
Correlation studies of distinct mutational signatures with common cancer pathological subtyping
Yue Shi, Zhengzheng Xu, Huan Lu, Weimin Ci
通讯作者:
编委: 孙玉洁
收稿日期:2018-07-19修回日期:2018-10-21网络出版日期:2018-11-20
Received:2018-07-19Revised:2018-10-21Online:2018-11-20
作者简介 About authors
史悦,博士,助理研究员,研究方向:肿瘤表观基因组学E-mail:shiyue@big.ac.cn。

摘要
关键词:
Abstract
Keywords:
PDF (896KB)元数据多维度评价相关文章导出EndNote|Ris|Bibtex收藏本文
本文引用格式
史悦, 许争争, 鲁欢, 慈维敏. 肿瘤突变特征与病理分型的关联研究[J]. 遗传, 2018, 40(11): 1033-1038 doi:10.16288/j.yczz.18-208
Yue Shi, Zhengzheng Xu, Huan Lu, Weimin Ci.
癌症被认为是一类源于细胞突变的疾病。细胞分裂次数多的组织,罹患肿瘤的几率相对较高。近期研究发现来源于DNA复制错误产生的突变约占人类癌症突变总数的2/3[1,2]。该结论引起科学界的强烈辩论和公众的迷惑。研究发现同种肿瘤内也存在巨大的异质性,不同的病理亚型可能细胞来源不同,其细胞复制的活跃程度不同,肿瘤的病理亚型及临床特征都可能不同[3]。而每一种突变过程根据DNA损伤、DNA修复或者复制机制等的不同在癌基因组上都留有一定的“印迹”,产生不同的突变组合,即“突变特征”[4]。
传统的肿瘤分型主要依赖于HE染色以及免疫组织化学法对癌组织进行病理学分析。这就和“活检”取材的大小、部位,肿瘤的进展程度等有一定关联。临床上获取患者肿瘤组织样本只有手术活检及穿刺活检两种方法。如果是早期肿瘤,可能肿瘤细胞本身量少以及形态学并未发生明显变化而导致传统检测方法无法鉴定出肿瘤。但实际上肿瘤细胞的基因组已积累了大量突变,形成了各自特异的“突变特征”。因为不同肿瘤病理亚型,代表的是不同的肿瘤细胞来源,其肿瘤发生发展的致病因素包括突变特征在内会存在一定差异,因此我们认为可以通过不同突变特征区分不同病理亚型,同时肿瘤的突变图谱还可能作为一类生物标记物为我们对某类癌症的诊断,尤其是早期诊断提供参考及帮助。本文拟探索在不同种肿瘤中突变累积的图谱特征和病理分型的关系。利用TCGA肾癌、食管癌和肺癌的测序数据我们发现相同病理亚型的肿瘤趋向聚类到一起。一些突变特征还可进一步将细胞来源不同的癌症亚型区分开,而且其中两种肾癌亚型预测的准确率达到了100%。同样地,突变特征在区分肺腺癌和肺鳞状细胞癌以及食管腺癌和食管鳞状细胞癌的准确率也分别达到了78%和84%。我们认为突变特征可作为一种新型分子标记物,对肿瘤的病理分型、诊断尤其是早诊具有一定的参考意义。
1 材料和方法
1.1 肿瘤患者相关数据来源和收集
本研究选择了3种常见癌症(肾癌、肺癌和食管癌),深入探索突变特征对提高癌症病理分型鉴定的作用。已知文献报道肾癌的主要病理类型是起源于近曲小管的透明肾细胞癌(clear cell renal cell carcinoma, KIRC),而嫌色肾细胞癌(chromophobe renal cell carcinoma, KICH)起源于肾集合小管暗细胞[5]。而肺腺癌(lung adenocarcinoma, LUAD)主要起源于支气管粘膜上皮,少数起源于大支气管的粘液腺,肺鳞状细胞癌(lung squamous cell carcinoma, LUSC)主要起源于支气管粘膜上皮,是发展中国家的主要病理类型,居于第二位,约占所有病理类型肺癌的30%[6]。与此类似,食管鳞状细胞癌(esophageal squamous cell carcinoma, ESCC)也是一类非常常见的恶性肿瘤,起源于食管的扁平细胞,最常见于食管的上部和中部。而且在亚洲某些地区食管鳞状细胞癌的发病率要高于起源于食管下1/3的Barrett粘膜的腺管状的食管腺癌(esophageal adenocarcinoma, EAC)[7]。为了鉴定突变特征是否可预测常见癌症的病理分型,本研究收集TCGA数据库中透明肾细胞癌和嫌色肾细胞癌患者的全外显子组单核苷酸突变(single nucleotide variants, SNV)数据,其中透明肾细胞癌451例,嫌色肾细胞癌66例;肺腺癌543例,肺鳞状细胞癌178例以及96例食管鳞状细胞癌患者和88例食管腺癌的全外显子组单核苷酸突变数据。
