
DAMAGE INDEX METHOD FOR DETECTION OF FLAWS BASED ON DYNAMIC RESPONSE 1)
Du Chengbin
通讯作者: 2) 杜成斌, 教授, 主要研究方向: 水工结构中力学问题研究. E-mail:cbdu@hhu.edu.cn
收稿日期:2019-08-14接受日期:2019-10-22网络出版日期:2019-10-22
基金资助: |
Received:2019-08-14Accepted:2019-10-22Online:2019-10-22
作者简介 About authors

摘要
结构振动响应对于检测结构内部损伤具有重要意义,利用距离缺陷不同位置处传感器实测响应与无损结构响应之间的差异,定义各传感器的不同权重, 在此基础上构建结构不同位置处的损伤指标.研究中运用动力扩展有限元法结合水平集法描述结构内部缺陷以避免迭代计算中的网格重划分.首先, 在得到不同位置的传感器权重后,利用阈值函数使原来呈线性的权重转换为非线性权重,即对不同位置处权重大小进行放缩,并通过引入双三次插值得到结构的损伤指标及其分布,结合插值成像技术识别缺陷数目及其所在的大致区域; 最后,在利用智能算法进行反演的过程中, 先剔除不必要的传感器,再引入加权系数改进目标函数进行精确反演. 若干算例的分析表明:损伤指标法能够在缺陷数目未知的情况下通过正向建模快速得出缺陷具体数目及位置初步信息,引入加权系数的目标函数反分析方法相较于以往智能算法可以更快达到收敛,更加高效地得出缺陷精确位置. 为了进一步证明该模型的可行性及工程应用前景,运用本文建立的模型对含圆形孔洞缺陷的钢筋混凝土板进行了缺陷检测,得到了较好的反演结果, 证明提出的模型切实可行且具有一定的工程意义.
关键词:
Abstract
:The vibration response of the structure is of great importance for detecting the damage inside the structure. Based on the difference between the measured response of sensors at different positions in the real structure and the nondestructive structure, the different weights of each sensor are defined, and the damage indexes at different positions in the structure are constructed. In this paper, dynamic extended finite element method combined with level set method is used to describe the internal defects of the structure to avoid grid redivision in iterative computation. After obtaining the weights of sensors at different positions, the traditional linear weights are replaced by the nonlinear weights depending on the comparing the traditional linear weight with threshold value, in order to reduce and amplify the weight value at different locations. The double-cubic interpolation is introduced to obtain the damage index and its distribution of the structure, the number of defects and their potential areas are identified with interpolation imaging technology. In the process of inversion using intelligent algorithms, the unnecessary sensors are removed at first and the weighting coefficients are introduced to improve the objective function for accurate inversion. The analysis of several examples indicates that the damage index method can obtain the specific number and preliminary position information of defects through forward modeling quickly when the number of defects is unknown, and the objective function inverse analysis method with weighting coefficient can reach the convergence faster and obtain the precise location of defects more efficiently than those in the previous intelligent algorithms. In order to further prove the feasibility and application prospect of the model, we use the model presented in this paper to detect the defects of reinforced concrete slab with two circular holes, and the good inversion results are shown that the proposed model is feasible and has certain engineering significance.
Keywords:
PDF (23681KB)元数据多维度评价相关文章导出EndNote|Ris|Bibtex收藏本文
本文引用格式
杜成斌, 金立成, 吴志勤. 基于动力响应识别结构内部缺陷的损伤指标法 1). 力学学报[J], 2019, 51(6): 1841-1855 DOI:10.6052/0459-1879-19-221
Du Chengbin, Jin Licheng, Wu Zhiqin.
引言
近年来建立在结构动力特性基础上的无损识别方法受到了特别的重视[1-3],基于脉冲响应函数、动力响应、模态参数或柔度矩阵的不同方法在实际损伤(缺陷)识别问题中均得到了成功应用[4-6], 其中运用结构动力响应进行结构内部缺陷识别的方法尤为常见[7-8].然而直接运用有限元法得到结构动力响应在处理复杂结构时非常耗时,在每次反演识别缺陷的迭代优化过程中, 有限元法均需要重新划分网格,在成百上千次迭代的网格生成中要耗费大量时间[9-10].为了获得更有效的计算方法, 许多****引入扩展有限元法(extended finite elementmethod, XFEM)建立结构内部缺陷的正向反演模型,用以解决反演分析问题中识别结构内部复杂缺陷的问题[11],该方法可以在不重新划分网格的情况下,结合水平集法直接描述缺陷的具体形状[12-13].扩展有限元与智能算法结合共同识别结构内部缺陷, 其中扩展有限元用于正向建模,优化后的智能算法用于反演迭代直到目标函数收敛, 以此来寻找缺陷参数.早在2010年, Rabinovich等[11,14]就基于XFEM和遗传算法(genetic algorithm,GA)提出了一种裂纹反演识别的数值方法,并利用该方法识别出静、动荷载下平板薄膜结构(均质材料)内部的裂纹; 相对来讲,静载作用下实际结构的位移值很难获取, 而动力荷载作用下容易得到结构的响应,因而基于实测动响应反演结构内部缺陷越来越受到重视.2013年Sun等[15]建立人工蜂群算法(artificial bee colony algorithm, ABC)反演模型反演结构内部不同缺陷,利用人工蜂群算法控制参数少、空间复杂度较低的特点来得到缺陷的数值解.以上模型主要针对结构内部单个缺陷, 为解决实际工程中出现的多缺陷识别问题,2017年Ma等[16]基于动力扩展有限元法和人工蜂群算法, 通过层次聚类法反复迭代得到缺陷的数目;2018年Zhao等[17]提出一种自适应的人工鱼群多尺度算法识别结构内部缺陷;同年王翔等[18]通过引入拓扑变量提出一种动力扩展有限元法和人工蜂群算法相结合的反演分析模型检测钢筋混凝土结构内部多缺陷问题,将结构内部多缺陷反演分为两个阶段:第一阶段利用引入拓扑变量的人工蜂群算法得到缺陷具体数目及位置范围,第二阶段使用改进人工蜂群算法继续在得到的缺陷范围内进行精确反演,得到最终各个缺陷的参数大小. 然而,上述未知数目的多缺陷识别过程通常需要预先假设可能存在的最大缺陷数[18];同时需要利用反向模型中的智能算法进行上百次迭代来确定缺陷数目,处理过程繁琐且耗时较长[19-20].
本文首先分析了有缺陷的真实结构与无损结构之间在不同位置处的动力响应,利用距离缺陷不同位置处传感器敏感性的差异, 赋予传感器不同权重. 其次,在得到各传感器权重后, 引入双三次插值得到损伤指标的方法,结合插值成像技术识别缺陷的数目及缺陷区域,再引入加权目标函数的人工蜂群算法对结构内部缺陷进行数值模型反演,建立基于损伤指标法的正分析与反分析结合共同识别结构内部缺陷的数值模型. 最后, 通过3个算例验证了建立的反演分析模型的准确性和高效性.
1 利用动力扩展有限元进行正向建模
1.1 水平集法描述结构缺陷
采用XFEM计算含缺陷结构的响应量估计值. XFEM通过引入非连续位移模式,使得不连续位移场的描述独立于网格划分, 可以在不重新划分网格的前提下,通过改变水平集函数反映缺陷位置及大小,这克服了常规有限元方法在面对复杂缺陷时网格划分的困难.各形状的缺陷水平集函数如下[21], 圆形孔洞的水平集函数如式(1)所示式中, $ x_{\rm c} $为圆心点的坐标向量, $r_{\rm c} $为半径. 相应椭圆形孔洞的水平集函数可以表示为
式中, $(x_{\rm c} ,y_{\rm c})$为椭圆中心点坐标, $r_{\rm a}$为椭圆的半长轴, $r_{\rm b}$为椭圆的半短轴, $\beta $为椭圆的方向角, 以逆时针为正, $\beta \in [0,\pi]$.
对于结构内包含多缺陷的情况, 水平集函数只需要取其最小值, 即
式中, $n_{\rm t} $为缺陷的总个数.
1.2 位移模式
扩展有限元的位移模式为[22-23]等号右边第一项为常规FEM部分, 等号右边第二项为改进部分. 式中, $N_i ({{ x}})$为结点$i$对应的常规FEM的插值形函数, ${{ u}}_i $为常规FEM结点$i$处的位移未知量, $\psi _i ({{ x}})$为XFEM的改进函数, ${{ a}}_i$为结点$i$的改进位移未知量, $N_i^\ast ({{ x}})$为单位分解函数, 其形式可以和$N_i ({{ x}})$相同, 也可以不同, 这里取二者相同[24].
