
1 江西师范大学心理学院, 南昌 330022
2 江西师范大学计算机信息工程学院, 南昌 330022
收稿日期:
2019-10-14出版日期:
2020-12-25发布日期:
2020-10-26通讯作者:
涂冬波E-mail:tudongbo@aliyun.com基金资助:
* 国家自然科学基金(61967009);国家自然科学基金(31660278);国家自然科学基金(31760288);国家自然科学基金(31960186);江西省教育厅科学技术研究项目(GJJ150356);江西省教育厅科学技术研究项目(GJJ160282)A new dual-objective CD-CAT item selection method based on the Gini index
LUO Fen1,2, WANG Xiaoqing2, CAI Yan1, TU Dongbo1(
1 School of Psychology, Jiangxi Normal University, Nanchang 330022, China
2 College of Computer Information Engineering, Jiangxi Normal University, Nanchang 330022, China
Received:
2019-10-14Online:
2020-12-25Published:
2020-10-26Contact:
TU Dongbo E-mail:tudongbo@aliyun.com摘要/Abstract
摘要: 双目标CD-CAT的测验结果既可用于形成性评估也可用于终结性评估。基尼指数可度量随机变量的不确定性程度, 值越小则随机变量的不确定程度越低。本文用基尼指数度量被试知识状态类别以及能力估计置信区间后验概率的变化, 提出基于基尼指数的选题策略。Monte Carlo实验表明与已有的选题策略相比, 新策略的知识状态分类精度和能力估计精度都较高, 同时能有效兼顾题库利用均匀性, 并能快速实时响应, 且受认知诊断模型和被试知识状态分布的影响较小, 可用于实际测验中含多种认知诊断模型的混合题库。
图/表 7
表120题各选题策略的模式判准率均值及标准差
CDM模型 | 知识状态 生成模型 | 选题策略 | |||||||
---|---|---|---|---|---|---|---|---|---|
Gini | ASI | IPA | JSD | ||||||
Mean/% | SD | Mean/% | SD | Mean/% | SD | Mean/% | SD | ||
G-DINA | HO | 97.00 | 0.009 | 89.28 | 0.025 | 96.10 | 0.010 | 85.04 | 0.024 |
MV-0.8 | 97.22 | 0.004 | 93.05 | 0.011 | 97.44 | 0.008 | 92.02 | 0.014 | |
MV-0.2 | 96.84 | 0.007 | 90.78 | 0.014 | 96.35 | 0.006 | 87.51 | 0.016 | |
DINA | HO | 97.45 | 0.010 | 90.99 | 0.032 | 97.18 | 0.011 | 75.31 | 0.060 |
MV-0.8 | 97.24 | 0.011 | 93.45 | 0.017 | 97.06 | 0.010 | 91.46 | 0.023 | |
MV-0.2 | 97.57 | 0.006 | 93.76 | 0.007 | 96.93 | 0.008 | 86.23 | 0.050 | |
R-RUM | HO | 95.41 | 0.010 | 87.61 | 0.021 | 95.38 | 0.010 | 76.64 | 0.028 |
MV-0.8 | 97.09 | 0.009 | 92.45 | 0.014 | 96.82 | 0.008 | 91.67 | 0.010 | |
MV-0.2 | 96.81 | 0.008 | 87.88 | 0.022 | 96.82 | 0.012 | 80.52 | 0.038 |
表120题各选题策略的模式判准率均值及标准差
CDM模型 | 知识状态 生成模型 | 选题策略 | |||||||
---|---|---|---|---|---|---|---|---|---|
Gini | ASI | IPA | JSD | ||||||
Mean/% | SD | Mean/% | SD | Mean/% | SD | Mean/% | SD | ||
G-DINA | HO | 97.00 | 0.009 | 89.28 | 0.025 | 96.10 | 0.010 | 85.04 | 0.024 |
MV-0.8 | 97.22 | 0.004 | 93.05 | 0.011 | 97.44 | 0.008 | 92.02 | 0.014 | |
MV-0.2 | 96.84 | 0.007 | 90.78 | 0.014 | 96.35 | 0.006 | 87.51 | 0.016 | |
