

A Method for the Automatic Determination of Scale Parameter During Segmenting Agricultural Drone Images
SHI YaJiao

通讯作者:
责任编辑: 杨鑫浩
收稿日期:2019-11-5接受日期:2020-02-9网络出版日期:2020-09-01
基金资助: |
Received:2019-11-5Accepted:2020-02-9Online:2020-09-01
作者简介 About authors
石雅娇,E-mail:

摘要
关键词:
Abstract
Keywords:
PDF (7457KB)元数据多维度评价相关文章导出EndNote|Ris|Bibtex收藏本文
本文引用格式
石雅娇, 陈鹏飞. 一种面向农业无人机影像分割的尺度参数自动确定方法[J]. 中国农业科学, 2020, 53(17): 3496-3508 doi:10.3864/j.issn.0578-1752.2020.17.007
SHI YaJiao, CHEN PengFei.
0 引言
【研究意义】相对于其他遥感平台,无人机遥感具有机动灵活的特点,容易获取高时间、空间分辨率影像,对于精准农业管理具有重要意义[1]。高空间分辨率的影像为准确区分作物植株与土壤等背景提供了数据支撑。长期以来,****们一直在设计各种光谱指数来去除土壤等背景的干扰[2,3,4]。如果基于高空间分辨率的无人机影像直接把土壤等背景去除掉,那对于提高作物生理生化参数反演精度具有重要意义。已有****的研究表明,基于高分辨率无人机影像剔除土壤背景可提高作物水分、植株氮浓度等参数的反演精度[5,6]。另外,准确提取田间作物,对于识别杂草、监测苗情等都非常重要。基于遥感影像区分作物与土壤背景,实际是对影像进行分类。对影像进行分类的方法包括人工目视解译法、基于像元的自动分类法和基于对象的自动分类。目前,已有研究在提取田间作物时,多采用目视解译法或基于像元分类法[6,7]。目视解译法工作量大,而像元法往往“椒盐现象”严重[8,9]。对于无人机超高空间分辨率影像来说,面向对象的分类方法往往能取得较好的结果[10,11]。面向对象分类法的基本流程是通过图像分割获得对象,然后对分割对象进行分类以达到识别目标地物的目的。因此,图像分割是面向对象遥感影像处理中的重要环节[12,13]。在分割对象时,需要设定尺度参数,它与对象大小密切相关。目前,研究人员多基于经验多次人工尝试获得,大大限制了其推广应用[14]。因此,针对无人机影像高精度自动分割需求,开展尺度参数自动确定方法研究,对精准农业管理具有重要意义。【前人研究进展】对于影像的自动分割,目前基于无人机影像的研究还较少,已有研究多基于高分辨率卫星影像数据开展[15,16,17,18]。但这些研究工作为开展无人机影像方面的相关应用提供了参考。根据“对象内部有较好的同质性,对象间有较好的异质性”的原则来构建评价函数,根据评价函数值变化的趋势,选择“变化速率最大”或“拐点”位置处的尺度参数值作为最优值,是已有尺度参数自动确定方法的核心思想[19,20,21,22]。构建对象内平均光谱角作为评价函数,YANG等[23]认为平均光谱角变化速率最快时所对应的尺度参数值为最优值。随后,针对平均光谱角只考虑对象内同质性的问题,YANG等[24]引入对象间光谱角作为对象间异质性来对前期研究进行改进。张俊等[25]在分割影像时,采用了与邻域绝对均值差分方差比作为评价分割尺度的指标,认为其局部峰值所对应的尺度为某一地物的最佳分割尺度,来实现尺度参数的自动确定;以局部方差(Local Variance,LV)[26]为切入点,王志华等[27]提出加权局部方差(weighted local variance,WLV)作为评价函数,根据评价函数“拐点”来自动确定分割尺度参数的方法。【本研究切入点】WLV法计算简单,较易实现,但其在分割时只考虑了对象间异质性,缺乏对同质性的考虑,可能会导致分割结果欠准确。【拟解决的关键问题】本研究提出了一种改进的分割尺度参数自动确定方法,并依托不同水、肥条件下获取的玉米、小麦多生育期、不同长势下无人机影像对改进前后的方法进行对比分析,为农业无人机影像自动分割提供新的技术支撑。1 材料与方法
1.1 田间试验
为满足实际应用需求,本研究构建了不同作物的田间试验,以丰富试验场景。1.1.1 玉米氮肥梯度试验 于2018年5月—10月在吉林省蔡家镇(124°40′25″E,43°24′00″N)开展试验。品种为“吉单558”。试验设5个处理,3个重复,共15个小区,各小区面积为7.8 m×13 m。其中,5个氮肥处理分别为0、70、140、210、280 kg·hm-2。除氮肥外,各小区其他管理措施相同,试验示意如图1所示。
图1
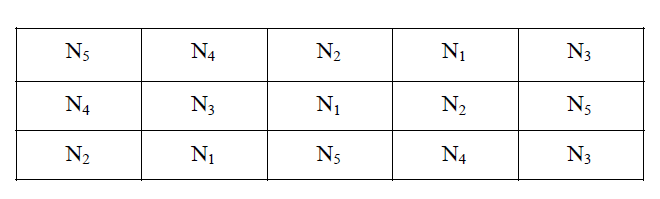
图1玉米氮肥试验示意图
N1—N5分别表示施肥量为0、70、140、210、280 kg·hm-2
Fig. 1Schematic diagram of the nitrogen experiment of corn
N1-N5 indicate applied nitrogen at 0, 70, 140, 210, 280 kg·hm-2
1.1.2 冬小麦水、氮耦合试验 试验于2018年10月至2019年6月在中国科学院禹城站(116°34′13″E,36°50′00″N)内开展。品种为“维麦8号”。试验设2个水分处理,5个氮肥处理,共32个小区,各小区面积为10 m×5 m。其中,水分处理分别为90 mm和60 mm灌溉量;氮肥处理分别为0、15 000 kg·hm-2厩肥、15 000 kg·hm-2厩肥+100 kg·hm-2氮肥、15 000 kg·hm-2厩肥+200 kg·hm-2氮肥、15 000 kg·hm-2厩肥+300 kg·hm-2氮肥。除氮肥外,各小区其他管理措施相同,试验示意如图2所示。
图2
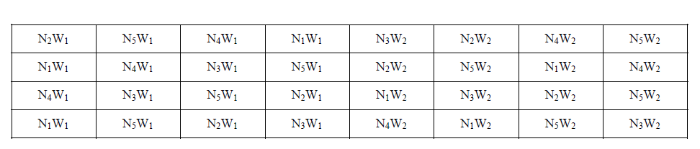
图2冬小麦水、氮耦合试验示意图
W1:90 mm灌溉量;W2:60 mm灌溉量;N1:不施肥;N2:15 000 kg·hm-2厩肥;N3:15 000 kg·hm-2厩肥+100 kg·hm-2氮肥;N4:15 000 kg·hm-2厩肥+200 kg·hm-2氮肥;N5:15 000 kg·hm-2厩肥+300 kg·hm-2氮肥
Fig. 2Schematic diagram of winter wheat water and nitrogen coupling experiment
W1: 90 mm irrigation amount, W2: 60 mm irrigation amount. N1: No fertilizer; N2: 15 000 kg·hm-2 of farmyard manure; N3: 15 000 kg·hm-2 of farmyard manure and 100 kg·hm-2 of nitrogen fertilizer; N4: 15 000 kg·hm-2 of farmyard manure and 200 kg·hm-2 of nitrogen fertilizer; N5: 15 000 kg·hm-2 of farmyard manure and 300 kg·hm-2 of nitrogen fertilizer
1.2 遥感数据
分别在玉米大喇叭口期和抽雄前期,冬小麦拔节期开展无人机飞行,基于3DR Solo无人机(3DR,加利福尼亚,美国)搭载RedEdge M多光谱传感器(MicaSense,华盛顿,美国)获取相关影像数据。其中,RedEdge M传感器共有5个波段,中心波长分别为蓝光波段(475 nm)、绿光波段(560 nm)、红光波段(668 nm)、近红外波段(840 nm)和红边波段(717 nm)。无人机作业时,其航向和旁向的重叠率均设为75%,并在飞行前拍摄白板以用于后期将影像的DN值转换为反射率。其中,玉米试验的无人机飞行高度为50 m,对应影像空间分辨率约为3.5 cm;小麦试验的无人机飞行高度为30 m,对应影像空间分辨率约为2.0 cm。Pix4D ag软件(Pix4D,洛桑,瑞士)用来进行无人机影像的拼接和反射率计算。另外,基于厘米级误差差分GPS天宝GEO 7X(Trimble,加利福尼亚,美国)采集的高精度地面样点数据对影像做几何精校正。1.3 改进的尺度参数自动确定方法
本研究在WLV方法的基础上,综合考虑对象内同质性和对象间异质性提出改进方法。WLV法借鉴了LV的理论,将对象及其相邻对象作为该对象的邻域对象集;计算各邻域对象集的局部方差,然后将所有对象的邻域对象集局部方差的算术均值作为WLV值。其中,在计算邻域对象集局部方差时,将相邻对象的面积和中心对象与相邻对象的公共边长的乘积作为权重。WLV的计算方法如公式(1)—(3)所示。可以看出,WLV方法着重计算对象间异质性,没有充分考虑对象内同质性。因此,本研究在WLV的基础上增加对象内同质性的计算,以标准差表示同质性,在公式(2)的基础上减去对象内标准差。此外,为了避免过分割和欠分割现象,将1/A(u)作为对象内同质性的权重,将1-1/A(u)作为对象间异质性的权重。本研究将改进后的方法称之为改进加权局部方差方法(improved weighted local variance,IWLV),计算如公式(4)和(5)所示。影像分割过程中,当分割对象与目标的尺寸相近时,对象间异质性最大,同质性最小,此时的IWLV值会大于在其他情况下分割结果的IWLV值,表现为最大。式中,WLV(R)为分割结果R的加权局部方差;#B为波段数;B为波段集合;b为波段集合B中的一个波段(为了消除不同波段的量级差异,对各波段做归一化处理);u为分割结果R中的一个对象;P(u)为对象u的权重,在此设置为1,表示每个对象的IWLVE在计算IWLV时同等重要;WLVE(u, b)为对象u在波段b上的加权局部方差,计算如公式(2)所示。
式中,N(u)为对象u的邻域对象集,包含u本身;v为N(u)中的一个对象;Q(v)为v的权重,由对象v的面积和对象u、v公共边长的乘积计算而来;mb(v)为对象v在波段b上的均值;mb(N(u))为对象u的邻域对象集在波段b上的加权均值,计算见公式(3)。
式中,IWLV(R)为分割结果R的改进加权局部方差;IWLVE(u, b)表示对象u在波段b上的改进加权局部方差,计算见公式(5)。
式中,STDb(u)为对象u在b波段上的内部标准差,A(u)为与对象u相邻的对象个数。
1.4 数据分析方法
为验证IWLV方法,本研究将WLV法与其进行比较。比较时,选择目前的主流影像分割方法之一均值漂移算法作为分割算法,并基于获取的玉米、小麦数据设计不同的比较情景。其中,玉米按2个生育期、5个氮肥处理分为10种分割场景(大喇叭口期不同氮肥处理用NiS1表示,抽雄前期不同氮肥处理用NiS2表示,i表示数字1到5,对应图1所示不同施肥量);小麦按2个水分处理、5个氮肥处理也分为10种分割场景,分别用NiWj表示(i表示数字1到5,j表示数字1到2,分别对应图2所示不同施肥量和灌溉量)。需要说明的是每种情景所涉各重复小区作为一个整体参与分割。数据处理中,分别对不同场景进行分割尺度的选择和分割结果的验证。此外,根据解译经验,综合考虑影像分辨率和目标大小,本研究将尺度参数范围设置为5—90之间,并以5为步长递增,来计算评价函数值。针对每种场景,首先分别计算不同尺度参数下的WLV和IWLV值,得到各场景下WLV和IWLV随尺度变化图;其次,分别以WLV、IWLV最大值所对应的尺度参数值作为影像分割的最优尺度,获取对应分割结果;最后,在情景所涉各小区内分别随机布设1 m×2 m的矩形,在矩形内采用人机交互方式进行分割,用于评价WLV和IWLV的精度。精度评价,采用单尺度对象精度(single-scale object accuracy,SOA)[28]作为评价指标。SOA是计算分割结果和真实结果相似程度的指标,取值范围在0—1之间,具体计算如公式(6)—(7)所示。其中,SOA越小,表明分割结果欠分割或过分割现象越严重;SOA越大,说明分割结果和真实结果越接近。另外,人机交互分割时,以WLV和IWLV方法获得的最优分割尺度参数取平均来分割影像,并采用人工目视解译的方法对分割结果进行修正。
式中,Ru表示参考分割影像中的对象;Pv表示待评价分割影像中的对象;#P表示待评价分割影像中对象的个数。
2 结果
2.1 影像基本情况
不同作物的冠层结构不同,玉米和小麦的结构差异较大,因此通过低空无人机获取的影像也具有较大差异。从图3—4可以看出,本研究获取的小麦影像比玉米影像纹理上显得更为细腻,不同处理小区间颜色对比度也大。这是由于玉米个体较大,在高分辨率无人机影像中个体信息体现得比较明显,影像纹理上也显得更为粗糙;在不同的水、肥处理下,各小区小麦展现出明显的长势差异,整体上随水、肥的增加长势更优。另外,对比图3-b和图3-c,可以看出处于玉米抽雄前期的植株比大喇叭口期的植株更加茂密。总体来说,本研究获取的数据具有较复杂的场景,能为方法间的对比提供很好的支撑。图3
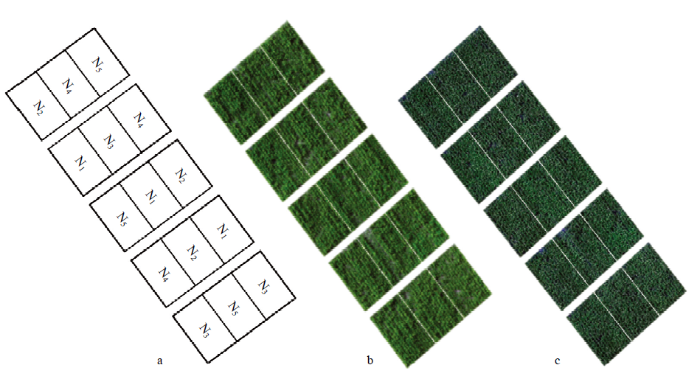
图3玉米试验小区示意图(a)及大喇叭口期(b)、抽雄前期(c)的无人机影像
N1—N5分别表示0、70、140、210、280 kg·hm-2氮肥处理
Fig. 3Schematic diagram of corn experiment (a) and corn drone images of bellmouth growth stage (b) and before heading growth stage (c)
N1-N5 represent applied nitrogen at 0, 70, 140, 210, 280 kg·hm-2
图4
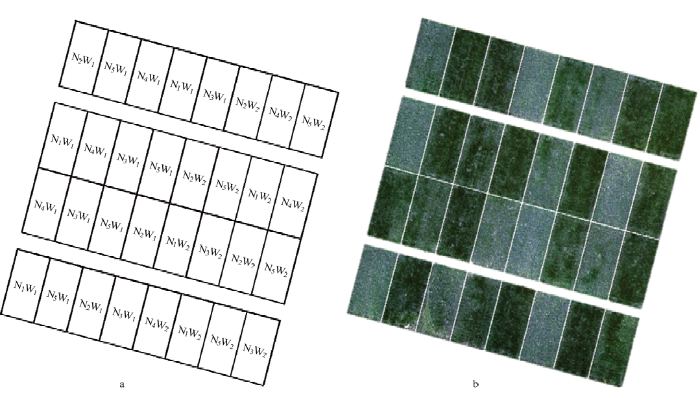
图4冬小麦试验小区示意图(a)及小麦拔节期无人机影像(b)
W1:90 mm灌溉量;W2:60 mm灌溉量;N1:不施肥;N2:15 000 kg·hm-2厩肥;N3:15 000 kg·hm-2厩肥+100 kg·hm-2氮肥;N4:15 000 kg·hm-2厩肥+200 kg·hm-2氮肥;N5:15 000 kg·hm-2厩肥+300 kg·hm-2氮肥
Fig. 4Schematic diagram of wheat experiment (a) and drone image of wheat jointing growth stage (b)
