

Surface Defect Detection of Navel Orange Based on Region Adaptive Brightness Correction Algorithm
ZHANG Ming1,2, WANG Teng2, LI Peng2, DENG Lie2, ZHENG YongQiang2, YI ShiLai2, Lü Qiang

通讯作者:
责任编辑: 杨鑫浩
收稿日期:2019-09-23网络出版日期:2020-06-16
基金资助: |
Received:2019-09-23Online:2020-06-16
作者简介 About authors
张明,E-mail:pine_torch@126.com。

摘要
关键词:
Abstract
Keywords:
PDF (2665KB)元数据多维度评价相关文章导出EndNote|Ris|Bibtex收藏本文
本文引用格式
张明, 王腾, 李鹏, 邓烈, 郑永强, 易时来, 吕强, 孙荣荣. 基于区域亮度自适应校正算法的脐橙表面缺陷检测[J]. 中国农业科学, 2020, 53(12): 2360-2370 doi:10.3864/j.issn.0578-1752.2020.12.005
ZHANG Ming, WANG Teng, LI Peng, DENG Lie, ZHENG YongQiang, YI ShiLai, Lü Qiang, SUN RongRong.
0 引言
【研究意义】柑橘是中国产量最大的水果之一,但由于采后检测、分级技术落后,造成上市后品质混杂、良莠不齐,其商品价值受到很大影响,特别是在国际市场上缺乏竞争力。当前国内对柑橘的采后分级方式主要还是依靠人工来完成,其结果受劳动者个体差异而有所差别,误差大,同时效率低。机器视觉技术的出现有效解决了上述问题且可同时对柑橘的多个指标进行检测、分级,包括大小、形状、颜色及表面缺陷等进行综合分级[1],其分级的客观性强、一致性好、效率高且具有无损、非接触等特点,是解决当前人工分级问题的有效途径之一。相较于水果的大小、形状、颜色等外在品质,水果表面缺陷一直是水果所有外在品质[2,3,4,5]检测中的难点,也是水果分级的重要依据之一。因此,对水果表面缺陷的快速检测研究具有十分重要的意义。【前人研究进展】早期文献报道中,对采集的图像直接采用阈值法分割缺陷,结果发现分割后图像边缘与背景、正常区域与缺陷区域存在“毛边”,而多阈值法又很容易在灰度值突变区域产生接缝等问题。近年来,基于机器视觉的脐橙表面缺陷检测技术受到了****们的关注。GóMEZ-SANCHIS等[6]通过假定脐橙为标准球体并以此提出一个朗伯表面模型,从水果曲面最高点到最低点对每个像素进行亮度校正,然而实际上水果是非标农产品,其大小、形状等信息很难预测。ZHANG等[2]利用平均值法对水果图像表面的环带区域灰度进行亮度校正,但当水果位置不在相机视场中心时,校正后的图像仍有亮度不均现象。NIPHADKAR等[7]通过图像形态学方法对溃疡脐橙果的高光谱图像边缘进行补偿来降低对缺陷的误检,但结果表明该方法效果不佳且该研究只选择了表面缺陷处较明显的溃疡脐橙果。LI等[8]基于光照-反射模型理论,通过构建巴特沃斯低通滤波器对脐橙表面亮度进行整体校正,但截止频率D0的首次确定需人工调校及该算法需要在时域与频域之间来回变换均影响了算法的性能。RONG等[9,10]提出一种基于积分图像的局部阈值快速检测脐橙表面缺陷的方法,缺陷检测率高,但该算法较为复杂且基于图像局部进行计算较为耗时。国内外****的研究表明,水果图像中部区域即使是缺陷,其灰度值通常也可能远高于图像边缘区域灰度值。分析后发现,水果一般呈类球体状,在光照反射时易造成水果表面亮度分布不均,表现为水果边缘区域灰度值低,中部区域灰度值高,而水果表面缺陷区域通常也是以灰度值较低的方式存在,即缺陷区域灰度值与正常区域存在交叉,给水果表面缺陷检测带来了困难[11,12]。通常对图像亮度分布不均的处理方法主要有直方图均衡化法[13,14](histogram equalization, HE)、基于Retinex理论算法[15,16]以及照度-反射理论算法[17]等。HE算法具有简单、运算量小等优点而被广泛用于图像处理中,尤其对图像整体偏亮或偏暗具有较好的处理效果,但对光照不均图像会出现过增强、失真[18]等问题;基于Retinex理论算法的原理是将一幅数字图像理解为光照分量与反射分量共同作用形成的,反射分量反映了图像的表面亮度信息,因此对反射分量进行增强就可以达到对图像表面亮度校正的目的,可有效增强图像暗处区域细节信息,但该算法过于复杂、容易在图像亮度突变区域产生光晕现象[19];照度-反射理论算法则是将图像分解为入射分量与反射分量,入射分量在空间域的变化较为缓慢,这一特征可以将图像的低频部分与照度相关联,将图像的低频部分从图像中提取出来,则此低频部分就代表了图像表面亮度信息,李江波[17]通过构建巴特沃斯低通滤波器,采用傅氏变换计算脐橙表面亮度信息,再对其校正即可得到脐橙表面亮度均一化图像,虽然取得了较好的校正效果,但部分数据由于校正过度致使数据溢出且该算法需要在时域与频域间来回变换,较为耗时。图像表面亮度分布不均导致图像中的一些重要细节信息无法表现出来甚至被掩盖,这不仅影响了图像的视觉效果,其应用价值也被削减,因此,消除或减弱物体表面亮度分布不均[20,21,22]问题日益成为研究的一个热点。【本研究切入点】类球形水果由于其自然形状属性原因,光照反射时易造成水果表面亮度分布不均匀,导致水果表面缺陷区域难以有效直接分割提取[23,24]。针对类球形水果表面缺陷区域难以有效直接分割问题,本文提出一种基于区域亮度自适应校正算法的水果表面缺陷快速检测方法。【拟解决的关键问题】本研究通过提取水果表面亮度信息并对其进行校正,达到快速检测水果表面缺陷的目的,以期为水果表面缺陷在线检测分级提供技术支持。1 材料与方法