1.3 支配方程
对于平面四节点等参元, 不考虑阻尼的情况下, 由弹性动力学的基本方程[25-26]并根据式(4)的位移模式, 利用虚功原理,可得到动力XFEM的支配方程为式中$\left[ {{\begin{array}{*{20}c} {{{ M}}^{uu}} & {{{ M}}^{ua}} & {{{ M}}^{ub}} & {{{ M}}^{uv}} \\ {{{ M}}^{au}} & {{{ M}}^{aa}} & {{{ M}}^{ab}} & {{{ M}}^{av}} \\ {{{ M}}^{bu}} & {{{ M}}^{ba}} & {{{ M}}^{bb}} & {{{ M}}^{bv}} \\ {{{ M}}^{vu}} & {{{ M}}^{va}} & {{{ M}}^{vb}} & {{{ M}}^{vv}} \\\end{array} }} \right]$为整体质量矩阵;$\left[ {{\begin{array}{*{20}c} {{{ K}}^{uu}} & {{{ K}}^{ua}} & {{{ K}}^{ub}} & {{{ K}}^{uv}} \\ {{{ K}}^{au}} & {{{ K}}^{aa}} & {{{ K}}^{ab}} & {{{ K}}^{av}} \\ {{{ K}}^{bu}} & {{{ K}}^{ba}} & {{{ K}}^{bb}} & {{{ K}}^{bv}} \\ {{{ K}}^{vu}} & {{{ K}}^{va}} & {{{ K}}^{vb}} & {{{ K}}^{vv}} \\\end{array} }} \right]$为整体刚度矩阵;$\left[ \begin{array}{*{20}c} {{{ f}}^u(t)} \\ {{{ f}}^a(t)} \\ {{{ f}}^b(t)} \\ {{{ f}}^v(t)} \\\end{array} \right]$为整体载荷列阵;$\left[ {{\begin{array}{*{20}c} {{\ddot{ u}}} \\ {{\ddot{ a}}} \\ {{\ddot{ b}}} \\ {{\ddot{ v}}} \\\end{array} }} \right]$和$\left[ {{\begin{array}{*{20}c} {{ u}} \\ {{ a}} \\ {{ b}} \\ {{ v}} \\\end{array} }} \right]$分别代表加速度列阵和位移列阵;每个列阵中除包含有限元常规自由度外, 还有反映结构内部孔洞、夹杂、贯穿单元的附加自由度未知量: 即$ u$为常规位移未知量, $ a$, $ b$和$ v$分别为孔洞、夹杂和贯穿单元中结点处相应的附加位移未知量.本文采用Newmark隐式算法对式(5)进行时间积分, 具体步骤见文献[27].
2 传感器定义损伤指标识别结构缺陷初步信息
2.1 加权目标函数
在反分析问题中, 往往是已知结构某些关键点的响应量(如位移、加速度、速度等)通过反演算法求解结构一些未知的物理特性.反分析法目前已被用于材料参数的反演.结构关键点的响应量通常通过安装在结构上的一系列传感器得到(本文由XFEM数值建模进行计算代替测量值).反演算法首先使用一系列参数来描述结构内部缺陷,使用一个合理的目标函数描述正分析所求得的预设关键点的响应量和实际测得的关键点的响应量之间的差距,通过不断迭代更新这些参数, 逐步缩小目标函数值直到算法收敛,即所求得的预设关键点的响应量和实际测得的响应量差别最小.在实际测量过程中, 接近缺陷的测量值可能比更远的测量值包含更多的缺陷信息.因此, 本文为各个传感器赋予不同的权重. 并在目标函数中引入加权系数,使加权最小二乘误差之和为
式中, ${{ \theta }}$ 为模型中待反演参数, $a_{ij}^{\rm c} \left( {{ \theta }} \right)$, $a_{ij}^{\rm m} $分别为传感器布置点的数值结果和真实值. 本文选定的传感器测值为测点的加速度, $N_S $ 为模型中传感器的总数, $N_T$为动力扩展有限元法中所取的时程点个数, $\omega _j$代表每个传感器分配的权重, 其表达式为[28]
式中, $u$为有损伤时测点加速度值, $\tilde {u}$为原始状态无损伤时的测点加速度值.通过式(7)的权重表达式可以初步描述传感器距离缺陷的远近程度,然而为了使不同位置处传感器描述缺陷位置的效果更加明显, 引入阈值函数,如式(8)所示. 通过阈值函数可以使原来式(7)呈线性的权重转换为非线性权重,式(8)表示的递增函数在自变量小于阈值时增速小于原权重表达式,大于阈值时增速大于原权重表达式,以此达到对原权重小于阈值的传感器进行权重弱化,大于阈值的传感器权重进行放大的效果. 随着大权重传感器所占比例增大,显著增强其描述缺陷位置的效果.
参考文献[29], 取$a=10$, $\beta=5$, 并设$\lambda$为0.5. 并将式(8)中加入阈值函数的$\hat{\omega }_j $代替式(7)的$\omega _j $代入目标函数式(6)中得到新的加权目标函数.
2.2 损伤指标法
实际工程中, 结构内部缺陷的数目及形状均是事先未知,因此确定缺陷的数量是首先需要解决的问题.为了进一步寻找测得的动力响应与缺陷位置的关系,通过动力扩展有限元法对含缺陷结构及其对应的无损状态下的结构进行正向建模,并得到各传感器处动力加速度, 代入式(7)和式(8)得到各传感器的权重, 由式(9)对各权重进行双三次插值即可得出损伤指标.式(9)表达式为式中, $a_{ij}^{mn} $为插值系数; $x_m $, $y_n $分别代表传感器所在的坐标点; 当位于传感器坐标点时, $S\left( {x,y} \right)$等于传感器的权重系数$\hat{\omega }_j $.
利用式(9)进行插值成像得出等高线图, 通过图中等高线信息得到$S\left( {x,y} \right)$的极大值(包括全局极大值)可确定损伤区域的个数及位置. 在本研究中,缺陷可能存在的子域被定义为损伤指标局部最大值周围的区域.选择每个子域的长度和宽度为传感器间距的两倍;若损伤指标局部最大值位于问题域的边缘,则直接使用传感器间距作为长度及宽度来定义子域.考虑到在进行插值过程中需要较多传感器而在后续反演过程中过多权重较小的传感器则会增加不必要的计算量,因而在进行正分析一次建模并得到相应损伤区域后可将其个数适当简化:将位于损伤区域外权重较小的传感器剔除, 以此来减少后续反演迭代计算的工作量.
3 损伤指标法与人工蜂群算法相结合识别结构内部缺陷的模型
3.1 人工蜂群算法
利用动力扩展有限元法进行正向建模并通过损伤指标得到缺陷的具体数目及缺陷初步位置区域之后,将智能算法进一步引入缺陷识别过程中进行反演分析即可得到缺陷的具体位置及大小.常用的群智能优化算法有很多, 包括人工蜂群算法、粒子群算法、蚁群算法等.由于已经运用了损伤指标法确定缺陷的数目及范围,后续反演只需在较小的范围内进一步搜索缺陷的精确位置及具体参数.本文采用原理上更为简单的人工蜂群算法. 该算法与遗传算法及其他智能算法相比,具有结构简单, 控制变量少, 易于实现等优点. 其搜索能力及开发能力均较强,且可以避免遗传算法在反演早期出现的``早熟''等现象.人工蜂群算法是土耳其埃尔吉耶斯大学的 Karaboga[30]在 2005年提出的一种基于蜜蜂觅食行为的群体智能算法, 主要含有3个控制参数:蜜源的数量 ($N_{\rm Food})$、优化极值不变的最大搜索限定次数 ($N_{\rm Limit})$和最大迭代次数 ($N_{\rm Iter})$, 这使得算法的空间复杂度较低. 在该算法中,蜜源的位置对应优化问题的可行解(缺陷的位置、大小),蜜源的花蜜量对应可行解的质量(适应度函数值),寻找花蜜速度对应可行解的优化速度, 最大花蜜量则对应优化问题最优解.人工蜂群算法与扩展有限元法结合可有效地减少反演分析的计算工作量,并能快速探测结构内部存在的缺陷. 在常规 ABC算法反演缺陷的模型中,蜜源对应缺陷各个参数, 其质量由适应度函数值表示. 为提高迭代反演速率,本文将反分析问题中的目标函数值设为式(6)所示形式.首先在搜索区域内根据式(10)得到一系列初始蜜源,并由式(6)得出适应度函数值以判断其质量.
