


Comparative analysis of the genomic sequences between commercial wheat varieties Jimai 22 and Liangxing 99
YANG Zheng-Zhao


通讯作者:
收稿日期:2020-01-15接受日期:2020-06-2网络出版日期:2020-08-10
基金资助: |
Received:2020-01-15Accepted:2020-06-2Online:2020-08-10
Fund supported: |

摘要
关键词:
Abstract
Keywords:
PDF (2121KB)元数据多维度评价相关文章导出EndNote|Ris|Bibtex收藏本文
本文引用格式
杨正钊, 王梓豪, 胡兆荣, 辛明明, 姚颖垠, 彭惠茹, 尤明山, 宿振起, 郭伟龙. 小麦主栽品种济麦22与良星99的基因组序列多态性比较分析[J]. 作物学报, 2020, 46(12): 1870-1883. doi:10.3724/SP.J.1006.2020.01009
YANG Zheng-Zhao, WANG Zi-Hao, HU Zhao-Rong, XIN Ming-Ming, YAO Ying-Yin, PENG Hui-Ru, YOU Ming-Shan, SU Zhen-Qi, GUO Wei-Long.
小麦是重要的口粮作物。我国是世界上最大的小麦生产和消费国, 提高单产是保障我国小麦生产可持续发展和粮食安全的重要途径。新中国成立以来, 我国小麦主产区经历了5~6次的品种更换, 小麦品种的产量、抗性、品质等重要性状得到了大幅提高。小麦品种的遗传改良对我国小麦单产和总产的提高发挥了重要作用[1]。不同小麦生态区中对照品种既是各时代育种水平的代表, 通常又是下一代品种改良的骨干亲本[2]。例如, 黄淮冬麦区的对照品种周麦18[3]、鲁麦14[4]、石4185[5]等在小麦的遗传改良中做出重要贡献。
常规育种是我国小麦遗传改良的主要手段, 但在亲本选配、后代选择等关键育种过程中依赖于有限的表型性状和育种者的经验。育种过程中存在不确定因素和盲目性, 影响了小麦遗传改良的效率。分子标记的开发和应用为小麦性状的基因定位、标记辅助选择、亲本遗传多样性分析等方面提供了重要支撑, 可有效提升抗性、品质等性状遗传的改良效率。继水稻、玉米、大麦等主要作物基因组测序的完成, 六倍体普通小麦中国春的高质量基因组参考基因组序列(IWGSCv1)的测序完成, 标志着小麦遗传研究已进入“后基因组学时代”[6]。与基于表型和有限分子标记辅助的选择相比, 利用基因组测序并从全基因组水平解析品种间的遗传差异为小麦的遗传研究和品种的改良提供高维度的组学数据支持[7]。
济麦22是山东省农业科学院作物研究所选育[8]。良星99是山东省德州市良星种子研究所选育[9]。济麦22和良星99分别于2006年通过黄淮冬麦区北片审定, 此后相继通过北部冬麦区和黄淮南片等麦区审定, 累计推广面积超过6000万公顷。由于综合性状突出, 济麦22 (2015至今)和良星99 (2010—2015)先后作为黄淮冬麦区北片区域试验的对照品种, 也是我国当前小麦品种改良的重要亲本资源。育种家在长期育种和生产实践中发现, 虽然济麦22和良星99来源及系谱不同, 但两品种间除株高外, 田间总体形态相近; 主要农艺、产量和抗病反应等性状表现一致, 两者的杂交后代亦无明显分离, 普遍认为两个品种遗传组成相似性较高, 亲缘关系较近。近期研究发现麦22和良星99的2个衍生材料的白粉病抗性相近, 通过分子标记定位发现两者具有相同Pm52基因[10]。但对其遗传相似性的认识仍以有限的表型性状描述和经验为主, 尚未从遗传水平上对两者进行系统解析。
本研究通过对济麦22和良星99的重测序数据分析, 在全基因组水平系统解析了两者的遗传组成差异, 以期对今后小麦育种的亲本利用、重要性状基因区间追溯、新品种系谱分析、基因组水平上研究重要骨干种质的遗传组成等方面提供重要参考。
1 材料与方法
1.1 小麦材料及田间表型考察
供试材料为小麦品种济麦22 (系谱: 935024× 935106)与良星99 [系谱: (济91102×鲁麦14)×PH85-16]。2018年10月1日将上述两个品种播种于中国农业大学上庄实验站(北京, 40°14′N, 116°19′E), 按照完全随机试验设计, 设3次重复, 2行区, 行长1.5 m, 行距25.0 cm, 株距3.0 cm。收获前每小区取中部10株调查株高、穗长、小穗数、穗粒数、旗叶长和宽等农艺性状; 收获后利用考种仪(良田高拍仪S500A3B)考察千粒重、粒长、粒宽等籽粒性状。品种间性状差异检验用t测验进行统计分析, 数据分析通过R语言计算完成。1.2 全基因组重测序与多态性位点鉴定
用CTAB的方法分别从济麦22和良星99幼根提取DNA。用Illumina HiSeq2500测序平台采用双端测序的方法进行全基因组测序, 建库和测序由北京诺禾致源科技股份有限公司完成。对重测序原始数据用Trimmomatic软件(v0.36)[11]进行过滤后再使用BWA软件(v0.7.15)[12]的BWA-MEM工具将过滤后的数据在小麦参考基因组(IWGSCv1)[13]上进行比对, 选择保留存在“唯一最优匹配(Unique Best Hit)”的读段对; 最后利用与samtools (v1.4)[14]工具进行过滤。利用GATK软件(v3.8)[15]中的HaplotypeCaller、GenotypeGVCF、SelectVariants和VariantFiltration等功能计算并过滤获得材料基因组上的高质量SNP和InDel位点。其中, SNP位点过滤参数为“QD < 2.0, FS > 60.0, MQRankSum < -12.5, ReadPosRankSum < -8.0, SOR > 3.0, MQ < 40.0, DP > 30||DP < 3”; InDel过滤参数为“QD < 2.0, FS > 200.0, ReadPosRankSum < -20.0, DP > 30||DP < 3”。对过滤后的SNP与InDel位点通过SNPEff软件(v4.3t)[16]进行注释。使用的中国春基因注释版本为IWGSC RefSeq v1.1 (
1.3 拷贝数变异区间鉴定及比较分析
拷贝数变异(copy-number variation, CNV)是小麦基因组研究的主要多态性类型之一。本研究为鉴定供试材料相对于中国春参照基因组的CNV变异区间, 在分析中以1 Mb为单位将全基因组划分为小窗, 利用bedtools (v2.26.0)[17]计算两个品种的重测序比对读段在每个窗口内的“平均覆盖深度”(Depbin); 并结合该材料的全基因组“平均读段覆盖深度”(Depave)进行归一化, 得到每个小窗的“平均相对覆盖深度”: Depbin/Depave。由于在CNV分析的结果中, 基因组序列丢失相比于序列插入更容易被检测, 本研究计算得到的CNV变异区间仅考虑相对于参照基因组低覆盖度(“丢失”)情况。下文中的CNV均指“丢失(低覆盖度)变异”。“相对平均覆盖深度”低于0.5的小窗被视为“CNV变异区间”(附图1)。CNV变异区间分析均由每个材料分别和“中国春”参照基因组的序列比较计算获得。其中, 在两个品种中同时存在CNV变异的区间被称为“共有CNV变异区间”。附图1
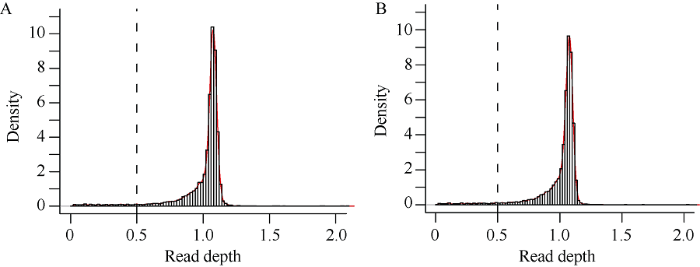
附图1济麦22(A)与良星99(B)全基因组reads覆盖度的密度分布直方图
X轴为经归一化的每1 Mb内的平均reads覆盖度; Y轴为覆盖度的密度。
Supplementary Fig. 1Density plot of bin-wise normalized average read coverage in Jimai 22 (A) and Liangxing 99 (B)
X-axis, the averaged read coverage per 1 Mb after normalization. Y-axis, the distribution density. A and B represent density plot of bin-wise normalized average read coverage in Jimai 22 and Liangxing 99, respectively.
1.4 品种间全基因组高频率SNP差异区段的鉴定
为鉴定两个品种的基因组序列差异区间, 本研究以1 Mb为单位对全基因组进行划分窗口, 对每个窗口中的单核苷酸多态性(single nucleotide polymorphism, SNP)的频率进行分析, 在每个窗口中统计两品种间存在差异的纯合SNP的密度分布(附图2)。这部分研究中仅考虑拷贝数正常的区间, CNV变异区间未被统计。考虑该密度分布具有明显的高斯混合分布的特点, 我们利用EM算法拟合出两个正态分布的模型, 并据此选择阈值x=1.5 (差异频率约为3.1×10-5)作为2个分布的分隔边界。其中, 高密度SNP分布区间被认为两个材料的多态性热点区间; 低密度SNP分布区间被认为两个材料的相似区间。附图2
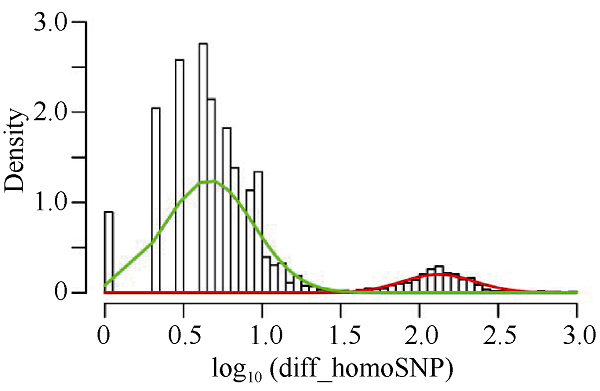
附图2济麦22与良星99间的单区间差异SNP的密度分布图
X轴, 每Mb区间内差异SNP位点数的对数(以10为底); Y轴, 位点数的密度。
Supplementary Fig. 2Density plot of bin-wise density of SNPs different between Jimai 22 and Liangxing 99
X-axis, 10-based logarithm of the counts of differential SNP sites per Mb. Y-axis, the distribution density.
1.5 差异CNV区间和基因序列差异差异位点的验证
对济麦22与良星99基因组内的特有与共有CNV区间分别进行验证, 选取区间内2000 bp左右长度的序列设计染色体区间特异的引物对CNV区间进行验证。扩增引物由在线软件Primer 3.0设计, 其中引物长度范围为18~24 bp, GC含量范围在40%~60%之间, 退火温度范围为54~60℃, 扩增产物大小1~2 kb (表1)。以济麦22、良星99与中国春的DNA为模板进行PCR扩增, 通过有无目标扩增产物判断CNV是否存在。对与小麦株高相关的候选基因TraesCS2D02G051500、TraesCS2D02G055700的序列差异SNP位点, 设计出基因组特异引物(表1)进行PCR扩增并测序。PCR反应体系为20 μL, 包括10 μL的2X M5 HiPer plus Taq HiFi PCR mix, 正、反向引物(10 μmol L-1)各1 μL, 150 ng μL-1模板DNA 2 μL, 用ddH2O补至20 μL。PCR扩增程序为95℃ 3 min; 95℃ 30 s, 56~57.4℃ 30~60 s (依引物退火温度以及目标序列而定), 72℃ 2 min, 35个循环; 72℃ 5 min。Table 1
表1
表1本研究使用的引物编号和序列
Table 1
引物编号 Primer ID | 引物序列 Primer sequence (5'-3') | 退火温度 Annealing temperature (℃) | 用途 Purpose |
---|---|---|---|
TraesCS2D02G055700_F | CAGGTCGAGACAGAGAACAA | 56 | 测序引物 Sequencing primers |
TraesCS2D02G055700_R | ATCGAGCCCCTCAATTTCAT | 58 | CNV标记特异引物 Specific primers for CNV markers |
TraesCS2D02G051500_F | TCAGCTCAGGGTTATCAAGC | ||
TraesCS2D02G051500_R | TTGGGTGCATTTTTCAGTCC | ||
2B_LX99_CNV1_F | AGCAGGTAATCCACACCAAA | ||
2B_LX99_CNV1_R | TGGAGAACCCACTTATCCAA | ||
2B_LX99_CNV2_F | AGCAGGTAATCCACACCAAA | ||
2B_LX99_CNV2_R | AATCCACACCAAATCCCCAT | ||
2B_COM_CNV_F | CACCCTAGATACAACCGAGG | ||
2B_COM_CNV_R | ATGTCACCGATCTTCTGAGC | ||
2D_COM_CNV_F | ATGATCACGATGGACCTAGC | ||
2D_COM_CNV_R | TGCCACGAAGATTTAGAGGA |
新窗口打开|下载CSV
2 结果与分析
2.1 济麦22与良星99的农艺性状比较
田间观察发现2个品种株形相近, 两者除株高、穗长存在显著差异外(P < 0.01) (图1), 旗叶大小、小穗数、千粒重、粒长、粒宽等主要农艺和产量相关性状差异均不显著(表2)。这表明济麦22和良星99在主要农艺和产量性状上存在较高的相似性。图1
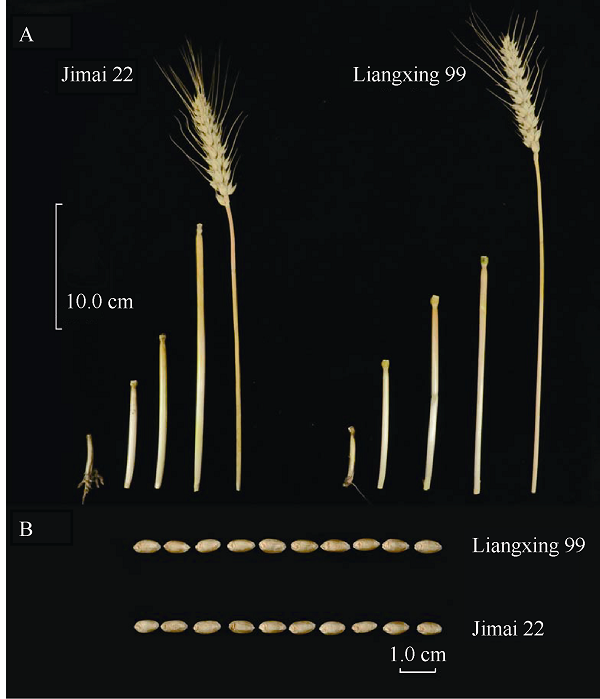
图1济麦22与良星99的表型比较
A: 济麦22 (左)与良星99 (右)的株高及节间长度比较; B: 济麦22 (上)与良星99 (下)的粒型比较。
Fig. 1Morphological comparison between Jimai 22 and Liangxing 99
A: plant height and internode length comparison between Jimai 22 (left) and Liangxing 99 (right); B: grain morphological comparison between Jimai 22 (top) and Liangxing 99 (bottom).
Table 2
表2
表2济麦22与良星99的主要农艺性状差异显著性分析
Table 2
表型 Phenotype | 济麦22 Jimai 22 | 良星99 Liangxing 99 | 差异显著性 Statistical significance of the difference | ||
---|---|---|---|---|---|
均值±标准误差 Mean ± SE | 样本量 Sample counts | 均值±标准误差 Mean ± SE | 样本量 Sample counts | P-value (t-test) | |
旗叶长Flag leaf length (cm) | 16.78±0.36 | 30 | 16.94±0.40 | 30 | 0.76 |
旗叶宽Flag leaf width (cm) | 1.88±0.024 | 30 | 1.85±0.039 | 30 | 0.53 |
每穗小穗数Spikelet number per spike | 21.87±0.45 | 30 | 21.80±0.28 | 30 | 0.91 |
每穗可育小穗数Fertile spikelet per spike | 21.23±0.50 | 30 | 21.17±0.14 | 30 | 1.00 |
每穗不育小穗数Infertile spikelet per spike | 0.63±0.14 | 30 | 0.63±0.23 | 30 | 0.90 |
穗长Spike length (cm) | 9.57±0.13 | 30 | 10.43±0.15 | 30 | 3.98E-5 ** |
株高Plant length (cm) | 75.05±0.57 | 30 | 80.23±0.75 | 30 | 1.90E-6 ** |
穗粒数Grain number per spike | 52.23±0.73 | 30 | 55.20±0.35 | 30 | 0.31 |
千粒重Thousand seed weight (g) | 49.20±0.33 | 6 | 48.30±0.03 | 6 | 0.66 |
粒长Grain length (cm) | 6.43±0.07 | 6 | 6.53±0.03 | 6 | 0.39 |