1.1 试验设计
1.1.1 试验样本 本试验选择区域经济价值较高的纽荷尔脐橙为研究对象,产地为重庆云阳,2018年12月初采摘于当地商品化果园,共356个,经中国农业科学院柑桔研究所植物病理专家根据国家标准GB/T21488-2008脐橙、国家标准GB/T 12947-2008鲜柑橘和病理学相关知识对果皮状况进行鉴别,其中正常果64个、溃疡病果29个、蓟马虫果44个、介壳虫果37个、虫伤果38个、黑星病果35个、风伤果61个、炭疽病果26个、裂伤果22个。试验前用清水清洗样本,然后自然风干,以防止样本表面水的存在增加反光影响成像质量。1.1.2 图像采集系统 试验中,拍摄离线静态RGB图像的视觉系统硬件包括Lumenera公司生产的LU075C型彩色CCD工业相机、光照系统和计算机设备(Intel(R) Core(TM) i5-4590 CPU @3.3GHz,8 GB RAM)等。光照系统主要由光照箱、4条LED灯条(PHILIPS LS152S LED8 8.7W/M)、漫反射板等组成。光照箱长×宽×高尺寸分别为650 mm×650 mm× 600 mm,其内表面设置为白色,4条LED灯条均匀分布于光箱四周并用漫反射板遮挡,避免光源直射在脐橙表面形成亮斑。打开照明设备15 min,待光照强度趋于稳定后开始采集样本原始RGB图像。为了增强背景与脐橙样本间的颜色对比度,采用黑色背景[25]采集脐橙样本离线图像,镜头与放置样本载物台的垂直直线距离为300 mm,其采集的图像大小为640×480 pixels,利用文献[26]方法计算出采集的图像分辨率为0.25 mm/pixel。
1.2 果面缺陷检测流程与方法
本文算法基于MATLAB R2018b开发,通过对采集的原始可见光RGB图像去除背景,提取表面亮度信息并对其做亮度校正处理,最后结合单阈值法一次性提取脐橙表面缺陷区域。1.2.1 图像背景去除 相机获取的原始RGB图像包含目标脐橙本身和黑色背景,因此,首先进行图像背景去除提取脐橙目标区域[27]。去除背景可以排除将图像前景目标物误判为背景的可能,且在去除背景等冗余信息后,其检测速度也会提升。由于试验采集图像背景(黑色)基本一致,与样本颜色对比度较大,考虑采用阈值法[28]去除图像背景。根据其灰度直方图信息选择合适的阈值构建掩模图像并通过图像形态学填洞操作填充可能产生的孔洞,得到完整的掩模图像,最后将得到的掩模图像与原始图像做逻辑与运算,即可实现图像背景分割(从采集的样本图像中随机选择一幅带有缺陷的图像作为案例进行说明)。
1.2.2 图像亮度校正及缺陷提取 从文献[29,30]中发现由于水果表面亮度分布不均匀,表现为图像中部区域灰度值高,边缘灰度值低,难以采用单阈值法分割缺陷区域。因此,对其表面亮度校正尤为重要。
对水果图像表面亮度校正最终目的是为了改善其表面亮度分布不均问题,其难点在于要缩小图像边缘区域与中部区域间的灰度差,同时增大缺陷区域与正常区域间的对比度。本文在对比研究HE算法、基于Retinex理论算法以及基于照度-反射理论算法的亮度校正效果基础上,提出一种基于区域亮度自适应校正算法的水果表面缺陷快速检测方法。首先,计算出图像的背景亮度,再对其进行亮度均一化校正处理,最后结合全局单阈值法一次性完成对缺陷区域的提取。具体步骤如下:
(1)根据目标图像Iorange上的点在其w×w(w一般为奇数)邻域内较亮像素点的集合来估计目标图像Iorange的背景亮度。在大小为M×N的目标图像Iorange(试验中M和N的值为CCD相机采集的原始RGB图像大小,即640和480)上逐像素的滑动w×w窗口,通过矩阵变换,将目标图像Iorange每个像素邻域内的w2个像素排成一列,变换后的矩阵有M×N列,生成w2×MN的新矩阵;对此新矩阵按列从小到大排序,去除最后一行,即最亮像素点一行(减少或有白噪声像素点对背景亮度的影响),选取后5行按列做均值运算得到1×MN的矩阵,对此矩阵进行反变换、存储,最终得到和目标图像Iorange等大小的矩阵图像,即目标图像Iorange的表面亮度图像L(x, y)。
(2)采用亮度均一化校正对得到的表面亮度图像L(x, y)处理,根据公式(1)计算亮度均一化校正图像F(x, y)。
式中,F(x, y)为亮度均一化处理后的图像;Iorange(x, y)为目标图像;L(x, y)为目标图像表面亮度图像。
(3)根据获得的亮度均一化图像结合全局单阈值法,根据公式(2)计算获得目标缺陷二值化图像B(x, y),并对其进行图像形态学填洞处理(实现对连通区域的大面积缺陷区域的检测)。
