

Identification of Candidate Genes for Seed Glucosinolate Content of Rapeseed by Using Genome-wide Association Mapping and Co-expression Networks Analysis
WEIDa-Yong

通讯作者:
收稿日期:2017-12-10
接受日期:2018-03-15
网络出版日期:2018-03-16
版权声明:2018作物学报编辑部作物学报编辑部
基金资助:
作者简介:
-->
展开
摘要
关键词:
Abstract
Keywords:
-->0
PDF (2011KB)元数据多维度评价相关文章收藏文章
本文引用格式导出EndNoteRisBibtex收藏本文-->
油菜(Brassica napus L., 基因组为AACC)由白菜(B. rapa L., 基因组为AA)和甘蓝(B. oleracea L., 基因组为CC)种间自然杂交并染色体加倍形成[1,2], 是我国乃至世界主要的油料作物。油菜籽粒榨油后的饼粕含丰富的蛋白质, 是良好的天然动物饲料[3]。然而硫代葡萄糖苷(glucosinolate, 简称硫苷)及其降解产物是油菜饼粕中主要的抗营养因子, 严重影响其作为动物饲料的食用安全和适口性, 限制了菜籽饼粕蛋白资源的开发利用[4], 因此降低油菜籽粒中硫苷的含量是油菜育种的重要目标之一。
硫苷是一类含氮和硫的次生代谢产物, 广泛存在于十字花科植物中[5]。硫苷的种类根据侧链R基团的来源不同, 分为脂肪族、芳香族和吲哚族三大类[6]。硫苷的生物合成途径大致可以分为前体氨基酸侧链延长、核心结构合成和次级修饰3个主要阶段, 主要基因在模式植物拟南芥中均已被证实[6,7,8,9,10]。随着油菜及其亲本种(白菜和甘蓝)参考基因组的释放, 大量的硫苷合成和降解基因已被鉴定, 其中在白菜[11]、甘蓝[12]和油菜[1]中分别鉴定出123、127和181个硫苷代谢相关基因。目前对油菜种子硫苷含量的研究主要是通过QTL定位[13,14,15]、全基因组关联分析(GWAS)[16,17]和关联转录组分析(associative transcriptomic)[18,19]挖掘候选基因和功能位点。
随着测序技术的不断更新和测序费用的不断降低, 海量的测序数据需要分析, 由此一种新的方法应运而生,即权重基因共表达网络分析(WGCNA)[20]。其首先假定基因网络服从无尺度分布, 根据功能相似的基因往往具有类似的表达变化, 构建基因模块。然后对模块进行深入挖掘, 比如筛选模块的枢纽基因、关联模块与性状、模块富集分析和建立基因表达网络等[20,21]。该技术已被广泛应用于人类的疾病研究, 比如Farber[22]结合GWAS和WGCNA分析策略, 找到调控人类骨密度的关键枢纽基因。
本研究将结合GWAS和WGCNA研究方法, 从全基因组和转录组水平挖掘油菜种子硫苷总量候选基因, 为油菜种子低硫苷分子育种和农作物复杂性状候选基因鉴定提供参考。
1 材料与方法
1.1 供试材料和表型测定与分析
157份具有广泛变异的甘蓝型油菜(Brassica napus L.)自交种由重庆市油菜工程技术研究中心提供, 于2013?2016连续4年种植于西南大学北碚实验基地, 3行区播种, 每行10株, 每年2次田间重复。采用当地常规田间管理方式。用福斯(FOSS)近红外光谱分析仪(NIRSystem, TR-3750)测定157份油菜自然群体的自交种籽粒硫苷总量, 取每份材料3株自交种, 每袋样品重复测定2次。以SAS软件(版本9.13)[23]对表型数据进行方差分析和相关性分析。根据4年数据从上述自然群体中选择稳定遗传的15份极端低硫苷和15份极端高硫苷材料继续种植, 于次年始花期统一剥蕾自交, 并于自交后第15天取角果提取RNA。1.2 油菜全基因组重测序
于八叶期选择鲜嫩叶片迅速冷冻在液氮中, 由北京百迈客生物科技公司完成总DNA的提取及全基因组重测序。质检合格的文库用Illumina HiSeq 4000平台的双末端150 bp (paired-end, PE150)模式测序, 共得到34.5亿对reads, 平均每个材料的测序深度大约为5倍, 每个材料的覆盖率达到85%。用BWA (版本0.6.1-r104)软件[24]将过滤后得到的干净有效的reads (clean reads)与油菜参考基因组v4.1 (http://www.genoscope.cns.fr/brassicanapus/data/)进行比对; 用GATK (版本2.4-7-g5e89f01)软件[25]检测单核苷酸多态性(single nucleotide polymorphisms, SNP)和插入缺失(insertions and deletions, Indel)。1.3 全基因组关联分析
采用R软件的GenABEL包[26]进行GWAS分析。通过Merk等[27]方法对4年油菜种子的硫苷含量数据进行最佳线性无偏预测(best linear unbiased prediction, BLUP), 估计种子硫苷含量的BLUP值作为表型数据。采用PCA+K (控制主成分和亲缘关系)的混合线性模型对BLUP和SNP标记进行关联位点的检测, 设定阈值为P<8.56×10-8 (0.05/所使用的标记, -lg (P) = 7)。将与显著SNP处于同一单体型块(R2>0.5)的区间, 定义为候选关联区间, 在此区间按以下标准预测候选基因: (1)在甘蓝型油菜或拟南芥参考基因组上与性状相关的已知功能的基因; (2)SNP直接落在基因内部; (3)参考已报道QTL定位的结果。1.4 油菜种子RNA提取及转录组测序
采用植物总RNA提取试剂盒DP432 (天根生化科技有限公司, 北京)提取30份极端材料发育15 d角果的总RNA, 然后将样品送北京百迈客生物科技有限公司构建文库, 并以HiSeq 2000基因分析系统(Illumina公司, USA)进行RNA-Seq PE100测序分析。1.5 差异表达基因鉴定
采用Tophat-Cufflinks-Cuffmerge-Cuffdiff分析流程鉴定DEGs, 利用TopHat 2.0.0调用bowtie 2将reads比对到甘蓝型油菜参考基因组, 以Cufflinks 2.0.0对测序reads进行组装[28], 分析30个样品中所有转录本的表达量, 以每1百万个map上的reads中map到外显子的每千个碱基上的reads数(reads per kilobase of exon per million mapped reads, RPKM)表示。采用Cuffdiff进行DEGs的筛选鉴定和统计检验, 筛选标准如下: (1)过滤掉在30份材料中基因表达量最大值小于1的低丰度基因; (2)差异表达倍数大于2, 即log2绝对值大于1; (3)错误发现率(false discovery rate, FDR) q-value小于0.05。1.6 权重基因共表达网络分析
通过基于R语言的WGCNA包[20]对筛选后得到的2275个DEGs构建基因模块, 经过计算设加权参数软阈值为9, 按照基因间两两不相似性聚类得到基因的系统聚类树(附图1-a), 然后根据动态混合剪切法对聚类树切割修剪, 得到17个分支模块(附图1-b), 计算每个模块的模块特征值(module eigengene, ME), 并对ME聚类, 通过相似度高的ME合并分支模块, 最终得到12个整合的基因模块(附图1-c)。每个模块含有50个到534个基因不等, 有200个基因未能分配到任何1个基因模块中。随后计算ME与样本种子硫苷含量的相关性和显著性, 确定模块在样本中的表达情况, 正(负)相关越高(低), 说明在这个模块内的基因表达量越高(低)。通过基因的连通性确定每个基因的权重值, 连通性高的基因在该模块中可能起枢纽作用。因此, 我们将显著模块内单个基因的平均连通性排名前10%的基因作为枢纽基因(hub gene)。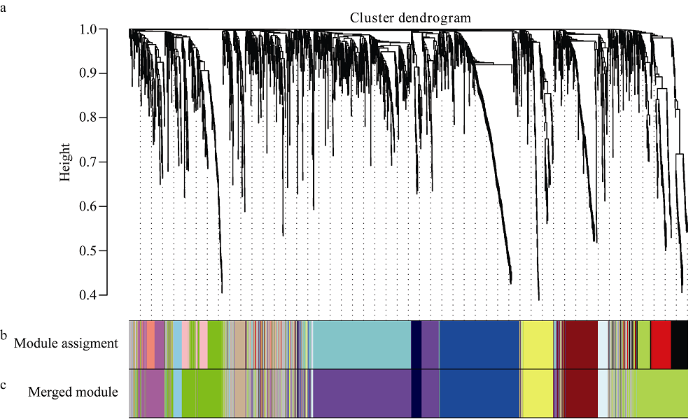
附图1基因聚类树及模块切割
a: 基因聚类树, 纵坐标表示各基因间的聚类距离; b: 动态混合切割得到的分支模块; c: 合并相似度高的模块。
-->Supplementary fig. 1Gene cluster dendrogram and module splitting
a: gene cluster dendrogram tree, y-coordinate indicates the cluster distance between genes; b: modules assignment cut using dynamic tree;
c: merging of modules whose expression profiles are very similar.