粒宽Grain width (cm) | 3.25±0.06 | 6 | 3.30±0.02 | 6 | 0.58 |
新窗口打开|下载CSV
2.2 济麦22和良星99全基因组多态性位点检测
利用Illumina HiSeq2500分别对济麦22和良星99进行了全基因组测序, 分别获得了88 Gb和128 Gb的重测序数据。通过和中国春参考基因组序列(IWGSCv1)进行测序数据的比对并去除重复序列, 选择“唯一最优匹配”的读段进行后续分析, 最终两品种的平均覆盖深度均为5.8×。经过SNP calling分析, 济麦22和良星99中分别鉴定出15,326,314个与15,060,004个和中国春参照基因组不同的高质量纯合SNP位点, 约占全基因组的0.109%和0.107% (表3)。其中, A、B基因组中的SNP的频率(0.130%~ 0.155%)明显高于D基因组(0.0224%~0.0225%)。两品种间基因型不一致的纯合SNP位点仅占总SNP位点个数的9.8%, 即超过90%的SNP是两个品种间共有的。Table 3
表3
表3济麦22与良星99的纯合SNP位点统计
Table 3
基因组 Genome | 济麦22中的纯合 SNP个数 Number of homozygous SNPs in Jimai 22 | 良星99中的纯合 SNP个数 Number of homozygous SNPs in Liangxing 99 | 两者基因型相同的纯合SNP个数 Number of homozygous SNPs with the same genotype | 两者基因型不同的纯合 SNP个数 Number of homozygous SNPs with different genotypes |
---|---|---|---|---|
A | 6,405,063 | 6,395,080 | 6,194,047 | 301,206 |
B | 8,033,789 | 7,781,369 | 7,248,448 | 1,148,764 |
D | 887,462 | 883,555 | 818,951 | 97,401 |
全基因组 Whole genome | 15,326,314 | 15,060,004 | 14,261,446 | 1,547,371 |
新窗口打开|下载CSV
同时, 在济麦22和良星99中分别鉴定出1,143,512与1,118,564个的InDel位点(表4), 分别占全基因组的0.0813%和0.0795%。与D基因组, A、B基因组中鉴定出的InDel频率更高。济麦22和良星99间差异的InDel多态性位点占鉴定出的InDel位点总数的11.8%。
Table 4
表4
表4济麦22与良星99的纯合InDel位点统计
Table 4
基因组 Genome | 济麦22中的纯合 InDel个数 Number of homozygous InDels in Jimai 22 | 良星99中的纯合 InDel个数 Number of homozygous InDels in Liangxing 99 | 两者基因型相同的纯合InDel个数 Number of homozygous InDels with the same genotype | 两者基因型不同的纯合 InDel个数 Number of homozygous InDels with different genotypes |
---|---|---|---|---|
A | 464,369 | 459,599 | 438,410 | 28,732 |
B | 562,658 | 543,395 | 492,112 | 95,316 |
D | 116,485 | 115,570 | 104,345 | 13,769 |
全基因组Whole genome | 1,143,512 | 1,118,564 | 1,034,867 | 137,817 |
新窗口打开|下载CSV
2.3 济麦22与良星99的基因组CNV区间的鉴定与比较
济麦22的基因组相对于中国春参照基因组中存在557 Mb的CNV变异区间, 占全基因组的4.0%; 而在良星99基因组中检测出511 Mb的CNV变异区间, 占全基因组的3.6% (表5)。其中, 两品种共有的CNV变异区间的长度为466 Mb, 主要集中于2B、2D、5A和6A染色体; 两品种特有的CNV区间分别为91 Mb和45 Mb, 共计约占总CNV区间的22.6%, 主要集中在2B和4B染色体上(图2)。Table 5
表5
表5济麦22与良星99的全基因组CNV区间长度统计
Table 5
基因组 Genome | 济麦22的特有CNV区间 Specific CNV region in Jimai 22 | 良星99的特有CNV区间 Specific CNV region in Liangxing 99 | 两品种的共有CNV区间 Common CNV regions in the two cultivars | |||
---|---|---|---|---|---|---|
长度 Length (Mb) | % | 长度 Length (Mb) | % | 长度 Length (Mb) | % | |
A | 8 | 0.06 | 4 | 0.03 | 135 | 0.96 |
B | 80 | 0.57 | 33 | 0.23 | 226 | 1.61 |
D | 3 | 0.02 | 8 | 0.06 | 105 | 0.75 |
全基因组Whole genome | 91 | 0.65 | 45 | 0.32 | 466 | 3.31 |
新窗口打开|下载CSV
图2
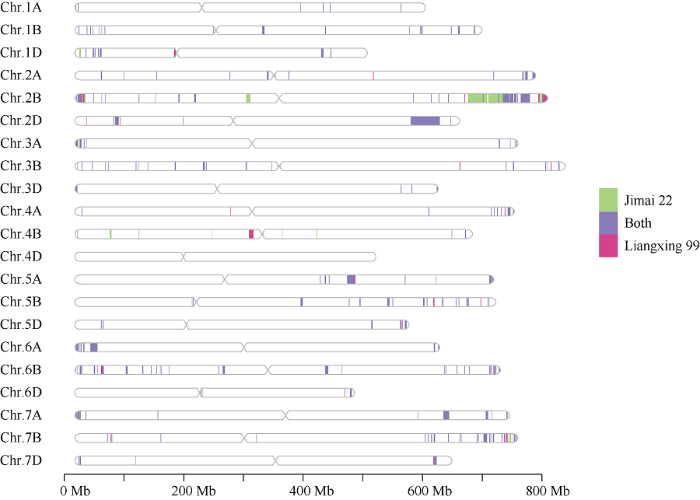
图2济麦22与良星99的CNV区间(相对于中国春参照基因组)在各染色体上的分布
绿色: 济麦22的特有CNV区间; 粉色: 良星99的特有CNV区间; 紫色: 济麦22与良星99的共有CNV区间。
Fig. 2Distribution of CNV regions detected in Jimai 22 and Liangxing 99 compared to the Chinese Spring reference genome
Green: specific CNV regions in Jimai 22; Pink: specific CNV regions in Liangxing 99; Purple: common CNV regions in the two varieties.
为了验证CNV区间的分析结果, 在2B和2D染色体上的CNV差异区间中设计了特异PCR引物。其中2B_LX99_CNV1与2B_LX99_CNV2位于2B染色体上良星99特有CNV区间(Chr.2B: 667 Mb~ 726 Mb), 2B_COM_CNV位于2B染色体上良星99与济麦22共有的CNV区间(Chr. 2B: 738 Mb~769 Mb), 2D_COM_CNV位于2D染色体上济麦22与良星99共有的CNV区间(Chr. 2D: 570 Mb~638 Mb)。利用这4对引物在济麦22、良星99和中国春材料内进行PCR扩增, 结果表明材料间的差异CNV区间可以通过扩增条带的有无进行验证(图3); 其中2B_ LX99_CNV1与2B_LX99_CNV2在中国春与济麦22中均扩增出目标条带, 但未在良星99中扩增出条带, 证明该区间仅在良星99中丢失, 2B_ COM_CNV与2D_COM_CNV仅在中国春中扩增出目标条带, 证明该区间在济麦22与良星99中均丢失。PCR验证结果与全基因组分析结果吻合, 表明该分析方法计算得到的CNV变异准确可靠。
图3
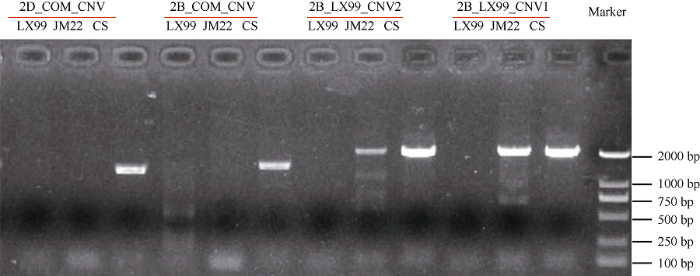
图3利用PCR对济麦22(JM22)、良星99(LX99)和中国春(CS)中检测的CNV区间进行验证
2D_COM_CNV和2B_COM_CV分别为2D和2B染色体上共有区间序列设计的引物; 2B_LX99_CNV1和2B_LX99_CNV2为在2B染色体上良星99特有的CNV区间中序列设计的引物。每对引物对应的3个泳道从左到右分别为: 良星99(LX99)、济麦22(JM22)和中国春(CS)。
Fig. 3PCR validation of detected CNVs in Jimai 22 (JM22), Liangxing 99 (LX99) and Chinese Spring (CS)
2D_COM_CNV and 2B_COM_CNV were designed utilizing sequences in common CNV regions in 2D and 2B chromosomes, respectively; 2B_LX99_CNV1 and 2B_ LX99_CNV2 were designed utilizing sequences in Liangxing 99-specific CNV regions in chromosome 2B. The corresponding three lanes from left to right for each primer are Liangxing 99 (LX99), Jimai 22 (JM 22) and Chinese Spring (CS).
通过对济麦22与良星99中特有的CNV区间内高可信度(HC)基因进行GO富集分析发现, 在济麦22的特有CNV区间内有982个基因, 其中294个基因显著富集在25个GO条目, 主要包括“ADP 结合”(GO: 0043531)、“肽酶抑制剂活性”(GO: 0030414), 辅酶结合”(GO: 0050662)、“酶抑制剂活性”(GO: 0004857)、“半胱氨酸型内肽酶抑制剂活性”(GO: 0004869)等分子功能, 以及“肽酶活性负调控”(GO: 0010466)、“内肽酶活性负调控”(GO: 0010951)、“催化活性负调控”(GO: 0043086)、“碳代谢过程”(GO: 0008152)等生物学过程中, 细胞组分分类内未有富集(图4-A)。在良星99特有CNV区间内包括513个基因, 其中富集到的395个基因分布在25个GO条目中, 主要有“ADP结合”(GO: 0043531)、“血红素结合”(GO: 0020037)、“过氧化物酶活性”(GO: 0004601)、“萜烯合酶活性”(GO: 0010333)、“O-甲基转移酶活性”(GO: 0008171)、“腺苷脱氨酶活性”(GO: 0004000)等分子功能, 以及“抗氧化反应”(GO: 0006979)、“过氧化氢分解过程”(GO: 0042744)、“细胞氧化解毒”(GO: 0098869)、“蛋白磷酸化”(GO: 0006468)、“糖原生物合成”(GO: 0005978)等生物学过程和“胞外区”(GO:0005576)这一细胞组分中(图4-B)。济麦22特有CNV区间内富集在GO条目, 如“生长素反应”(GO: 0009733), “色素合成过程”(GO: 0046148)下的基因, 可能是影响两材料间的表型差异的候选基因。
图4
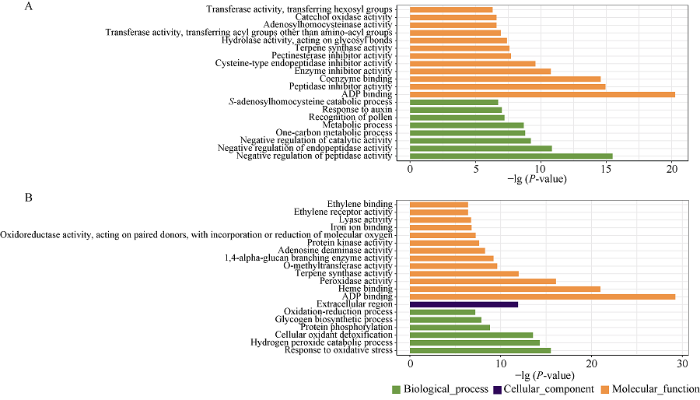
图4济麦22(A)与良星99(B)特有CNV区间中基因的GO富集分析结果
绿色: 生物过程; 紫色: 细胞组分; 橙色: 分子功能。
Fig. 4GO enrichment analysis of the genes in Jimai 22 (A) and Liangxing 99 (B) specified CNV regions
2.4 济麦22和良星99间的SNP多态性热点区间和序列相似区间分析
以中国春IWGSCv1参考基因组为模板, 利用剔除品种间大片段CNV后的13.5 G大小的基因组序列进行分析。以差异纯合SNP的密度为3.1×10-5为阈值将染色体区分为“多态性热点区间”(高密度差异SNP区间)和“序列相似区间”(低密度差异SNP区间)。济麦22与良星99之间共有1915 Mb (14.2%)区间为多态性热点区间(图5-A)。在多态性热点区间中, 两品种间有1,375,981个纯合差异SNP位点, 约占两品种全部纯合差异SNP位点总数的96.5% (图5-B), 这些多态性热点区间主要集中于1D、2B、3B和4B染色体(图5-C)。图5
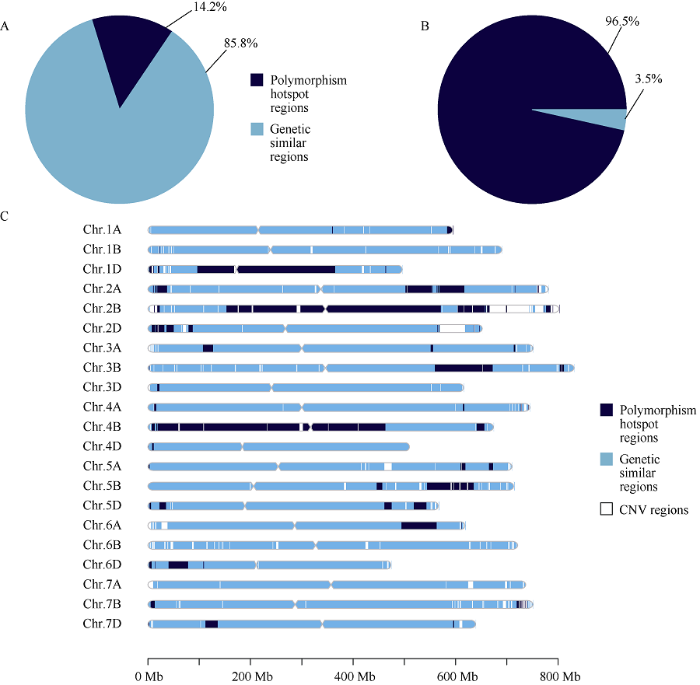
图5济麦22与良星99全基因组内多态性热点区间分布
A: 两品种间差异SNP位点中分布在多态性热点区间与序列相似区间的比例; B: 多态性热点区间与序列相似区间的长度在全基因组上的比例; C: 多态性热点区间与序列相似区间在全基因组分布。深蓝色: 多态性热点区间; 浅蓝色: 序列相似区间; 白色: CNV区间。
Fig. 5Statistics and distribution of the SNP hotspot regions between Jimai 22 and Liangxing 99
A: pie chart for the proportions of differential SNPs in the polymorphism hotspot regions (PHRs) and the genetic similar regions (GSRs); B: pie chart for the proportions of the PHRs and GSRs in length; C: distributions of the PHRs and the GSRs across chromosomes.
通过对两品种多态性热点区间内的14,306个高可信度基因进行GO富集分析, 其中1461个基因显著富集在20个GO条目中, 主要包括“5'-3' RNA聚合酶活性” (GO: 0003899)、“二磷酸核酮糖羧化酶活性” (GO: 0016984)、“光合作用的循环电子转运通路内转移电子的电子转运器活性” (GO: 0045156)、“醛糖1-表异构酶活性” (GO: 0004034)、以NAD或NADP为受体提供CH-OH基团的氧化还原酶活性” (GO: 0016616)等分子功能, “光合作用” (GO: 0015979)、“己糖代谢过程” (GO: 0019318)和“光系统II中的光合电子传输” (GO: 0009772)等生物过程, 以及“光系统II” (GO: 0009523)、“光系统II反应中心” (GO: 0009539)、“叶绿体” (GO: 0009507)、“质体” (GO: 0009536)和“类囊体” (GO: 0007579)等细胞组分内(图6)。综合看来, 该部分基因的GO富集分析结果主要集中在光合作用通路相关条目。
图6
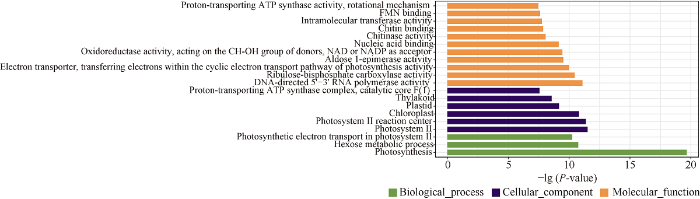
图6济麦22与良星99间多态性热点区间的基因GO富集分析结果
绿色: 生物过程; 紫色: 细胞组分; 橙色: 分子功能。
Fig. 6GO enrichment result of the genes in the SNP hotspot regions between in Jimai 22 and Liangxing 99
2.5 济麦22和良星99的多态性热点区间和序列相似区间的突变类型分析
为比较两品种间多态性热点区间和序列相似区间中的突变类型的异同, 我们首先统计差异SNP位点的碱基变异类型的频率(图7-A和表6)。无论在多态性热点区间还是序列相似区间, T?C和G?A类型的突变频率均为最多, 在多态性热点区间内约占71.4%, 略高于在序列相似区间内所占总SNP的比例(66.5%)。同时, 我们还统计了两品种间差异InDel的长度分布(图7-B和表7)。多态性热点区间中的单碱基的InDel占该区间内所有InDel位点的64.7%, 其比例高于单碱基的InDel在序列相似区间中所占的比例(49.0%)。图7
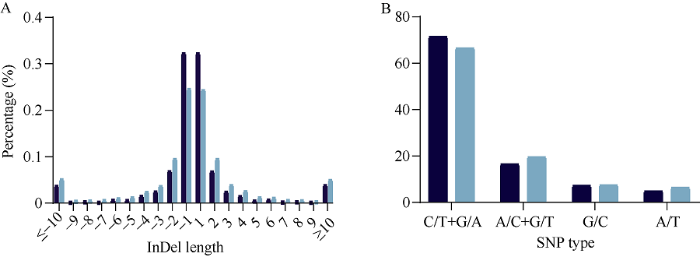
图7济麦22与良星99间多态性热点区间与序列相似区间中各自的InDel长度分布(A)和SNP突变类型(B)
深蓝: 多态性热点区间; 浅蓝: 序列相似区间。
Fig. 7Length distributions of homozygous InDels (A) and mutation types of homozygous SNPs (B) in the polymorphism hotspot regions and genetic similar regions between Jimai 22 and Liangxing 99
