本文提出了频域空间、能量最小化的目标跟踪方法,提出了精准的稠密循环采样方法,解决计算过程中的精确输入问题;提出了循环矩阵的概念,极大的简化了方法的计算过程;提出了频域空间的能量最小化方法,解决对跟踪过程中目标特征的精确提取问题。
1 相关工作 目标跟踪是一个很早就确立了的计算机视觉问题[1-2],也是一个流行的视觉研究核心问题之一,其研究方法可以分为2类:判别式方法和生成性方法。判别式方法将跟踪问题归纳为一个分类问题,并且建立一个分类器从背景中分离目标。代表性的判别式方法是Ensemble跟踪、在线的boosting跟踪器[3]、多实例学习[4]和结构化的支持向量机[5]。生成性方法主要是集中建模目标的外观,如基于直方图的跟踪器、子空间表达跟踪器[6]、WSL外观跟踪器和稀疏表达跟踪器[7]。
跟踪的关键问题之一是目标的表达,如颜色直方图、外观表达[8-9]和判别模型[5, 10]等。运动估计是另一个核心的视觉跟踪问题,运动估计的常用方法是粒子滤波器[10]。该方法的特点是用一系列的粒子权重表达概率密度,通过重要的位置采样和贝叶斯推理来跟踪目标,为了获得更好的结果,大量的粒子需要采样,这样就带来了计算的负担。另一个运动估计方法是Mean shift方法,该方法只是估计目标的位置信息,并没有估计出目标的朝向和模式信息,这极大地限制了该方法的应用范围。
近年来基于形状和语法先验的稠密静态目标的跟踪方法[11]得到了进一步的改进,比如PWP(Pixel-Wise Posteriors)[12]方法,这个方法可以用于视频序列的实时目标的跟踪,但是在离线跟踪方面效果不理想。另外,Struck[5]是综合测试后比较好的跟踪方法,但是当目标的视点发生偏移的时候,这种方法就会跟踪失败。文献[13-14]提出了基于目标关键点的跟踪方法,用SIFT或者SURF描述表达相关区域,但是这种定位方法也存在着目标的丢失问题。文献[15]提出的TLD(Tracking-Learning-Detection)方法将跟踪任务分成了几个同时实现的步骤来完成,如学习和检测,但是这种方法不能处理目标尺度的改变,当目标的尺度发生了严重变化的情况下,TLD会跟踪失败。
2 方法概述 在第1帧中就对目标进行检测并进行建模,这对目标的检测将起到至关重要的作用。随着视点的改变,本文方法检测目标的不同方面并对模型进行再次精确更新。如果没有发生遮挡,依旧更新模型。跟踪目标的同时需要知道目标是不是发生变形或者是被遮挡,如果只有40%在包围盒内部,那么判断目标发生了遮挡。针对视频跟踪目标的不稳定性和背景的复杂多变性,本文方法将用消除抖动、高级降噪、增强亮度和对比度、去除马赛克、增强细节、增加色调和饱和度等方面去解决问题。
很多方法都是基于目标运动轨迹是光滑的、运动是匀速的、外观是不再继续变化的这样一个假设。在这里会涉及到一个目标的表示问题,如文献[16]将目标表示为一个点,文献[17]将目标表示为一个椭圆。然而,跟踪的目标在跟踪过程中会发生外观、形状和光照明暗程度等变化,针对这种情况人们根据直方图等建立了代价函数,来表达目标和模板的相似程度。运动轨迹决定了检测器的搜索区域c,也决定了目标是不是发生旋转和偏移等信息。本文的方法流程如图 1所示。
![]() |
图 1 本文方法框架 Fig. 1 Architecture of proposed method |
图选项 |
3 精准采样 3.1 稠密的滑动窗口 本文方法进行的是以开始检测到的目标为主并且不断学习、精简目标的采样方式,而目前流行的方法进行的是关键帧的随机采样。本文方法从连续的10帧视频图像中,提取特征,检测目标的模型。本文及经典方法的采样方式如图 2所示。
![]() |
图 2 本文方法和经典方法采样方式的对比 Fig. 2 Comparison of sampling mode between proposed method and classic method |
图选项 |
3.2 相关区域和核心区域 本文方法首先根据目标在前一帧的估计位置,将目标搜索区域分为目标区域和背景区域,在目标区域再分为相关区域和核心区域;其次在区域划分的基础上定义了一种新的颜色和运动特征,这种特征能有效抑制相邻背景的混乱干扰,这种融合了颜色和运动特征的跟踪方法在跟踪过程中是互补的,从而提高了方法跟踪的准确性。本文方法的相关区域和核心区域如图 3所示,目标区域每一帧更新一次,由于方法的相关性约束,每一帧中只有少数的相关性区域是被收集的,以便减少计算的数据量。
![]() |
图 3 相关采样区域和核心采样区域 Fig. 3 Related area and core area of sampling |
图选项 |
3.3 循环矩阵 通过一个n×1的向量u,可以得到一个酉空间的循环矩阵C(u),矩阵的第1行是向量u,第2行是第1行向左移动一个元素,依次类推。C(u)v为u和v的卷积,C(u)v=F-1(F*(u)·F(v)),其中F*(u)·F(v)表示按元素的点积,F和F-1分别为傅里叶变换和逆变换。图像是作为矩阵中的一个元素来存储的,对图像的一次遍历就是对矩阵的一次搜索。循环矩阵的特征使得它很适合用作目标跟踪的计算,因为循环矩阵的和矩阵、乘积矩阵、逆矩阵都是循环矩阵。其原理如图 4所示。
![]() |
图 4 循环矩阵的代数模型和循环采样过程 Fig. 4 Algebra model of circulation matrix and circulation sampling process |
图选项 |
![]() | (1) |
式中:X为测试数据的变换结果;U(x)为循环矩阵,x为像素;xn为循环矩阵中的元素。
4 频域空间运算的定义 本文方法定义一套频率域的计算法则,将图像时空域内的时序信号转变为频域信号,在频率空间内定义线性核、多项式核和点积核的运算形式,如下:
![]() | (2) |
式中:x、

频域的计算结果如图 5所示。
![]() |
图 5 频域的计算结果 Fig. 5 Calculation results of frequency domain |
图选项 |
5 精准跟踪 给定标签:(x1, y1), (x2, y2), …, (xn, yn)和分类器f(x),其中f(x)=(w, x)+b,(w, x)表示w与x的点积,则这个最小化的问题变为
![]() | (3) |
式中:Z为误差函数;f(xi)为傅里叶域内的模型函数;yi为回归目标;L(yi, f(xi))为损失函数;λ为控制过度拟合的正则化参数;θ为损失参数。要求式(3)的最小值,就要使每一项取得最小值,即
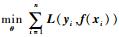

6 实验 评价本文方法好坏的标准为中心位置错误率和平均包围盒覆盖率(AOR),其中中心位置错误率定义为
![]() | (4) |
式中:a为本文方法的跟踪结果;b为数据集标准结果。平均包围盒覆盖率定义为
![]() | (5) |
式中:area为包围盒面积;RT为计算的面积;RGT为标准值的面积。
中心位置错误率和平均包围盒覆盖率如图 6所示,重叠面积CtG∩Ct0为数据集标准结果与本文计算结果的交集。
![]() |
图 6 中心位置错误率和平均包围盒覆盖率示意图 Fig. 6 Schematic of center position error rate and average bounding box error rate |
图选项 |
第1组实验:本文方法在最近发布的目标跟踪标准数据集[2]上与目前常见的方法进行了比较,如IVT[6]、SPLTT[7]、ASLA[16]、OAB[15]、SMS[17]、Frag[18]、SSB[18]、MIL[19]、VTD[20]、LIT[21]、TLD[22]、DF[23]、MTT[24]、Struck[5]、ConT[25]、MOS[26]、CT[27]、CST[28]、LGT[29]、L1APG[30]。这组目标跟踪标准数据集被标注为12个不同的特征,即场景光照变化、目标尺度模式变化、目标部分遮挡、目标变形、运动模糊、快速运动、平面内旋转、平面外旋转、视点变化、复杂背景、目标朝向变化和低分辨率等情形。为了估计本文方法和其他方法的精度和效率,从不同挑战性的跟踪标准数据集中选取了12个代表性的序列如表 1所示。测试结果见表 2。
表 1 标准数据集中选取的主要挑战序列 Table 1 Main challenge sequence selected from standard database
主要挑战序列 | 视频序列 |
场景光照变化 | Car4 |
目标尺度模式变化 | Walking2 |
目标部分遮挡 | Singer1 |
目标变形 | Faceocc2 |
运动模糊 | Caviar |
快速运动 | David2 |
平面内旋转 | CarDark |
平面外旋转 | Woman |
视点变化 | Singer1 |
目标朝向变化 | Dudek |
复杂背景 | David |
低分辨率 | Faceocc2 |
表选项
表 2 目标跟踪标准数据集中各挑战序列和相关的跟踪结果 Table 2 Each challenge sequence and relative tracking results in standard target tracking database
主要挑战序列 | 跟踪效果 |
场景光照变化 | ![]() |
快速运动 | ![]() |
目标朝向变化 | ![]() |
目标尺度模式变化 | ![]() |
视频抖动 | ![]() |
目标部分遮挡 | ![]() |
相似背景干扰 | ![]() |
其他情形 | ![]() |
表选项
第2组实验:在另一组最新的目标跟踪标准数据集上进行了测试,并跟数据集标准结果进行了精度和成功率的比较。同时与一些在线的跟踪方法进行了比较,如IVT[6]、Frag[18]、TLD[22]、MTT[24]、Struck[5]、L1APG[30]、LSK[31]、MIL[32]、DFT[33]、LSK[34]。也与一些离线的跟踪方法如OMA[35]和ASLA[36]等进行了比较。其中中心位置错误率如表 3所示。本文方法的平均包围盒覆盖率测试结果见表 4。本文方法的精度、鲁棒性、平均重叠率测试结果如表 5所示。表中数值格式表征算法结果的好坏,加粗数值表示测试结果中最好的,加下划线的数值表示结果在测试结果中排名第二的。
表 3 中心位置错误率测试结果 Table 3 Test results of center position error rate
% | |||||||||||||
测试数据集 | 本文方法 | IVT | Frag | TLD | MTT | Struck | L1APG | LSK | MIL | DFT | LSK | OMA | ASLA |
Basketball | 2.11 | 4.5 | 12.11 | 22.99 | 3.21 | 3.85 | 3.24 | 11.32 | 10.23 | 1.97 | 3.55 | 11.58 | 1.88 |
Car4 | 1.17 | 1.56 | 23 | 31.21 | 1.54 | 10.46 | 1.38 | 5.64 | 9.35 | 1.45 | 2.56 | 7.68 | 1.18 |
Singer | 3.5 | 3.65 | 38.46 | 38.41 | 9.87 | 9.62 | 65.23 | 24.36 | 15.34 | 27.36 | 9.65 | 12.54 | 4.23 |