1.2 肿瘤特异突变特征的选择
癌症体细胞突变目录(Catalog of Somatic Mutations in Cancer,COSMIC)数据库目前根据癌症基因组数据库(The Cancer Genome Atlas, TCGA)收录的40多种不同肿瘤的外显子组和全基因组测序数据,共发现6种DNA碱基的变异(C>A、C>G、C>T、T>A、T>C和T>G),考虑到变异碱基上游和下游各有4种碱基类型,则会出现96类三核苷酸的突变类型[3,8~10]。针对每一个样本的单核苷酸突变都能计算96种突变形式的比例。由于DNA复制、DNA损伤物质暴露、修复缺失等不同原因产生的突变类型具有某种特异性即96种突变形式的比例组成不同,这被称作该种肿瘤的突变特征[4]。1.3 突变特征分析方法
突变特征计算:首先利用deconstructsigs R https://cran.r-project.org/web/packages/deconstructSigs/index.html)对从TCGA下载的肾癌、肺癌、食管癌外显子数据进行突变特征分析做突变特征分析[11]。考虑到某些肿瘤突变负荷(tumor mutation burden)低,处于外显子的突变数目可能少于96个,因此本研究进一步将突变类型简化,将96个突变形式中第一个碱基例如AT>CG,CT>CG,TT>CG,GT>CG合并为一种T>CG,最终成为24种突变形式。突变特征预测模型分析:对各样本进行随机排序并按照1:9模式将数据拆分成测试集合和训练集合。接着利用randomForest (https://cran.r-project. org/src/contrib/Archive/randomForest/) R包进行建模并用测试集合进行模型检验。最后结合ROCR包(https://cran.r-project.org/src/contrib/Archive/ROCR/)进行ROC曲线展示模型预测效果。
2 结果与分析
2.1 突变特征可以准确、特异地区分两种不同病理亚型的肾癌
本研究下载TCGA中肾癌的外显子组数据,首先关注两种不同病理亚型的肾癌:来源于近曲小管的透明肾细胞癌和来源于远曲小管的嫌色细胞癌(图1A)。每组各选择了1个典型病例,检测对应的24个突变形式的分布情况。图1B表明,两个不同病理亚型的肾癌突变特征显著不同,KIRC以T>A变异比例最高,而KICH以C>T的变异比例最高。进一步采用非监督聚类的方法,结果发现虽然同一病理亚型的患者相同突变类型频率存在差异,但同一病理亚型的患者更趋向聚类到一起(图1C)。为进一步确定这24种突变特征哪些能够将同种癌症不同病理亚型有效分开,随后用随机森林法进行了特征选择分析。与预期一致,随机森林法选择出来的突变特征可以很好的将细胞来源不同的两组肾癌亚型KIRC和KICH分开,而且准确率达到了100% (95% confidence interval (CI): 0.93~1.00) (图 1D)。上述结果表明,突变特征可以准确、特异地区分两种不同细胞来源的肾癌病理亚型。图1
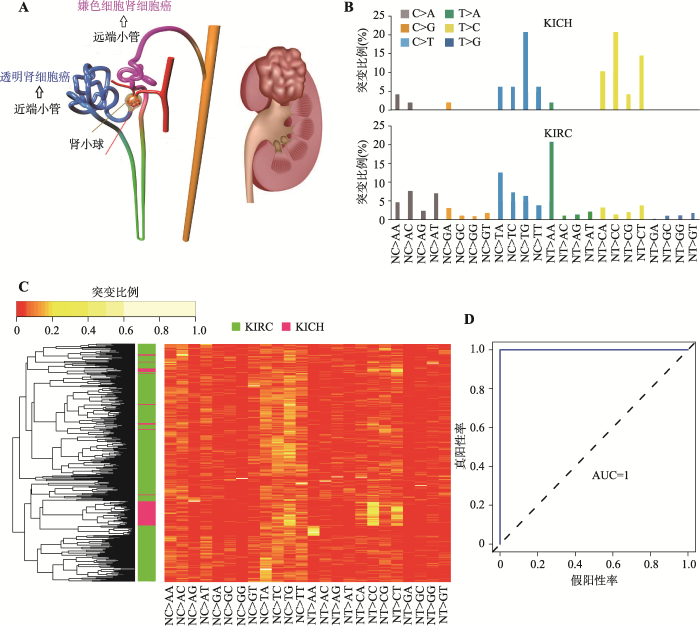
图1透明肾细胞癌和嫌色肾细胞癌能够被突变特征准确、特异地区分
A:肾癌两种不同病理亚型的细胞来源示意图。B:肿瘤患者的突变特征谱。两个肾癌亚型中分别选取1例患者。C:突变特征谱的非监督聚类。D:随机森林法选取突变特征对肾癌两种不同亚型分类准确性的ROC曲线。KIRC和KICH分别代表透明肾细胞癌和嫌色肾细胞癌。
Fig. 1Mutational signatures predict histological subtypes within kidney cancers