式中,B(x, y)为目标缺陷二值化图像;T为全局单阈值。
(4)最后通过面积滤波法对获得的目标缺陷二值化图像B(x, y)进行杂散点及噪声去除,最终获得脐橙表面缺陷图像D(x, y)。
2 结果
2.1 背景去除
试验过程中发现,通过提取原始RGB图像中的R分量与B分量并做差值运算得到的分量融合图像Ir-b在灰度直方图上呈明显双峰分布,因此选择此分量构建掩模,根据灰度直方图信息选择合适的阈值T1(本研究T1=60)对Ir-b分量阈值分割并通过图像形态学填充分割后产生的孔洞,得到掩模图像Imask(图1-d)。尽管其他分量图像也可以将背景去除,但此分量融合图像Ir-b不仅能将图像中的背景去除,且能将目标前景凸显出来,拉大目标物表面正常区域与缺陷区域的对比度,有利于后续图像分析处理。将得到的掩模图像Imask与Ir-b分量图像做逻辑与运算,即可获得去除背景的目标脐橙图像Iorange,处理流程如图1所示。图1
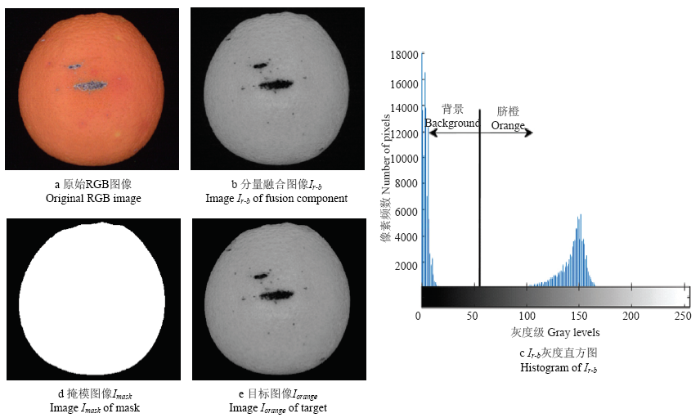
图1图像背景去除
Fig. 1Image background removal
2.2 图像亮度校正及单阈值缺陷分割
本文提出的自适应亮度校正算法通过邻域窗口内较亮像素点的集合计算出目标图像Iorange的表面亮度图像L(x, y),如图2-b所示;对得到的表面亮度图像L(x, y)根据公式(1)对其亮度均一化处理,结果如图2-c所示。图2
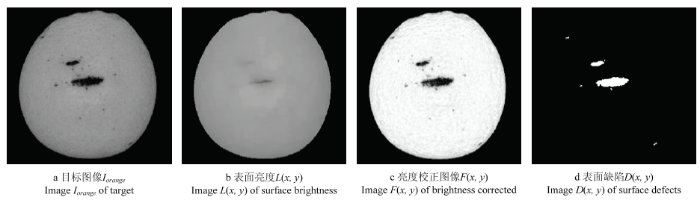
图2亮度校正及缺陷提取
Fig. 2Brightness correction and defect extraction
经亮度校正后的图像其表面缺陷区域与正常组织区域灰度对比度较大,可采用单阈值法直接对亮度校正后的脐橙图像F(x, y)进行缺陷分割,该分割阈值T通过对脐橙缺陷样本的亮度校正灰度图像分析比较,选定设置T=194;对缺陷分割后的二值化图像进行面积滤波以去除杂散点及噪声,如图2-d所示。
2.3 缺陷检测结果
为了验证本研究提出算法的适用性及鲁棒性,对溃疡病果、蓟马虫果、介壳虫果、虫伤果、黑星病果、风伤果、炭疽病果、裂伤果等共计8种脐橙病虫害缺陷果进行表面缺陷检测,图像中缺陷位置随机分布,校正后的图像表面缺陷区域与正常区域灰度差较明显,宜采用单阈值法直接对缺陷区域进行分割提取。部分样本缺陷分割结果如图3所示。图3
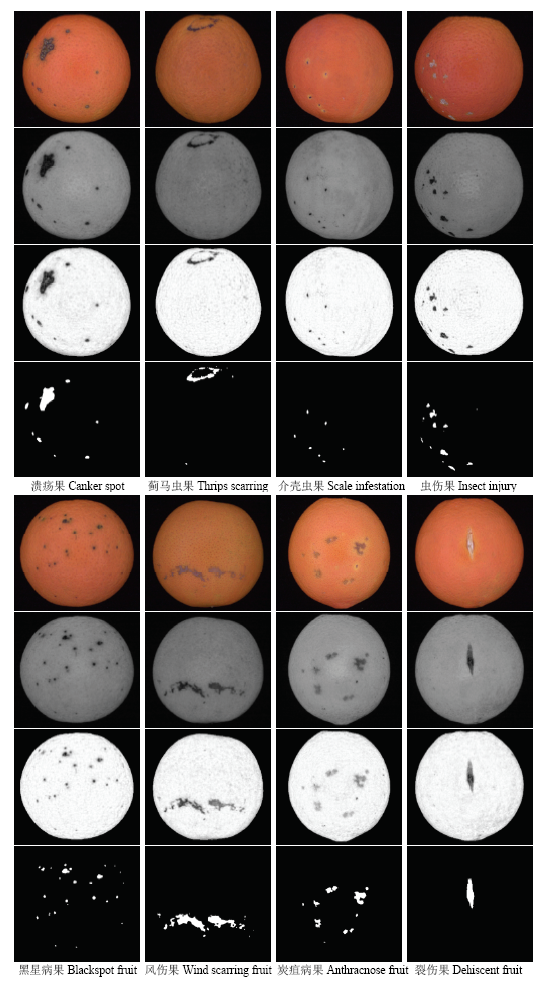
图3不同脐橙表面缺陷处理结果
原始RGB图像(第1、5行),R-B差值分量图像(第2、6行),亮度校正图像(第3、7行),缺陷图像(第4、8行)
Fig. 3Processing results of different navel oranges surface defects
Original RGB images (1st and 5th rows), R-B difference component image (2nd and 6th), brightness corrected images (3rd and 7th rows), and defect region images (4th and 8th rows)
对采集的共计356幅脐橙样本可见光RGB图像进行缺陷检测,其整体缺陷识别率达95.8%,结果如表1所示。
Table 1
表1
表1基于区域亮度自适应校正算法脐橙表面缺陷检测结果
Table 1
表皮类型 Epidermis type | 样本数 Number of samples | 正确识别样本数 Number of correctly recognized samples | 误判样本数 Number of misjudged samples | 准确率 Detection rate (%) | 误判率 False rate (%) |
---|---|---|---|---|---|
正常果Normal fruit | 64 | 59 | 5 | 92.2 | 7.8 |
溃疡病果Canker spot | 29 | 29 | 0 | 100.0 | 0.0 |
蓟马虫果Thrips scarring | 44 | 42 | 2 | 95.5 | 4.5 |
介壳虫果Scale infestation | 37 | 36 | 1 | 97.3 | 2.7 |
虫伤果Insect injury | 38 | 36 | 2 | 94.7 | 5.3 |
黑星病果Blackspot fruit | 35 | 34 | 1 | 97.1 | 2.9 |
风伤果Wind scarring fruit | 61 | 57 | 4 | 93.4 | 6.6 |
炭疽病果Anthracnose fruit | 26 | 26 | 0 | 100.0 | 0.0 |
裂伤果Dehiscent fruit | 22 | 22 | 0 | 100.0 | 0.0 |
合计Total | 356 | 341 | 15 | 95.8 | 4.2 |
新窗口打开|下载CSV
2.4 邻域窗口大小