Dark blue: polymorphism hotspot regions; Light blue: genetic similar regions.
Table 6
表6
表6济麦22与良星99全基因组差异纯合SNP的突变类型的统计
Table 6
SNP突变类型 Types of SNP mutations | 多态性热点区间 Differential genetic region | 相似区间 Similar genetic region | 全基因组 Whole genome region | |||
---|---|---|---|---|---|---|
数量 Counts | 每Mb密度 Density per Mb | 数量 Counts | 每Mb密度 Density per Mb | 数量 Counts | 每Mb密度 Density per Mb | |
T>A | 33,545 | 17.52 | 1701 | 0.15 | 35,246 | 2.62 |
A>T | 33,868 | 17.69 | 1713 | 0.15 | 35,581 | 2.64 |
G>C | 49,065 | 25.62 | 2020 | 0.17 | 51,085 | 3.79 |
C>G | 49,521 | 25.86 | 1956 | 0.17 | 51,477 | 3.82 |
A>C | 47,712 | 24.91 | 2089 | 0.18 | 49,801 | 3.70 |
T>G | 47,862 | 24.99 | 1888 | 0.16 | 49,750 | 3.69 |
G>T | 65,670 | 34.29 | 3204 | 0.28 | 68,874 | 5.11 |
C>A | 66,047 | 34.49 | 3226 | 0.28 | 69,273 | 5.14 |
A>G | 200,697 | 104.80 | 6364 | 0.55 | 207,061 | 15.37 |
T>C | 201,236 | 105.08 | 6540 | 0.57 | 207,776 | 15.42 |
G>A | 289,933 | 151.40 | 11,276 | 0.98 | 301,209 | 22.36 |
C>T | 290,292 | 151.59 | 11,151 | 0.96 | 301,443 | 22.38 |
转换Transition | 982,158 | 512.88 | 35,331 | 3.06 | 1,017,489 | 75.53 |
颠换Transversion | 393,290 | 205.37 | 17,797 | 1.54 | 411,087 | 30.51 |
全部Total | 1,375,448 | 718.25 | 53,128 | 4.60 | 1,428,576 | 106.04 |
新窗口打开|下载CSV
Table 7
表7
表7济麦22与良星99的纯合InDel变异的长度分布
Table 7
InDel类型 Types of InDel | InDel长度 Length of InDel | 差异区间 Differential genetic region | 相似区间 Similar genetic region | 全基因组 Whole genome region | |||
---|---|---|---|---|---|---|---|
数量 Count | 每Mb密度 Density per Mb | 数量 Count | 每Mb密度 Density per Mb | 数量 Count | 每Mb密度 Density per Mb | ||
在济麦22中插入, 在良星99中丢失 Insertion in Jimai 22, deletion in Liangxing 99 | 1 | 34,031 | 17.77 | 7723 | 0.67 | 41,754 | 3.10 |
2 | 7259 | 3.79 | 3017 | 0.26 | 10,276 | 0.76 | |
3 | 2664 | 1.39 | 1242 | 0.11 | 3906 | 0.29 | |
4 | 1685 | 0.88 | 843 | 0.07 | 2528 | 0.19 | |
5 | 701 | 0.37 | 419 | 0.04 | 1120 | 0.08 | |
6 | 883 | 0.46 | 370 | 0.03 | 1253 | 0.09 | |
7 | 453 | 0.24 | 225 | 0.02 | 678 | 0.05 | |
8 | 476 | 0.25 | 216 | 0.02 | 692 | 0.05 | |
9 | 363 | 0.19 | 153 | 0.01 | 516 | 0.04 | |
≥10 | 4131 | 2.16 | 1599 | 0.14 | 5730 | 0.43 | |
全部Total | 52,646 | 27.49 | 15,807 | 1.37 | 68,453 | 5.08 | |
在良星99中插入, 在济麦22中丢失 Insertion in Liangxing 99, deletion in Jimai 22 | 1 | 34,083 | 17.80 | 7796 | 0.67 | 41,879 | 3.11 |
2 | 7315 | 3.82 | 3037 | 0.26 | 10,352 | 0.77 | |
3 | 2690 | 1.40 | 1190 | 0.10 | 3880 | 0.29 | |
4 | 1710 | 0.89 | 785 | 0.07 | 2495 | 0.19 | |
5 | 746 | 0.39 | 420 | 0.04 | 1166 | 0.09 | |
6 | 832 | 0.43 | 356 | 0.03 | 1188 | 0.09 | |
7 | 412 | 0.22 | 227 | 0.02 | 639 | 0.05 | |
8 | 509 | 0.27 | 208 | 0.02 | 717 | 0.05 | |
9 | 402 | 0.21 | 183 | 0.02 | 585 | 0.04 | |
≥10 | 3993 | 2.09 | 1637 | 0.14 | 5630 | 0.42 | |
全部Total | 52,696 | 27.52 | 15,835 | 1.37 | 68,531 | 5.09 |
新窗口打开|下载CSV
我们分别对多态性热点区间与序列相似区间内两品种间差异SNP与InDel位点对于基因功能的影响进行了注释与比较(表8), 结果表明多态性热点区间与序列相似区间中基因序列上的SNP和InDel变异的类型主要以错义突变(missense variation)和同义突变(synonymous variation)为主。在多态性热点区间中错义突变(51.3%)与终止子相关突变(stop-codon gain/lost)(1.5%)所占比例要分别略高于两者在序列相似区间的44.7%和0.8%比例。
Table 8
表8
表8济麦22与良星99的纯合点突变对蛋白编码功能影响的统计
Table 8
变异所在区间 Region of variations | 错义突变 Missense mutation | 同义突变 Synonymous mutation | 移码突变 Frame-shift mutation | 提前终止突变 Stop-gained mutation | 终止子丢失突变 Stop-lost mutation | |||||
---|---|---|---|---|---|---|---|---|---|---|
数量 Count | % | 数量 Count | % | 数量 Count | % | 数量 Count | % | 数量 Count | % | |
差异区间Different region | 2981 | 51.3 | 2431 | 41.9 | 304 | 5.2 | 68 | 1.2 | 16 | 0.3 |
相似区间Similar region | 348 | 44.7 | 330 | 42.4 | 94 | 12.1 | 6 | 0.8 | 0 | 0 |
全部Total | 3329 | 50.6 | 2761 | 42.0 | 398 | 6.1 | 74 | 1.1 | 16 | 0.2 |
新窗口打开|下载CSV
2.6 株高候选基因在济麦22和良星99之间的序列差异分析及重测序结果验证
济麦22与良星99在株高和穗长上存在显著差异, 利用两者的重测序数据对小麦目前已克隆的矮秆基因Rht-B1[18]、Rht-D1[18]、株高相关基因Ppd-D1[19]和在2D染色体的QPht/Sl.cau-2D.1内定位到2个株高穗长候选基因[20]等进行序列分析, 发现QPht/Sl.cau-2D.1区间内的2个基因在品种间存在序列差异(表9)。Table 9
表9
表9小麦株高相关基因列表及在序列相似区间和多态性热点区间的分布
Table 9
株高基因/QTL Plant height gene/QTL | 基因编号 Gene ID | 染色体 Chromosome | 区间 Region | 基因功能注释 Function annotation | 序列差异类型 Variation type |
---|---|---|---|---|---|
Rht-B1 | TraesCS4B02G043100 | 4B | 多态性热点区间 Polymorphic hotspot regions | 编码DELLA蛋白 DELLA protein-coding gene | 无 None |
Rht-D1 | TraesCS4D02G040400 | 4D | 相似区间 Genetic similar region | 编码DELLA蛋白 DELLA protein-coding gene | 无 None |
Ppd-D1 | TraesCS2D02G079600 | 2D | 相似区间 Genetic similar region | 编码PRRs蛋白 PRRs protein-coding gene | 无 None |
QPht/Sl.cau-2D.1 | TraesCS2D02G051500 | 2D | 多态性热点区间 Polymorphic hotspot regions | 编码WRKY转录因子 WRKY transcription factor protein-coding gene | InDel |
QPht/Sl.cau-2D.1 | TraesCS2D02G055700 | 2D | 多态性热点区间 Polymorphic hotspot regions | 编码GRAS转录因子 GRAS transcription factor protein-coding gene | InDel, SNP |
新窗口打开|下载CSV
利用基因特异性引物对QPht/Sl.cau-2D.1 QTL区间内的TraesCS2D02G051500和TraesCS2D02 G055700基因分别在济麦22与良星99中进行扩增、测序发现, 2个基因在品种间存在引起氨基酸改变的差异位点(图8)。该QTL区间是矮秆基因Rht8所在区间, 该位点对小麦的株高和穗长均有影响[21], 但其遗传功能仍需进一步试验验证。
图8
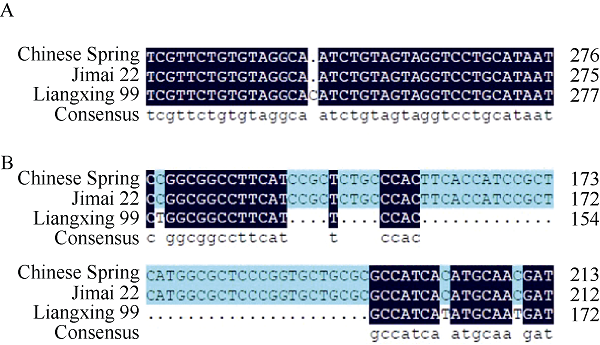
图8TraesCS2D02G051500(A)与TraesCS2D02G055700(B)在中国春、济麦22和良星99中功能改变位点附近的核苷酸序列
Fig. 8Sequence of TraesCS2D02G051500 (A) and TraesCS 2D02G055700 (B) near the coding-function affected sites in Chinese Spring, Jimai 22, and Liangxing 99
3 讨论
本研究通过对济麦22和良星99两个小麦品种的重测序数据进行分析, 系统研究了两个品种在基因组水平上存在的CNV区间和SNP热点区间。与中国春参照基因组比较发现, 两个品种均有大量CNV丢失变异区间, 约占全基因组的4.3%。除集中分布在2B染色体上148 Mb的CNV变异外, 大部分CNV变异区间零散地分布在不同染色体上。这一结果表明, 与中国春参考基因组序列相比, 济麦22和良星99中存在大量的CNV变异(特别是CNV丢失变异)。通过比较2个材料中CNV变异区间的异同, 发现大部分CNV区间是共有的, 但仍有22.6%的差异CNV区间; 其中60.3%的差异CNV区间集中于2B染色体。需要指出的是, 本研究中发现的“CNV缺失区间”是通过读段比对后的覆盖度确定的, 不能排除该区域可能来源于遗传距离较远的基因组序列的可能性。根据品种间差异SNP的分布密度对染色体区间进行了划分, 鉴定出基因组14.2%的区间是两品种间的多态性热点区间, 集中了全基因组96.5%差异SNP位点, 这些区间主要位于1D、2B和4B染色体上。通过鉴定相似区间和多态性热点区间变异位点的特征, 发现两类区间在SNP变异类型、InDel长度分布、突变影响编码功能的比例上有一定区别。上述结果表明济麦22和良星99在全基因组水平具有较高的相似性, 但在特定的染色体上确实存大片段的差异区间。济麦22和良星99的主要农艺和产量性状存在较高相似性, 只有在株高和穗长上存在差异。结合品种间序列差异位点分析, 我们推测控制品种间株高和穗长的差异基因可能位于差异SNP热点区域或差异CNV区间。结合目前已经在小麦中克隆的株高或穗长的5个相关基因, 并分析两品种间基因序列, 发现了2个位于差异SNP热点区且存在影响编码功能的变异的候选基因。本研究在已有基因组变异和表型信息的基础上对已知性状相关基因和品种间差异序列区间进行了初步分析, 找到了位于多态性热点区间的2个具有序列差异的基因, 但其生物学功能和关联仍需进一步验证。利用表型差异和全基因组序列多态性分析相结合的方法, 本研究的方法可为今后借助基因组测序方法快速定位和克隆候选基因提供了新的参考。
Zhao等[22]研究发现, 良星99的2BL上有一个控制抗白粉病的主效基因, 该基因被命名为Pm52[23]。利用中国春参照基因组的信息, 将Pm52基因定位在chr2B的581 Mb~596 Mb的区间[24]。本研究的结果显示, 济麦22和良星99的2BL的绝大部分区间为多态性热点区间, 但575 Mb~603 Mb区段为本研究中鉴定的“序列相似区间”, 该区段包含Pm52所在的物理区间; 本研究间接支持了Qu等[24]在济麦22和良星99的衍生材料中均携带相同的Pm52基因的结论。该结果也表明本研究鉴定的序列相似区间可为抗病等关键性状基因的定位研究提供参考。
小麦品种的重测序研究为探索小麦基因组序列的多态性提供高维度、高精细度、类型丰富的基因组变异信息。本研究也为比较小麦品种间的基因组序列差异分析提供了方法学参考。随着小麦材料重测序数据的不断积累, 小麦品种间基因组序列变异的特征和规律也将得到进一步揭示。有效分析和利用丰富的基因组变异信息, 将有助于对关键性状基因功能进行阐释, 辅助小麦育种对优异基因区间的利用, 提高小麦品种选育的效率。
4 结论
济麦22和良星99两个重要小麦品种的田间表型相似。本研究通过对两个品种进行全基因序列差异分析发现, 济麦22和良星99间的遗传组成相似性较高(序列相似区间占85.8%), 但仍存在大区段CNV变异(136 M)和占全基因组14.2%的差异多态性热点区间; 其中差异SNP热点区间主要集中在1D、2B和4B染色体上。本工作为进一步研究和利用济麦22和良星99提供了重要的基因组变异数据; 也为小麦品种间的基因组变异热点区间的鉴定提供了方法参考。说明: 本研究中的原始测序数据已提交至中国科学院北京基因组研究所BIG数据中心[25]的组学原始数据归档库[26]中(
参考文献 原文顺序
文献年度倒序
文中引用次数倒序
被引期刊影响因子
[本文引用: 1]
[本文引用: 1]
[本文引用: 1]
[本文引用: 1]
[本文引用: 1]
[本文引用: 1]
DOI:10.3724/SP.J.1006.2012.00954URL [本文引用: 1]