Dudek | 6.24 | 11.32 | 87.99 | 31.87 | 17.82 | 18.32 | 6.91 | 17.35 | 9.65 | 11.27 | 11.89 | 17.65 | 8.75 |
Faceocc2 | 5.22 | 7.23 | 15.19 | 18 | 6.03 | 6.55 | 9.35 | 16.89 | 12.36 | 5.99 | 17.86 | 15.28 | 22.75 |
CarDark | 3.81 | 12.68 | 29 | 10.77 | 14.32 | 13.78 | 4.6 | 14.19 | 8.34 | 5.67 | 15.25 | 9.49 | 3.86 |
David | 3.65 | 71.45 | 19.66 | 63.8 | 65.09 | 66.06 | 66.87 | 14.57 | 5.38 | 62.9 | 65.85 | 6.29 | 3.67 |
Caviar | 3.37 | 138.65 | 113.26 | 59.27 | 3.38 | 3.56 | 123.65 | 34.75 | 35.62 | 134.61 | 55.21 | 65.32 | 146.32 |
Woman | 2.56 | 1.87 | 4.62 | 2.54 | 2.94 | 2.99 | 4.65 | 6.34 | 6.54 | 46.66 | 5.32 | 4.59 | 1.65 |
MotorRolling | 3.15 | 2.96 | 61.23 | 60.66 | 11.99 | 11.98 | 3.52 | 3.69 | 56.32 | 2.1 | 12.34 | 10.63 | 3.16 |
Shaking | 18.88 | 45.32 | 35.21 | 21.45 | 18.87 | 18.89 | 41.32 | 17.54 | 18.65 | 41.36 | 25.34 | 19.65 | 44.8 |
表选项
表 4 平均包围盒覆盖率测试结果 Table 4 Test results of average bounding box error rate
% | |||||||||||||
测试数据集 | 本文方法 | IVT | Frag | TLD | MTT | Struck | L1APG | LSK | MIL | DFT | LSK | OMA | ASLA |
Basketball | 0.89 | 0.78 | 0.46 | 0.62 | 0.98 | 0.48 | 0.78 | 0.67 | 0.81 | 0.68 | 0.59 | 0.59 | 0.84 |
Car4 | 0.87 | 0.85 | 0.18 | 0.36 | 0.85 | 0.87 | 0.85 | 0.89 | 0.83 | 0.69 | 0.59 | 0.71 | 0.83 |
Singer | 0.79 | 0.69 | 0.27 | 0.49 | 0.35 | 0.56 | 0.27 | 0.76 | 0.68 | 0.95 | 0.35 | 0.68 | 0.68 |
Dudek | 0.91 | 0.78 | 0.48 | 0.67 | 0.72 | 0.66 | 0.78 | 0.67 | 0.69 | 0.58 | 0.68 | 0.8 | 0.75 |
Faceocc2 | 0.92 | 0.74 | 0.62 | 0.59 | 0.87 | 0.67 | 0.69 | 0.38 | 0.65 | 0.67 | 0.67 | 0.76 | 0.56 |
CarDark | 0.79 | 0.49 | 0.34 | 0.67 | 0.46 | 0.39 | 0.78 | 0.67 | 0.57 | 0.37 | 0.65 | 0.59 | 0.79 |
David | 0.93 | 0.26 | 0.46 | 0.18 | 0.23 | 0.24 | 0.27 | 0.68 | 0.69 | 0.59 | 0.58 | 0.68 | 0.85 |
Caviar | 0.86 | 0.2 | 0.27 | 0.26 | 0.19 | 0.77 | 0.19 | 0.69 | 0.76 | 0.49 | 0.32 | 0.67 | 0.16 |
Woman | 0.87 | 0.74 | 0.67 | 0.69 | 0.33 | 0.71 | 0.67 | 0.38 | 0.34 | 0.69 | 0.68 | 0.67 | 0.79 |
MotorRolling | 0.85 | 0.73 | 0.28 | 0.27 | 0.89 | 0.51 | 0.76 | 0.59 | 0.59 | 0.69 | 0.68 | 0.67 | 0.85 |
Shaking | 0.83 | 0.24 | 0.19 | 0.26 | 0.24 | 0.53 | 0.29 | 0.68 | 0.78 | 0.67 | 0.84 | 0.69 | 0.23 |
表选项
表 5 精度、鲁棒性、平均重叠率测试结果 Table 5 Test results of accuracy, robustness and average overlap rate
编号 | 方法 | 精度/% | 鲁棒性/% | 平均帧速/(帧·s-1) | 平均误差/% |
1 | 本文方法 | 81.90 | 8.580 | 186 | 7.330 |
2 | IVT | 65.60 | 10.13 | 20 | 7.430 |