试验中,本文算法邻域窗口大小参数w(即滑动窗口大小),其值直接影响检测速度和样本整体缺陷识别率。因此,对邻域窗口大小w的选取至关重要。分别取不同的w值,计算356幅样本图像平均每幅图像的耗时以及样本图像的整体缺陷识别率。结果表明,当w值较小时,即滑动窗口较小,算法运算速度较快,但与此同时算法对样本表面亮度校正的效果不理想,采用单阈值法分割缺陷时存在少分割、漏分割等问题;当w值较大时,即滑动窗口较大,此时样本的整体缺陷识别率有了较大提高,但同时算法处理过程较为耗时,难以满足在线检测需求。如表2所示,可以发现,随着w值的增大,尽管对样本图像表面缺陷的整体识别率在提高,但同时平均处理每幅图像耗时也在增加。综合考虑算法处理速度及对缺陷的识别率,确定本研究邻域窗口大小参数w为13,其平均处理每幅图像耗时0.29 s,样本图像表面缺陷识别准确率为95.8%。Table 2
表2
表2邻域窗口大小对算法运算速度与缺陷识别率的影响
Table 2
邻域窗口大小 w | 平均耗时 Average time consuming (s) | 识别率 Recognition rate (%) |
---|---|---|
7 | 0.11 | 92.1 |
9 | 0.16 | 92.9 |
11 | 0.21 | 93.5 |
13 | 0.29 | 95.8 |
15 | 0.45 | 95.9 |
17 | 0.72 | 96.1 |
新窗口打开|下载CSV
2.5 不同亮度校正算法性能评估
采用基于区域亮度自适应校正算法校正亮度不均匀的脐橙图像,校正效果直接影响缺陷区域的提取结果。研究同时采用了HE算法、基于Retinex理论算法以及基于照度-反射理论算法等亮度校正算法分别对目标图像Iorange进行校正(图4),并进行比较分析。经HE算法校正后的亮度图像出现图像失真,改变了图像的原始面貌,导致局部细节丢失,如图4-a;经Retinex算法校正后的亮度图像虽然改变了目标图像的亮度,但并没有达到使案例图像正常表面提亮,缺陷区域保持低灰度值的目的,反而在图像亮暗突变区域出现光晕现象,易导致误分割,如图4-b;经照度-反射理论算法校正的亮度图像表面亮度分布较均匀且边缘灰度也被提高,缺陷区域仍维持在低灰度值(图4-c),与李江波等[17]对脐橙表面亮度不均的处理结果一致,但处理后的图像明显存在过增强现象(截止频率D0=7);本研究提出的算法对图像表面亮度校正的效果如图4-d所示,较好地提升了脐橙整体表面亮度且整体亮度分布均匀,同时其缺陷区域依旧保持在低灰度值范围,有利于对缺陷区域的提取分割。图4
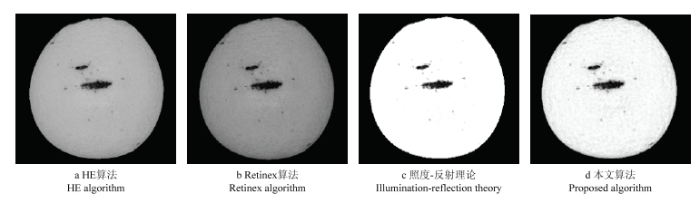
图4不同算法的亮度校正结果
Fig. 4Results of brightness correction based on different algorithms
利用灰度曲线穿过案例图像缺陷区域进一步验证各算法的有效性,分别从HE算法、基于Retinex理论算法、基于照度-反射理论算法以及本文算法得到的亮度校正图像中提取第235列(穿过缺陷区域)像素的灰度曲线(图5),图中灰度曲线中部低值部分为缺陷区域。从曲线中可以看出,照度-反射理论算法对图像亮度校正过度致使数据出现溢出,尤其是边缘区域,若缺陷位于边缘区域且颜色轻微则会被校正过度成高亮区域,从而误判为正常区域,导致漏分割;本文算法亮度校正结果显示,图像果皮正常区域无论是中部、还是边缘区域均被校正为高亮且两者灰度差波动范围很小,其灰度值在0.9—1.0之间附近波动,而缺陷区域依旧表现为低灰度,其灰度值在0—0.15之间,较HE算法(正常区域校正后的灰度值在0.7—0.8之间,缺陷区域校正后的灰度值在0—0.2之间)与Retinex算法(正常区域校正后的灰度值在0.55—0.65之间,缺陷区域校正后的灰度值在0—0.1之间)正常区域与缺陷区域灰度差更大,有利于对缺陷的提取。李江波等[31]通过对脐橙边缘亮度灰度进行补偿,在一定程度上避免了脐橙边缘亮度低,中部区域亮度高而导致的误分割现象,但边缘区域灰度突变与亮度不均匀的特征未变。本文所提算法对目标案例图像整体上取得了较好的亮度校正效果,尽管校正后的图像可能还存在少数噪声点,但并不影响图像整体的亮度校正效果。
图5
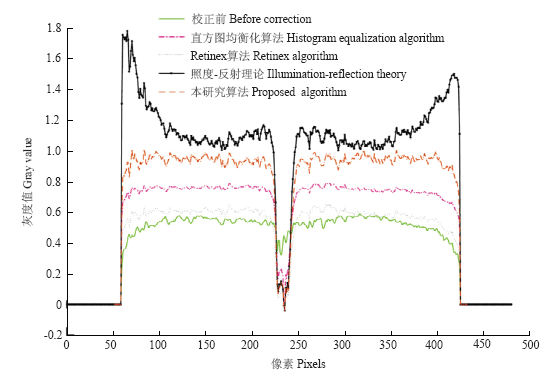
图5不同算法校正后图像第235列灰度曲线
Fig. 5Gray-scale curve of 235 columns of image corrected by various algorithms
然而,图5仅仅是从局部(一条像素灰度曲线)观察亮度校正效果,图6从脐橙整个表面强度能量观察本研究算法的亮度校正效果。图像中不同数值对应不同强度能量,从0到1,其强度能量等级随之增加。从图6-a中可以发现脐橙表面缺陷区域比正常区域的能量值低,边缘正常果皮区域的能量值也低于中心部位正常区域但接近于缺陷区域,因此,在单阈值分割时极易引起误分割。
图6
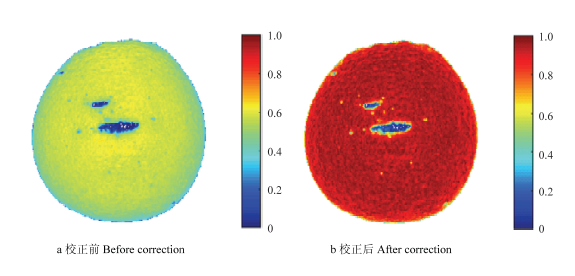
图6脐橙表面强度能量分布图
Fig. 6Intensity energy distribution of navel orange surface