DINA | HO | 97.45 | 0.010 | 90.99 | 0.032 | 97.18 | 0.011 | 75.31 | 0.060 |
MV-0.8 | 97.24 | 0.011 | 93.45 | 0.017 | 97.06 | 0.010 | 91.46 | 0.023 | |
MV-0.2 | 97.57 | 0.006 | 93.76 | 0.007 | 96.93 | 0.008 | 86.23 | 0.050 | |
R-RUM | HO | 95.41 | 0.010 | 87.61 | 0.021 | 95.38 | 0.010 | 76.64 | 0.028 |
MV-0.8 | 97.09 | 0.009 | 92.45 | 0.014 | 96.82 | 0.008 | 91.67 | 0.010 | |
MV-0.2 | 96.81 | 0.008 | 87.88 | 0.022 | 96.82 | 0.012 | 80.52 | 0.038 |
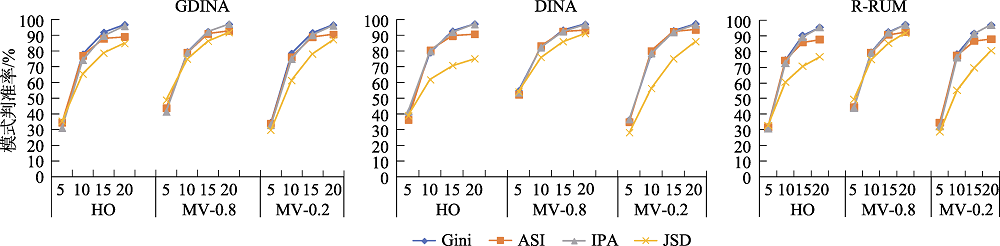
图1不同测验长度的模式判准率
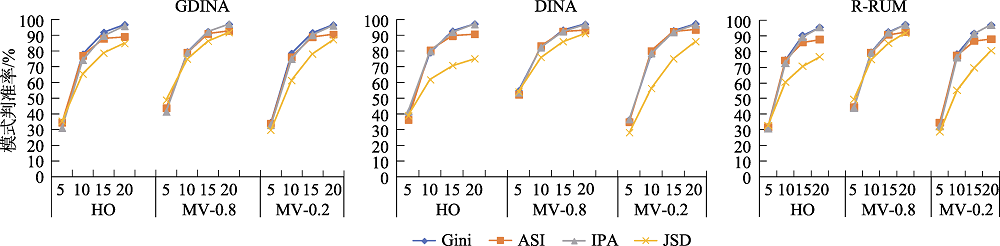
表220题各选题策略的Bias和RMSE
CDM模型 | 知识状态 生成模型 | 选题策略 | |||||||
---|---|---|---|---|---|---|---|---|---|
Gini | ASI | IPA | JSD | ||||||
Bias | RMSE | Bias | RMSE | Bias | RMSE | Bias | RMSE | ||
G-DINA | HO | 0.02 | 0.32 | 0.00 | 0.41 | 0.04 | 0.28 | 0.02 | 0.40 |
MV-0.8 | 0.00 | 0.29 | 0.01 | 0.29 | 0.02 | 0.29 | 0.02 | 0.30 | |
MV-0.2 | 0.03 | 0.27 | 0.02 | 0.32 | 0.07 | 0.27 | 0.05 | 0.42 | |
DINA | HO | -0.08 | 0.40 | -0.02 | 0.41 | -0.14 | 0.37 | -0.05 | 0.46 |
MV-0.8 | 0.02 | 0.34 | 0.01 | 0.32 | -0.03 | 0.35 | -0.08 | 0.35 | |
MV-0.2 | -0.12 | 0.38 | -0.09 | 0.36 | -0.24 | 0.42 | -0.28 | 0.52 | |
R-RUM | HO | -0.07 | 0.35 | -0.01 | 0.42 | -0.14 | 0.35 | -0.02 | 0.45 |
MV-0.8 | 0.00 | 0.30 | -0.02 | 0.30 | -0.03 | 0.30 | -0.03 | 0.32 | |
MV-0.2 | -0.04 | 0.31 | -0.01 | 0.43 | -0.10 | 0.29 | -0.05 | 0.51 |
表220题各选题策略的Bias和RMSE
CDM模型 | 知识状态 生成模型 | 选题策略 | |||||||
---|---|---|---|---|---|---|---|---|---|
Gini | ASI | IPA | JSD | ||||||
Bias | RMSE | Bias | RMSE | Bias | RMSE | Bias | RMSE | ||
G-DINA | HO | 0.02 | 0.32 | 0.00 | 0.41 | 0.04 | 0.28 | 0.02 | 0.40 |
MV-0.8 | 0.00 | 0.29 | 0.01 | 0.29 | 0.02 | 0.29 | 0.02 | 0.30 | |
MV-0.2 | 0.03 | 0.27 | 0.02 | 0.32 | 0.07 | 0.27 | 0.05 | 0.42 | |
DINA | HO | -0.08 | 0.40 | -0.02 | 0.41 | -0.14 | 0.37 | -0.05 | 0.46 |
MV-0.8 | 0.02 | 0.34 | 0.01 | 0.32 | -0.03 | 0.35 | -0.08 | 0.35 | |
MV-0.2 | -0.12 | 0.38 | -0.09 | 0.36 | -0.24 | 0.42 | -0.28 | 0.52 | |
R-RUM | HO | -0.07 | 0.35 | -0.01 | 0.42 | -0.14 | 0.35 | -0.02 | 0.45 |
MV-0.8 | 0.00 | 0.30 | -0.02 | 0.30 | -0.03 | 0.30 | -0.03 | 0.32 | |
MV-0.2 | -0.04 | 0.31 | -0.01 | 0.43 | -0.10 | 0.29 | -0.05 | 0.51 |

图2不同测验长度的能力估计均方差

表320题各选题策略的题库使用均匀性指标