-->
1.7 GO富集和KEGG代谢通路分析
以BLASTP将每个模块的油菜蛋白序列与拟南芥蛋白参考序列(TAIR10)比对, 阈值为1×10-10。根据比对结果, 分别采用在线软件agriGO (http://bioinfo. cau.edu.cn/agriGO/)和在线工具DAVID (https://david. ncifcrf.gov/), 以及共同采用Fisher精确检测(P<0.05)和Benjamini Hochberg多重比对检验(FDR<0.05), 以同源性最高的拟南芥蛋白为油菜相对应的蛋白进行GO富集和KEGG代谢途径分析。2 结果与分析
2.1 表型数据分析
157份油菜自然群体的种子硫苷含量存在广泛的遗传变异, 介于27.38~169.20, 平均为(70.13± 36.50) μmol g-1(表1和附表1)。该性状4年均表现频率为连续近正态分布, 表明油菜种子硫苷含量是1个数量性状, 受多基因控制。Table 1
表1
表1关联分析群体中油菜种子硫苷含量的表型变异
Table 1Phenotypic variation of seed GSL in association population
年份 Year | 范围 Range | 平均值±标准偏差 Mean±SD | 峰度值 Kurtosis | 偏度值 Skewness | 变异系数 CV (%) |
---|---|---|---|---|---|
2013 | 27.38-159.40 | 68.72±36.07 | -1.06 | 0.67 | 50.29 |
2014 | 25.25-149.60 | 70.24±35.49 | -0.99 | 0.59 | 54.98 |
2015 | 24.17-153.70 | 69.78±38.37 | -0.95 | 0.65 | 50.54 |
2016 | 24.57-169.20 | 71.78±36.09 | -0.84 | 0.57 | 52.49 |
新窗口打开
Supplementary table 1
附表1
附表1材料来源
Supplementary table 1Source of the accessions in this study
编号 Code | 名称 Name | 种子硫苷含量 Seed GSL content (μmol g-1) | |||
---|---|---|---|---|---|
2016 | 2015 | 2014 | 2013 | ||
1 | 自交种 Inbreed line | 28.06 | 32.25 | 25.25 | 28.06 |
2 | 自交种 Inbreed line | 24.57 | 27.11 | 28.88 | 28.06 |
3 | 自交种 Inbreed line | 28.41 | 29.21 | 26.79 | 30.40 |
4 | 自交种 Inbreed line | 30.45 | 27.61 | 27.20 | 30.33 |
5 | 苏油3号 Suyou 3 | 28.18 | 33.83 | 29.82 | 30.46 |
6 | Rivette | 30.00 | 27.05 | 32.50 | 29.12 |
7 | 自交种 Inbreed line | 38.14 | 30.50 | 33.01 | 27.38 |
8 | 自交种 Inbreed line | 34.63 | 33.41 | 30.85 | 31.50 |
9 | 自交种 Inbreed line | 29.28 | 28.72 | 28.79 | 33.88 |
10 | 华双5号 Huashuang 5 | 30.95 | 31.07 | 33.47 | 29.52 |
11 | 自交种 Inbreed line | 35.33 | 37.41 | 30.12 | 33.00 |
12 | 自交种 Inbreed line | 28.74 | 25.62 | 28.67 | 34.74 |
13 | 自交种 Inbreed line | 29.84 | 31.20 | 31.06 | 32.71 |
14 | 自交种 Inbreed line | 32.15 | 30.71 | 32.50 | 32.08 |
15 | 自交种 Inbreed line | 30.22 | 30.78 | 31.62 | 33.59 |
16 | Larissa | 35.12 | 25.38 | 34.82 | 30.92 |
17 | 自交种Inbreed line | 33.40 | 38.23 | 28.41 | 37.88 |
18 | 自交种 Inbreed line | 32.24 | 28.43 | 36.95 | 29.40 |
19 | Altex | 33.04 | 28.34 | 32.66 | 35.42 |
20 | 自交种Inbreed line | 36.75 | 34.01 | 36.23 | 32.31 |
21 | Bronowski | 32.02 | 33.49 | 32.11 | 36.81 |
22 | 西南大学7号 SWU 7 | 34.10 | 36.77 | 36.17 | 32.75 |
23 | 自交种 Inbreed line | 42.75 | 38.88 | 37.30 | 31.90 |
24 | 自交种 Inbreed line | 33.71 | 33.61 | 35.58 | 33.78 |
25 | Korall | 37.66 | 30.19 | 38.69 | 31.61 |
26 | 自交种 Inbreed line | 37.08 | 32.68 | 35.05 | 36.10 |
27 | 两优586 (F2)-6-3 Liangyou 586 (F2)-6-3 | 28.39 | 33.34 | 29.66 | 41.63 |
28 | 自交种 Inbreed line | 35.90 | 33.57 | 37.52 | 34.14 |
29 | Pauline | 34.96 | 25.22 | 33.89 | 38.58 |
30 | ACS N45 | 31.48 | 24.17 | 35.29 | 37.59 |
31 | Ability | 38.19 | 30.50 | 35.69 | 37.34 |
32 | 自交种 Inbreed line | 34.60 | 28.83 | 39.01 | 34.57 |
33 | Campino | 31.97 | 32.19 | 38.15 | 35.81 |
34 | 自交种 Inbreed line | 34.64 | 39.29 | 38.90 | 35.67 |
35 | 自交种 Inbreed line | 51.38 | 31.85 | 38.73 | 37.54 |
36 | 自交种 Inbreed line | 35.53 | 34.29 | 37.13 | 39.33 |
37 | 自交种 Inbreed line | 37.15 | 39.56 | 33.93 | 42.90 |
编号 Code | 名称 Name | 种子硫苷含量 Seed GSL content (μmol g-1) | |||
2016 | 2015 | 2014 | 2013 | ||
38 | 自交种 Inbreed line | 41.53 | 38.44 | 35.13 | 41.86 |
39 | 自交种 Inbreed line | 42.87 | 73.00 | 37.94 | 39.10 |
40 | Omega | 104.23 | 91.61 | 38.38 | 38.71 |
41 | 自交种 Inbreed line | 37.47 | 38.82 | 42.36 | 35.69 |
42 | Q2 | 90.64 | 56.73 | 36.82 | 44.85 |
43 | 自交种 Inbreed line | 31.31 | 30.93 | 44.48 | 34.73 |