与之相对照,在对脐橙表面亮度校正后,如图6-b所示,脐橙边缘能量等级得到提升,而缺陷区域依旧保持较低的能量等级,为单阈值法提取缺陷带来可能。
尽管本文算法是针对图像每个像素邻域进行计算的,但算法简单,运算速度较其他3种算法有大幅提高,对采集的全部正常果、8类缺陷果,共356幅脐橙样本图像进行亮度校正、缺陷检测,平均处理每幅图像仅耗时0.29 s,较基于其他3种亮度校正算法的缺陷检测方法,分别减少0.27 s、0.14 s和1.45 s;脐橙表面缺陷果的正确识别率达到了95.8%,对比其他3种算法,其缺陷果正确识别率提高了2.6%—8.2%,结果如表3所示。
Table 3
表3
表3各算法处理时间及样本表面缺陷识别率
Table 3
算法 Algorithm | 样本数 Number of samples | 识别样本数 Number of recognized samples | 缺陷识别率 Defect recognition rate (%) | 平均耗时 Average time consuming (s) |
---|---|---|---|---|
HE算法HE algorithm | 356 | 312 | 87.6 | 0.43 |
Retinex算法Retinex algorithm | 323 | 90.7 | 0.56 | |
照度-反射理论Illumination-reflection theory | 332 | 93.3 | 1.74 | |
本文算法Proposed algorithm | 341 | 95.8 | 0.29 |
新窗口打开|下载CSV
3 讨论
由表1可知,溃疡病果、炭疽病果、裂伤果的缺陷识别率为100%,是由于这类缺陷区域面积大,且经过亮度校正后与正常果皮区域的灰度差大,有利于缺陷区域分割;部分介壳虫果、蓟马虫果和黑星病果由于其缺陷区域面积小,在对其进行面积滤波去噪时而去除,调整面积滤波窗口,将原先3×3窗口大小改为2×2窗口大小,以免将小面积病害缺陷区域去除,将缺陷区域检测精度(单个最小检测面积)由0.56 mm2提高到0.25 mm2,远低于国家标准GB/T 21488-2008脐橙中规定容许的单个缺陷最大面积(0.30 cm2),对3种缺陷果识别率由之前的91.8%、93.1%和94.2%分别提高到97.2%、95.4%和97.1%;部分风伤果未能被有效检测到是由于这类缺陷颜色轻微,与正常果皮颜色相近且处于图像中央位置,即图像高亮区域,其灰度值较高,导致在单阈值分割下,尽管目标图像经过亮度校正,但其缺陷区域灰度值仍高于设定的分割阈值T,从而导致漏分割;部分虫伤果缺陷处颜色与正常区域颜色接近且缺陷面积较小从而导致无法检测到缺陷区域;而部分正常果被误判的主要原因是由于其部分边缘正常组织区域存在褶皱,其灰度值较低,易将其误分割为缺陷区域,进而误判为缺陷果,而人类视觉系统在鉴别脐橙表皮状况时会自动克服果皮褶皱组织区域的影响。黑星病果与风伤果的缺陷区域分布在整个果面(同一种缺陷处于不同位置),包括中央位置(偏亮区域)和边缘区域(偏暗区域),当缺陷处于偏亮区域时,可能会造成少分割现象;当缺陷区域位于偏暗区域时,可能会造成漏分割现象;而提出的算法仍能较好地将缺陷区域分割出来,进一步验证了算法的有效性。
研究针对同一年度(2018年)、同一产地(重庆云阳)、同一品种(纽荷尔脐橙),且达到采收或上市要求的果实,其表面色泽、成熟度基本一致,本文算法检测缺陷果识别率达95.8%。但由于不同产区、不同年度、不同品种的成熟脐橙果实之间存在一定的色泽差异,本文选定参数(如背景去除分割阈值、缺陷区域分割阈值等)可能会对算法检测结果造成一定影响。
通常一条果实品质分级线的覆盖产地相对较小。在该特定区域(产地)内,同一年度、同一品种的成熟果实,表面色泽较为一致;对不同年度、不同品种的脐橙果实,可通过微调参数进行果实缺陷区域检测。对跨产地、跨年度、跨品种的成熟脐橙果实混合样本缺陷区域检测对算法鲁棒性提出了较高的要求,下一步将针对此类情况开展检测算法参数优化和算法鲁棒性研究。
4 结论
为解决类球形水果表面亮度分布不均导致的单阈值法难以有效分割缺陷区域问题,提出一种基于区域亮度自适应校正算法的脐橙表面缺陷快速检测方法。该算法对溃疡病果、蓟马虫果、介壳虫果、虫伤果、黑星病果、风伤果、炭疽病果、裂伤果等8种常见的脐橙表面缺陷的准确识别率达到了95.8%,平均处理每幅图像耗时0.29 s。本研究算法克服了大小、形状不规则的脐橙类水果表面亮度分布不均导致的缺陷难分割问题,为脐橙精确分级提供了技术支持,也为其他类球形果品表面缺陷快速检测提供了一种新的解决思路。
参考文献 原文顺序
文献年度倒序
文中引用次数倒序
被引期刊影响因子
[D].
[本文引用: 1]
[D].
[本文引用: 1]
DOI:10.1016/j.jfoodeng.2014.08.024URL [本文引用: 2]
DOI:10.1080/10942912.2015.1020439URL [本文引用: 1]
DOI:10.1016/j.compag.2010.02.001URL [本文引用: 1]
[本文引用: 1]
[本文引用: 1]