2.2 突变特征可以准确、特异地区分两种不同病理亚型的肺癌和食管癌
鳞癌和腺癌是多种肿瘤中都存在的两个病理亚型,其治疗方式和对放化疗的敏感性均存在显著差异,因此病理类型的诊断对于癌症病人来说至关重要。为进一步探究通过突变特征预测肿瘤病理分型的可行性和准确度,本研究继续选取了两种常见的肿瘤肺癌和食管癌进行突变特征与肿瘤病理亚型(鳞癌和腺癌)的相关性和预测研究。类似地,在肺癌和食管癌中,非监督聚类方法显示:同一病理类型的患者趋向聚类在一起(图2,A和B)。进一步用随机森林法选择可以用来区分两种病理类型的突变特征,在肺癌中分类准确性达到了78% (95% CI: 0.66~ 0.86) (图2C),而在食管癌中达到了84% (95% CI: 0.60~0.97) (图2D)。并且,在不同类型肿瘤中相同病理亚型的患者突变特征总体比较相似,略有不同,暗示细胞来源以及致癌因素共同刻画了肿瘤中的突变特征(图2E)。上述结果表明,突变特征本身在很大程度上就可以代表了常见癌症不同病理亚型的“自然属性”,从而将其区分开来。图2
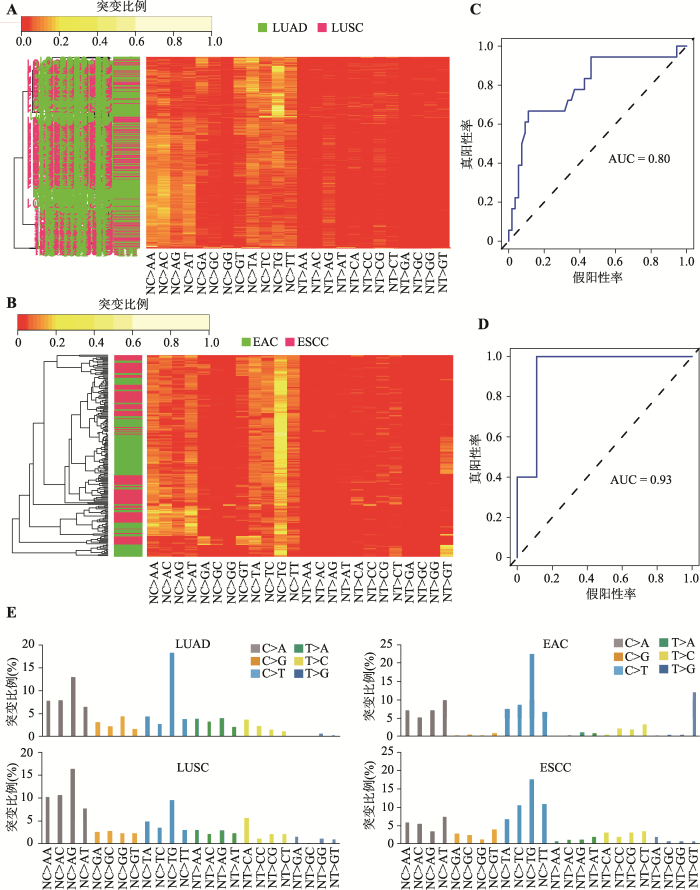
图2在肺癌和食管癌中突变特征可以准确、特异地区分各自不同的病理亚型
A:肺癌两种不同病理亚型的突变特征谱非监督聚类;B:食管癌两种不同病理亚型的突变特征谱非监督聚类;C:随机森林法选取突变特征对肺癌两种不同亚型分类准确性的ROC曲线;D:随机森林法选取突变特征对食管癌癌两种不同亚型分类准确性的ROC曲线;E:肿瘤患者的突变特征谱。肺癌和食管癌中两种亚型中分别选取1例患者。LUAD和LUSC分别代表肺腺癌和肺鳞状细胞癌。EAC和ESCC分别代表食管腺癌和食管鳞状细胞癌。
Fig. 2Mutational signatures predict histological subtypes within lung cancers and esophageal cancers
3 讨 论
病理类型的诊断对于癌症病人来说至关重要,因为其能预测病人对不同治疗方式的敏感度以及药物的毒性反应。经典的癌症病理类型鉴定是利用HE染色以及免疫组织化学法对癌组织进行病理学分析,而对于穿刺等活检样本(样本的组织空间结构信息不完整)的诊断灵敏性有待提高。如早期肿瘤患者,有可能由于处于进展早期以及取材的限制,利用经典的HE染色和免疫组化法不一定能观察到肿瘤细胞,及其组织形态的明显改变。但实际上这些肿瘤细胞已积累了相当多的突变,形成了各自的“突变特征”。如果利用基因组测序和分析技术就可以弥补这一不足,提高病理诊断的灵敏度。本文尝试将病理分型和基因组学相结合,展示了利用突变特征对3种常见肿瘤不同病理亚型进行分类的可行性。研究结果也表明突变特征可以作为辅助手段来帮助更多不同种癌症的鉴定。美国食品药物管理局在2017年5月23号批准同意了首款不依据肿瘤来源,而是依据生物标志物进行区分的抗肿瘤药物——Keytruda[12],用于治疗携带某特定基因特征的多种实体瘤。Keytruda是针对PD-1/PD-L1通路发挥的作用。通过阻碍这一通路,Keytruda可帮助人体免疫系统对抗癌细胞。而以往FDA一直依据癌症的发病部位批准治疗方法,如肺癌,胃癌等。这是第一次基于肿瘤生物标志物而非肿瘤的发生部位批准的一个新药。这预示着今后深入探索突变特征在癌症病人精准化医疗的临床应用价值将是一个有利方向。例如本文所述的突变特征就可能作为一类早期诊断中液体活检或者临床预后等的生物标记。相反,如果仅仅只检测体细胞特定基因的突变对鉴定某类癌症的敏感性和特异性均不佳,不仅突变频率低,而且重现性突变比例也很低[13]。因此,利用常见的体细胞染色体拷贝数变化或者突变特征作为生物标记分子,预期可提高早诊的特异性和敏感度。综上所述,突变特征作为新型分子标记物,对肿瘤的病理分型、诊断尤其是早诊的预测具有一定的参考意义。
参考文献 原文顺序
文献年度倒序
文中引用次数倒序
被引期刊影响因子
URL [本文引用: 1]
URLPMID:28336671 [本文引用: 1]