其中, $x_i^j $表示第$i$个蜜源的第$j$维参数, $x_{\min} $代表该反演参数的下界, $x_{\max} $代表该维参数上界, $\gamma _i^j $表示对应于初始解$x_i^j $的随机数, 大小在0到1之间.
初始蜜源给定之后, 采蜜蜂会根据式(11)在各个蜜源周围进行邻域搜索,根据贪婪准则将新蜜源与原蜜源的质量进行比较并保留下质量好的一部分
其中, $x_k^j $是第$j$维参数中不同于$x_i^j $而随机产生的第$k$个解, $\theta _i^j $是对应邻域搜索下产生的新的随机数, 范围是($-1$, 1),替换后跟随蜂由式(12)所示概率公式确定将跟随的采蜜蜂
式中, $p\left( {\alpha _i } \right)$是第$i$个解对应的跟随概率. 根据轮盘赌机制, $p\left( {\alpha _i } \right)$越大的采蜜蜂被跟随几率就越大,在跟随蜂进行完选择跟随后会再次根据式(11)进行邻域搜索并计算适应度函数值. 若某处蜜源搜索达到一定次数没有得到优化, 则将其放弃,同时跟随蜂转化为侦察蜂开始下一轮迭代.
3.2 缺陷识别模型
在缺陷数目未知的情况下, 运用损伤指标法与人工蜂群算法识别结构内部缺陷,强化了结构缺陷识别过程中正分析模型的作用,在缺陷数目的确定过程中只需进行一次正向建模,减少了以往反演过程中大量重复迭代的过程.首先建立起实际含缺陷模型和与其相对应的无损伤结构的数值模型,得到外界动荷载作用下两模型各相同测点处动力时程响应,通过式(7)计算各测点位置处传感器的不同权重,并由阈值函数(8)进行传感器权重比例调节,在此基础上通过式(9)结合插值成像技术得出损伤指标插值等高线轮廓,由此显示出缺陷数目及缺陷的识别子域. 根据缺陷识别子域可以更新缺陷搜索参数,本研究中选择每个子域的长度和宽度为传感器间距距离的两倍.在根据损伤指标法得到缺陷的数目及位置初步信息后更新传感器位置,通过式(6)改进目标函数式的反演算法在各缺陷所在的参数搜索区域内进行精确反演,得到最终的缺陷参数结果. 具体损伤指标法识别缺陷的流程图如图1所示.图1
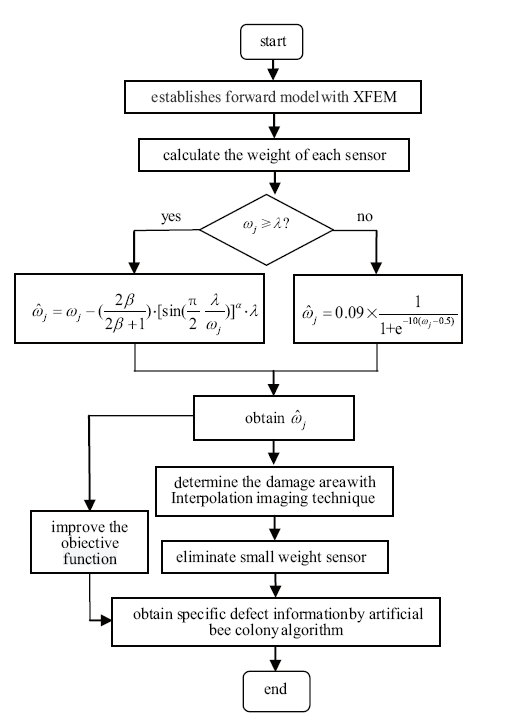
图1损伤指标法反演流程图
Fig.1Damage index inversion flow chart
4 算例
4.1 两个椭圆形缺陷
一正方形板边长为5 m, 板内含两椭圆形缺陷, 一椭圆中心点坐标(1.93 m, 1.28 m), 长半轴0.25 m, 短半轴0.13 m, 方向角为45.02$^\circ$, 另一椭圆中心点坐标(3.20 m, 3.21 m), 长半轴0.3 m, 短半轴0.12 m, 方向角为135.43$^\circ$, 坐标系$xoy$如图2(a)所示. 板的下侧受固定约束, 右侧受冲击荷载$P$激励. 假设方形板处于平面应力状态, 其弹性模量$E = 30$~GPa, 泊松比$v = 0.2$. 除了固定边, 在板的表面均匀布置了3个传感器, 其分布位置如图2(b)所示.利用扩展有限元对含缺陷的真实结构及其对应的无损结构进行正向建模,且模型均将板划分为$50\times 50$的网格, 如图2(b)和图2(c)所示.图2
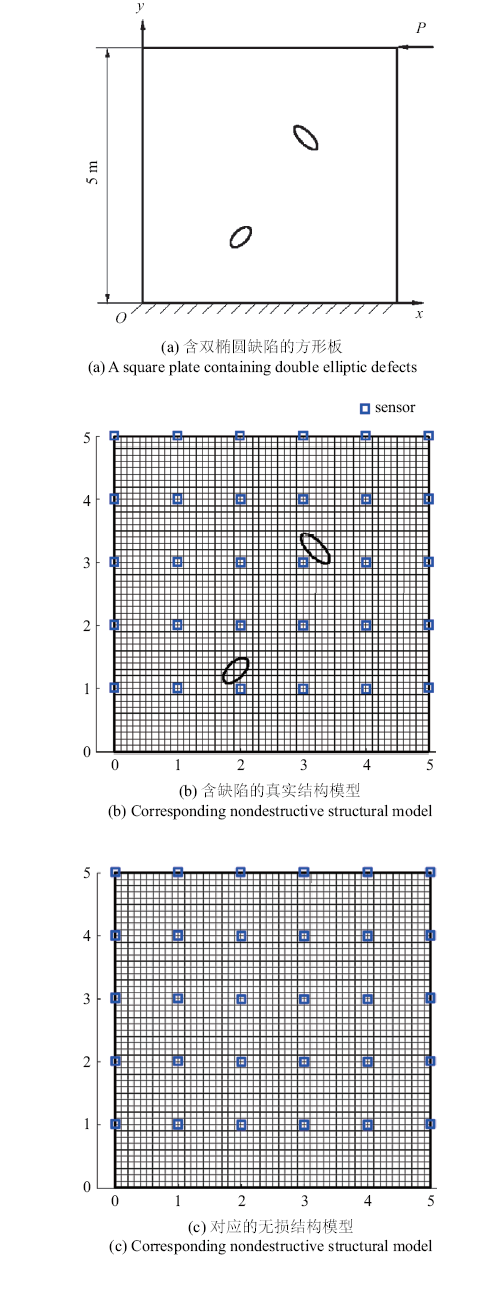
图2板的载荷、约束信息及传感器布置情况将
Fig.2Load, constraint information and sensor layout of the board
图2中两模型各测点处传感器测得的动力响应值代入式(7)及式(8)中得到各传感器权重,并运用式(9)对各测点权重进行插值, 结合插值成像技术得到相应的损伤指标表面分布图如图3(a)所示, 其等高线图如图3(b)所示. 根据图3损伤指标表面分布图及等高线图即可得缺陷初步信息.