DOI:10.1016/j.jfoodeng.2007.06.036URL [本文引用: 1]
[本文引用: 1]
DOI:10.1016/j.postharvbio.2013.02.016URL [本文引用: 1]

Automatic detection of fruit peel defects by a computer vision system is difficult due to the challenges of acquiring images from the surface of spherical fruit and the visual similarity between the stem-ends and the true defects. In this study, oranges with wind scarring, thrips scarring, scale infestation, dehiscent fruit, anthracnose, copper burn, canker spot and normal surface were researched. A lighting transform method based on a low pass Butterworth filter with a cutoff frequency D-0 = 7 was first developed to convert the non-uniform intensity distribution on spherical oranges into a uniform intensity distribution over the whole fruit surface. However, the stem-ends were easily confused with defective areas. In order to solve this problem, different color components (R, G and B) and their combinations were analyzed. It was found that a ratio method and Rand G component combination coupled with a big area and elongated region removal algorithm (BER) could be used to differentiate stem-ends from defects effectively. Finally, a processing and classification algorithm based on a simple thresholding method was proposed. The result with 98.9% overall detection rate for the 720 independent sample images indicated that the proposed algorithm was effective in differentiation of normal and defective oranges. The method, however, could not discriminate between different types of defects. (C) 2013 Elsevier B.V.
[本文引用: 1]
[本文引用: 1]
[本文引用: 1]
[本文引用: 1]
[本文引用: 1]
DOI:10.1109/TCE.2010.5505998URL [本文引用: 1]
DOI:10.1049/el.2011.3740URL [本文引用: 1]

The core and winding of an inductor are designed to distribute flux for distributing energy uniformly in the core to utilise magnetic material throughout the core volume. The structure is designed to avoid crowding of flux for low core loss. The winding is patterned for shorter length and larger area of the cross-section for low winding loss. The footprint area of a three-cell inductor is 1.48 times less than that of the conventional toroidal inductor with same energy, maximum flux density, material properties, winding loss, and core loss.