W1: 90 mm irrigation amount, W2: 60 mm irrigation amount. N1: No fertilizer; N2: 15 000 kg·hm-2 of farmyard manure; N3: 15 000 kg·hm-2 of farmyard manure and 100 kg·hm-2 of nitrogen fertilizer; N4: 15 000 kg·hm-2 of farmyard manure and 200 kg·hm-2 of nitrogen fertilizer; N5: 15 000 kg·hm-2 of farmyard manure and 300 kg·hm-2 of nitrogen fertilizer
2.2 玉米影像分割结果
2.2.1 最优尺度参数选择 各种场景下WLV、IWLV随尺度参数值变化情况如图5所示。可以看出,相对于WLV,IWLV随尺度参数增大,先上升后下降的趋势更加明显。这表明增加考虑对象内部同质性后,IWLV法比WLV法对分割尺度变化引起的分割差异更加敏感。另外,各场景基于WLV法和IWLV法选定的最优尺度参数值如表1所示。从表中可以看出,各分割场景基于IWLV所选的尺度参数值都比WLV法所选的尺度参数值小,说明IWLV法相对于WLV法倾向把影像分割得更细。此外,对比不同生育期的分割结果,可以发现玉米抽雄前期各场景下的最佳尺度参数值普遍比大喇叭口期的相应尺度参数值小。这主要是因为随着植株生长,叶片相互交织,原本大块的裸露土壤由于被覆盖形成零碎的裸露土壤斑块,识别这些小土壤斑块需要更小的尺度参数。对比各生育期不同处理的分割结果,各处理最优分割尺度值未体现出规律性变化。图5
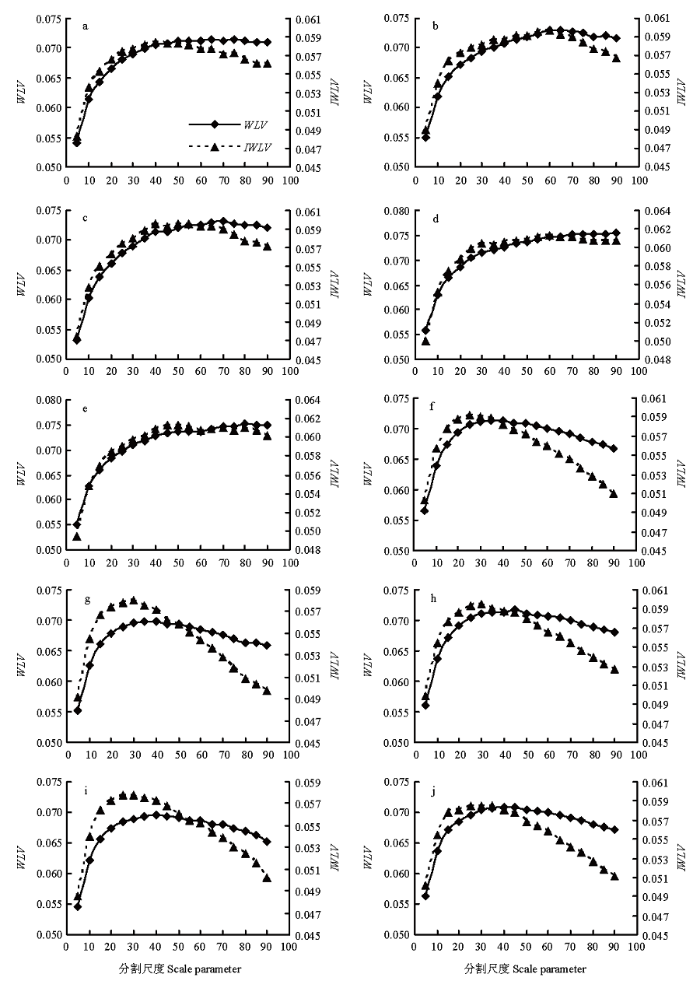
图5基于玉米影像不同分割尺度下WLV和IWLV变化曲线
图a—e分别对应N1S1—N5S1,图f—j分别对应N1S2—N5S2
Fig. 5Curves of WLV and IWLV under different scale parameters using corn images
Figure a-e denotes N1S1-N5S1 respectively, and figure f-j denotes N1S2-N5S2 respectively
Table 1
表1
表1基于玉米影像WLV法和IWLV法在各情景选择的最优尺度值
Table 1
方法 Method | N1S1 | N2S1 | N3S1 | N4S1 | N5S1 | N1S2 | N2S2 | N3S2 | N4S2 | N5S2 |
---|---|---|---|---|---|---|---|---|---|---|
WLV | 65 | 65 | 70 | 90 | 80 | 35 | 40 | 45 | 40 | 45 |
IWLV | 40 | 60 | 40 | 60 | 45 | 25 | 30 | 30 | 30 | 30 |
新窗口打开|下载CSV
2.2.2 分割精度评价 如前所述,在各分割场景下所涉每个小区中随机布设一个1 m×2 m的矩形,在矩形中采用人机交互方式进行分割,将结果用以评价WLV法和IWLV法在其选择的尺度下分割结果的精度。图6展示了各矩形分布情况,各场景下计算的WLV法和IWLV法的SOA值如表2所示。可以看出,各情景下基于IWLV法的SOA值要比WLV法高。另外,图7展示部分影像人机交互分割结果和分别基于WLV法和IWLV法选择尺度下的分割结果。其中,展示样区位置如图6所示。从图中可以看出,IWLV所选尺度对应的分割结果与参考分割结果更接近,而WLV所选尺度对应的分割结果则存在较为明显的欠分割。以上结果说明,基于玉米各场景影像,IWLV方法分割的结果精度更高。
图6
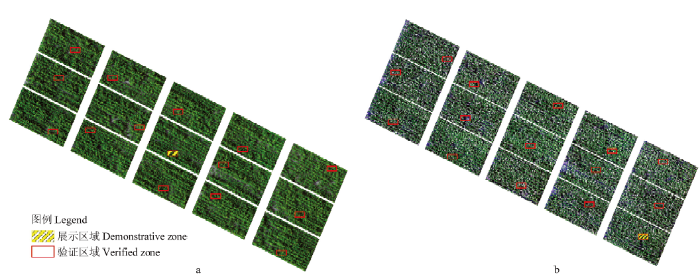
图6基于玉米影像的验证区域分布图
a:大喇叭口期;b抽雄前期 a: Bellmouth growth stage; b: Before heading growth stage
Fig. 6The distribution of verified zone using corn images
Table 2
表2
表2基于玉米影像WLV法和IWLV法在各情景选择尺度下分割结果的SOA值
Table 2
方法 Method | N1S1 | N2S1 | N3S1 | N4S1 | N5S1 | N1S2 | N2S2 | N3S2 | N4S2 | N5S2 |
---|---|---|---|---|---|---|---|---|---|---|
WLV | 0.626 | 0.693 | 0.675 | 0.654 | 0.722 | 0.747 | 0.755 | 0.682 | 0.726 | 0.711 |
IWLV | 0.770 | 0.765 | 0.767 | 0.770 | 0.795 | 0.768 | 0.811 | 0.796 | 0.807 | 0.771 |
新窗口打开|下载CSV
图7
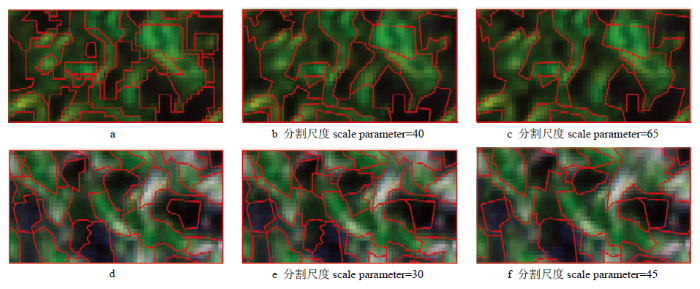
图7基于玉米影像的分割结果
人机交互分割结果(a:N1S1场景;d:N3S2场景);基于IWLV法选择尺度分割结果(b:N1S1场景;e:N3S2场景);基于WLV法选择尺度分割结果(c:N1S1场景;f:N3S2场景)
Fig. 7Segmentation results using corn image
Segmentation result by human-machine interactive method (a: N1S1 scenario; d: N3S2 scenario); segmentation result of selected optimal scale by the IWLV method (b: N1S1 scenario; e: N3S2 scenario); segmentation result of selected optimal scale by the WLV method (c: N1S1 scenario; f: N3S2 scenario)
2.3 冬小麦影像分割结果
2.3.1 最优尺度参数选择 各种场景下WLV、IWLV随尺度参数值变化情况如图8所示。与玉米结果类似,在各分割场景中,随着尺度参数增大,IWLV虽有波动但先上升后下降的趋势比WLV方法更加明显。另外,在部分场景中,随尺度参数增大,WLV呈先上升后平稳甚至上升的趋势,导致所选尺度参数值偏大。以上结果表明,相对于WLV法,本研究提出的IWLV法在小麦不同场景下均能取得较稳健的结果。基于WLV法和IWLV法选定的各场景尺度值如表3所示。从表中可以看出,2种方法除N1W1所选的尺度参数相同外,在其他各分割场景中,基于IWLV所选的尺度都比WLV所选的尺度小,同样说明IWLV相对于WLV倾向把影像分割得更细。此外,可以发现随着施氮量的增加,选定的最优分割尺度整体呈增大趋势。其主要原因是施氮量小时,小麦群体长势较弱,相对于施氮量大时,小麦群体长势旺盛的影像需要设置相对小的尺度来识别。不同水分处理,小麦长势差异不显著,因此,选定的最优分割尺度没有趋势性变化。图8
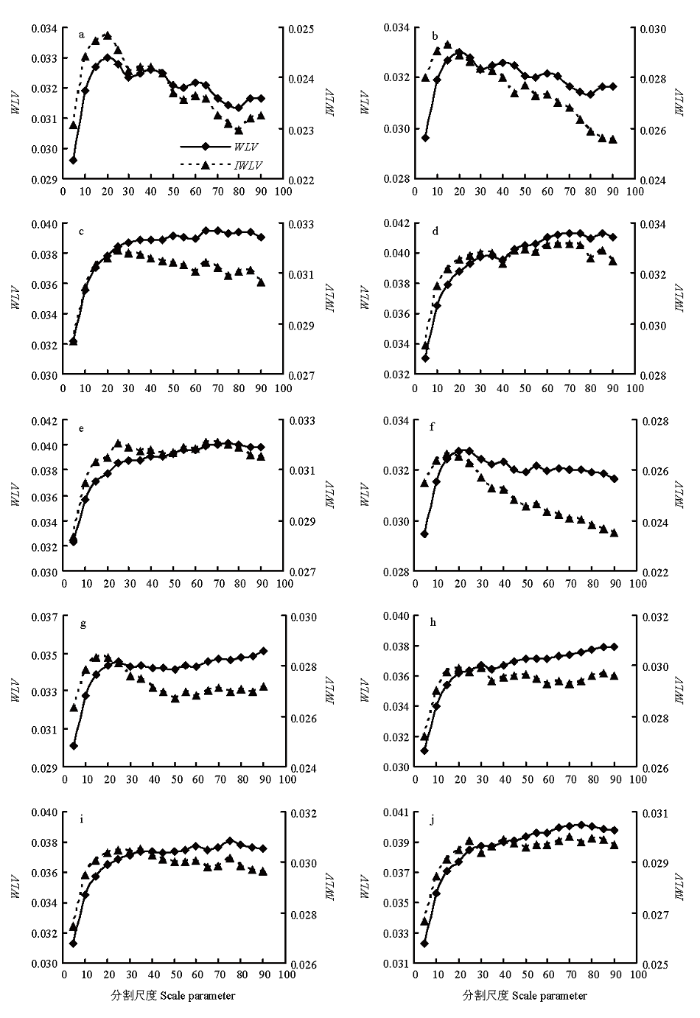
图8基于小麦影像不同分割尺度参数下WLV和IWLV变化曲线
图a—e分别对应N1W1—N5W1,图f—j分别对应N1W2—N5W2 Figure a-e denotes N1W1-N5W1 respectively, and figure f-j denotes N1W2-N5W2 respectively
Fig. 8Curves of WLV and IWLV under different scale parameters using wheat images
Table 3
表3
表3基于小麦影像WLV和IWLV法所选的各情景下最优尺度值
Table 3
方法 Method | N1W1 | N2W1 | N3W1 | N4W1 | N5W1 | N1W2 | N2W2 | N3W2 | N4W2 | N5W2 |
---|---|---|---|---|---|---|---|---|---|---|
WLV | 20 | 35 | 65 | 85 | 75 | 20 | 90 | 85 | 75 | 75 |
IWLV | 20 | 15 | 25 | 70 | 65 | 15 | 15 | 20 | 35 | 70 |
新窗口打开|下载CSV
2.3.2 分割精度评价 基于人机交互结果对WLV法和IWLV法选定尺度下的分割结果进行评价,结果如表4所示。图9展示了所选矩形样区的空间分布情况。从表4可以看出,除在N1W1情景下,2种方法取得相同的SOA值外,其他各情景下IWLV方法的SOA值比WLV方法的SOA值高。另外,图10展示了部分影像人机交互分割结果和分别基于WLV法和IWLV法选择尺度下的分割结果。其中,展示样区位置如图9所示。从图中可以看出,IWLV所选尺度对应分割结果与人机交互分割结果更为接近,而WLV所选尺度对应的分割结果存在较为明显的欠分割现象。以上结果说明,基于小麦各场景影像,IWLV方法分割的结果精度更高。
图9
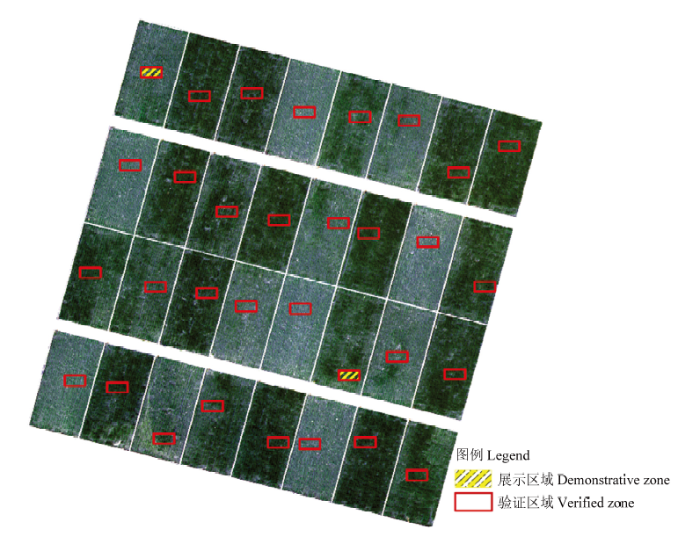
图9小麦影像验证区域分布图
Fig. 9The distribution of verified zone in wheat image
Table 4
表4
表4基于小麦影像WLV法和IWLV法在各情景选择尺度下分割结果的SOA值
Table 4
方法 Method | N1W1 | N2W1 | N3W1 | N4W1 | N5W1 | N1W2 | N2W2 | N3W2 | N4W2 | N5W2 |
---|---|---|---|---|---|---|---|---|---|---|
WLV | 0.675 | 0.535 | 0.548 | 0.553 | 0.661 | 0.618 | 0.430 | 0.446 | 0.536 | 0.646 |
IWLV | 0.675 | 0.660 | 0.717 | 0.602 | 0.705 | 0.667 | 0.716 | 0.708 | 0.685 | 0.648 |
新窗口打开|下载CSV
图10
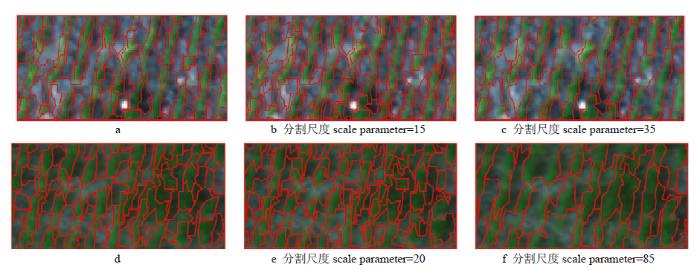
图10基于小麦影像的分割结果
人机交互分割结果(a:N2W1场景;d:N3W2场景);基于IWLV法选择尺度分割结果(b:N2W1场景;e:N3W2场景);基于WLV法选择尺度分割结果(c:N2W1场景;f:N3W2场景)
Fig. 10Segmentation results using wheat image
Segmentation result by human-machine interactive method (a: N2W1 scenario;d: N3W2 scenario); segmentation result of selected optimal scale by the IWLV method (b: N2W1 scenario; e: N3W2 scenario); segmentation result of selected optimal scale by the WLV method (c: N2W1 scenario; f: N3W2 scenario)
3 讨论
相比于WLV方法,本研究提出的IWLV方法既考虑了对象内部同质性,又考虑了对象间异质性,并用相邻对象个数作为权重参数,对同质性和间异质性赋予不同的权重,最终表明IWLV方法能较好解决WLV方法欠分割的问题,在各分割场景下都取得了更好的分割效果。此外,在基于WLV和IWLV等方法进行影像自动分割时,若分割尺度参数范围设置过大,则随着尺度的增大,分割对象从包含部分目标地物到包含1个、2个或者更多目标地物的过程中,计算的WLV、IWLV值会有波动现象。因此,自动分割过程中,设定合适的尺度范围非常重要[29],先验知识是确定最优尺度参数值的重要辅助因素。分割尺度与对象大小密切相关。同一分辨率影像下,对象越小其最优分割尺度越小。如玉米影像中,由于玉米在大喇叭口期、抽雄前期冠层基本覆盖垄间空隙,叶片与土壤交织形成的土壤斑块大小成为限定影像分割尺度的主要因素,所以随着玉米生长,抽雄前期的土壤斑块要比大喇叭口期的土壤斑块更为细碎,其需要的分割尺度也小于大喇叭口期的分割尺度;小麦影像中,由于拔节期小麦未封垄,垄间存在大片裸露地表,小麦冠层成为限定影像分割尺度的主要因素,所以长势越好,小麦整体冠层越大,其分割尺度越大。
此外,相对分辨率(影像分辨率与目标地物大小的比值)与分割精度密切相关。对比不同作物,可以看出玉米的分割精度整体高于冬小麦的分割精度。这是因为虽然小麦影像的分辨率(2.0 cm)高于玉米影像的分辨率(3.5 cm),但是小麦叶片长度约3—10 cm、宽度1 cm左右,玉米叶片长度在40—80 cm、宽度5 cm左右,分别对应2.0 cm和3.5 cm分辨率影像来说,小麦影像的相对分辨率要低于玉米影像的相对分辨率。所以,小麦分割结果的精度值(SOA值)要小于玉米分割结果。
本研究只是基于小麦、玉米数据对WLV法和IWLV方法进行对比评价,今后要进一步验证它们在其他作物上的应用效果。
4 结论
通过引入对象间同质性的计算,本研究对WLV法进行改进,提出IWLV法以支撑农业无人机影像的自动分割。利用玉米和小麦不同生育期、不同长势条件下无人机影像,通过设计不同影像场景,对WLV法和IWLV法的精度进行评价,结果表明,WLV法经改进为IWLV法后,可有效抑制其欠分割现象。所有场景下,与人机交互方法获得的分割结果相比,基于IWLV法自动选择分割尺度的分割结果比基于WLV法自动选择分割尺度的分割结果获得了更高的SOA值,取得更好的分割效果。因此,改进方法提高了已有方法的精度,可以有效支撑农业无人机影像的自动分割,为精准农业管理提供技术支撑。致谢:
感谢徐志涛、刘金然、贾丹和曹庆军在田间试验过程中给予的支持和帮助;感谢李静老师为本研究工作的开展提供小麦试验基地。参考文献 原文顺序
文献年度倒序
文中引用次数倒序
被引期刊影响因子
[本文引用: 1]
[本文引用: 1]
[本文引用: 1]
DOI:10.1016/0034-4257(94)90134-1URL [本文引用: 1]
DOI:10.1016/0034-4257(95)00186-7URL [本文引用: 1]
[本文引用: 1]
[本文引用: 1]
DOI:10.3864/j.issn.0578-1752.2019.13.003URL [本文引用: 2]