CDM模型 | 知识状态 生成模型 | 选题策略 | |||||||
---|---|---|---|---|---|---|---|---|---|
Gini | ASI | IPA | JSD | ||||||
χ2 | TOE | χ2 | TOE | χ2 | TOE | χ2 | TOE | ||
G-DINA | HO | 82.38 | 0.41 | 98.75 | 0.47 | 85.34 | 0.42 | 44.45 | 0.26 |
MV-0.8 | 69.37 | 0.36 | 77.30 | 0.39 | 77.11 | 0.39 | 53.26 | 0.29 | |
MV-0.2 | 72.50 | 0.37 | 91.36 | 0.44 | 82.94 | 0.41 | 37.08 | 0.23 | |
DINA | HO | 70.91 | 0.36 | 86.88 | 0.43 | 72.68 | 0.37 | 53.52 | 0.29 |
MV-0.8 | 56.55 | 0.31 | 66.74 | 0.35 | 58.98 | 0.32 | 59.31 | 0.32 | |
MV-0.2 | 72.11 | 0.37 | 83.17 | 0.41 | 67.31 | 0.35 | 58.41 | 0.31 | |
R-RUM | HO | 95.78 | 0.46 | 109.29 | 0.52 | 94.55 | 0.46 | 58.22 | 0.31 |
MV-0.8 | 85.70 | 0.42 | 84.99 | 0.42 | 87.92 | 0.43 | 56.27 | 0.30 | |
MV-0.2 | 88.92 | 0.44 | 105.01 | 0.50 | 95.48 | 0.46 | 60.78 | 0.32 |
表320题各选题策略的题库使用均匀性指标
CDM模型 | 知识状态 生成模型 | 选题策略 | |||||||
---|---|---|---|---|---|---|---|---|---|
Gini | ASI | IPA | JSD | ||||||
χ2 | TOE | χ2 | TOE | χ2 | TOE | χ2 | TOE | ||
G-DINA | HO | 82.38 | 0.41 | 98.75 | 0.47 | 85.34 | 0.42 | 44.45 | 0.26 |
MV-0.8 | 69.37 | 0.36 | 77.30 | 0.39 | 77.11 | 0.39 | 53.26 | 0.29 | |
MV-0.2 | 72.50 | 0.37 | 91.36 | 0.44 | 82.94 | 0.41 | 37.08 | 0.23 | |
DINA | HO | 70.91 | 0.36 | 86.88 | 0.43 | 72.68 | 0.37 | 53.52 | 0.29 |
MV-0.8 | 56.55 | 0.31 | 66.74 | 0.35 | 58.98 | 0.32 | 59.31 | 0.32 | |
MV-0.2 | 72.11 | 0.37 | 83.17 | 0.41 | 67.31 | 0.35 | 58.41 | 0.31 | |
R-RUM | HO | 95.78 | 0.46 | 109.29 | 0.52 | 94.55 | 0.46 | 58.22 | 0.31 |
MV-0.8 | 85.70 | 0.42 | 84.99 | 0.42 | 87.92 | 0.43 | 56.27 | 0.30 | |
MV-0.2 | 88.92 | 0.44 | 105.01 | 0.50 | 95.48 | 0.46 | 60.78 | 0.32 |
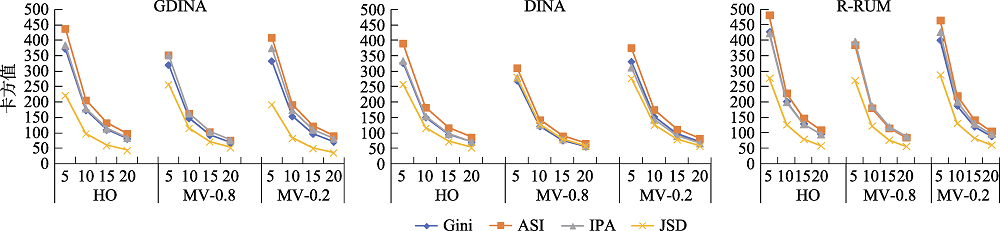
图3不同测验长度的卡方值
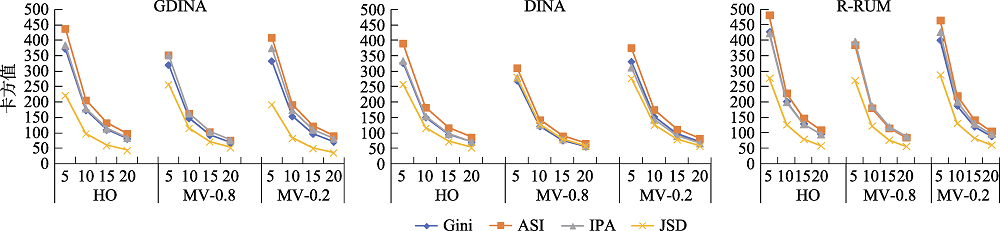
表420题各选题策略的选题用时指标(单位:秒)
CDM模型 | 知识状态 生成模型 | 选题策略 | |||
---|---|---|---|---|---|
Gini | ASI | IPA | JSD | ||
G-DINA | HO | 2.27 | 0.82 | 22.27 | 0.16 |
MV-0.8 | 2.27 | 0.82 | 21.95 | 0.16 | |
MV-0.2 | 2.27 | 0.81 | 22.18 | 0.16 | |
DINA | HO | 2.27 | 0.81 | 21.96 | 0.16 |
MV-0.8 | 2.28 | 0.80 | 21.91 | 0.16 | |
MV-0.2 | 2.26 | 0.78 | 22.04 | 0.16 | |
R-RUM | HO | 2.28 | 0.86 | 21.96 | 0.16 |
MV-0.8 | 2.27 | 0.81 | 22.14 | 0.16 | |
MV-0.2 | 2.26 | 0.81 | 22.01 | 0.16 |
表420题各选题策略的选题用时指标(单位:秒)