DOI:10.1016/j.ijleo.2013.04.115URL [本文引用: 1]
DOI:10.1016/j.patrec.2013.02.017URL [本文引用: 1]
URL [本文引用: 3]

The presence of skin defects is one of the main influential factors on the price of fruit. Most of the researches used static images and more complex algorithm to segment defects on fruit surface. The lighting correction approach and threshold method was proposed to segment fruit surface defects. The fruit images with defects were acquired from an on-line sorting system. Firstly, hue (H) component was obtained by transforming RGB colour space to HIS colour space. The mask was created based on H component and used to remove R component image background. Then, based on illumination-reflectance model, the illumination component was extracted from R component image by low pass filter. The illumination component was used to correct illumination on R-component image. Finally, defects were successfully segmented at one time by subjecting the corrected image to a single threshold value. The experimental results with an over 99% recognition rate for 416 images showed that the proposed algorithm was simple and effective for application in real-time detection of defects on fruit.
URL [本文引用: 3]

The presence of skin defects is one of the main influential factors on the price of fruit. Most of the researches used static images and more complex algorithm to segment defects on fruit surface. The lighting correction approach and threshold method was proposed to segment fruit surface defects. The fruit images with defects were acquired from an on-line sorting system. Firstly, hue (H) component was obtained by transforming RGB colour space to HIS colour space. The mask was created based on H component and used to remove R component image background. Then, based on illumination-reflectance model, the illumination component was extracted from R component image by low pass filter. The illumination component was used to correct illumination on R-component image. Finally, defects were successfully segmented at one time by subjecting the corrected image to a single threshold value. The experimental results with an over 99% recognition rate for 416 images showed that the proposed algorithm was simple and effective for application in real-time detection of defects on fruit.
DOI:10.1109/TIP.2012.2202670URL [本文引用: 1]

Illumination compensation and normalization play a crucial role in face recognition. The existing algorithms either compensated low-frequency illumination, or captured high-frequency edges. However, the orientations of edges were not well exploited. In this paper, we propose the orientated local histogram equalization (OLHE) in brief, which compensates illumination while encoding rich information on the edge orientations. We claim that edge orientation is useful for face recognition. Three OLHE feature combination schemes were proposed for face recognition: 1) encoded most edge orientations; 2) more compact with good edge-preserving capability; and 3) performed exceptionally well when extreme lighting conditions occurred. The proposed algorithm yielded state-of-the-art performance on AR, CMU PIE, and extended Yale B using standard protocols. We further evaluated the average performance of the proposed algorithm when the images lighted differently were observed, and the proposed algorithm yielded the promising results.
DOI:10.1016/j.neucom.2013.01.038URL [本文引用: 1]
DOI:10.1109/TIP.2013.2261309URL [本文引用: 1]

Image enhancement plays an important role in image processing and analysis. Among various enhancement algorithms, Retinex-based algorithms can efficiently enhance details and have been widely adopted. Since Retinex-based algorithms regard illumination removal as a default preference and fail to limit the range of reflectance, the naturalness of non-uniform illumination images cannot be effectively preserved. However, naturalness is essential for image enhancement to achieve pleasing perceptual quality. In order to preserve naturalness while enhancing details, we propose an enhancement algorithm for non-uniform illumination images. In general, this paper makes the following three major contributions. First, a lightness-order-error measure is proposed to access naturalness preservation objectively. Second, a bright-pass filter is proposed to decompose an image into reflectance and illumination, which, respectively, determine the details and the naturalness of the image. Third, we propose a bi-log transformation, which is utilized to map the illumination to make a balance between details and naturalness. Experimental results demonstrate that the proposed algorithm can not only enhance the details but also preserve the naturalness for non-uniform illumination images.
[本文引用: 1]
[本文引用: 1]
[本文引用: 1]
[本文引用: 1]
[D].
[本文引用: 1]
[D].
[本文引用: 1]
[本文引用: 1]
[本文引用: 1]
[D].
[本文引用: 1]
[D].
[本文引用: 1]
[D].
[本文引用: 1]
[D].