【Objective】 Based on the high spatial resolution images of unmanned aerial vehicle (UAV), the effects of removing soil background information and increasing image texture information on the inversion of cotton plant nitrogen concentration were investigated, in order to provide new technology for accurate estimation of cotton nitrogen nutrition status. 【Method】 Cotton water and nitrogen coupling experiment was conducted, and UAV images and plant nitrogen concentration data were measured during different cotton growth stages. Based on the above data, the effect of soil background on cotton canopy spectrum was firstly investigated. Secondly, the correlations between image texture parameters and plant nitrogen concentration were analyzed. Finally, the obtained data was divided into calibration dataset and validation dataset. Different scenarios, including before and after removing the soil background, and adding texture features, were set. The inversion models of plant nitrogen concentration under various scenarios were designed by using the coupled method of spectral indexes and principal component regression, and the performances of the models were compared. 【Result】 The soil background had an effect on the cotton canopy spectrum, and the trends were not the same at different growth stages. There existed significant correlations between image texture parameters and plant nitrogen concentration. For the scenarios before removal soil background, the plant nitrogen concentration prediction model had determination coefficient (R 2) value of 0.33 and root mean square error (RMSE) value of 0.21% during model calibration, and R 2 value of 0.19 and RMSE value of 0.23% during validation. For the scenarios after removing soil background, the plant nitrogen concentration prediction model had R 2 value of 0.38 and RMSE value of 0.20% during model calibration, and R 2 value of 0.30 and RMSE value of 0.21% during validation. For the scenarios adding image texture information, the plant nitrogen concentration prediction model had R 2 value of 0.57 and RMSE value of 0.17% during model calibration, and R 2 value of 0.42 and RMSE value of 0.19% during validation. 【Conclusion】 Based on high spatial resolution images of low-altitude UAVs, both removing soil background and adding image texture information could improve the inversion accuracy of cotton plant nitrogen concentration. Image texture could be considered as important information to support prediction of crop nitrogen nutrition status using UAV images.
DOI:10.3864/j.issn.0578-1752.2019.13.003URL [本文引用: 2]