3 | Frag | 43 | 13.99 | 615 | 10.39 |
4 | TLD | 48 | 15.65 | 38 | 11.06 |
5 | MTT | 46.80 | 10.13 | 9 | 11.40 |
6 | Struck | 39.80 | 16.71 | 64 | 11.44 |
7 | L1APG | 61.50 | 6.980 | 28 | 11.51 |
8 | LSK | 19.74 | 4.000 | 65 | 11.87 |
9 | MIL | 37.51 | 15.10 | 35 | 15.24 |
10 | DFT | 76.8 | 14.47 | 115 | 15.29 |
11 | LSK | 52.65 | 16.78 | 86 | 15.94 |
12 | OMA | 23.82 | 9.670 | 94 | 16.74 |
13 | ASLA | 48.51 | 22.51 | 66 | 16.80 |
表选项
7 结论 本文实现了对视频序列图像目标稳定精确跟踪,其方法具有如下的特点:
1) 使用频域变换将图像像素值从时空阈转换到频率域,并且这个过程中保证了图像的极值、最大值、最小值和单调性都不发生变化。在图像的频率空间实现目标的跟踪研究。通过大量的实验可以看出,在目标快速运动、运动目标姿态发生较大变化或目标部分被遮挡的情况下,本文方法也能获得更加精确的跟踪效果。
2) 提出了基于频域空间的精准的循环采样方法。
3) 实现了频域空间的能量最小化方法,用轻量级的方法解决大数据环境下的目标跟踪问题,实现了目标的精确稳定的跟踪。
4) 从大量的实验可以看出,本文方法速度可以达到186帧/s,精度也可以达到了81.90%。由于本文方法是在整个频率空间计算量小,而且又是在整个梯度空间直接计算,因此方法的速度比常用的方法快很多,精度也提高较多。
参考文献
[1] | SUPANCIC J S, RAMANAN D.Self-paced learning for long-term tracking[C]//Proceedings of the IEEE Computer Society Conference on Computer Vision and Pattern Recognition. Piscataway, NJ:IEEE Press, 2013:2379-2386.https://www.computer.org/csdl/proceedings/cvpr/2013/4989/00/4989c379.pdf |
[2] | WU Y, LIM J, YANG M H.Online object tracking:A benchmark[C]//Proceedings of the IEEE Computer Society Conference on Computer Vision and Pattern Recognition.Piscataway, NJ:IEEE Press, 2013:2411-2418.http://ieeexplore.ieee.org/document/6619156/ |
[3] | GRABNER H, BISCHOF H.On-line boosting and vision[C]//Proceedings of the IEEE Computer Society Conference on Computer Vision and Pattern Recognition.Piscataway, NJ:IEEE Press, 2006:260-267.http://ieeexplore.ieee.org/document/1640768/ |
[4] | BABENKO B, YANG M H, BELONGIE S.Visual tracking with online multiple instance learning[C]//Proceedings of the IEEE Computer Society Conference on Computer Vision and Pattern Recognition.Piscataway, NJ:IEEE Press, 2009:983-990.http://ieeexplore.ieee.org/document/5206737/ |
[5] | HARE S, GOLODETZ S, SAFFARI A, et al. Struck:Structured output tracking with kernels[J].IEEE Transactions on Pattern Analysis and Machine Intelligence, 2016, 38(10): 2096–2109.DOI:10.1109/TPAMI.2015.2509974 |
[6] | ROSS D A, LIM J, LIN R S, et al. Incremental learning for robust visual tracking[J].International Journal of Computer Version, 2008, 77(1-3): 125–141.DOI:10.1007/s11263-007-0075-7 |
[7] | MEI X, LING H. Robust visual tracking and vehicle classification via sparse representation[J].IEEE Transactions on Pattern Analysis and Machine Intelligence, 2011, 33(11): 2259–2272.DOI:10.1109/TPAMI.2011.66 |