为了解骨干亲本鲁麦14对山东新选育小麦品种的遗传贡献,对鲁麦14及其6个衍生品种(系)进行了全基因组SSR扫描分析。在350个SSR位点上共检测到662个等位变异,每个位点1~5个等位变异,平均1.9个, 位点平均多态性指数(PIC)为0.21。UPGMA聚类分析表明,济麦22和鲁麦14聚为一类、青丰系列4个品种(系)聚为一类,这两类形成一个大的分支,而济麦20与这些品种的关系较远。在所检测的350个位点中,与鲁麦14相同的位点数,济麦22有235个(67.1%), 济麦20有210个(60.0%), 青丰1号有229个(65.4%), 青农2号有247个(70.6%)。这些相同位点多数以一个大的染色体区段传递至子代,且有的区段在6个衍生品种(系)中共享,如5A的gwm304–barc360–gwm415–barc1区段和6D的barc196–gdm127–barc123区段等。济麦22在21条染色体上都有与鲁麦14相同的位点,但染色体间差异较大,相同位点比例超过70%的染色体有3A、4A、7A,2B、4B、7B、1D、3D和4D;相同位点比例最低的是3B,仅46%。同一连锁群上,位点之间多呈连续区段分布,大多与已发现的重要性状分布簇相吻合。因此认为,鲁麦14的优良遗传背景对济麦22有重要贡献。在育种实践中,除需关注重要基因的导入外,还应注意骨干材料主体背景的选择。
[本文引用: 1]
[本文引用: 1]
[本文引用: 1]
[本文引用: 1]
[本文引用: 1]
DOI:10.1016/j.pbi.2017.01.007URLPMID:28346895 [本文引用: 1]

