DOIURL [本文引用: 1]
[本文引用: 1]
DOIPMID [本文引用: 1]

The OsbZIP23 transcription factor has been characterized for its essential role in drought resistance in rice (Oryza sativa), but the mechanism is unknown. In this study, we first investigated the transcriptional activation of OsbZIP23. A homolog of SnRK2 protein kinase (SAPK2) was found to interact with and phosphorylate OsbZIP23 for its transcriptional activation. SAPK2 also interacted with OsPP2C49, an ABI1 homolog, which deactivated the SAPK2 to inhibit the transcriptional activation activity of OsbZIP23. Next, we performed genome-wide identification of OsbZIP23 targets by immunoprecipitation sequencing and RNA sequencing analyses in the OsbZIP23-overexpression, osbzip23 mutant, and wild-type rice under normal and drought stress conditions. OsbZIP23 directly regulates a large number of reported genes that function in stress response, hormone signaling, and developmental processes. Among these targets, we found that OsbZIP23 could positively regulate OsPP2C49, and overexpression of OsPP2C49 in rice resulted in significantly decreased sensitivity of the abscisic acid (ABA) response and rapid dehydration. Moreover, OsNCED4 (9-cis-epoxycarotenoid dioxygenase4), a key gene in ABA biosynthesis, was also positively regulated by OsbZIP23. Together, our results suggest that OsbZIP23 acts as a central regulator in ABA signaling and biosynthesis, and drought resistance in rice.© 2016 American Society of Plant Biologists. All Rights Reserved.
DOIURL [本文引用: 1]
DOIURL [本文引用: 1]
DOIURL [本文引用: 1]
DOIURL [本文引用: 1]
[本文引用: 1]
DOIURL [本文引用: 1]
DOIURL [本文引用: 1]