44 | 自交种 Inbreed line | 31.29 | 33.77 | 51.03 | 29.67 |
45 | SW Sinatra | 39.61 | 26.67 | 30.63 | 51.22 |
46 | Andor | 36.17 | 25.78 | 40.15 | 42.52 |
47 | Tenor | 37.59 | 38.33 | 45.89 | 37.66 |
48 | Aurora | 36.04 | 34.34 | 47.48 | 36.77 |
49 | Clipper | 39.78 | 45.41 | 40.64 | 43.75 |
50 | 自交种 Inbreed line | 41.80 | 45.28 | 44.52 | 40.35 |
51 | 自交种 Inbreed line | 41.59 | 36.32 | 45.48 | 39.77 |
52 | 自交种 Inbreed line | 41.30 | 41.84 | 44.09 | 42.22 |
53 | Granit | 38.66 | 34.06 | 39.92 | 44.87 |
54 | 自交种 Inbreed line | 33.59 | 34.04 | 42.23 | 44.32 |
55 | 自交种 Inbreed line | 38.73 | 48.88 | 43.76 | 43.72 |
56 | Montego | 42.93 | 34.94 | 44.67 | 43.51 |
57 | Allure | 49.91 | 41.99 | 46.39 | 42.28 |
58 | 自交种 Inbreed line | 40.08 | 41.41 | 46.04 | 42.97 |
59 | 自交种 Inbreed line | 38.54 | 45.50 | 44.62 | 44.85 |
60 | NK Passion | 48.49 | 46.20 | 46.13 | 43.47 |
61 | Campari | 46.73 | 52.85 | 48.12 | 42.50 |
62 | Lord | 61.09 | 81.47 | 45.95 | 45.95 |
63 | 自交种 Inbreed line | 43.36 | 41.15 | 53.41 | 39.47 |
64 | DRAKKAR | 33.97 | 30.30 | 48.14 | 45.05 |
65 | 中双5号 Zhongshuang 5 | 53.71 | 47.45 | 48.14 | 45.61 |
66 | Lilian | 48.66 | 42.94 | 50.87 | 43.28 |
67 | Rapid | 50.27 | 49.00 | 49.92 | 44.69 |
68 | Aragon | 54.04 | 43.79 | 55.15 | 39.59 |
69 | Lisandra | 48.37 | 31.85 | 54.87 | 40.51 |
70 | 821选×品93-498 F8 821 Xuan × Pin 93-498 F8 | 57.52 | 46.82 | 43.17 | 53.81 |
71 | 油研2号× 84-24016 Youyan 2 × 84-24016 | 76.70 | 79.30 | 41.67 | 56.24 |
72 | Pivot | 31.05 | 31.35 | 58.42 | 41.61 |
73 | SLM 0512 | 56.17 | 30.58 | 53.72 | 48.36 |
74 | Musette | 61.05 | 59.73 | 53.41 | 49.47 |
75 | Lion | 92.53 | 103.65 | 55.58 | 48.03 |
76 | Baros | 52.43 | 38.73 | 59.54 | 45.92 |
77 | Korinth | 58.77 | 50.00 | 47.20 | 57.10 |
78 | Odin | 68.97 | 60.00 | 60.20 | 50.25 |
79 | Beluga | 59.42 | 52.59 | 54.80 | 58.19 |
80 | Express 617 | 83.78 | 46.97 | 60.90 | 52.10 |
81 | SWGospel | 65.64 | 52.66 | 62.86 | 52.86 |
82 | Fortis | 77.72 | 45.97 | 65.66 | 53.94 |
83 | Remy | 68.03 | 53.29 | 65.36 | 54.30 |
84 | BRISTOL | 76.19 | 64.94 | 71.36 | 49.38 |
85 | Jantar | 69.91 | 47.81 | 61.98 | 60.50 |
86 | Binera | 86.68 | 81.60 | 62.24 | 60.40 |
87 | Boston | 74.55 | 52.60 | 64.13 | 59.12 |
88 | Licapo | 60.23 | 44.54 | 66.08 | 57.42 |
89 | Alesi | 73.32 | 50.75 | 66.78 | 59.90 |
90 | Nugget | 73.98 | 52.76 | 68.27 | 58.44 |
91 | Jessica | 71.92 | 73.47 | 69.80 | 57.61 |
92 | HANNA | 76.35 | 76.02 | 62.78 | 67.88 |
93 | Duplo | 74.18 | 76.93 | 74.05 | 57.65 |
94 | Wesroona | 71.19 | 79.28 | 67.37 | 64.48 |
95 | Laser | 76.81 | 58.87 | 75.33 | 57.31 |
96 | Recital | 70.94 | 52.25 | 72.36 | 62.24 |
97 | Escort | 82.04 | 65.80 | 75.95 | 65.02 |
98 | Darmor | 96.64 | 141.00 | 78.38 | 63.00 |
99 | Ww 1286 | 76.39 | 97.97 | 73.08 | 70.24 |
100 | (D57×Oro)×油研2号-F6 (D57×Oro)×Youyan 2-F6 | 72.92 | 56.81 | 67.07 | 76.86 |
101 | Falcon | 72.21 | 73.11 | 76.75 | 71.03 |
102 | 华油6号 Huayou 6 | 84.04 | 91.28 | 73.50 | 78.47 |
103 | 贵农78-6-112 Guinong 78-6-112 | 78.63 | 89.86 | 71.61 | 85.40 |
104 | Chuosenshu | 86.26 | 108.84 | 88.64 | 88.43 |
105 | Lirabon | 109.95 | 104.49 | 98.28 | 86.98 |
106 | Pera | 91.52 | 98.47 | 76.04 | 111.30 |
107 | Manitoba | 87.89 | 94.70 | 114.99 | 73.71 |
108 | Nugget | 105.01 | 94.40 | 104.71 | 87.41 |
109 | Regina II | 89.18 | 95.83 | 93.33 | 99.70 |
110 | Oro | 74.40 | 88.15 | 85.84 | 108.59 |
111 | Galant | 94.22 | 73.92 | 87.67 | 106.82 |
112 | V8 | 108.70 | 89.26 | 109.77 | 85.73 |