Advances in wheat genomics have lagged behind other major cereals (e.g., rice and maize) due to its highly repetitive and large polyploid genome. Recent technological developments in sequencing and assembly methods, however, have largely overcome these barriers. The community now moves to an era centred on functional characterisation of the genome. This includes understanding sequence and structural variation as well as how information is integrated across multiple homoeologous genomes. This understanding promises to uncover variation previously hidden from natural and human selection due to the often observed functional redundancy between homoeologs. Key functional genomic resources will enable this, including sequenced mutant populations and gene editing technologies which are now available in wheat. Training the next-generation of genomics-enabled researchers will be essential to ensure these advances are quickly translated into farmers' fields.
DOI:10.3724/SP.J.1006.2009.01425URL [本文引用: 1]
[本文引用: 1]
[本文引用: 1]
[本文引用: 1]
DOI:10.1016/S2095-3119(19)62644-4URL [本文引用: 1]
DOI:10.1093/bioinformatics/btu170URL [本文引用: 1]

Results: The value of NGS read preprocessing is demonstrated for both reference-based and reference-free tasks. Trimmomatic is shown to produce output that is at least competitive with, and in many cases superior to, that produced by other tools, in all scenarios tested.]]>
DOI:10.1093/bioinformatics/btp324URLPMID:19451168 [本文引用: 1]