CDM模型 | 知识状态 生成模型 | 选题策略 | |||
---|---|---|---|---|---|
Gini | ASI | IPA | JSD | ||
G-DINA | HO | 2.27 | 0.82 | 22.27 | 0.16 |
MV-0.8 | 2.27 | 0.82 | 21.95 | 0.16 | |
MV-0.2 | 2.27 | 0.81 | 22.18 | 0.16 | |
DINA | HO | 2.27 | 0.81 | 21.96 | 0.16 |
MV-0.8 | 2.28 | 0.80 | 21.91 | 0.16 | |
MV-0.2 | 2.26 | 0.78 | 22.04 | 0.16 | |
R-RUM | HO | 2.28 | 0.86 | 21.96 | 0.16 |
MV-0.8 | 2.27 | 0.81 | 22.14 | 0.16 | |
MV-0.2 | 2.26 | 0.81 | 22.01 | 0.16 |
参考文献 47
[1] | Bock R. D., & Mislevy R. J. (1982). Adaptive EAP estimation of ability in a microcomputer environment. Applied Psychological Measurement, 6(4), 431-444. |
[2] | Breiman L., Friedman J., Stone C. J., & Olshen R. A. (1984). Classification and regression trees, Chapman & Hall / CRC, Boca Raton, FL. |
[3] | Cai Y., Miao Y., & Tu D. B. (2016). The polytomously scored cognitive diagnosis computerized adaptive testing, Acta Psychologica Sinica, 48(10), 1338-1346. doi: 10.3724/SP.J.1041.2016.01338URL |
[蔡艳, 苗莹, 涂冬波. (2016). 多级评分的认知诊断计算机化适应测验. 心理学报, 48(10), 1338-1346.] | |
[4] | Chalmers R. P. (2012). Mirt: A multidimensional item response theory package for the renvironment. Journal of Statistical Software, 48(6), 1-29. |
[5] | Chang H. -H., & Ying Z. L. (1996). A global information approach to computerized adaptive testing. Applied Psychological Measurement, 20(3), 213-229. |
[6] | Chen P., Li Z., & Xin T. (2011). A note on the uniformity of item bank usage in cognitive diagnostic computerized adaptive testing. Studies of Psychology and Behavior, 37(1), 212-216. |
[ 陈平, 李珍, 辛涛.(2011). 认知诊断计算机化自适应测验的题库使用均匀性初探. 心理与行为研究, 37(1), 212-216.] | |
[7] | Cheng Y. (2007). The dual information method for item selection in cognitive diagnostic computerized adaptive testing (Unpublished Master’s thesis). University of Illinois at Urbana-Champaign. |
[8] | Cheng Y. (2009). When cognitive diagnosis meets computerized adaptive testing. Psychometrika. 74(4), 619-632. |
[9] | Cheng Y., & Chang H. -H. (2009). The maximum priority index method for severely constrained item selection in computerized adaptive testing. British Journal of Mathematical and Statistical Psychology, 62(2), 369-383. |