113 | Nakate Chousen | 126.30 | 124.36 | 91.44 | 104.60 |
114 | CRESOR | 92.36 | 84.73 | 89.55 | 106.61 |
115 | Hankkija’s Lauri | 99.37 | 128.28 | 96.18 | 100.79 |
116 | TANTAL | 103.22 | 115.34 | 95.86 | 104.73 |
117 | 云油14 Youyou 14 | 96.84 | 102.85 | 106.21 | 96.13 |
118 | K26-96 | 114.75 | 102.00 | 103.92 | 100.34 |
119 | Spaeths Zollerngold | 104.30 | 106.79 | 100.98 | 108.09 |
120 | Baltia | 112.01 | 93.61 | 106.74 | 102.92 |
121 | Mali | 101.14 | 105.85 | 102.20 | 114.77 |
122 | RESYN-H048 | 112.25 | 106.00 | 104.38 | 113.97 |
123 | Daichousen (fuku) | 114.21 | 106.34 | 114.41 | 106.62 |
124 | Orpal | 95.24 | 96.20 | 110.46 | 111.72 |
125 | Orriba | 110.66 | 112.91 | 112.52 | 111.31 |
126 | EMERALD | 110.66 | 112.91 | 109.30 | 114.72 |
127 | 湘油16 Xiangyou 16 | 100.05 | 94.64 | 110.22 | 116.66 |
128 | Daichousen (mizuyasu) | 102.05 | 100.97 | 113.01 | 114.26 |
129 | Zephyr | 114.07 | 105.58 | 117.34 | 112.79 |
130 | Wesreo | 114.03 | 101.87 | 118.95 | 113.23 |
131 | Miyauchi Na | 106.39 | 138.65 | 119.01 | 110.30 |
132 | Olivia | 115.30 | 115.82 | 119.21 | 113.47 |
133 | Taisetsu | 107.13 | 116.00 | 111.10 | 122.19 |
134 | JANETZKIS | 133.00 | 129.88 | 115.00 | 119.73 |
135 | JetNeuf | 112.14 | 132.64 | 120.52 | 114.68 |
136 | Gulliver | 110.21 | 102.68 | 113.37 | 119.94 |
137 | Leonessa | 123.22 | 112.63 | 114.65 | 122.08 |
138 | Ceska Krajova | 107.40 | 105.93 | 123.48 | 115.89 |
139 | Skrzeszowicki | 143.05 | 131.53 | 119.89 | 119.54 |
140 | CANARD | 74.88 | 122.32 | 114.91 | 125.84 |
141 | Mansholt | 102.00 | 107.84 | 122.01 | 121.82 |
142 | Palu | 140.92 | 128.05 | 126.79 | 118.74 |
143 | Parapluie | 131.00 | 142.78 | 124.71 | 122.51 |
144 | Kruglik | 119.41 | 114.38 | 118.23 | 129.02 |
145 | Czyzowska | 108.55 | 122.55 | 116.10 | 131.66 |
146 | Edita | 147.21 | 115.40 | 131.10 | 119.89 |
147 | Sonnengold | 128.77 | 144.22 | 133.00 | 125.70 |
148 | Conny | 139.65 | 122.66 | 134.30 | 128.54 |
149 | 湘农油-1 Xiangnongou-1 | 120.54 | 101.40 | 135.57 | 123.31 |
150 | 西农长角×((D57×Oro)×85-64)F7 Xinongchangjiao×((D57×Oro)×85-64)F7 | 106.86 | 128.13 | 122.10 | 141.81 |
151 | MOANA | 139.74 | 145.62 | 138.69 | 125.34 |
152 | Mlochowski | 118.99 | 120.69 | 133.42 | 131.32 |
153 | Samo | 169.19 | 152.80 | 137.73 | 132.82 |
154 | Suigenshu | 124.93 | 144.33 | 147.49 | 126.56 |
155 | Nunsdale | 134.00 | 151.62 | 144.68 | 131.49 |
156 | Gisora | 150.75 | 148.00 | 131.50 | 159.43 |
157 | Dippes | 149.04 | 153.73 | 149.56 | 143.58 |
新窗口打开
方差分析结果表明, 基因型、环境以及基因型与环境互作间都存在显著差异(P<0.01), 但年度内的重复间不存在显著差异(附表2)。4年种子硫苷含量相关性在0.92~0.96之间。
Supplementary table 2
附表2
附表2关联分析种子硫苷含量表型方差分析
Supplementary table 2ANOVA of phenotype for seed GSL in association population
变异来源 Source | 自由度 df | 均方 MS | 概率值 P-value |
---|---|---|---|
基因型 Genotype | 156 | 9238.54 | <0.001 |
环境 Environment | 3 | 406.70 | 0.0002 |
基因型×环境 Genotype×Environment | 459 | 165.83 | <0.001 |
重复 Repeat | 1 | 0.18 | 0.9561 |
新窗口打开
2.2 以GWAS预测控制种子硫苷含量的候选基因
以全基因组重测序共检测到5 294 158个SNP和1 307 151个InDel。过滤最小等位基因频率小于5%和杂合率大于25%的位点, 剩下690 953个特异的SNP位点用于GWAS分析, GWAS共检测到45个与油菜种子硫苷含量显著相关的SNP, 主要分布在A09 (10个, 物理位置位于2 372 597~3 118 196 bp)、C02 (5个, 物理位置位于44 926 609~44 991 771 bp)和C09 (29个, 物理位置位于2 375 598~3 198 893 bp)染色体的3个区间(图1), 单个位点解释的表型变异介于13.45%~23.34%。这个结果与以前报道的QTL结果一致[14,16,18-19], 表明上述3个区间存在控制油菜种子硫苷含量的主效位点。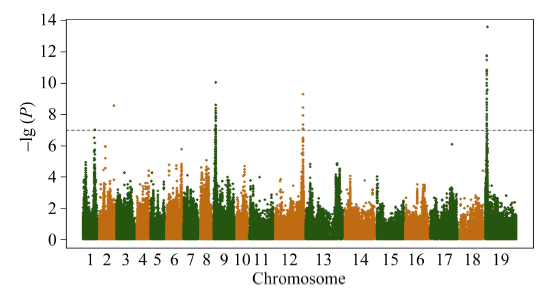
图1全基因组关联分析曼哈顿图
虚线代表矫正后的阈值-lg (P) = 7。
-->Fig. 1Manhattan plot of GWAS
The dashed lines represent the bonferroni-adjusted significance threshold -lg (P) = 7.