图3
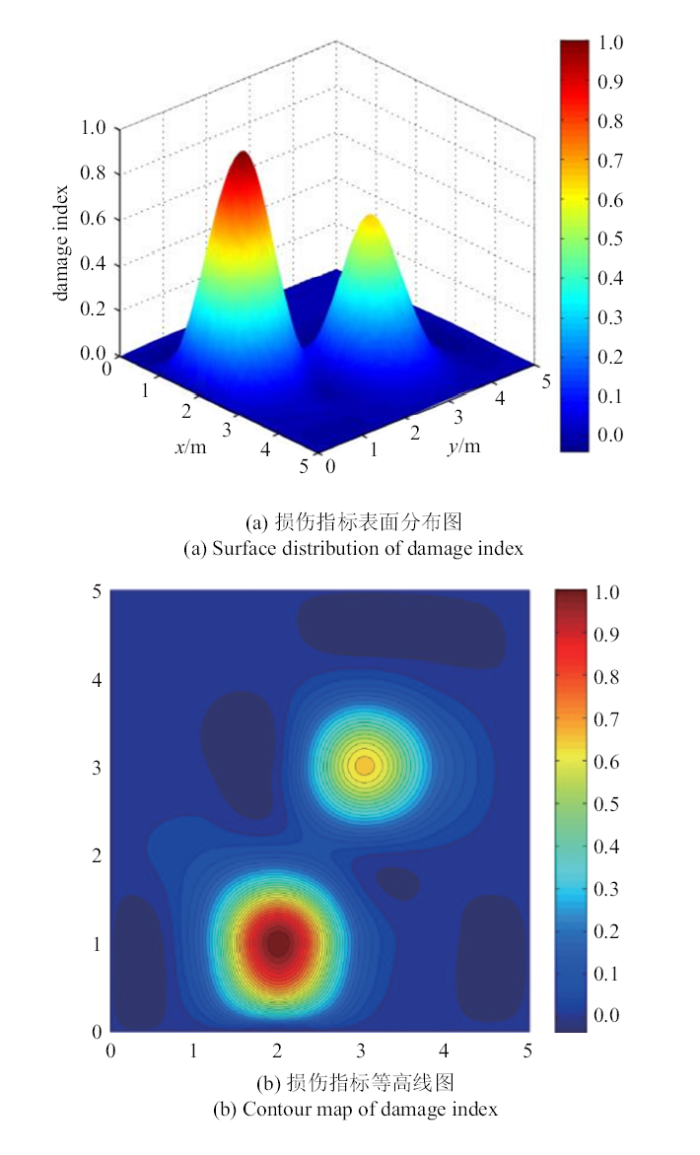
图3损伤指标表面分布图及其等高线图
Fig.3Surface distribution map and contour map of damage index
从图3可看到求解域内含两个极大值, 等高线图也反映出板内含两个孔洞,将两个极大值周围子域用蓝色方框表示;进一步在该缩小的缺陷范围内进行改进目标函数的ABC算法反演,最终可得缺陷具体的形状及位置信息, 其中ABC算法中各控制变量设为:蜜源数量$N_{\rm Food} = 100$, 最大搜索限定次数$N_{\rm Limit}=20$,最大迭代次数$N_{\rm Iter} = 200$. 此时需反演的参数为各椭圆缺陷的中心坐标($x_{\rm c}$,$y_{\rm c}$), 长半轴长$r_{\rm a}$, 短半轴长$r_{\rm b}$以及椭圆方向角$\beta$.在利用ABC算法进行迭代反演前先剔除权重较小的传感器, 如图4所示,其中红色方块表示被剔除的传感器, 蓝色方块为剩下的传感器.图5给出了ABC算法在缩小的缺陷范围内进行缺陷精确反演的过程.其中黑色椭圆图形表示实际缺陷, 红色图形表示反演结果.迭代过程中反演结果及目标函数值如表1所示.
图4
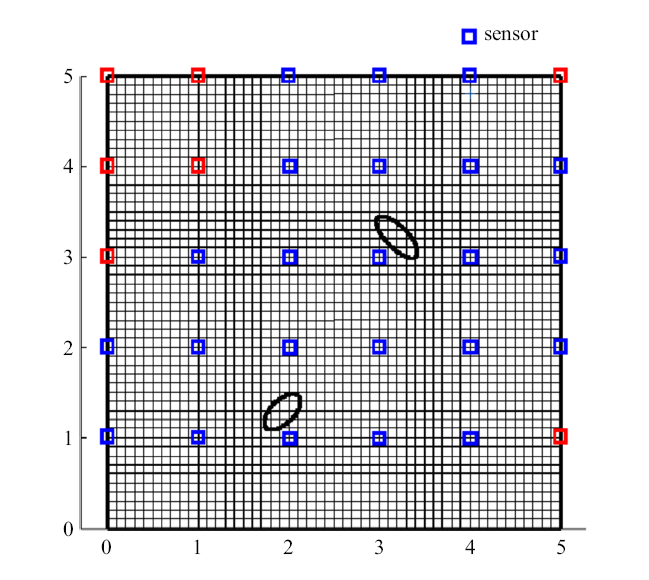
图4剔除小权重传感器的正方形板
Fig.4Square plate with small weight sensor excluded
图5
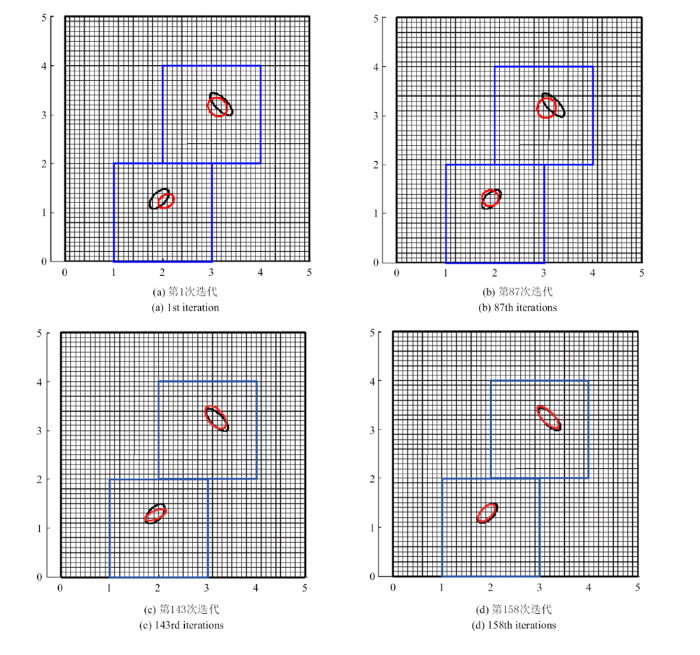
图5利用人工蜂群算法在缩小的范围内进行搜索
Fig.5Search within a reduced range by using artificial bee colony algorithm
Table 1
表1
表1人工蜂群算法进行双椭圆缺陷反演的迭代过程表
Table 1
![]() |
新窗口打开|下载CSV
从图5中可以看到, 反演迭代到第158步时已经得到缺陷的精确位置及大小,相比于原来在缺陷数目未知的情况下进行两阶段的迭代反演,并引入拓扑变量进行缺陷数目确定的常规人工蜂群算法模型,减少了几百步的迭代次数, 效率要提高很多.这两种方法与损伤指标法确定缺陷数目但未经目标函数加权进行改进的算法进行比较,得到缺陷的迭代反演曲线图如图6所示.图6中圆形点线代表常规含拓扑变量反演第1阶段,菱形点线为常规反演第2阶段[18];正方形点线为利用本文提出的损伤指标法确定缺陷的数目及范围后,未使用传感器加权系数改进目标函数反演得到的收敛曲线;三角形点线则为本文所提损伤指标法结合引入加权系数改进目标函数的反演算法计算得到的收敛曲线.从图6中可以看出, 由损伤指标法首先得到缺陷的数目及范围,再结合改进目标函数的反演算法得到缺陷具体位置和大小的反演方法,相较于以往引入拓扑变量进行反演的算法模型省去了第1阶段为确定缺陷数目进行的反复迭代过程,且在均由损伤指标得到缺陷具体数目及范围的情况下,改进目标函数的反演模型的速率及准确度均优于未改进目标函数的反演算法,说明本文提出的损伤指标结合改进目标函数的方法收敛到真实缺陷的效率更高.