【Objective】 Based on the high spatial resolution images of unmanned aerial vehicle (UAV), the effects of removing soil background information and increasing image texture information on the inversion of cotton plant nitrogen concentration were investigated, in order to provide new technology for accurate estimation of cotton nitrogen nutrition status. 【Method】 Cotton water and nitrogen coupling experiment was conducted, and UAV images and plant nitrogen concentration data were measured during different cotton growth stages. Based on the above data, the effect of soil background on cotton canopy spectrum was firstly investigated. Secondly, the correlations between image texture parameters and plant nitrogen concentration were analyzed. Finally, the obtained data was divided into calibration dataset and validation dataset. Different scenarios, including before and after removing the soil background, and adding texture features, were set. The inversion models of plant nitrogen concentration under various scenarios were designed by using the coupled method of spectral indexes and principal component regression, and the performances of the models were compared. 【Result】 The soil background had an effect on the cotton canopy spectrum, and the trends were not the same at different growth stages. There existed significant correlations between image texture parameters and plant nitrogen concentration. For the scenarios before removal soil background, the plant nitrogen concentration prediction model had determination coefficient (R 2) value of 0.33 and root mean square error (RMSE) value of 0.21% during model calibration, and R 2 value of 0.19 and RMSE value of 0.23% during validation. For the scenarios after removing soil background, the plant nitrogen concentration prediction model had R 2 value of 0.38 and RMSE value of 0.20% during model calibration, and R 2 value of 0.30 and RMSE value of 0.21% during validation. For the scenarios adding image texture information, the plant nitrogen concentration prediction model had R 2 value of 0.57 and RMSE value of 0.17% during model calibration, and R 2 value of 0.42 and RMSE value of 0.19% during validation. 【Conclusion】 Based on high spatial resolution images of low-altitude UAVs, both removing soil background and adding image texture information could improve the inversion accuracy of cotton plant nitrogen concentration. Image texture could be considered as important information to support prediction of crop nitrogen nutrition status using UAV images.
URL [本文引用: 1]