[10] | Dai B. Y., Zhang M. Q., & Li G. M. (2016). Exploration of item selection in dual purpose cognitive diagnostic computerized adaptive testing: Based on the RRUM. Applied Psychological Measurement, 40(8), 625-640. doi: 10.1177/0146621616666008URLpmid: 29882535 |
[11] | Du X. X. (2010). A new strategy of item selection of cognitive diagnosis computerized adaptive testing (Unpublished Master’s thesis). Jiangxi Normal University, Nanchang, China. |
[ 杜宣宣. (2010). 具有认知诊断功能的计算机化自适应测验的选题策略研究(硕士学位论文). 江西师范大学, 南昌.] | |
[12] | de la Torre J., (2011). The generalized DINA model framework. Psychometrika, 76(2), 179-199. |
[13] | de la Torre, J., & Douglas J. A. (2004). Higher-order latent trait models for cognitive diagnosis. Psychometrika, 69(3), 333-353. |
[14] | Fan Z. W., Wang C., Chang H. -H., & Douglas J. (2012). Utilizing response time distributions for item selection in CAT. Journal of Educational and Behavioral Statistics, 37(5), 655-670. |
[15] | Han Y. T., Gao X. L., Wang D. X., Cai Y., & Tu D. B. (2018). Item selection methods in multidimensional polytomous computerized adaptive testing. Journal of Psychological Science, 41(6), 1500-1507. |
[ 韩雨婷, 高旭亮, 汪大勋, 蔡艳, 涂冬波. (2018). 多级评分项目的多维CAT选题策略开发. 心理科学, 41(6), 1500-1507.] | |
[16] | Hartz S. M. (2002). A bayesian framework for the unified model for assessing cognitive abilities: blending theory with practicality (Unpublished Doctoral dissertation). University of Illinois at Urbana-Champaign, Urbana-Champaign, IL. |
[17] | Hsu C. -L., & Wang W. -C. (2015). Variable-length computerized adaptive testing using the higher order DINA model. Journal of Educational Measurement, 52(2), 125-143. |
[18] | Hsu C. -L., & Wang W. -C. (2019). Multidimensional computerized adaptive testing using non-compensatory item response theory models. Applied Psychological Measurement, 43(6), 464-480. doi: 10.1177/0146621618800280URLpmid: 31452555 |
[19] | Huang H. -Y. (2020). Utilizing response times in cognitive diagnostic computerized adaptive testing under the higher- order deterministic input, noisy ‘and’ gate model. British Journal of Mathematical and Statistical Psychology, 73(1), 109-141. URLpmid: 30793768 |