图6
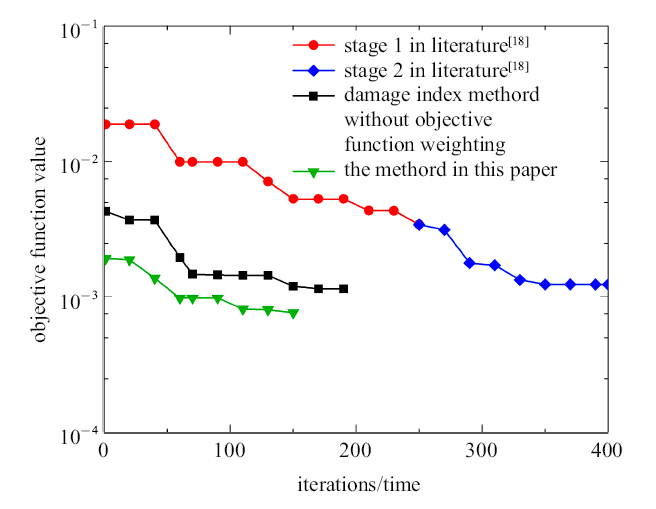
图6损伤指标法与拓扑变量法收敛曲线比较图
Fig.6Comparison of convergence curves of inversion process of three different defect recognition methods
4.2 三个圆形缺陷的识别
一方形板内含有3个圆形缺陷, 板的信息参数同算例4.1,假设方形板处于平面应力状态. 缺陷圆心坐标分别为(1.38 m, 1.09 m), (2.30 m, 3.81 m),(4.21 m, 3.22 m), 半径分别为0.16 m, 0.18 m, 0.19 m.为得到缺陷的数目及初步位置信息,利用动力扩展有限元法建立实际含缺陷的模型及其对应的无缺陷的结构模型,两模型均将板划分为$50\times 50$的网格, 其中真实含缺陷结构模型如图7(b),对应的无损结构模型同算例4.1中图2(c)所示.由动力扩展有限元分别计算得到两个模型各测点动力响应之后,将这些动力响应值代入式(7)、式(8)计算各测点位置传感器权重,并结合式(9)对其进行插值成像,得到如图8(a)的损伤指标表面分布图和图8(b)所示的损伤指标等高线图.即可根据该分布图及等高线图得到缺陷数目及位置范围信息.图7
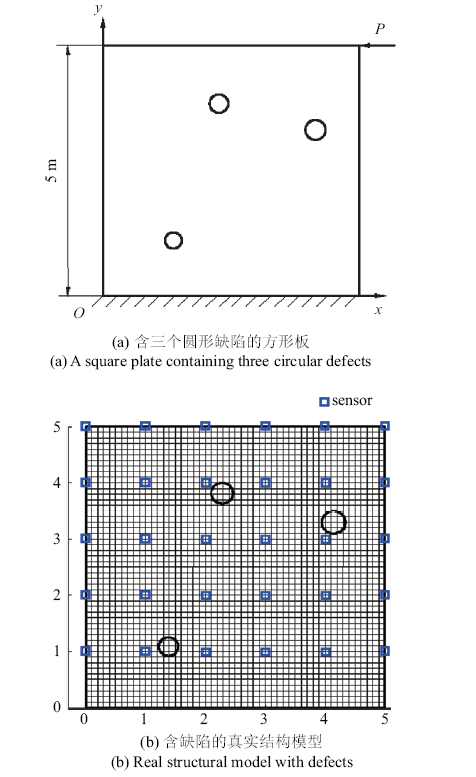
图7板的载荷、约束信息及传感器布置情况
Fig.7Load, constraint information and sensor layout of the board
图8
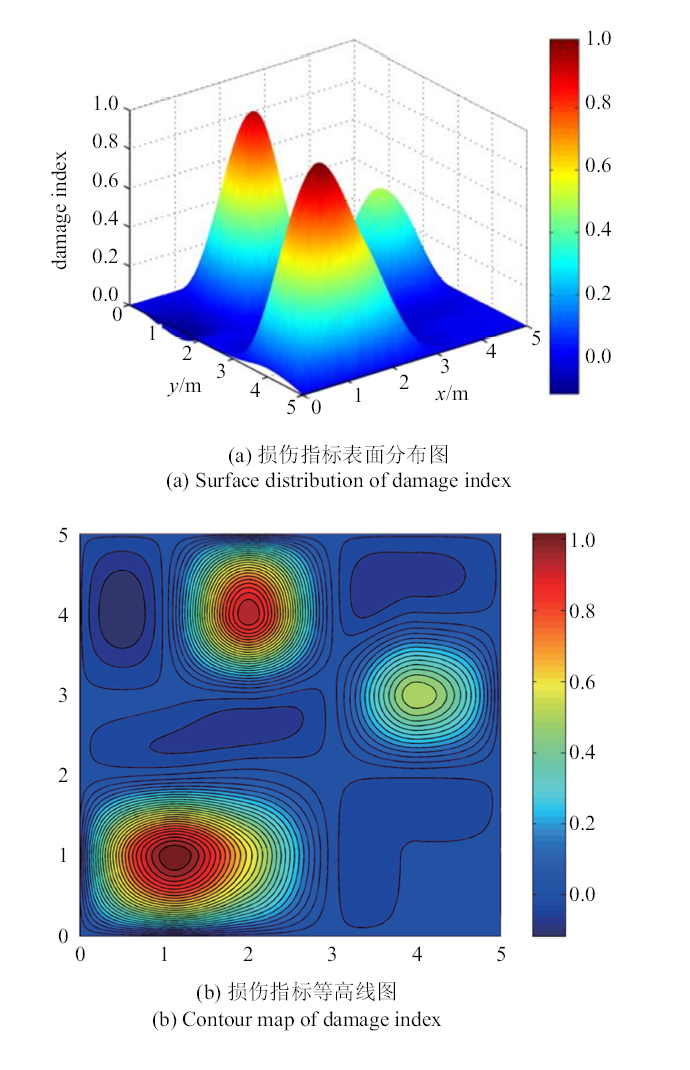
图8损伤指标表面分布图及其等高线图
Fig.8Surface distribution map and contour map of damage index
从图8中可以清楚地看出结构内部含3个缺陷, 且各自的位置范围均可初步得到.运用由加权系数改进目标函数的人工蜂群算法在这些缺陷各自所在搜索范围内进行精确反演,其中人工蜂群算法的各控制变量设为: 蜜源数量$N_{\rm Food} = 100$,最大搜索限定次数$N_{\rm Limit}= 20$, 最大迭代次数$N_{\rm Iter} = 200$.在运用人工蜂群算法进行反演之前, 剔除权重较小的传感器, 如图9所示,其中红色方块表示被剔除的传感器, 蓝色方块为剩下的传感器.此时待反演参数为各圆形缺陷的圆心坐标($x$, $y$)及圆形缺陷的半径$r$,得到如图10所示的反演迭代过程图, 图中红色圆表示反演结果,黑色圆表示实际缺陷位置及大小.运用本文方法进行反演过程中的目标函数值如表2所示.
图9
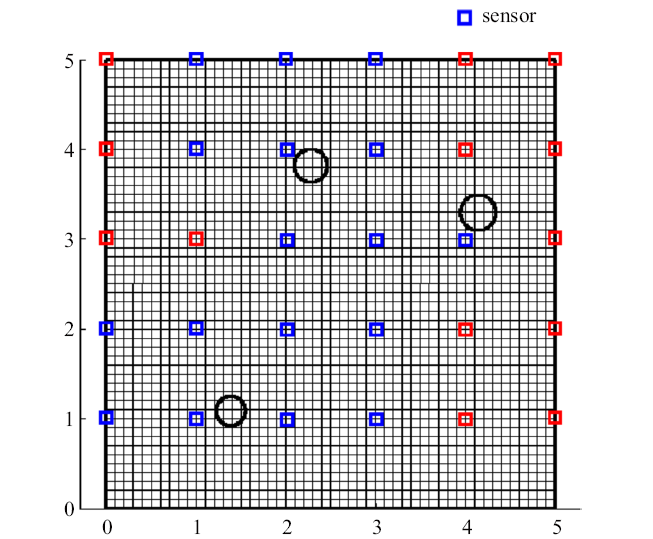
图9剔除小权重传感器的方形板
Fig.9Square plate with small weight sensor excluded
图10
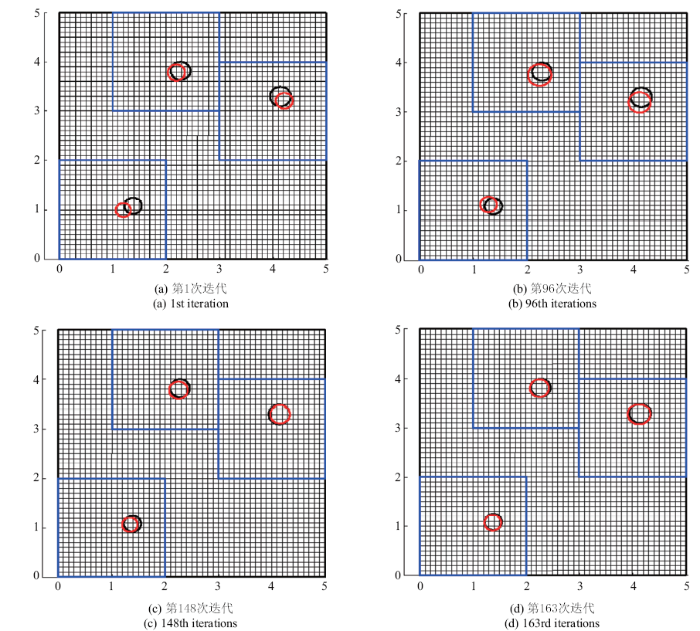
图10利用人工蜂群算法在缩小的范围内进行搜索
Fig.10Search within a reduced range by using artificial bee colony algorithm
Table 2
表2
表2人工蜂群算法进行三圆缺陷反演的迭代过程表
Table 2
![]() |
新窗口打开|下载CSV
从图10可看出, 通过损伤指标进行插值得到缺陷具体数目及范围后, 利用ABC算法进行精确反演可以很快得到缺陷的具体位置及大小, 省去了两阶段反演模型中第1阶段为得到缺陷数目而进行的大量迭代,节省了大量计算时间, 提高了工作效率.