MOTIVATION: The enormous amount of short reads generated by the new DNA sequencing technologies call for the development of fast and accurate read alignment programs. A first generation of hash table-based methods has been developed, including MAQ, which is accurate, feature rich and fast enough to align short reads from a single individual. However, MAQ does not support gapped alignment for single-end reads, which makes it unsuitable for alignment of longer reads where indels may occur frequently. The speed of MAQ is also a concern when the alignment is scaled up to the resequencing of hundreds of individuals. RESULTS: We implemented Burrows-Wheeler Alignment tool (BWA), a new read alignment package that is based on backward search with Burrows-Wheeler Transform (BWT), to efficiently align short sequencing reads against a large reference sequence such as the human genome, allowing mismatches and gaps. BWA supports both base space reads, e.g. from Illumina sequencing machines, and color space reads from AB SOLiD machines. Evaluations on both simulated and real data suggest that BWA is approximately 10-20x faster than MAQ, while achieving similar accuracy. In addition, BWA outputs alignment in the new standard SAM (Sequence Alignment/Map) format. Variant calling and other downstream analyses after the alignment can be achieved with the open source SAMtools software package. AVAILABILITY: http://maq.sourceforge.net.
DOI:10.1126/science.aav0887URLPMID:30262485 [本文引用: 1]
DOI:10.1093/bioinformatics/btp352URLPMID:19505943 [本文引用: 1]