[20] | Junker B. W., & Sijtsma K. (2001). Cognitive assessment models with few assumptions, and connections with nonparametric item response theory. Applied Psychological Measurement, 25(3), 258-272. doi: 10.1177/01466210122032064URL |
[21] | Kang H. -A., Zhang S. S., & Chang H. -H. (2017). Dual-objective item selection criteria in cognitive diagnostic computerized adaptive testing. Journal of Educational Measurement, 54(2), 165-183. doi: 10.1111/jedm.12139URL |
[22] | Kaplan M., & de la Torre J. (2020). A blocked-CAT procedure for CD-CAT. Applied Psychological Measurement, 44(1), 49-64. doi: 10.1177/0146621619835500URLpmid: 31853158 |
[23] | Kaplan M., de la Torre J., & Barrada J. R. (2015). New item selection methods for cognitive diagnosis computerized adaptive testing. Applied Psychological Measurement, 39(3), 167-188. doi: 10.1177/0146621614554650URLpmid: 29881001 |
[24] | Li H. (2012). Statistical learning method. Beijing: Tsinghua University Press. |
[ 李航. (2012). 统计学习方法. 北京: 清华大学出版社.] | |
[25] | Lin C. -J., & Chang H. -H. (2019). Item selection criteria with practical constraints in cognitive diagnostic computerized adaptive testing. Educational and Psychological Measurement, 79(2), 335-357. doi: 10.1177/0013164418790634URLpmid: 30911196 |
[26] | Liu S. C., Cai Y., & Tu D. B. (2018). On-the-fly constraint- controlled assembly methods for multistage adaptive testing for cognitive diagnosis. Journal of Educational Measurement, 55(4), 595-613. |
[27] | Lord M. F. (1980). Applications of item response theory to practical testing problems. Hillsdale NJ: Erlbaum. |
[28] | Luo F., Wang X. Q., Ding S. L., & Xiong J. H. (2018). The design and selection strategies of adaptive multigroup Testing for Cognitive Diagnosis. Journal of Psychological Science, 41(3), 720-726. |
[ 罗芬, 王晓庆, 丁树良, 熊建华. (2018). 自适应分组认知诊断测验设计及其选题策略. 心理科学, 41(3), 720-726.] | |
[29] | Ma W. C., & de la Torre J. (2020). GDINA: The generalized DINA model framework. R package version 2.7.9, https:// CRAN.R-project.org/package=GDINA. |
[30] | McGlohen M. K., & Chang H. -H. (2008). Combining computer adaptive testing technology with cognitively diagnostic assessment. Behavior Research Methods, 40(3), 808-821. doi: 10.3758/brm.40.3.808URLpmid: 18697677 |
[31] | Nah F. F. -H. (2004). A study on tolerable waiting time: How long are web users willing to wait? Behaviour and Information Technology, 23(3), 153-163. |