将新提出的损伤指标法结合改进目标函数的反演算法与常规引入拓扑变量的ABC反演等方法进行对比,图11为不同模型得到的曲线收敛图, 图中各符号含义同图6.
图11
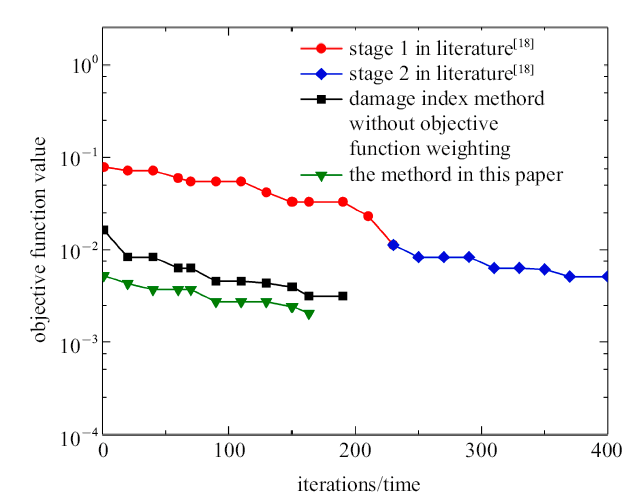
图11损伤指标法与拓扑变量法收敛曲线比较图
Fig.11Comparison of convergence curves of inversion process of three different defect recognition methods
从图11可以看出利用所提出的损伤指标法首先得到缺陷的数目及范围,再结合改进目标函数的反演算法得到缺陷具体位置及大小的方法,较以往引入拓扑变量进行反演的算法模型省去了第1阶段为确定缺陷数目进行的反复迭代过程,且进一步使用加权系数改进目标函数的模型进行反演,速率及准确度均优于未改进目标函数的反演算法,说明本文提出的损伤指标结合改进目标函数的方法收敛到真实缺陷的效率更高.
4.3 钢筋混凝土板含两个圆形缺陷的识别
一含两个圆形孔洞缺陷的钢筋混凝土板如图12(a)所示,模型简化自某河口闸站的导流墙.板内两圆形缺陷圆心坐标分别为(3.25 m, 3.73 m)及(1.75 m, 2.23 m),半径分别为0.27 m及0.26 m. 板的下侧受固定约束, 右侧受冲击荷载$P$激励.反演模型采用组合式钢筋混凝土有限元模型, 将钢筋植入混凝土单元的内部,两种单元的组合服从位移协调条件: 钢筋单元的结点位移服从混凝土单元的位移场.假定板处于平面应力状态, 基体的弹性模量$E = 30$~GPa, 泊松比$v = 0.167$, 钢筋的弹性模量$E = 200$~GPa, 横向钢筋的直径为16 mm, 竖向钢筋的直径为18 mm,板内钢筋的分布情况见图12(b)所示.图12
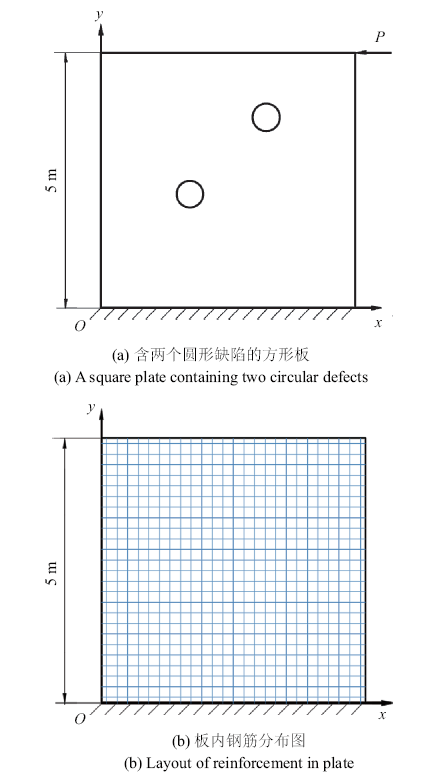
图12板的载荷、约束信息及钢筋布置情况
Fig.12Load, constraint information and reinforcement layout of the board
将板划分为$50\times 50$的网格, 其中真实含缺陷结构模型如图13,无损结构模型的网格划分同算例4.1中图2(c).由动力扩展有限元分别计算得到两个模型各测点的动力响应之后,将这些动力响应值代入式(7)、式(8)计算各测点位置传感器权重,并结合式(9)对其进行插值成像,得到如图14(a)的损伤指标表面分布图和图14(b)所示的损伤指标等高线图.即可根据该分布图及等高线图得到缺陷的数目及位置范围信息.
图13
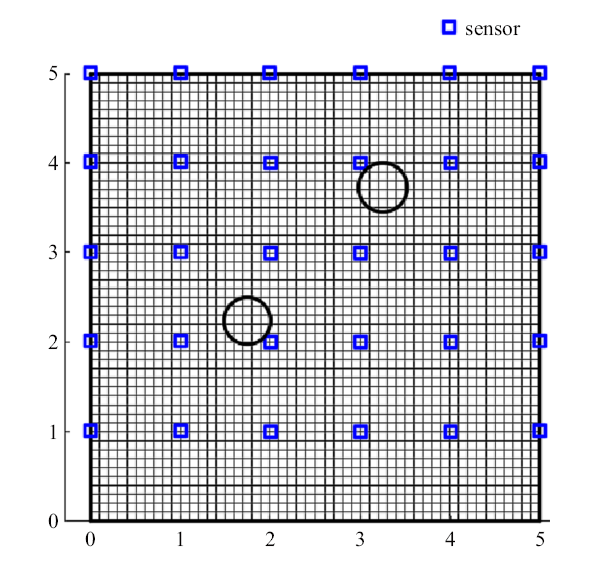
图13含缺陷的真实结构模型
Fig.13Real structural model with defects
图14
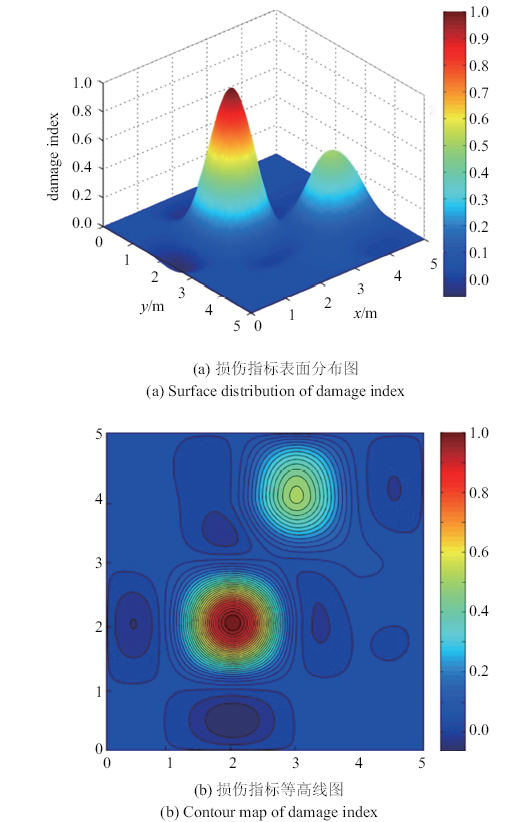
图14损伤指标表面分布图及其等高线图
Fig.14Surface distribution map and contour map of damage index
从图14中可以清楚地看出结构内部含两个缺陷, 且各自位置范围均可初步得到.运用由加权系数改进目标函数的ABC算法在这些缺陷各自所在搜索范围内进行精确反演,其中ABC算法各控制变量设为: 蜜源数量$N_{\rm Food} = 100$,最大搜索限定次数$N_{\rm Limit }= 20$, 最大迭代次数$N_{\rm Iter} = 200$. 在运用ABC算法进行反演之前, 剔除权重较小的传感器,此时待反演参数为各圆形缺陷的圆心坐标($x$, $y$)及缺陷半径$r$,得到如图15所示的反演迭代过程图, 图中红色圆表示反演结果,黑色圆表示实际缺陷位置及大小.运用本文方法进行反演过程中的目标函数值如表3所示.