-->
与油菜参考基因组的基因注释比对, 在上述3个区间共发现285个基因, 其中5个为已知的硫苷代谢基因。候选基因BnaA09g05480D位于A09染色体2.699 Mb处(显著SNP区间内); 候选基因BnaC09g05060D和BnaC09g05300D分别位于C09染色体2.927 Mb和3.100 Mb处(显著SNP区间内); 候选基因BnaC02g41790D和BnaC02g41860D分别位于C02染色体44.600 Mb和44.703 Mb处(显著SNP上游大约0.2~0.3 Mb处)。与模式植物拟南芥的参考基因组比对后发现, 候选基因BnaC09g05300D与拟南芥基因AT5G61420 (MYB28)同源, 候选基因BnaC02g41790D与AT5G23010 (MAM1)同源, 其他3个候选基因均与AT5G60890 (MYB34)同源, 反映出油菜在进化过程中的基因组复制事件。
2.3 以WGCNA鉴定控制种子硫苷含量的枢纽基因
WGCNA分析发现ME与种子硫苷含量的相关性在-0.76~0.76之间, 其中显著正和负相关的模块分别有4个(Brown、Blue、Purple和Yellow)和2个(Greenyellow和Magenta)(P<0.001)。进一步对显著相关模块内的基因进行GO富集和KEGG分析, 只有1个模块(Brown)内的基因显著富集在硫苷生物合成进程(GO: 0019761)和硫苷生物合成代谢通路(KEGG: ko00966)(表2), 说明该模块内存在控制油菜种子硫苷含量的关键基因。Table 2
表2
表2Brown模块GO富集和KEGG通路分析
Table 2GO enrichment and KEGG pathway analysis for brown module
编号 ID | GO/KEGG项 GO/KEGG term | P值 P-value | 错误发现率 False discovery rate |
---|---|---|---|
GO: 0019761 | 硫苷生物合成进程 Glucosinolate biosynthetic process | 1.90×10-30 | 1.90×10-28 |
KEGG: ko00966 | 硫苷生物合成 Glucosinolate biosynthesis | 6.00×10-07 | 5.30×10-04 |
KEGG: ko01210 | 2-氧代羧酸代谢 2-Oxocarboxylic acid metabolism | 3.40×10-10 | 3.00×10-07 |
新窗口打开
进一步对Brown模块内163个基因进行权重分析, 确定枢纽基因。一共检测到6100条节点线, 每条线的节点连接着1个基因。单个基因的权重值在0.02~0.28之间, 平均值为0.10。删除位于随机染色体的基因, 将剩下的126个基因蛋白序列与拟南芥蛋白参考序列进行同源比对和功能注释分析, 共检测到33个(占26.2%)已知的硫苷代谢基因, 包括13对同源基因, 每对同源基因分别来自于油菜的A和C亚基因组, 说明这些同源基因共表达可能参与种子硫苷的代谢。权重分析中, 连通性排名前10% (权重值>0.20)的基因有13个, 功能注释显示, 其中9个为已知的硫苷代谢基因, 且3对为同源基因, 这些基因在油菜种子硫苷代谢中可能起枢纽作用(表3)。
Table 3
表3
表3Brown模块枢纽基因分析
Table 3Hub genes analysis for Brown module
排名 Rank | 枢纽基因 Hub genes | 染色体和位置 Chromosome and position | 权重值 Weight value | 已知的硫苷代谢基因 Known GSL genes | 拟南芥同源基因 Arabidopsis homologue genes |
---|---|---|---|---|---|
1 | BnaC09g23550D | C09:21069980-21071017 | 0.28 | BAT5 | AT4G12030 |
2 | BnaC05g29760D | C05:28673017-28675449 | 0.27 | / | AT3G22740 |
3 | BnaC08g08320D | C08:12425941-12428358 | 0.25 | / | AT4G14040 |
4 | BnaC04g12860D | C04:10121243-10124859 | 0.25 | UDP-G | AT2G31790 |
5 | BnaC05g12520D | C05:7308750-7311146 | 0.24 | CYP79A2 | AT1G16410 |
6 | BnaC05g33030D | C05:32516433-32519269 | 0.24 | BCAT4 | AT3G19710 |
7 | BnaC04g50950D | C04:48340448-48341488 | 0.22 | / | AT2G46650 |
8 | BnaA04g06630D | A04:5277893-5279719 | 0.22 | CYP83A1 | AT4G13770 |
9 | BnaA08g07580D | A08:7544499-7547006 | 0.22 | / | AT4G14040 |
10 | BnaA09g21170D | A09:13876818-13877853 | 0.21 | BAT5 | AT4G12030 |
11 | BnaA03g35400D | A03:17272166-17274784 | 0.21 | BCAT4 | AT3G19710 |
12 | BnaC02g41790D | C02:44598027-44600079 | 0.20 | MAM1 | AT5G23010 |
13 | BnaA06g11010D | A06:5753484-5755863 | 0.20 | CYP79A2 | AT1G16410 |
新窗口打开
2.4 候选基因与种子硫苷含量的相关性及效应分析
对GWAS鉴定到的5个已知硫苷代谢基因和利用WGCNA发现的13个枢纽基因进行表达分析发现, 其中14个基因的表达量与种子硫苷含量的积累显著正相关(r = 0.376~0.638, P<0.05), 1个基因显著负相关(r = -0.489, P<0.01)(附图2)。此外, 两种方法鉴定到1个共同的基因BnaC02g41790D (MAM1), 该基因与C02染色体上通过GWAS得到的5个显著SNP构成1个单体型块(R2>0.5)(图2-a), 等位基因效应分析发现, 删除自然群体中11份单体型数目小于3的材料, 剩下146份材料被分为五种单体型, 其中99份材料(占群体总数的63%)均为Hap 5, 其平均硫苷含量为50.79 μmol g-1, 与另外四种单体型材料的种子硫苷含量(95.04~110.28 μmol g-1)存在极显著差异(P<0.01)(图2-b)。上述结果表明, 两种方法鉴定到的候选基因与表型性状具有较高的相关性, 并且结合两种方法可能鉴定到比较重要的关键基因。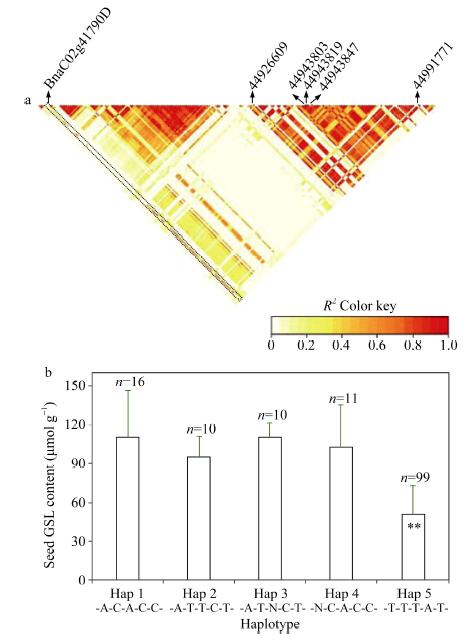
图2连锁不平衡和单体型效应分析
a: 候选基因BnaC02g41790D与C02染色体5个显著SNP构成的单体型连锁不平衡分析, 箭头指示核心基因和显著SNP的物理位置。b: C02染色体5个显著SNP构成的单体型效应分析; **表示在0.01水平显著。
-->Fig. 2Linkage disequilibrium and haplotype effect analysis
a: the LD analysis between the candidate gene BnaC02g41790D and five significant SNPs from GWAS, the arrows shows physical location of the candidate gene and five significant SNPs on chromosome C02. b: the haplotype effect analysis for five significant SNPs in chromosome C02; ** indicates significant difference at P<0.01.
-->
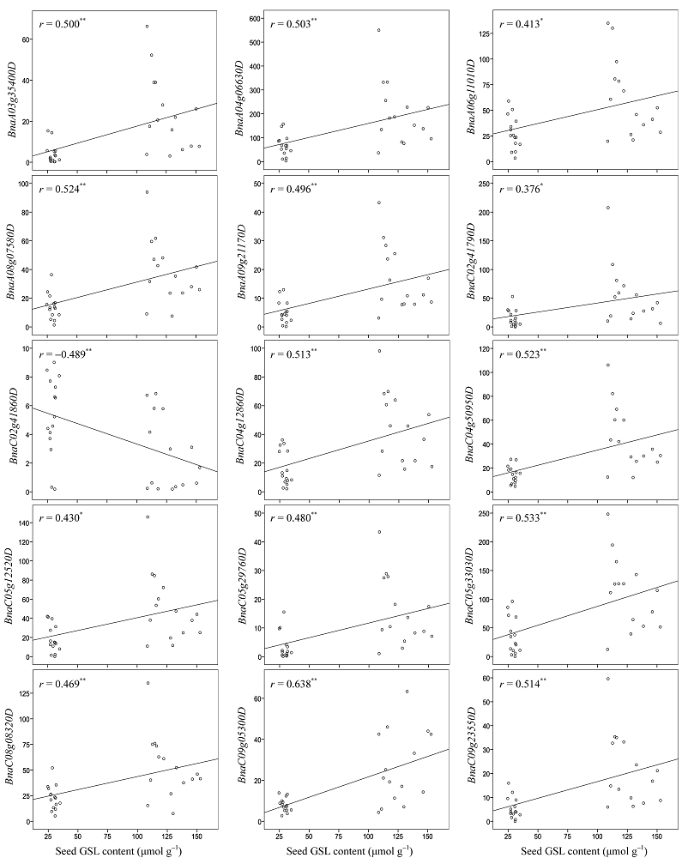
附图2核心基因在30份极端材料中表达水平与种子硫苷含量的相关性分析
*和**分别表示在0.05和0.01水平上相关显著。
-->Supplementary fig. 2Correlation analysis between core gene expression levels and seed GSL contents in 30 extreme accessions
* and ** indicate significant correlation at P<0.05 and 0.01, respectively.