Abstract Cancers are caused by mutations that may be inherited, induced by environmental factors, or result from DNA replication errors (R). We studied the relationship between the number of normal stem cell divisions and the risk of 17 cancer types in 69 countries throughout the world. The data revealed a strong correlation (median = 0.80) between cancer incidence and normal stem cell divisions in all countries, regardless of their environment. The major role of R mutations in cancer etiology was supported by an independent approach, based solely on cancer genome sequencing and epidemiological data, which suggested that R mutations are responsible for two-thirds of the mutations in human cancers. All of these results are consistent with epidemiological estimates of the fraction of cancers that can be prevented by changes in the environment. Moreover, they accentuate the importance of early detection and intervention to reduce deaths from the many cancers arising from unavoidable R mutations. Copyright 2017, American Association for the Advancement of Science.
URLPMID:24981601 [本文引用: 2]

The collective somatic mutations observed in a cancer are the outcome of multiple mutagenic processes that have been operative over the lifetime of a patient. Each process leaves a characteristic imprint - a mutational signature - on the cancer genome, which is defined by the type of DNA damage and DNA repair processes that result in base substitutions, insertions and deletions or structural variations. With the advent of whole-genome sequencing, researchers are identifying an increasing array of these signatures. Mutational signatures can be used as a physiological readout of the biological history of a cancer and also have potential use for discerning ongoing mutational processes from historical ones, thus possibly revealing new targets for anticancer therapies.