图15
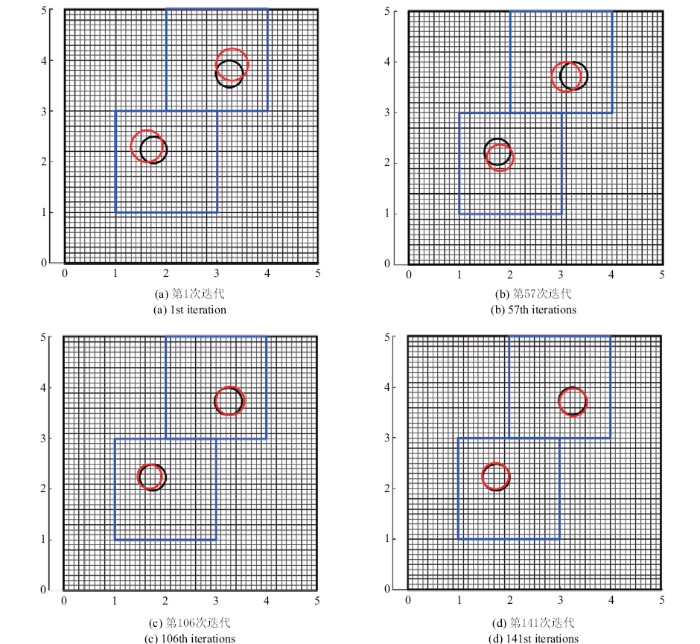
图15利用人工蜂群算法在缩小的范围内进行搜索
Fig.15Search within a reduced range by using artificial bee colony algorithm
Table 3
表3
表3人工蜂群算法进行双圆缺陷反演的迭代过程表
Table 3
![]() |
新窗口打开|下载CSV
从表3及图15可看出, 通过损伤指标进行插值得到缺陷具体数目及范围后,利用ABC算法进行精确反演可以很快得到缺陷的具体位置及大小,省去了两阶段反演模型中第1阶段为得到缺陷数目而进行的大量迭代,节省了大量计算时间, 提高了工作效率.
5 结论
本文利用距离缺陷不同位置处传感器实测响应与无损结构响应的差异,构建了传感器的不同权重, 再运用双三次插值法对权重进行插值得到各点损伤指标,结合插值成像技术得到缺陷数目及缺陷区域, 最后结合改进目标函数的智能反演算法进行缺陷精确反演得到最终缺陷参数.若干算例结果表明:损伤指标法能够在缺陷数目未知的情况下通过正向建模快速得出缺陷具体数目及位置范围,避免了两阶段反演方法需要的多次迭代以确定缺陷数目的繁杂计算.直接通过正向建模一次得出缺陷的数目及初步位置,将原来缺陷数目未知的反演问题转化为简单的缺陷数目已知的问题,提高了缺陷识别效率并降低了识别的难度和计算量;提出的加权系数改进目标函数的方法较以往未改进目标函数的智能算法,进一步提高了反演的速率和精确度.参考文献 原文顺序
文献年度倒序
文中引用次数倒序
被引期刊影响因子
DOIURL [本文引用: 1]
DOIURL
[本文引用: 1]
[本文引用: 1]
[本文引用: 1]
DOIURL
[本文引用: 1]
[本文引用: 1]
[本文引用: 1]
[本文引用: 1]
DOIURLPMID [本文引用: 1]

Heart failure is a major cause of morbidity and mortality worldwide. LongShengZhi capsule (LSZ), a traditional Chinese medicine, is used for treatment of patients with vascular diseases. Herein we investigated the effect of LSZ treatment on doxorubicin (DOX)-induced heart failure in mice. C57BL/6 mice randomly in 3 groups received following treatment: Control group, mice were fed normal chow; DOX group, mice were intraperitoneally injected DOX to induce heart failure and fed normal chow; and LSZ group, mice were injected DOX and fed normal chow containing LSZ. DOX induced heart failure as evidenced by increased serum creatine kinase, lactic dehydrogenase and α-hydroxybutyrate dehydrogenase, and cardiac fibrosis. However, LSZ treatment substantially inhibited DOX-induced heart failure parameters. Mechanistically, LSZ reduced collagen content and fibrosis by inhibiting expression of collagen type I α1 (COL1α1), COL1α2, α-smooth muscle actin and transforming growth factor β1. In addition, DOX-induced cell apoptosis was inhibited by LSZ, coupled with reduced caspase 3 activity and mRNA expression. LSZ decreased inflammatory cytokine levels. More importantly, LSZ decreased oxidative stress by inducing expression of anti-oxidative stress enzymes including superoxide dismutase 1 (SOD1), SOD2, catalase and glutathione peroxidase 1 through activation of forkhead box O3A and sirtuin 3. In conclusion, our study demonstrates that LSZ reduces heart failure by reducing production of reactive oxygen species and inhibiting inflammation/apoptosis. Our study also suggests the potential application of LSZ for heart failure treatment.
[本文引用: 1]
[本文引用: 1]
[本文引用: 1]
[本文引用: 1]
DOIURL [本文引用: 2]
DOIURL [本文引用: 1]

主要研究了扩展有限元法(extended finite element method, XFEM)在处理弱不连续问题时不同改进函数形式对XFEM数值求解精度的影响,阐述了各种改进函数影响XFEM求解精度的关键因素,指出校正的扩展有限元法(corrected-XFEM)能够提高数值求解精度的实质在于它拓展了改进结点域,即将常规扩展有限元法(standard-XFEM)的改进结点域增加一层作为corrected-XFEM的改进结点域,文中建议延拓corrected-XFEM的改进结点域,即在corrected-XFEM的改进结点域基础上再增加一层改进结点. 利用水平集函数表征材料内部的不连续界面,推导了XFEM求解的支配方程,给出了一种改进单元的数值积分方案以及改进单元处高精度应力的求解方法. 含夹杂问题的数值计算结果表明:建议的延拓corrected-XFEM改进结点域的方法能够明显提高XFEM的数值求解精度.
DOIURL [本文引用: 1]

主要研究了扩展有限元法(extended finite element method, XFEM)在处理弱不连续问题时不同改进函数形式对XFEM数值求解精度的影响,阐述了各种改进函数影响XFEM求解精度的关键因素,指出校正的扩展有限元法(corrected-XFEM)能够提高数值求解精度的实质在于它拓展了改进结点域,即将常规扩展有限元法(standard-XFEM)的改进结点域增加一层作为corrected-XFEM的改进结点域,文中建议延拓corrected-XFEM的改进结点域,即在corrected-XFEM的改进结点域基础上再增加一层改进结点. 利用水平集函数表征材料内部的不连续界面,推导了XFEM求解的支配方程,给出了一种改进单元的数值积分方案以及改进单元处高精度应力的求解方法. 含夹杂问题的数值计算结果表明:建议的延拓corrected-XFEM改进结点域的方法能够明显提高XFEM的数值求解精度.
URL [本文引用: 1]

For the structures containing multiple discontinuities (voids, inclusions, and cracks), the simulation technologies in the framework of extended finite element method (XFEM) are discussed in details. The level set method is used for representing the location of inner discontinuous interfaces so that the mesh does not need to align with these discontinuities. Several illustrations have been given to verify that the implemented XFEM program is effective. Then, the implemented XFEM program is used to investigate the effects of the voids, inclusions, and minor cracks on the path of major crack propagation. For a plate containing cracks and voids, two possibly crack path can be observed: i) the crack propagates into the void; ii) the crack initially curves towards the void, then, the crack reorients itself and propagates along its original orientation. For a plate with a soft inclusion, the final predicted crack paths tend to close with the inclusion, and an evident difference of crack paths can be observed with different inclusion material properties. However, for a plate with a hard inclusion, the paths tend to away from the inclusion, and a slightly difference of crack paths can only be seen with different inclusion material properties. For a plate with several minor cracks, the trend of crack paths can still be described as that the crack initially curves towards these minor cracks, and then, the crack reorients itself and propagates almost horizontally along its original orientation.