-->
3 讨论
3.1 GWAS得到的候选基因比较分析
基于全基因组重测序相对高的图谱密度, 本研究通过GWAS共检测到5个已知的硫苷代谢基因。其中, 调控吲哚族硫苷代谢的候选基因MYB34的3个同源拷贝分别分布在染色体A09 (BnaA09g05480D)、C02 (BnaC02g41860D)和C09 (BnaC09g05060D)。另外, 控制脂肪族硫苷生物合成的基因MYB28仅仅1个拷贝在染色体C09 (BnaC09g05300D)被检测到, 该候选基因的低表达与种子低硫苷显著相关, 这个结果与以前报道的结果一致。研究发现MYB28在A09和C02染色体上的2个拷贝在低硫苷油菜中已经丢失[18], 而本研究重测序数据比对的甘蓝型油菜参考基因组是1个法国双低冬性油菜品种“Darmor-bzh”, 所以通过GWAS在A09和C02染色体上未检测到MYB28的SNP变异。事实上, 基因组的插入和缺失在作物上是很普遍的, 并且是性状变异的重要机制。在玉米上, 基因ZmVTE4启动子上117 bp的插入和内含子35 bp的缺失, 导致了生育酚含量显著的提升[29]。3.2 GWAS与WGCNA重复鉴定到关键候选基因MAM1
WGCNA对差异表达基因进行模块化分类, 可以有效分离出真实与性状相关的基因, 提高功能富集的检测功效, 对复杂性状的候选基因挖掘提供新的途径[22]。本研究通过WGCNA共检测到13个枢纽基因, 其中9个已被证实参与硫苷的代谢途径[1], 但只有1个基因BnaC02g41790D (MAM1)在GWAS中被重复检测到, 该基因在拟南芥中已被证实编码甲硫烷基化苹果酸合酶(methylthioalkylmalate synthase), 是控制甲硫氨酸起源的脂肪族硫苷侧链延长的1个主要基因[30]。转录组表达分析发现, 该基因的表达与种子硫苷含量的积累显著正相关(r=0.376, P<0.05)。比较五种单体型发现, Hap 5与另外4种单体型的差别主要是C02染色体上SNP位点44 943 847等位基因由C变为A, 使得种子硫苷含量降低52%。根据该位点开发基于PCR的功能标记, 将使油菜进一步降低种子硫苷含量成为可能。3.3 两种方法检测效率分析及其应用
两种方法只检测到1个重复的基因, 原因可能是: (1) WGCNA中的其他12个枢纽基因在本研究油菜自然群体的DNA水平上没有差异, 导致通过GWAS检测不到显著的位点; (2) WGCNA中单一枢纽基因对油菜种子硫苷的贡献度太小, 它们的显著性淹没在背景噪音中, 通过GWAS难以准确挖掘出来, 对微效多基因控制的位点检测能力不足, 正是GWAS面临的主要问题。因此, 在植物复杂农艺性状候选基因挖掘中, 结合GWAS和WGCNA分析是一种新的思路, 可以提高对微效位点的检测能力和构建关键基因的调控网络。另外, 硫苷总量是由各分量构成, 控制硫苷各组分的相关基因的变异(包括转录水平的差异)均能影响硫苷总量的表现型。本研究通过GWAS和WGCNA方法试图挖掘影响硫苷总量的基因, 但这些基因可能参与硫苷分量的代谢, 需进一步研究确定。另外, 用WGCNA可以深入挖掘显著模块内枢纽基因, 并对功能未知的基因进行功能注释预测。因为, 在权重网络中, 节点线连接的2个基因表达模式是相似的, 且有潜在的相似功能, 若节点线一端基因功能已知的话, 就可以预测另一端基因的功能, 为以后验证功能未知基因提供参考。
4 结论
通过GWAS和WGCNA共鉴定到18个控制油菜种子硫苷含量的候选基因, 其中多数为已知的硫苷基因, 且其表达量与种子硫苷含量显著相关。1个控制脂肪族硫苷侧链延长的关键基因BnaC02g41790D (MAM1)被两种方法共同检测出, 与该基因连锁的5个SNP构成5种单体型, 其中Hap 5覆盖了63%的材料, 其种子硫苷含量显著低于含其他4种单体型的材料。因此, 结合GWAS和WGCNA是一种鉴定复杂性状候选基因的新策略, 可兼顾微效位点的检出率以及对关键基因的确定。The authors have declared that no competing interests exist.
作者已声明无竞争性利益关系。
参考文献 原文顺序
文献年度倒序
文中引用次数倒序
被引期刊影响因子
[1] | . |
[2] | . CAB Direct is the most thorough and extensive source of reference in the applied life sciences, incorporating the leading bibliographic databases CAB Abstracts and Global Health |
[3] | |
[4] | . This paper provides a brief overview of the biochemistry, genetics andbiological activity of glucosinolates and their degradation products.These compounds are found in vegetative and reproductive tissues of16 plant families, but are most well known as the major secondarymetabolites in the Brassicaceae. Following tissue disruption, theyare hydrolysed to a variety of products of which isothiocyanates(`mustard oils') are the most prominent. The majority of geneticstudies have concentrated on reducing the levels of these compoundsin the seeds of oilseed Brassica crops due to antinutritionalfactors associated with 2-hydroxy-3-butenyl glucosinolate. However,current interest is concerned with the anticarcinogenic activity ofisothiocyanates derived from cruciferous vegetables and salad crops. |
[5] | . |
[6] | . Abstract Glucosinolates are sulfur-rich, anionic natural products that upon hydrolysis by endogenous thioglucosidases called myrosinases produce several different products (e.g., isothiocyanates, thiocyanates, and nitriles). The hydrolysis products have many different biological activities, e.g., as defense compounds and attractants. For humans these compounds function as cancer-preventing agents, biopesticides, and flavor compounds. Since the completion of the Arabidopsis genome, glucosinolate research has made significant progress, resulting in near-complete elucidation of the core biosynthetic pathway, identification of the first regulators of the pathway, metabolic engineering of specific glucosinolate profiles to study function, as well as identification of evolutionary links to related pathways. Although much has been learned in recent years, much more awaits discovery before we fully understand how and why plants synthesize glucosinolates. This may enable us to more fully exploit the potential of these compounds in agriculture and medicine. |
[7] | . The first committed step in the biosynthesis of indole glucosinolates is the conversion of indole-3-acetaldoxime into an indole-3-S-alkyl-thiohydroximate. The initial step in this conversion is catalyzed by CYP83B1 in Arabidopsis (S. Bak, F.E. Tax, K.A. Feldmann, D.A. Galbraith, R. Feyereisen [2001] Plant Cell 13: 101-111). The knockout mutant of the CYP83B1 gene (rnt1-1) shows a strong auxin excess phenotype and are allelic to sur-2. CYP83A1 is the closest relative to CYP83B1 and shares 63% amino acid sequence identity. Although expression of CYP83A1 under control of its endogenous promoter in the rnt1-1 background does not prevent the auxin excess and indole glucosinolate deficit phenotype caused by the lack of the CYP83B1 gene, ectopic overexpression of CYP83A1 using a 35S promoter rescues the rnt1-1 phenotype. CYP83A1 and CYP83B1 heterologously expressed in yeast (Saccharomyces cerevisiae) cells show marked differences in their substrate specificity. Both enzymes convert indole-3-acetaldoxime to a thiohydroximate adduct in the presence of NADPH and a nucleophilic thiol donor. However, indole-3-acetaldoxime has a 50-fold higher affinity toward CYP83B1 than toward CYP83A1. Both enzymes also metabolize the phenylalanine- and tyrosine-derived aldoximes. Enzyme kinetic comparisons of CYP83A1 and CYP83B1 show that indole-3-acetaldoxime is the physiological substrate for CYP83B1 but not for CYP83A1. Instead, CYP83A1 catalyzes the initial conversion of aldoximes to thiohydroximates in the synthesis of glucosinolates not derived from tryptophan. The two closely related CYP83 subfamily members therefore are not redundant. The presence of putative auxin responsive cis-acting elements in the CYP83B1 promoter but not in the CYP83A1 promoter supports the suggestion that CYP83B1 has evolved to selectively metabolize a tryptophan-derived aldoxime intermediate shared with the pathway of auxin biosynthesis in Arabidopsis. |
[8] | . Glucosinolates and their associated degradation products have long been recognized for their distinctive benefits to human nutrition and plant defense. Because most of the structural genes of glucosinolate metabolism have been identified and functionally characterized in Arabidopsis thaliana , current research increasingly focuses on questions related to the regulation of glucosinolate synthesis, distribution and degradation as well as to the feasibility of engineering customized glucosinolate profiles. Here, we highlight recent progress in glucosinolate research, with particular emphasis on the biosynthetic pathway and its metabolic relationships to auxin homeostasis. We further discuss emerging insight into the signaling networks and regulatory proteins that control glucosinolate accumulation during plant development and in response to environmental challenge. |