准确、快速地获取关键生育期冬小麦氮素含量,对农业管理者进行田间氮素施肥有重要的决策作用。利用无人机(unmanned aerial vehicle,UAV)搭载数码相机,可以短时间内获取冬小麦长势信息,实现对冬小麦氮素含量动态监测。该研究利用2015年北京市小汤山冬小麦无人机数码影像,采用3种阈值分割方法,将田间植株作物与土壤背景分离。对比影像分割方法的时效性与准确性,最终确定可见光波段差异植被指数VDVI(visible-band difference vegetation index)提取植被信息。按照试验方案要求,在不同的氮肥与水分胁迫管理下,将冬小麦3次重复试验分成48个试验小区,依据小区边界提取小区的红、绿和蓝通道的平均DN(digital number)值,选取25个植被指数,同时与各个试验小区冬小麦不同器官氮含量进行相关性分析,筛选数码影像变量。由于植被指数之间耦合度较高,因此采用主成分分析对原始数据进行成分提取,提取特征向量参与建模,最后利用多元线性回归分析建立氮素反演模型,通过决定系数(R2)、均方根误差(RMSE)和归一化的均方根误差(nRMSE)3个指标筛选出最佳模型,探究各器官氮素含量与数码变量的相关性。结果表明,实验室实测氮素含量与UAV数码影像氮素反演结果及基本一致。在反演模型构建精度方面,3种数据处理结果整体>部分>植被指数,反演效果叶氮>植株氮>茎氮。以冬小麦挑旗期为例,叶片氮含量整体信息提取验证模型的R2、RMSE和nRMSE分别为0.85、0.235和6.10%,比部分信息提取验证模型的R2高0.14,RMSE和nRMSE分别降低0.068和1.77个百分点;比植被指数信息提取验证模型的R2高0.43,RMSE和nRMSE分别降低0.141和3.67个百分点。研究表明,基于UAV数码影像利用多元线性回归构建冬小麦氮素含量反演模型,对试验小区整体提取作物信息的方式反演冬小麦叶氮含量效果最好,相比传统反演方法,模型稳定性更高,可为冬小麦田间水肥决策管理提供参考。
URL [本文引用: 1]