[8] | LIU B, HUANG J, KULIKOWSKI C, et al. Robust visual tracking using local sparse appearance model and k-selection[J].IEEE Transactions on Pattern Analysis and Machine Intelligence, 2013, 35(12): 2968–2981.DOI:10.1109/TPAMI.2012.215 |
[9] | LI M, MA F, NIAN F. Robust visual tracking via appearance modeling and sparse representation[J].Journal of Computers, 2014, 9(7): 1612–1619. |
[10] | HUA Y, ALAHARI K, SCHMID C.Occlusion and motion reasoning for long-term tracking[C]//Proceedings of European Conference on Computer Vision.Berlin:Springer Verlag, 2014:172-187.http://link.springer.com/10.1007/978-3-319-10599-4_12 |
[11] | BROX T, MALIK J.Object segmentation by long term analysis of point trajectories[C]//Proceedings of European Conference on Computer Vision.Berlin:Springer Verlag, 2010:282-295.https://dl.acm.org/citation.cfm?id=1888173 |
[12] | BROX T, MALIK J. Large displacement opticalow:Descriptor matching in variational motion estimation[J].IEEE Transactions on Pattern Analysis and Machine Intelligence, 2011, 33(3): 510–513. |
[13] | FIASCHI L, DIEGO F, GREGOR K, et al.Tracking indistinguishable translucent objects over time using weakly supervised structured learning[C]//Proceedings of the IEEE Computer Society Conference on Computer Vision and Pattern Recognition.Piscataway, NJ:IEEE Press, 2014:2736-2743.http://ieeexplore.ieee.org/document/6909746/ |
[14] | BERCLAZ J, FLEURET F, TURETKEN E, et al. Multiple object tracking using k-shortest paths optimization[J].IEEE Transactions on Pattern Analysis and Machine Intelligence, 2011, 33(9): 1806–1819.DOI:10.1109/TPAMI.2011.21 |
[15] | KALAL Z, MIKOLAJCZYK K, MATAS J. Tracking-learning-detection[J].IEEE Transactions on Pattern Analysis and Machine Intelligence, 2012, 34(7): 1409–1422.DOI:10.1109/TPAMI.2011.239 |
[16] | JIA X, LU H, YANG M H.Visual tracking via adaptive structural local sparse appearance model[C]//Proceedings of the IEEE Computer Society Conference on Computer Vision and Pattern Recognition.Piscataway, NJ:IEEE Press, 2012:1822-1829.http://ieeexplore.ieee.org/document/6247880/ |
[17] | PATNAIK R.Distortion-invariant kernel correlation lters for general object recognition[D].Pittsburgh:Carnegie Mellon University, 2009.https://dl.acm.org/citation.cfm?id=1751269 |
[18] | GRABNER H, LEISTNER C, BISCHOF H.Semi-supervised on-line boosting for robust tracking[C]//Proceedings of European Conference on Computer Vision.Berlin:Springer Verlag, 2008:234-247.https://link.springer.com/chapter/10.1007/978-3-540-88682-2_19 |