[9] | . Summary We report characterization of SUPERROOT1 (SUR1) as the C–S lyase in glucosinolate biosynthesis. This is evidenced by selective metabolite profiling of sur1 , which is completely devoid of aliphatic and indole glucosinolates. Furthermore, following invivo feeding with radiolabeled p -hydroxyphenylacetaldoxime to the sur1 mutant, the corresponding C–S lyase substrate accumulated. C–S lyase activity of recombinant SUR1 heterologously expressed in Escherichia coli was demonstrated using the C–S lyase substrate djenkolic acid. The abolishment of glucosinolates in sur1 indicates that the SUR1 function is not redundant and thus SUR1 constitutes a single gene family. This suggests that the ‘high-auxin’ phenotype of sur1 is caused by accumulation of endogenous C–S lyase substrates as well as aldoximes, including indole-3-acetaldoxime (IAOx) that is channeled into the main auxin indole-3-acetic acid (IAA). Thereby, the cause of the ‘high-auxin’ phenotype of sur1 mutant resembles that of two other ‘high-auxin’ mutants, superroot2 ( sur2 ) and yucca1 . Our findings provide important insight to the critical role IAOx plays in auxin homeostasis as a key branching point between primary and secondary metabolism, and define a framework for further dissection of auxin biosynthesis. |
[10] | . Glucosinolates are natural plant products gaining increasing interest as cancer-preventing agents and crop protectants. Similar to cyanogenic glucosides, glucosinolates are derived from amino acids and have aldoximes as intermediates. We report cloning and characterization of cytochrome P450 CYP79A2 involved in aldoxime formation in the glucosinolate-producing Arabidopsis thaliana L. The CYP79A2 cDNA was cloned by polymerase chain reaction, and CYP79A2 was functionally expressed in Escherichia coli. Characterization of the recombinant protein shows that CYP79A2 is an N-hydroxylase converting L-phenylalanine into phenylacetaldoxime, the precursor of benzylglucosinolate. Transgenic A. thaliana constitutively expressing CYP79A2 accumulate high levels of benzylglucosinolate. CYP79A2 expressed in E. coli has a K(m) of 6.7 micromol liter(-1) for L-phenylalanine. Neither L-tyrosine, L-tryptophan, L-methionine, nor DL-homophenylalanine are metabolized by CYP79A2, indicating that the enzyme has a narrow substrate specificity. CYP79A2 is the first enzyme shown to catalyze the conversion of an amino acid to the aldoxime in the biosynthesis of glucosinolates. Our data provide the first conclusive evidence that evolutionarily conserved cytochromes P450 catalyze this step common for the biosynthetic pathways of glucosinolates and cyanogenic glucosides. This strongly indicates that the biosynthesis of glucosinolates has evolved based on a cyanogenic predisposition. |
[11] | . |
[12] | . |
[13] | . The orthologues of Arabidopsis involved in seed glucosinolates metabolism within QTL confidence intervals were identified, and functional markers were developed to facilitate breeding for ultra-low glucosinolates in canola. Further reducing the content of seed glucosinolates will have a positive impact on the seed quality of canola (Brassica napus). In this study 43 quantitative trait loci (QTL) for seed glucosinolate (GSL) content in a low-GSL genetic background were mapped over seven environments in Germany and China in a doubled haploid population from a cross between two low-GSL oilseed rape parents with transgressive segregation. By anchoring these QTL to the reference genomes of B. rapa and B. oleracea, we identified 23 orthologues of Arabidopsis involved in GSL metabolism within the QTL confidence intervals. Sequence polymorphisms between the corresponding coding regions of the parental lines were used to develop cleaved amplified polymorphic site markers for two QTL-linked genes, ISOPROPYLMALATE DEHYDROGENASE1 and ADENOSINE 5'-PHOSPHOSULFATE REDUCTASE 3. The genic cleavage markers were mapped in the DH population into the corresponding intervals of QTL explaining 3.36-6.88 and 4.55-8.67 % of the phenotypic variation for seed GSL, respectively. The markers will facilitate breeding for ultra-low seed GSL content in canola. |
[14] | . |
[15] | . Abstract A genetic linkage map of Brassica napus constructed from a cross between a low glucosinolate cultivar ‘H5200’ and a high glucosinolate line ‘NingRS-1’ was used to identify loci associated with seed glucosinolate content and to understand the association between specific glucosinolate components and Sclerotinia resistance. Seed glucosinolate content was assessed by standard High pressure Liquid Chromatogram (HPLC) protocol. Seven components of seed glucosinolate, including four types of aliphatic glucosinolate, two types of indolyl glucosinolates and one aromatic glucosinolate were detected in the seeds. Three quantitative trait loci (QTLs) were identified for seed total glucosinolate content. From three to 15 loci were found to be responsible for different types of glucosinolates, and by comparing the overlapped intervals, eight genomic regions were defined. One of the nine loci associated with aliphatic glucosinolate content was found to be associated with Sclerotinia resistance on the leaf at the seedling stage, and one locus, responsible for 3-indolyl-methyl glucosinolate content, was probably linked with Sclerotinia resistance on the stem of the maturing plant. The association between seed glucosinolate content and Sclerotinia resistance is discussed. |
[16] | . Abstract Association mapping can quickly and efficiently dissect complex agronomic traits. Rapeseed is one of the most economically important polyploid oil crops, although its genome sequence is not yet published. In this study, a recently developed 60K Brassica Infinium(脗庐) SNP array was used to analyse an association panel with 472 accessions. The single-nucleotide polymorphisms (SNPs) of the array were in silico mapped using 'pseudomolecules' representative of the genome of rapeseed to establish their hypothetical order and to perform association mapping of seed weight and seed quality. As a result, two significant associations on A8 and C3 of Brassica napus were detected for erucic acid content, and the peak SNPs were found to be only 233 and 128 kb away from the key genes BnaA.FAE1 and BnaC.FAE1. BnaA.FAE1 was also identified to be significantly associated with the oil content. Orthologues of Arabidopsis thaliana HAG1 were identified close to four clusters of SNPs associated with glucosinolate content on A9, C2, C7 and C9. For seed weight, we detected two association signals on A7 and A9, which were consistent with previous studies of quantitative trait loci mapping. The results indicate that our association mapping approach is suitable for fine mapping of the complex traits in rapeseed. The Author 2014. Published by Oxford University Press on behalf of Kazusa DNA Research Institute. |
[17] | . |