准确、快速地获取关键生育期冬小麦氮素含量,对农业管理者进行田间氮素施肥有重要的决策作用。利用无人机(unmanned aerial vehicle,UAV)搭载数码相机,可以短时间内获取冬小麦长势信息,实现对冬小麦氮素含量动态监测。该研究利用2015年北京市小汤山冬小麦无人机数码影像,采用3种阈值分割方法,将田间植株作物与土壤背景分离。对比影像分割方法的时效性与准确性,最终确定可见光波段差异植被指数VDVI(visible-band difference vegetation index)提取植被信息。按照试验方案要求,在不同的氮肥与水分胁迫管理下,将冬小麦3次重复试验分成48个试验小区,依据小区边界提取小区的红、绿和蓝通道的平均DN(digital number)值,选取25个植被指数,同时与各个试验小区冬小麦不同器官氮含量进行相关性分析,筛选数码影像变量。由于植被指数之间耦合度较高,因此采用主成分分析对原始数据进行成分提取,提取特征向量参与建模,最后利用多元线性回归分析建立氮素反演模型,通过决定系数(R2)、均方根误差(RMSE)和归一化的均方根误差(nRMSE)3个指标筛选出最佳模型,探究各器官氮素含量与数码变量的相关性。结果表明,实验室实测氮素含量与UAV数码影像氮素反演结果及基本一致。在反演模型构建精度方面,3种数据处理结果整体>部分>植被指数,反演效果叶氮>植株氮>茎氮。以冬小麦挑旗期为例,叶片氮含量整体信息提取验证模型的R2、RMSE和nRMSE分别为0.85、0.235和6.10%,比部分信息提取验证模型的R2高0.14,RMSE和nRMSE分别降低0.068和1.77个百分点;比植被指数信息提取验证模型的R2高0.43,RMSE和nRMSE分别降低0.141和3.67个百分点。研究表明,基于UAV数码影像利用多元线性回归构建冬小麦氮素含量反演模型,对试验小区整体提取作物信息的方式反演冬小麦叶氮含量效果最好,相比传统反演方法,模型稳定性更高,可为冬小麦田间水肥决策管理提供参考。
DOI:10.1016/j.isprsjprs.2013.09.014URLPMID:24623958 [本文引用: 1]