SUMMARY: The Sequence Alignment/Map (SAM) format is a generic alignment format for storing read alignments against reference sequences, supporting short and long reads (up to 128 Mbp) produced by different sequencing platforms. It is flexible in style, compact in size, efficient in random access and is the format in which alignments from the 1000 Genomes Project are released. SAMtools implements various utilities for post-processing alignments in the SAM format, such as indexing, variant caller and alignment viewer, and thus provides universal tools for processing read alignments. AVAILABILITY: http://samtools.sourceforge.net.
[本文引用: 1]
DOI:10.4161/fly.19695URLPMID:22728672 [本文引用: 1]

We describe a new computer program, SnpEff, for rapidly categorizing the effects of variants in genome sequences. Once a genome is sequenced, SnpEff annotates variants based on their genomic locations and predicts coding effects. Annotated genomic locations include intronic, untranslated region, upstream, downstream, splice site, or intergenic regions. Coding effects such as synonymous or non-synonymous amino acid replacement, start codon gains or losses, stop codon gains or losses, or frame shifts can be predicted. Here the use of SnpEff is illustrated by annotating ~356,660 candidate SNPs in ~117 Mb unique sequences, representing a substitution rate of ~1/305 nucleotides, between the Drosophila melanogaster w(1118); iso-2; iso-3 strain and the reference y(1); cn(1) bw(1) sp(1) strain. We show that ~15,842 SNPs are synonymous and ~4,467 SNPs are non-synonymous (N/S ~0.28). The remaining SNPs are in other categories, such as stop codon gains (38 SNPs), stop codon losses (8 SNPs), and start codon gains (297 SNPs) in the 5'UTR. We found, as expected, that the SNP frequency is proportional to the recombination frequency (i.e., highest in the middle of chromosome arms). We also found that start-gain or stop-lost SNPs in Drosophila melanogaster often result in additions of N-terminal or C-terminal amino acids that are conserved in other Drosophila species. It appears that the 5' and 3' UTRs are reservoirs for genetic variations that changes the termini of proteins during evolution of the Drosophila genus. As genome sequencing is becoming inexpensive and routine, SnpEff enables rapid analyses of whole-genome sequencing data to be performed by an individual laboratory.
DOI:10.1093/bioinformatics/btq033URLPMID:20110278 [本文引用: 1]

MOTIVATION: Testing for correlations between different sets of genomic features is a fundamental task in genomics research. However, searching for overlaps between features with existing web-based methods is complicated by the massive datasets that are routinely produced with current sequencing technologies. Fast and flexible tools are therefore required to ask complex questions of these data in an efficient manner. RESULTS: This article introduces a new software suite for the comparison, manipulation and annotation of genomic features in Browser Extensible Data (BED) and General Feature Format (GFF) format. BEDTools also supports the comparison of sequence alignments in BAM format to both BED and GFF features. The tools are extremely efficient and allow the user to compare large datasets (e.g. next-generation sequencing data) with both public and custom genome annotation tracks. BEDTools can be combined with one another as well as with standard UNIX commands, thus facilitating routine genomics tasks as well as pipelines that can quickly answer intricate questions of large genomic datasets. AVAILABILITY AND IMPLEMENTATION: BEDTools was written in C++. Source code and a comprehensive user manual are freely available at http://code.google.com/p/bedtools CONTACT: aaronquinlan@gmail.com; imh4y@virginia.edu SUPPLEMENTARY INFORMATION: Supplementary data are available at Bioinformatics online.
DOI:10.1038/22307URLPMID:10421366 [本文引用: 2]

World wheat grain yields increased substantially in the 1960s and 1970s because farmers rapidly adopted the new varieties and cultivation methods of the so-called 'green revolution'. The new varieties are shorter, increase grain yield at the expense of straw biomass, and are more resistant to damage by wind and rain. These wheats are short because they respond abnormally to the plant growth hormone gibberellin. This reduced response to gibberellin is conferred by mutant dwarfing alleles at one of two Reduced height-1 (Rht-B1 and Rht-D1) loci. Here we show that Rht-B1/Rht-D1 and maize dwarf-8 (d8) are orthologues of the Arabidopsis Gibberellin Insensitive (GAI) gene. These genes encode proteins that resemble nuclear transcription factors and contain an SH2-like domain, indicating that phosphotyrosine may participate in gibberellin signalling. Six different orthologous dwarfing mutant alleles encode proteins that are altered in a conserved amino-terminal gibberellin signalling domain. Transgenic rice plants containing a mutant GAI allele give reduced responses to gibberellin and are dwarfed, indicating that mutant GAI orthologues could be used to increase yield in a wide range of crop species.
DOI:10.1007/s00122-007-0603-4URL [本文引用: 1]

Ppd-D1 on chromosome 2D is the major photoperiod response locus in hexaploid wheat (Triticum aestivum). A semi-dominant mutation widely used in the “green revolution” converts wheat from a long day (LD) to a photoperiod insensitive (day neutral) plant, providing adaptation to a broad range of environments. Comparative mapping shows Ppd-D1 to be colinear with the Ppd-H1 gene of barley (Hordeum vulgare) which is a member of the pseudo-response regulator (PRR) gene family. To investigate the relationship between wheat and barley photoperiod genes we isolated homologues of Ppd-H1 from a ‘Chinese Spring’ wheat BAC library and compared them to sequences from other wheat varieties with known Ppd alleles. Varieties with the photoperiod insensitive Ppd-D1a allele which causes early flowering in short (SD) or LDs had a 2kb deletion upstream of the coding region. This was associated with misexpression of the 2D PRR gene and expression of the key floral regulator FT in SDs, showing that photoperiod insensitivity is due to activation of a known photoperiod pathway irrespective of day length. Five Ppd-D1 alleles were found but only the 2kb deletion was associated with photoperiod insensitivity. Photoperiod insensitivity can also be conferred by mutation at a homoeologous locus on chromosome 2B (Ppd-B1). No candidate mutation was found in the 2B PRR gene but polymorphism within the 2B PRR gene cosegregated with the Ppd-B1 locus in a doubled haploid population, suggesting that insensitivity on 2B is due to a mutation outside the sequenced region or to a closely linked gene.]]>
[本文引用: 1]
[本文引用: 1]
DOI:10.1007/s00122-018-3177-4URLPMID:30267114 [本文引用: 1]