URLPMID:2023945592 [本文引用: 2]

All cancers are caused by somatic mutations; however, understanding of the biological processes generating these mutations is limited. The catalogue of somatic mutations from a cancer genome bears the signatures of the mutational processes that have been operative. Here we analysed 4,938,362 mutations from 7,042 cancers and extracted more than 20 distinct mutational signatures. Some are present in many cancer types, notably a signature attributed to the APOBEC family of cytidine deaminases, whereas others are confined to a single cancer class. Certain signatures are associated with age of the patient at cancer diagnosis, known mutagenic exposures or defects in DNA maintenance, but many are of cryptic origin. In addition to these genome-wide mutational signatures, hypermutation localized to small genomic regions, 'kataegis', is found in many cancer types. The results reveal the diversity of mutational processes underlying the development of cancer, with potential implications for understanding of cancer aetiology, prevention and therapy.
URLPMID:17621606 [本文引用: 1]

Recent advances in molecular genetics and immunocytochemistry have clarified the of origin in many . Several are thought to involve specific segments of the nephron. -secreting arise from juxtaglomerular . Clear and papillary renal (RCC) recapitulate the epithelium of the proximal tubules. and chromophobe RCC differentiate towards Type A and Type B intercalated of the cortical collecting duct, respectively. Medullary collecting ducts are the target sites for the development of , and . Renal papillae are susceptible to unique changes such as necrosis or . The purpose of our article is threefold: to illustrate the imaging findings of that show segmental involvement of the nephron, to describe proximal and distal nephron disorders and to correlate imaging findings of some entities with histopathological features.
URL [本文引用: 1]
URL [本文引用: 1]
URL [本文引用: 1]
URL
URLPMID:27899578 [本文引用: 1]

Abstract COSMIC, the Catalogue of Somatic Mutations in Cancer (http://cancer.sanger.ac.uk) is a high-resolution resource for exploring targets and trends in the genetics of human cancer. Currently the broadest database of mutations in cancer, the information in COSMIC is curated by expert scientists, primarily by scrutinizing large numbers of scientific publications. Over 4 million coding mutations are described in v78 (September 2016), combining genome-wide sequencing results from 28 366 tumours with complete manual curation of 23 489 individual publications focused on 186 key genes and 286 key fusion pairs across all cancers. Molecular profiling of large tumour numbers has also allowed the annotation of more than 13 million non-coding mutations, 18 029 gene fusions, 187 429 genome rearrangements, 1 271 436 abnormal copy number segments, 9 175 462 abnormal expression variants and 7 879 142 differentially methylated CpG dinucleotides. COSMIC now details the genetics of drug resistance, novel somatic gene mutations which allow a tumour to evade therapeutic cancer drugs. Focusing initially on highly characterized drugs and genes, COSMIC v78 contains wide resistance mutation profiles across 20 drugs, detailing the recurrence of 301 unique resistance alleles across 1934 drug-resistant tumours. All information from the COSMIC database is available freely on the COSMIC website. The Author(s) 2016. Published by Oxford University Press on behalf of Nucleic Acids Research.
URL [本文引用: 1]
URL [本文引用: 1]
URLPMID:24132290 [本文引用: 1]

The Cancer Genome Atlas (TCGA) has used the latest sequencing and analysis methods to identify somatic variants across thousands of tumours. Here we present data and analytical results for point mutations and small insertions/deletions from 3,281 tumours across 12 tumour types as part of the TCGA Pan-Cancer effort. We illustrate the distributions of mutation frequencies, types and contexts across tumour types, and establish their links to tissues of origin, environmental/carcinogen influences, and DNA repair defects. Using the integrated data sets, we identified 127 significantly mutated genes from well-known (for example, mitogen-activated protein kinase, phosphatidylinositol-3-OH kinase, Wnt/ -catenin and receptor tyrosine kinase signalling pathways, and cell cycle control) and emerging (for example, histone, histone modification, splicing, metabolism and proteolysis) cellular processes in cancer. The average number of mutations in these significantly mutated genes varies across tumour types; most tumours have two to six, indicating that the number of driver mutations required during oncogenesis is relatively small. Mutations in transcriptional factors/regulators show tissue specificity, whereas histone modifiers are often mutated across several cancer types. Clinical association analysis identifies genes having a significant effect on survival, and investigations of mutations with respect to clonal/subclonal architecture delineate their temporal orders during tumorigenesis. Taken together, these results lay the groundwork for developing new diagnostics and individualizing cancer treatment.