The amount of scientific literature on (Geographic) Object-based Image Analysis - GEOBIA has been and still is sharply increasing. These approaches to analysing imagery have antecedents in earlier research on image segmentation and use GIS-like spatial analysis within classification and feature extraction approaches. This article investigates these development and its implications and asks whether or not this is a new paradigm in remote sensing and Geographic Information Science (GIScience). We first discuss several limitations of prevailing per-pixel methods when applied to high resolution images. Then we explore the paradigm concept developed by Kuhn (1962) and discuss whether GEOBIA can be regarded as a paradigm according to this definition. We crystallize core concepts of GEOBIA, including the role of objects, of ontologies and the multiplicity of scales and we discuss how these conceptual developments support important methods in remote sensing such as change detection and accuracy assessment. The ramifications of the different theoretical foundations between the 'per-pixel paradigm' and GEOBIA are analysed, as are some of the challenges along this path from pixels, to objects, to geo-intelligence. Based on several paradigm indications as defined by Kuhn and based on an analysis of peer-reviewed scientific literature we conclude that GEOBIA is a new and evolving paradigm.
DOI:10.1007/s10661-012-3022-1URLPMID:23255169 [本文引用: 1]

Discrete trees and small groups of trees in nonforest settings are considered an essential resource around the world and are collectively referred to as trees outside forests (ToF). ToF provide important functions across the landscape, such as protecting soil and water resources, providing wildlife habitat, and improving farmstead energy efficiency and aesthetics. Despite the significance of ToF, forest and other natural resource inventory programs and geospatial land cover datasets that are available at a national scale do not include comprehensive information regarding ToF in the United States. Additional ground-based data collection and acquisition of specialized imagery to inventory these resources are expensive alternatives. As a potential solution, we identified two remote sensing-based approaches that use free high-resolution aerial imagery from the National Agriculture Imagery Program (NAIP) to map all tree cover in an agriculturally dominant landscape. We compared the results obtained using an unsupervised per-pixel classifier (independent component analysis-[ICA]) and an object-based image analysis (OBIA) procedure in Steele County, Minnesota, USA. Three types of accuracy assessments were used to evaluate how each method performed in terms of: (1) producing a county-level estimate of total tree-covered area, (2) correctly locating tree cover on the ground, and (3) how tree cover patch metrics computed from the classified outputs compared to those delineated by a human photo interpreter. Both approaches were found to be viable for mapping tree cover over a broad spatial extent and could serve to supplement ground-based inventory data. The ICA approach produced an estimate of total tree cover more similar to the photo-interpreted result, but the output from the OBIA method was more realistic in terms of describing the actual observed spatial pattern of tree cover.
DOI:10.1016/j.compag.2015.03.019URL [本文引用: 1]
[本文引用: 1]
[本文引用: 1]
DOI:10.1016/j.rse.2006.01.013URL [本文引用: 1]
DOI:10.1109/LGRS.2015.2421736URL [本文引用: 1]
DOI:10.3390/ijgi4042292URL [本文引用: 1]
DOI:10.3724/SP.J.1047.2014.00902URL [本文引用: 1]

随着卫星遥感影像空间分辨率的不断提高,面向对象的地物信息提取技术发展迅速。图像分割作为面向对象分类的关键步骤之一,其分割尺度的参数设置目前仍以分类者的多次尝试和主观判断为依据,效率较低且分割结果因人而异。本文以WorldView2影像数据为例,结合当前现有的理论和方法,实现了一种计算机可自动进行主成分变换的高分辨率遥感图像全局最优分割尺度选取算法。改进后的算法以主成分变换所得的主成分影像作为图像分割的编辑层,主成分的特征值百分比作为计算异质性参数和分割质量评价值的权重,自动计算当分割尺度从20增至200时分割图像的分割质量评价值(GS),解决了人为确定图像分割编辑层的片面性问题,并利用三次样条插值选取出GS最高值所对应的尺度即为最优分割尺度。结果表明,该最优分割尺度选取方法可有效避免人为确定分割尺度的主观性、片面性和低效性,提升了高分辨率影像分割质量。
DOI:10.3724/SP.J.1047.2014.00902URL [本文引用: 1]

随着卫星遥感影像空间分辨率的不断提高,面向对象的地物信息提取技术发展迅速。图像分割作为面向对象分类的关键步骤之一,其分割尺度的参数设置目前仍以分类者的多次尝试和主观判断为依据,效率较低且分割结果因人而异。本文以WorldView2影像数据为例,结合当前现有的理论和方法,实现了一种计算机可自动进行主成分变换的高分辨率遥感图像全局最优分割尺度选取算法。改进后的算法以主成分变换所得的主成分影像作为图像分割的编辑层,主成分的特征值百分比作为计算异质性参数和分割质量评价值的权重,自动计算当分割尺度从20增至200时分割图像的分割质量评价值(GS),解决了人为确定图像分割编辑层的片面性问题,并利用三次样条插值选取出GS最高值所对应的尺度即为最优分割尺度。结果表明,该最优分割尺度选取方法可有效避免人为确定分割尺度的主观性、片面性和低效性,提升了高分辨率影像分割质量。
[本文引用: 1]
DOI:10.1080/13658810903174803URL [本文引用: 1]
DOI:10.1016/j.isprsjprs.2013.11.018URL [本文引用: 1]