KEY MESSAGE: Two QTL with pleiotropic effects on plant height and spike length linked in coupling phase on chromosome 2DS were dissected, and diagnostic marker for each QTL was developed. Plant height (PHT) is a crucial trait related to plant architecture and yield potential, and dissection of its underlying genetic basis would help to improve the efficiency of designed breeding in wheat. Here, two quantitative trait loci (QTL) linked in coupling phase on the short arm of chromosome 2D with pleiotropic effects on PHT and spike length, QPht/Sl.cau-2D.1 and QPht/Sl.cau-2D.2, were separated and characterized. QPht/Sl.cau-2D.1 is a novel QTL located between SNP makers BS00022234_51 and BobWhite_rep_c63957_1472. QPht/Sl.cau-2D.2 is mapped between two SSR markers, SSR-2062 and Xgwm484, which are located on the same genomic interval as Rht8. Moreover, the diagnostic marker tightly linked with each QTL was developed for the haplotype analysis using diverse panels of wheat accessions. The frequency of the height-reduced allele of QPht/Sl.cau-2D.1 is much lower than that of QPht/Sl.cau-2D.2, suggesting that this novel QTL may be an attractive target for genetic improvement. Consistent with a previous study of Rht8, a significant difference in cell length was observed between the NILs of QPht/Sl.cau-2D.2. By contrast, there was no difference in cell length between NILs of QPht/Sl.cau-2D.1, indicating that the underlying molecular mechanism for these two QTL may be different. Collectively, these data provide a new example of QTL dissection, and the developed diagnostic markers will be useful in marker-assisted pyramiding of QPht/Sl.cau-2D.1 and/or QPht/Sl.cau-2D.2 with the other genes in wheat breeding.
DOI:10.1007/s00122-013-2194-6URLPMID:24061485 [本文引用: 1]

KEY MESSAGE: The effectiveness of wheat cultivar Liangxing 99 against powdery mildew was shown to be controlled by a single dominant gene located on a new locus of chromosome 2BL in the bin 2BL2-0.35-0.50. Liangxing 99, one of the most widely grown commercial cultivars in the winter wheat (Triticum aestivum) producing regions in northern China, was shown to provide a broad spectrum of resistance to Blumeria graminis f. sp. tritici (Bgt) isolates originating from that region. Using an F2 population and F2:3 lines derived from a cross of Liangxing 99 x Zhongzuo 9504, genetic analysis demonstrated that a single dominant gene, designated MlLX99, was responsible for the resistance of Liangxing 99 to Bgt isolate E09. The results of molecular analysis indicated that this gene is located on chromosome 2BL and flanked by the SSR marker Xgwm120 and EST-STS marker BE604758 at genetic distances of 2.9 and 5.5 cM, respectively. Since the flanking markers of MlLX99 were previously mapped to the bin 2BL2-0.36-0.50, MlLX99 must be located in this chromosomal region. MlLX99 showed a different resistance reaction pattern to 60 Bgt isolates from Pm6, Pm33, and PmJM22, which were all previously mapped on chromosome 2BL, but differed in their positions from MlLX99. Due to its unique position on chromosome 2BL, MlLX99 appears to be a new locus for resistance to powdery mildew. Liangxing 99 has shown superior yield performance and wide adaptation to different agricultural conditions, which has resulted in its extensive use as a wheat cultivar in China. The identification of resistance gene MlLX99 facilitates the use of this cultivar in the protection of wheat from damage caused by powdery mildew.
DOI:10.3724/SP.J.1006.2017.00332URL [本文引用: 1]

Pm52。利用来自不同小麦生产区的123个小麦白粉菌菌株进行抗性鉴定, 良星99可抗80%的菌株。2012-2016年连续5个生长季抗性鉴定中, 良星99在成株期对接种的白粉菌混合菌株都表现免疫或高抗。采用Pm52基因紧密连锁分子标记Xgwm120和27个菌株对10个利用良星99培育的品系进行分子检测和抗性分析发现, 衡4568、邯农2312、中信麦99和DH51302可能携带Pm52基因, 而郑麦369和冀麦729的白粉病抗性基因可能与Pm52不同。石U09-4366、XR4429、衡10-5039和农大3486苗期和成株期都表现感病, 不含Pm52基因。这些品种的成株期抗性反应与苗期的抗性反应一致。本研究的结果有利于良星99的抗白粉病基因Pm52在育种和生产上的有效利用。]]>
DOI:10.3724/SP.J.1006.2017.00332URL [本文引用: 1]

Pm52 on chromosome 2BL. A total of 123 isolates of Blumeria graminis f. sp. tritici collected from different wheat producing regions were used in assessment of disease resistance, and Liangxing 99 was resistant to 80% of them. During the five consecutive growing seasons from 2012 to 2016, Liangxing 99 was immune or highly resistant to the mixture of Bgt isolate inoculated at the adult plant stage. A gene-specific marker Xgwm120 closedly linked to Pm52 and 27 Bgt isolates were used to analyze ten wheat cultivars with Liangxing 99 as a parent. Heng 4568, Hannong 2312, Zhongxinmai 99, and DH51302 may inherit Pm52 from Liangxing 99, while the powdery mildew resistance genes of Zhengmai 369 and Jimai 729 may differ from Pm52. Shi U09-4366, XR4429, Heng 10-5039, and Nongda 3486 were susceptible to all the Bgt isolates examined and did not carry gene Pm52. The responses of these cultivars at the adult plant stage were consistent with those at the seedling stage. Results from this study are favorable to facilitate the effective application of Pm52 from Liangxing 99 in breeding programs and the production of wheat.]]>
DOI:10.1007/s00122-019-03291-7URLPMID:30719526 [本文引用: 2]

KEY MESSAGE: A high-resolution genetic linkage map was constructed using the comparative genomics analysis approach and the wheat reference genome, which placed wheat powdery mildew resistance gene Pm52 in a 0.21-cM genetic interval on chromosome arm 2BL. The gene Pm52 confers resistance to powdery mildew and has been previously mapped on chromosome arm 2BL in winter wheat cultivar Liangxing 99. Because of its effectiveness against the disease, this study was initiated to finely map Pm52 using the comparative genomics analysis approach and the wheat reference genomic sequence. Based on the EST sequences that were located in the chromosome region flanking Pm52, four EST-SSR markers were developed, and another nine SSR markers were developed using the comparative genomics technology. These thirteen markers were integrated into a genetic linkage map using an F2:3 subpopulation of the Liangxing 99 x Zhongzuo 9504 cross. Pm52 was mapped within a 3.2-cM genetic interval in the subpopulation that corresponded to a ~40-Mb genomic interval on chromosome arm 2BL of the Chinese Spring reference genome. The Pm52-flanking markers Xicsl163 and Xicsl62 identified 344 recombinant individuals from 8820 F2 plants. Nine SSR markers generated from the Chinese Spring genomic interval were incorporated into a high-resolution genetic linkage map, which placed Pm52 in a 0.21-cM genetic interval corresponding to 5.6-Mb genomic region. The constructed high-resolution genetic linkage map will facilitate the map-based cloning of Pm52 and its marker-assisted selection.
DOI:10.1016/j.gpb.2017.01.001URL [本文引用: 1]
DOI:10.1093/nar/gkz913URLPMID:31702008 [本文引用: 1]

The National Genomics Data Center (NGDC) provides a suite of database resources to support worldwide research activities in both academia and industry. With the rapid advancements in higher-throughput and lower-cost sequencing technologies and accordingly the huge volume of multi-omics data generated at exponential scales and rates, NGDC is continually expanding, updating and enriching its core database resources through big data integration and value-added curation. In the past year, efforts for update have been mainly devoted to BioProject, BioSample, GSA, GWH, GVM, NONCODE, LncBook, EWAS Atlas and IC4R. Newly released resources include three human genome databases (PGG.SNV, PGG.Han and CGVD), eLMSG, EWAS Data Hub, GWAS Atlas, iSheep and PADS Arsenal. In addition, four web services, namely, eGPS Cloud, BIG Search, BIG Submission and BIG SSO, have been significantly improved and enhanced. All of these resources along with their services are publicly accessible at https://bigd.big.ac.cn.
DOI:10.1093/gigascience/giaa060URLPMID:32501478 [本文引用: 1]

BACKGROUND: The cost of high-throughput sequencing is rapidly decreasing, allowing researchers to investigate genomic variations across hundreds or even thousands of samples in the post-genomic era. The management and exploration of these large-scale genomic variation data require programming skills. The public genotype querying databases of many species are usually centralized and implemented independently, making them difficult to update with new data over time. Currently, there is a lack of a widely used framework for setting up user-friendly web servers to explore new genomic variation data in diverse species. RESULTS: Here, we present SnpHub, a Shiny/R-based server framework for retrieving, analysing, and visualizing large-scale genomic variation data that can be easily set up on any Linux server. After a pre-building process based on the provided VCF files and genome annotation files, the local server allows users to interactively access single-nucleotide polymorphisms and small insertions/deletions with annotation information by locus or gene and to define sample sets through a web page. Users can freely analyse and visualize genomic variations in heatmaps, phylogenetic trees, haplotype networks, or geographical maps. Sample-specific sequences can be accessed as replaced by detected sequence variations. CONCLUSIONS: SnpHub can be applied to any species, and we build up a SnpHub portal website for wheat and its progenitors based on published data in recent studies. SnpHub and its tutorial are available at http://guoweilong.github.io/SnpHub/. The wheat-SnpHub-portal website can be accessed at http://wheat.cau.edu.cn/Wheat_SnpHub_Portal/.