[18] | . Association genetics can quickly and efficiently delineate regions of the genome that control traits and provide markers to accelerate breeding by marker-assisted selection. But most crops are polyploid, making it difficult to identify the required markers and to assemble a genome sequence to order those markers. To circumvent this difficulty, we developed associative transcriptomics, which uses transcriptome sequencing to identify and score molecular markers representing variation in both gene sequences and gene expression, and correlate this with trait variation. Applying the method in the recently formed tetraploid crop Brassica napus, we identified genomic deletions that underlie two quantitative trait loci for glucosinolate content of seeds. The deleted regions contained orthologs of the transcription factor HAG1 (At5g61420), which controls aliphatic glucosinolate biosynthesis in Arabidopsis thaliana. This approach facilitates the application of association genetics in a broad range of crops, even those with complex genomes. |
[19] | . |
[20] | . |
[21] | . WGCNA(weighted gene co-expression network analysis)算法是一种构建基因共表达网络的典型系统生物学算法,该算法基于高通量的基因信使RNA(mRNA)表达芯片数据,被广泛应用于国际生物医学领域。本文旨在介绍wGCNA的基本数理原理,并依托R软件包WGNCA以实例的方式介绍其应用。WGCNA算法首先假定基因网络服从无尺度分布,并定义基因共表达相关矩阵、基因网络形成的邻接函数,然后计算不同节点的相异系数,并据此构建分层聚类树(hierarchical clustering tree),该聚类树的不同分支代表不同的基因模块(module),模块内基因共表达程度高,而分数不同模块的基因共表达程度低。最后,探索模块与特定表型或疾病的关联关系,最终达到鉴定疾病治疗的靶点基因、基因网络的目的。 . WGCNA(weighted gene co-expression network analysis)算法是一种构建基因共表达网络的典型系统生物学算法,该算法基于高通量的基因信使RNA(mRNA)表达芯片数据,被广泛应用于国际生物医学领域。本文旨在介绍wGCNA的基本数理原理,并依托R软件包WGNCA以实例的方式介绍其应用。WGCNA算法首先假定基因网络服从无尺度分布,并定义基因共表达相关矩阵、基因网络形成的邻接函数,然后计算不同节点的相异系数,并据此构建分层聚类树(hierarchical clustering tree),该聚类树的不同分支代表不同的基因模块(module),模块内基因共表达程度高,而分数不同模块的基因共表达程度低。最后,探索模块与特定表型或疾病的关联关系,最终达到鉴定疾病治疗的靶点基因、基因网络的目的。 |
[22] | . Abstract Genome-wide association studies (GWAS) have emerged as the method of choice for identifying common variants affecting complex disease. In a GWAS, particular attention is placed, for obvious reasons, on single-nucleotide polymorphisms (SNPs) that exceed stringent genome-wide significance thresholds. However, it is expected that many SNPs with only nominal evidence of association (e.g., P < 0.05) truly influence disease. Efforts to extract additional biological information from entire GWAS datasets have primarily focused on pathway-enrichment analyses. However, these methods suffer from a number of limitations and typically fail to lead to testable hypotheses. To evaluate alternative approaches, we performed a systems-level analysis of GWAS data using weighted gene coexpression network analysis. A weighted gene coexpression network was generated for 1918 genes harboring SNPs that displayed nominal evidence of association (P 0.05) from a GWAS of bone mineral density (BMD) using microarray data on circulating monocytes isolated from individuals with extremely low or high BMD. Thirteen distinct gene modules were identified, each comprising coexpressed and highly interconnected GWAS genes. Through the characterization of module content and topology, we illustrate how network analysis can be used to discover disease-associated subnetworks and characterize novel interactions for genes with a known role in the regulation of BMD. In addition, we provide evidence that network metrics can be used as a prioritizing tool when selecting genes and SNPs for replication studies. Our results highlight the advantages of using systems-level strategies to add value to and inform GWAS. |
[23] | |
[24] | . |
[25] | . |
[26] | . Abstract Here we describe an R library for genome-wide association (GWA) analysis. It implements effective storage and handling of GWA data, fast procedures for genetic data quality control, testing of association of single nucleotide polymorphisms with binary or quantitative traits, visualization of results and also provides easy interfaces to standard statistical and graphical procedures implemented in base R and special R libraries for genetic analysis. We evaluated GenABEL using one simulated and two real data sets. We conclude that GenABEL enables the analysis of GWA data on desktop computers. AVAILABILITY: http://cran.r-project.org. |
[27] | . Alterations in SHANK genes were repeatedly reported in (). is a group of diagnosed by persistent deficits in social communication/interaction across multiple contexts, with restricted/repetitive patterns of . To date, diagnostic criteria for are purely behaviorally defined and reliable biomarkers have still not been identified. The validity of models for therefore strongly relies on their behavioral phenotype. Here, we studied communication by means of isolation-induced pup ultrasonic vocalizations (USV) in the model for by comparing (-/-) null mutant, (+/-) heterozygous, and (+/+) wildtype littermate controls. The first aim of the present study was to evaluate the effects of deletions on developmental aspects of communication in order to see whether -related communication deficits are due to general impairment or delay in development. Second, we focused on social context effects on USV production. We show that (-/-) pups vocalized less and displayed a delay in the typical inverted U-shaped developmental USV emission pattern with USV rates peaking on postnatal day () 9, resulting in a prominent genotype difference on PND6. Moreover, testing under social conditions revealed even more prominently genotype-dependent deficits regardless of the familiarity of the social context. As communication by definition serves a social function, introducing a social component to the typically nonsocial test environment could therefore help to reveal communication deficits in models for . Together, these results indicate that is involved in acoustic communication across species, with genetic alterations in resulting in social communication/interaction deficits. 2015. 2015 International Society for Research, Wiley Periodicals, Inc. |
[28] | . |
[29] | . Abstract Tocopherols are a class of four natural compounds that can provide nutrition and function as antioxidant in both plants and animals. Maize kernels have low α-tocopherol content, the compound with the highest vitamin E activity, thus, raising the risk of vitamin E deficiency in human populations relying on maize as their primary vitamin E source. In this study, two insertion/deletions (InDels) within a gene encoding γ-tocopherol methyltransferase, Zea mays VTE4 (ZmVTE4), and a single nucleotide polymorphism (SNP) located ~85 kb upstream of ZmVTE4 were identified to be significantly associated with α-tocopherol levels in maize kernels by conducting an association study with a panel of ~500 diverse inbred lines. Linkage analysis in three populations that segregated at either one of these three polymorphisms but not at the other two suggested that the three polymorphisms could affect α-tocopherol content independently. Furthermore, we found that haplotypes of the two InDels could explain 6533% of α-tocopherol variation in the association panel, suggesting ZmVTE4 is a major gene involved in natural phenotypic variation of α-tocopherol. One of the two InDels is located within the promoter region and associates with ZmVTE4 transcript level. This information can not only help in understanding the underlying mechanism of natural tocopherol variations in maize kernels, but also provide valuable markers for marker-assisted breeding of α-tocopherol content in maize kernels, which will then facilitate the improvement of maize as a better source of daily vitamin E nutrition. |
[30] | . |