We introduce a new automated approach to parameterising multi-scale image segmentation of multiple layers, and we implemented it as a generic tool for the eCognition (R) software. This approach relies on the potential of the local variance (LV) to detect scale transitions in geospatial data. The tool detects the number of layers added to a project and segments them iteratively with a multiresolution segmentation algorithm in a bottom-up approach, where the scale factor in the segmentation, namely, the scale parameter (SP), increases with a constant increment. The average LV value of the objects in all of the layers is computed and serves as a condition for stopping the iterations: when a scale level records an LV value that is equal to or lower than the previous value, the iteration ends, and the objects segmented in the previous level are retained. Three orders of magnitude of SP lags produce a corresponding number of scale levels. Tests on very high resolution imagery provided satisfactory results for generic applicability. The tool has a significant potential for enabling objectivity and automation of GEOBIA analysis. (C) 2013 International Society for Photogrammetry and Remote Sensing, Inc. (ISPRS) Published by Elsevier B.V.
DOI:10.1016/j.isprsjprs.2011.02.006URL [本文引用: 1]

In this study, a multi-scale approach is used to improve the segmentation of a high spatial resolution (30 cm) color infrared image of a residential area. First, a series of 25 image segmentations are performed in Definiens Professional 5 using different scale parameters. The optimal image segmentation is identified using an unsupervised evaluation method of segmentation quality that takes into account global intra-segment and inter-segment heterogeneity measures (weighted variance and Moran's I, respectively). Once the optimal segmentation is determined, under-segmented and over-segmented regions in this segmentation are identified using local heterogeneity measures (variance and Local Moran's I). The under- and over-segmented regions are refined by (1) further segmenting under-segmented regions at finer scales, and (2) merging over-segmented regions with spectrally similar neighbors. This process leads to the creation of several segmentations consisting of segments generated at three different segmentation scales. Comparison of single- and multi-scale segmentations shows that identifying and refining underand over-segmented regions using local statistics can improve global segmentation results. Published by Elsevier B.V. on behalf of International Society for Photogrammetry and Remote Sensing, Inc. (ISPRS).
DOI:10.1080/01431160600617194URL [本文引用: 1]
DOI:10.3390/rs10020222URL [本文引用: 1]
URL [本文引用: 1]

Nowadays, the internet has been gaining social influence over China and internet media is becoming a mainstream form of publication in China. The China Earthquake Administration Website is the official web portal of the China Earthquake Administration and it is a key information platform of disaster protection andreduction during earthquake emergencies. At the same time, it is an authoritative channel and source of earthquakerelated information for interested organizations, mass media and the general public. Hence, with the development of the internet, the roles of the China Earthquake Administration Website, in regard to guiding public opinions, policy propagation, communications, earthquake relief incentives, online interaction, popular science of earthquake, assisting earthquake relief, etc. all are studied.
URL [本文引用: 1]

Nowadays, the internet has been gaining social influence over China and internet media is becoming a mainstream form of publication in China. The China Earthquake Administration Website is the official web portal of the China Earthquake Administration and it is a key information platform of disaster protection andreduction during earthquake emergencies. At the same time, it is an authoritative channel and source of earthquakerelated information for interested organizations, mass media and the general public. Hence, with the development of the internet, the roles of the China Earthquake Administration Website, in regard to guiding public opinions, policy propagation, communications, earthquake relief incentives, online interaction, popular science of earthquake, assisting earthquake relief, etc. all are studied.
DOI:10.1016/j.isprsjprs.2014.04.008URL [本文引用: 1]

Image segmentation is one of key steps in object based image analysis of very high resolution images. Selecting the appropriate scale parameter becomes a particularly important task in image segmentation. In this study, an unsupervised multi-band approach is proposed for scale parameter selection in the multi-scale image segmentation process, which uses spectral angle to measure the spectral homogeneity of segments. With the increasing scale parameter, spectral homogeneity of segments decreases until they match the objects in the real world. The index of spectral homogeneity is thus used to determine multiple appropriate scale parameters. The performance of the proposed method is compared to a single-band based method through qualitative visual interpretation and quantitative discrepancy measures. Both methods are applied for segmenting two images: a QuickBird scene of an urban area within Beijing, China and a Woldview-2 scene of a suburban area in Kashiwa, Japan. The proposed multi-band based segmentation scale parameter selection method outperforms the single-band based method with the better recognition for diverse land cover objects in different urban landscapes. (C) 2014 International Society for Photogrammetry and Remote Sensing, Inc. (ISPRS) Published by Elsevier B.V.
DOI:10.1109/LGRS.2015.2393255URL [本文引用: 1]
[本文引用: 1]
[本文引用: 1]
DOI:10.1016/0034-4257(87)90015-0URL [本文引用: 1]
DOI:10.3724/SP.J.1047.2016.00639URL [本文引用: 1]

面向对象解译技术在高分辨率遥感影像信息提取中得到广泛应用,但影像分割的基础问题仍严重制约其自动化水平,尤其是分割参数选择。因此,本文以广泛使用的分型网络演化分割算法为例,开展尺度参数选择研究。借鉴对遥感影像分辨率敏感的局部方差指标,引入边长和面积权重,构造加权局部方差(WLV)指标,对多个分割结果进行评价,进而实现最佳尺度参数选择。在珠江区域2.5 m的SPOT 5融合影像上进行实验,通过计算最佳分割结果与人工分割结果的相似度对WLV进行定量验证。此外,还对WLV在分割对象最小为一个像元、最大为整景影像的全范围尺度参数的变化规律进行了实验,结果表明:在WLV随尺度参数的变化曲线中,不同极大值点的分割结果反映了实验区不同景观层级上的斑块,其中第1个极大值点对应的分割结果能够较好地反映影像的最小可识别单元。
DOI:10.3724/SP.J.1047.2016.00639URL [本文引用: 1]

面向对象解译技术在高分辨率遥感影像信息提取中得到广泛应用,但影像分割的基础问题仍严重制约其自动化水平,尤其是分割参数选择。因此,本文以广泛使用的分型网络演化分割算法为例,开展尺度参数选择研究。借鉴对遥感影像分辨率敏感的局部方差指标,引入边长和面积权重,构造加权局部方差(WLV)指标,对多个分割结果进行评价,进而实现最佳尺度参数选择。在珠江区域2.5 m的SPOT 5融合影像上进行实验,通过计算最佳分割结果与人工分割结果的相似度对WLV进行定量验证。此外,还对WLV在分割对象最小为一个像元、最大为整景影像的全范围尺度参数的变化规律进行了实验,结果表明:在WLV随尺度参数的变化曲线中,不同极大值点的分割结果反映了实验区不同景观层级上的斑块,其中第1个极大值点对应的分割结果能够较好地反映影像的最小可识别单元。
DOI:10.1109/TGRS.2014.2381632URL [本文引用: 1]
DOI:10.3390/rs9080769URL [本文引用: 1]