[19] | BABENKO B, YANG M H, BELONGIE S. Robust object tracking with online multiple instance learning[J].IEEE Transactions on Pattern Analysis and Machine Intelligence, 2011, 33(8): 1619–1632.DOI:10.1109/TPAMI.2010.226 |
[20] | KWON J, LEEK M.Visual tracking decomposition[C]//Proceedings of the IEEE Computer Society Conference on Computer Vision and Pattern Recognition.Piscataway, NJ:IEEE Press, 2010:1269-1276.http://ieeexplore.ieee.org/document/5539821/ |
[21] | HAN Z J, YE Q X, JIAO J B.Robust visual object tracking via sparse representation and reconstruction[C]//Proceedings of International Conference on Computer Analysis of Images and Patterns.Berlin:Springer Verlag, 2013:282-289.https://link.springer.com/chapter/10.1007/978-3-642-40246-3_35 |
[22] | KALAL Z, MATAS J, MIKOLAJCZYK K.PN learning:Bootstrapping binary classifiers by structural constraints[C]//Proceedings of the IEEE Computer Society Conference on Computer Vision and Pattern Recognition.Piscataway, NJ:IEEE Press, 2010:49-56.http://ieeexplore.ieee.org/document/5540231/ |
[23] | 石武祯, 梅林, 王文斐, 等. 面向复杂场景的局部分布场跟踪方法[J].计算机辅助设计与图形学学报, 2014, 26(11): 2031–2038. SHI W Z, MEI L, WANG W F, et al. Local distribution fields for tracking in complex scenarios[J].Journal of Computer-Aided Design & Computer Graphics, 2014, 26(11): 2031–2038.(in Chinese) |
[24] | ZHANG T, GHANEM B, LIU S, et al.Robust visual tracking via multi-task sparse learning[C]//Proceedings of the IEEE Computer Society Conference on Computer Vision and Pattern Recognition.Piscataway, NJ:IEEE Press, 2012:2042-2049.https://link.springer.com/article/10.1007/s11263-012-0582-z |
[25] | DINH T B, VO N, MEDIONI G.Context tracker:Exploring supporters and distracters in unconstrained environments[C]//Proceedings of the IEEE Computer Society Conference on Computer Vision and Pattern Recognition.Piscataway, NJ:IEEE Press, 2011:1177-1184.http://ieeexplore.ieee.org/document/5995733/ |
[26] | BOLME D S, BEVERIDGE J R, DRAPER B A, et al.Visual object tracking using adaptive correlation filters[C]//Proceedings of the IEEE Computer Society Conference on Computer Vision and Pattern Recognition.Piscataway, NJ:IEEE Press, 2011:2544-2550.http://ieeexplore.ieee.org/document/5539960/ |