[本文引用: 1]
DOI:10.1007/s11760-014-0718-4URL [本文引用: 1]
[本文引用: 1]
[本文引用: 1]
[本文引用: 1]
[本文引用: 1]
DOI:10.3864/j.issn.0578-1752.2019.02.011URL [本文引用: 1]

【Objective】 The purpose of this study was to effectively solve the problem that some defects of fruit images with defective peels were mistakenly divided into backgrounds when removing background, and it was difficult to effectively segment and extract fruit surface defects.【Method】 Taking Newhall navel orange as the research object, this paper proposed to remove the background based on HSI color space model method to construct the mask template with I component image, and to select a single threshold (T=75) by bimodal method according to its gray histogram information and filled the holes to obtain a mask template. At last, the mask template and I component image were obtained by dot multiplication to obtain I component image from which the background was removed. A multi-scale Gaussian function image brightness correction algorithm was proposed to correct the brightness of I component image after removing the background. By constructing a multi-scale Gaussian function filter, I component image with the background removed and the constructed multi-scale Gaussian function filter were convoluted to obtain the surface illumination component image of I component image after the background was removed. Finally, the I component image after removing the background and the obtained illumination component image were subjected to dot division operation to obtain a luminance correction image of the I component image after the background was removed. At last, the surface defects of navel orange were extracted by a single global threshold method.【Result】 The background was removed based on the HSI color space model method, and the surface information of the navel orange could be preserved while the background was effectively removed, which was beneficial to subsequent operations. The image brightness correction algorithm based on multi-scale Gaussian function was used to extract the defects of the six common navel orange defects, and then the single-threshold method was used to extract the defects. Therefore the surface defects of navel oranges with different gray levels were successfully segmented at one time, and the segmentation rate was up to 100%, the lowest was 88.5%, and the total was 92.7%. Through experimental analysis, it was found that the cause of partial mis-segmentation or leakage segmentation was mainly due to the fact that some defects were lighter in color, and the difference in gray level from normal region was smaller, resulting in leakage segmentation. There were still some defects due to the small defect area, which was mistaken for noise removal during image morphology processing. At the same time, the false positive rate of normal fruit was also found to be 10.8%. It was found that the fold of a part of the normal fruit epidermal tissue area was located in the edge area of the image, which was mistaken for the defect of the edge area, resulting in misjudgment.【Conclusion】 The experimental results showed that image removal based on HSI color space model and image brightness unevenness correction algorithm based on multi-scale Gaussian function had achieved good results for background image segmentation of Newhall navel orange image and I component image surface brightness correction after background removal. It provided technical support for the precise grading of navel oranges and also provided a new idea for the rapid detection of other fruit surface defects.
DOI:10.3864/j.issn.0578-1752.2019.02.011URL [本文引用: 1]

【Objective】 The purpose of this study was to effectively solve the problem that some defects of fruit images with defective peels were mistakenly divided into backgrounds when removing background, and it was difficult to effectively segment and extract fruit surface defects.【Method】 Taking Newhall navel orange as the research object, this paper proposed to remove the background based on HSI color space model method to construct the mask template with I component image, and to select a single threshold (T=75) by bimodal method according to its gray histogram information and filled the holes to obtain a mask template. At last, the mask template and I component image were obtained by dot multiplication to obtain I component image from which the background was removed. A multi-scale Gaussian function image brightness correction algorithm was proposed to correct the brightness of I component image after removing the background. By constructing a multi-scale Gaussian function filter, I component image with the background removed and the constructed multi-scale Gaussian function filter were convoluted to obtain the surface illumination component image of I component image after the background was removed. Finally, the I component image after removing the background and the obtained illumination component image were subjected to dot division operation to obtain a luminance correction image of the I component image after the background was removed. At last, the surface defects of navel orange were extracted by a single global threshold method.【Result】 The background was removed based on the HSI color space model method, and the surface information of the navel orange could be preserved while the background was effectively removed, which was beneficial to subsequent operations. The image brightness correction algorithm based on multi-scale Gaussian function was used to extract the defects of the six common navel orange defects, and then the single-threshold method was used to extract the defects. Therefore the surface defects of navel oranges with different gray levels were successfully segmented at one time, and the segmentation rate was up to 100%, the lowest was 88.5%, and the total was 92.7%. Through experimental analysis, it was found that the cause of partial mis-segmentation or leakage segmentation was mainly due to the fact that some defects were lighter in color, and the difference in gray level from normal region was smaller, resulting in leakage segmentation. There were still some defects due to the small defect area, which was mistaken for noise removal during image morphology processing. At the same time, the false positive rate of normal fruit was also found to be 10.8%. It was found that the fold of a part of the normal fruit epidermal tissue area was located in the edge area of the image, which was mistaken for the defect of the edge area, resulting in misjudgment.【Conclusion】 The experimental results showed that image removal based on HSI color space model and image brightness unevenness correction algorithm based on multi-scale Gaussian function had achieved good results for background image segmentation of Newhall navel orange image and I component image surface brightness correction after background removal. It provided technical support for the precise grading of navel oranges and also provided a new idea for the rapid detection of other fruit surface defects.
URL [本文引用: 1]

Detection of fruits surface defects is always a challenging project for automated fruit grading of computer vision. A new method was developed to solve the problem of a part of defects easily being mistaken for the background when the fruits with defects were segmented from the background. First the B-component image of navel orange was extracted and built mask, then R-component image was masked by B-component image, thus 100% fruits and background segmentation with intact defects was achieved. Considering false segmentation of defects owing to the lower edge gray values of spherical fruits, an algorithm of fast fruit image edge gray value compensation was advanced. Using this algorithm, six kinds of common defects of navel orange, for a total of 220 images, setting the threshold for 165, the defects of different gray value was successfully segmented at one time. The highest segmentation rate was 100% and the lowest was 79.5%. Test results showed that the segmentation efficiency of the defects was improved as a result of the use of a single threshold.
URL [本文引用: 1]

Detection of fruits surface defects is always a challenging project for automated fruit grading of computer vision. A new method was developed to solve the problem of a part of defects easily being mistaken for the background when the fruits with defects were segmented from the background. First the B-component image of navel orange was extracted and built mask, then R-component image was masked by B-component image, thus 100% fruits and background segmentation with intact defects was achieved. Considering false segmentation of defects owing to the lower edge gray values of spherical fruits, an algorithm of fast fruit image edge gray value compensation was advanced. Using this algorithm, six kinds of common defects of navel orange, for a total of 220 images, setting the threshold for 165, the defects of different gray value was successfully segmented at one time. The highest segmentation rate was 100% and the lowest was 79.5%. Test results showed that the segmentation efficiency of the defects was improved as a result of the use of a single threshold.