[32] | Quinlan J. R. (1986). Induction of decision trees. Machine Learning, 1(1), 81-106. |
[33] | Quinlan J. R. (1993). C4.5: programs for machine learning. Morgan Kaufmann, San Mateo, CA. |
[34] | Rupp A. A., Templin J., & Henson R. A. (2010). Diagnostic measurement: theory, method, and application. New York: The Guilford Press. |
[35] | Tatsuoka C. (2002). Data analytic methods for latent partially ordered classification models. Journal of the Royal Statistical Society, Series C: Applied Statistics, 51(3), 337-350. |
[36] | Tu D. B., & Cai Y. (2015). The Development of CD-CAT with polytomous attributes. Acta Psychologica Sinica, 47(11), 1405-1414. |
[ 涂冬波, 蔡艳. (2015). 基于属性多级化的认知诊断计算机化自适应测验设计与实现. 心理学报, 47(11), 1405-1414.] | |
[37] | Veerkamp W. J. J., & Berger M. P. F. (1994). Some new item selection criteria for adaptive testing (Research Rep. 94-6). Enschede, The Netherlands: University of Twente, Department of Educational Measurement and Data Analysis. |
[38] | Wang C., & Chang H. -H. (2011). Item selection in multidimensional computerized adaptive testing-gaining information from different angles. Psychometrika, 76(3), 363-384. |
[39] | Wang C., Chang H. -H., & Douglas J. (2012). Combining CAT with cognitive diagnosis: A weighted item selection approach. Behavior Research Methods, 44(1), 95-109. doi: 10.3758/s13428-011-0143-3URLpmid: 21853408 |
[40] | Wang C., Chang H. -H., & Huebner A. (2011). Restrictive stochastic item selection methods in cognitive diagnostic computerized adaptive testing. Journal of Educational Measurement, 48(3), 255-273. |
[41] | Wang C., Zheng C. J., & Chang H. -H. (2014). An enhanced approach to combine item response theory with cognitive diagnosis in adaptive testing. Journal of Educational Measurement, 51(4), 358-380. |
[42] | Xu X. L., Chang H. -H., & Douglas J. (2003, April). A simulation study to compare CAT strategies for cognitive diagnosis. Paper presented at the annual meeting of National Council on Measurement in Education, Chicago, IL. |
[43] | Zhang X. G. (2010). Pattern recognition (Third Edition). Beijing: Tsinghua University Press. |
[ 张学工. (2010). 模式识别(第三版). 北京: 清华大学出版社.] | |