[27] | ZHANG K, ZHANG L, YANG M H.Real-time compressive tracking[C]//Proceedings of European Conference on Computer Vision.Berlin:Springer Verlag, 2012:864-877.http://rd.springer.com/chapter/10.1007/978-3-642-33712-3_62 |
[28] | HENRIQUES J, CASEIRO R, MARTINS P, et al.Exploiting the circulant structure of tracking-by-detection with kernels[C]//Proceedings of European Conference on Computer Vision.Berlin:Springer Verlag, 2012:702-715.https://link.springer.com/chapter/10.1007/978-3-642-33765-9_50 |
[29] | CEHOVIN L, KRISTAN M, LEONARDIS A. Robust visual tracking using an adaptive coupledlayer visual model[J].IEEE Transactions on Pattern Analysis and Machine Intelligence, 2013, 35(4): 941–953.DOI:10.1109/TPAMI.2012.145 |
[30] | BAO C, WU Y, LING H, et al.Real time robust tracker using accelerated proximal gradient approach[C]//Proceedings of the IEEE Computer Society Conference on Computer Vision and Pattern Recognition.Piscataway, NJ:IEEE Press, 2012:1830-1837.http://ieeexplore.ieee.org/document/6247881/ |
[31] | LIU B, HUANG J, YANG L, et al.Robust tracking using local sparse appearance model and k-selection[C]//Proceedings of the IEEE Computer Society Conference on Computer Vision and Pattern Recognition.Piscataway, NJ:IEEE Press, 2011:1313-1320.http://ieeexplore.ieee.org/document/5995730/ |
[32] | HENRIQUES J F, CASEIRO R, MARTINS P, et al. High-speed tracking with kernelized correlation filters[J].IEEE Transactions on Pattern Analysis and Machine Intelligence, 2015, 37(7): 583–596. |
[33] | SEVILLA-LARA L, LEARNED-MILLER E.Distribution elds for tracking[C]//Proceedings of IEEE International Conference on Advanced Video and Signal Based Surveillance-AVSS 2015.Piscataway, NJ:IEEE Press, 2015:474-479.http://ieeexplore.ieee.org/document/6247891/ |
[34] | KWON J, LEE K M.Tracking by sampling trackers[C]//Proceedings of the IEEE International Conference on Computer Vision, International Conference on Computer Vision.Piscataway, NJ:IEEE Press, 2011:1195-1202.http://ieeexplore.ieee.org/document/6126369/ |
[35] | HONG S, KWAK S, HAN B.Orderless tracking through model-averaged posterior estimation[C]//Proceedings of the IEEE International Conference on Computer Vision.Piscataway, NJ:IEEE Press, 2013:2296-2303.http://ieeexplore.ieee.org/document/6751396/ |
[36] | GU S, ZHENG Y, TOMASI C.Linear time offline tracking and lower envelope algorithms[C]//Proceedings of the IEEE International Conference on Computer Vision.Piscataway, NJ:IEEE Press, 2011:1840-1846.http://ieeexplore.ieee.org/document/6126451/ |