DOIURL [本文引用: 1]
DOIURLPMID [本文引用: 1]

Active development of quantum informational components such as quantum computers and quantum key distribution systems requires parameter characterization of single photon detectors. A key property of the single photon detectors is detection efficiency. One of the methods of the detection efficiency measurement, as listed in the international standard ETSI, is the reference-free twin-photon-based Klyshko method. The signal-to-noise ratio (SNR) of this method depends on the combination of the pump wavelength, the nonlinear crystal's axis angle, and the type of detector's sensitive element. When the combination is difficult, one has to deal with the low SNR of the detector counts measurement. To gain the high SNR, one has to average the long record complicated with the "random telegraph signal" noise. This type of noise exhibits high spectral density at a zero frequency, where simple averaging works. The heterodyne based method we have proposed is to perform averaging at the higher frequency of the modulation introduced to the standard Klyshko measurement scheme. The method was numerically simulated and experimentally tested. The 14 times improvement in SNR for the proposed method relative to the simple averaging was demonstrated by the numerical simulation and confirmed experimentally.
DOIURL [本文引用: 1]
DOIURLPMID [本文引用: 1]

The main aim of this article is to compare various heating methods of the shrink-fit connection and to analyse its shrinkage mechanism. The shrink fit connection consists of the crank and the pin or the ring and the pin, which have negative value of the geometrical clearance. The experimental part of research included the measurement of the temperature at the various points in the surface of the crank, the measurement of the residual stress 5 mm from the crank hole and the measurement of the maximum torsional moment, at which sliding of the shrink-fit connection occurs. Conformity between data from the Finite Element Analysis and the experimental data was obtained in relation to the von Mises stress. The analysis results show that Lamé equations can be successfully used for calculation of the load capacity even for parts with the irregular shape.
[硕士论文].
[本文引用: 3]
[Master Thesis].
[本文引用: 3]
DOIURL [本文引用: 1]
DOIURL [本文引用: 1]

We present a novel algorithm based on the extended finite element method (XFEM) and an enhanced artificial bee colony (EABC) algorithm to detect and quantify multiple flaws in structures. The concept is based on recent work that have shown the excellent synergy between XFEM, used to model the forward problem, and a genetic-type algorithm to solve an inverse identification problem and converge to the ` best' flaw parameters.
In this paper, an adaptive algorithm that can detect multiple flaws without any knowledge on the number of flaws beforehand is proposed. The algorithm is based on the introduction of topological variables into the search space, used to adaptively activate/deactivate flaws during run time until convergence is reached. The identification is based on a limited number of strain sensors assumed to be attached to the structure surface boundaries. Each flaw is approximated by a circular void with the following three variables: center coordinates (x(c), y(c)) and radius (r(c)), within the XFEM framework. In addition, the proposed EABC scheme is improved by a guided-to-best solution updating strategy and a local search (LS) operator of the NelderMead simplex type that show fast convergence and superior global/LS abilities compared with the standard ABC or classic genetic algorithms.
Several numerical examples, with increasing level of difficulty, are studied in order to evaluate the proposed algorithm. In particular, we consider identification ofmultiple flaws with unknown a priori information on the number of flaws (which makes the inverse problem harder), the proximity of flaws, flaws having irregular shapes (similar to artificial noise), and the effect of structured/unstructured meshes. The results show that the proposed XFEM-EABC algorithm is able to converge on all test problems and accurately identify flaws. Hence, this methodology is found to be robust and efficient for nondestructive detection and quantification of multiple flaws in structures. Copyright (C) 2013 John Wiley & Sons, Ltd.
[本文引用: 1]
[本文引用: 1]
[本文引用: 1]
DOIURLPMID [本文引用: 1]

Active development of quantum informational components such as quantum computers and quantum key distribution systems requires parameter characterization of single photon detectors. A key property of the single photon detectors is detection efficiency. One of the methods of the detection efficiency measurement, as listed in the international standard ETSI, is the reference-free twin-photon-based Klyshko method. The signal-to-noise ratio (SNR) of this method depends on the combination of the pump wavelength, the nonlinear crystal's axis angle, and the type of detector's sensitive element. When the combination is difficult, one has to deal with the low SNR of the detector counts measurement. To gain the high SNR, one has to average the long record complicated with the "random telegraph signal" noise. This type of noise exhibits high spectral density at a zero frequency, where simple averaging works. The heterodyne based method we have proposed is to perform averaging at the higher frequency of the modulation introduced to the standard Klyshko measurement scheme. The method was numerically simulated and experimentally tested. The 14 times improvement in SNR for the proposed method relative to the simple averaging was demonstrated by the numerical simulation and confirmed experimentally.
DOIURL [本文引用: 1]

传统的结构检测方法一般需要钻孔取样,对结构本身有一定的破坏作用,而无损检测方法在检测过程中不破坏结构本身,这项技术的重要性日益显著. 结合扩展有限元法和人工蜂群智能优化算法的优点,建立了结构内部缺陷(夹杂)的反演分析模型,为结构的无损检测技术提供了一条新的途径.扩展有限元法通过引入非连续位移模式可以在不重新划分网格的情况下通过改变水平集函数反映缺陷(夹杂)的位置及大小,避免了反演分析每次迭代过程中的网格重剖分,人工蜂群智能优化算法在每次迭代中都采用全局和局部搜索,找到最优解的概率大大增加并可很好地避免局部最优,因此,扩展有限元法与人工蜂群智能优化算法的结合有效地减少了反演分析的计算工作量. 通过若干算例的分析表明:建立的反演分析模型能准确地探测结构内部存在的单个缺陷(夹杂).
DOIURL [本文引用: 1]

传统的结构检测方法一般需要钻孔取样,对结构本身有一定的破坏作用,而无损检测方法在检测过程中不破坏结构本身,这项技术的重要性日益显著. 结合扩展有限元法和人工蜂群智能优化算法的优点,建立了结构内部缺陷(夹杂)的反演分析模型,为结构的无损检测技术提供了一条新的途径.扩展有限元法通过引入非连续位移模式可以在不重新划分网格的情况下通过改变水平集函数反映缺陷(夹杂)的位置及大小,避免了反演分析每次迭代过程中的网格重剖分,人工蜂群智能优化算法在每次迭代中都采用全局和局部搜索,找到最优解的概率大大增加并可很好地避免局部最优,因此,扩展有限元法与人工蜂群智能优化算法的结合有效地减少了反演分析的计算工作量. 通过若干算例的分析表明:建立的反演分析模型能准确地探测结构内部存在的单个缺陷(夹杂).
[本文引用: 1]
[本文引用: 1]
DOIURL [本文引用: 1]
DOIURLPMID [本文引用: 1]

The main aim of this article is to compare various heating methods of the shrink-fit connection and to analyse its shrinkage mechanism. The shrink fit connection consists of the crank and the pin or the ring and the pin, which have negative value of the geometrical clearance. The experimental part of research included the measurement of the temperature at the various points in the surface of the crank, the measurement of the residual stress 5 mm from the crank hole and the measurement of the maximum torsional moment, at which sliding of the shrink-fit connection occurs. Conformity between data from the Finite Element Analysis and the experimental data was obtained in relation to the von Mises stress. The analysis results show that Lamé equations can be successfully used for calculation of the load capacity even for parts with the irregular shape.
DOIURL [本文引用: 1]
[本文引用: 1]
[本文引用: 1]
DOIURL [本文引用: 1]

Swarm intelligence is a research branch that models the population of interacting agents or swarms that are able to self-organize. An ant colony, a flock of birds or an immune system is a typical example of a swarm system. Bees’ swarming around their hive is another example of swarm intelligence. Artificial Bee Colony (ABC) Algorithm is an optimization algorithm based on the intelligent behaviour of honey bee swarm. In this work, ABC algorithm is used for optimizing multivariable functions and the results produced by ABC, Genetic Algorithm (GA), Particle Swarm Algorithm (PSO) and Particle Swarm Inspired Evolutionary Algorithm (PS-EA) have been compared. The results showed that ABC outperforms the other algorithms.