[44] | Zheng C. J., & Chang H. -H. (2016). High-efficiency response distribution-based item selection algorithms for short-length cognitive diagnostic computerized adaptive testing. Applied Psychological Measurement, 40(8), 608-624. URLpmid: 29881073 |
[45] | Zheng C. J., He G., & Gao C. L. (2018). The information product methods: A unified approach to dual-purpose computerized adaptive testing. Applied Psychological Measurement, 42(4), 321-324. doi: 10.1177/0146621617730392URLpmid: 29882539 |
[46] | Zheng C. J., & Wang C. (2017). Application of binary searching for item exposure control in cognitive diagnostic computerized adaptive testing. Applied Psychological Measurement, 41(7), 561-576. doi: 10.1177/0146621617707509URLpmid: 29881106 |
[47] | Zhou Z. H. (2016). Machine learning. Beijing: Tsinghua University Press. |
[ 周志华. (2016). 机器学习. 北京: 清华大学出版社.] |
相关文章 15
[1] | 汪大勋,高旭亮,蔡艳,涂冬波. 基于类别水平的多级计分认知诊断Q矩阵修正:相对拟合统计量视角[J]. 心理学报, 2020, 52(1): 93-106. |
[2] | 王璞珏,刘红云. 让自适应测验更知人善选——基于推荐系统的选题策略[J]. 心理学报, 2019, 51(9): 1057-1067. |
[3] | 詹沛达,于照辉,李菲茗,王立君. 一种基于多阶认知诊断模型测评科学素养的方法[J]. 心理学报, 2019, 51(6): 734-746. |
[4] | 高旭亮,汪大勋,王芳,蔡艳,涂冬波. 基于分部评分模型思路的多级评分认知诊断模型开发[J]. 心理学报, 2019, 51(12): 1386-1397. |
[5] | 陈平. 两种新的计算机化自适应测验在线标定方法[J]. 心理学报, 2016, 48(9): 1184-1198. |
[6] | 高椿雷;罗照盛;喻晓锋; 彭亚风;郑蝉金. CD-MST初始阶段模块组建方法比较[J]. 心理学报, 2016, 48(8): 1037-1046. |
[7] | 孟祥斌;陶剑;陈莎莉. 四参数Logistic模型潜在特质参数的 Warm加权极大似然估计[J]. 心理学报, 2016, 48(8): 1047-1056. |
[8] | 郭磊; 郑蝉金; 边玉芳; 宋乃庆; 夏凌翔. 认知诊断计算机化自适应测验中新的选题策略:结合项目区分度指标[J]. 心理学报, 2016, 48(7): 903-914. |
[9] | 刘彦楼;辛涛;李令青;田伟;刘笑笑. 改进的认知诊断模型项目功能差异检验方法 ——基于观察信息矩阵的Wald统计量[J]. 心理学报, 2016, 48(5): 588-598. |
[10] | 詹沛达;边玉芳;王立君. 重参数化的多分属性诊断分类模型及其判准率影响因素[J]. 心理学报, 2016, 48(3): 318-330. |
[11] | 彭亚风;罗照盛;喻晓锋;高椿雷;李喻骏. 认知诊断评价中测验结构的优化设计[J]. 心理学报, 2016, 48(12): 1600-1611. |
[12] | 汪文义; 宋丽红;丁树良. 复杂决策规则下MIRT的分类准确性和分类一致性[J]. 心理学报, 2016, 48(12): 1612-1624. |
[13] | 蔡艳;苗莹;涂冬波. 多级评分的认知诊断计算机化适应测验[J]. 心理学报, 2016, 48(10): 1338-1346. |
[14] | 詹沛达;陈平;边玉芳. 使用验证性补偿多维IRT模型进行认知诊断评估[J]. 心理学报, 2016, 48(10): 1347-1356. |
[15] | 康春花;任平;曾平飞. 非参数认知诊断方法:多级评分的聚类分析[J]. 心理学报, 2015, 47(8): 1077-1088. |
PDF全文下载地址:
http://journal.psych.ac.cn/xlxb/CN/article/downloadArticleFile.do?attachType=PDF&id=4844