HTML
--> --> -->The magnitudes of the CRF are highly dependent on the cloud vertical structures, including cloud-top height (CTH), cloud-base height (CBH), cloud thickness (CT), and the vertical overlapping pattern of cloud layers (Ramanathan et al., 1989; Mitchell and Finnegan, 2009; Li et al., 2019). The impact of cloud vertical structures on the CRF is often investigated through the role of the CTH in the estimation of the CRF at the TOA (Loeb et al., 2012; Chen and Fu, 2018). However, recent studies suggest a strong dependence of the CRF on the CBH, which considerably affects the land-atmosphere energy exchanges. For example, Kato et al. (2011) indicated that the underestimation of the CBH results in an increase of the global annual mean of instantaneous surface downward long-wave irradiance. Viúdez-Mora et al. (2015) quantified the average CRF at the surface using the CBH determined from ceilometer measurements and demonstrated that the CRF decreased with CBH at a rate of 4?5 W m?2 km?1 with the average CRF observed to be in the range of 50?80 W m?2. George et al. (2018) investigated the relationships of the CBH with the CRF at the surface and TOA using soundings and found that the shortwave CRF depended on the total depth of cloud layers, whereas the longwave CRF depended on the height of cloud layers. However, there are few previous studies on the CBH impact on the estimates of the CRF in the atmosphere, and thus the role of the CBH upon the CRF estimates remains largely unknown, particularly in China.
One of the major obstacles in quantifying the impact of the CBH on the CRF lies in the difficulties in determining the CBH on a large scale. On the one hand, ground-based instruments such as lidars, ceilometers, and millimeter wavelength cloud radars (e.g., Martucci et al., 2010; Costa-Surós et al., 2013), gain competitive edges in determining CBHs for their relatively higher accuracy and stronger temporally continuous sampling capabilities (e.g., Sharma et al., 2016; Zhou et al., 2019). However, these instruments are generally deployed at very few locations (Dai et al., 2006; Zhang et al., 2019), thereby having poor spatial representation. On the other hand, the CBH cannot be directly retrieved from passive satellite observations such as the moderate resolution imaging spectroradiometer (MODIS) onboard both Terra and Aqua. Active sensors such as the Cloud-Aerosol Lidar with Orthogonal Polarization (CALIOP) onboard the CALIPSO and the millimeter-wavelength cloud profiling radar (CPR) onboard the CloudSat can resolve the vertical distribution of cloud layers, including the CBH and CTH (Winker et al., 2009; Wang et al., 2015). However, in terms of the accuracy of the CBH detected from the aforementioned active sensors, large uncertainties exist due to the strong attenuation of radar or laser beams, especially in the presence of deep convective clouds (e.g., Zhang et al., 2017; Zhou et al., 2019).
The operational radiosonde networks have higher detection accuracy of cloud layers, making it possible to determine CBH over a large spatial domain, albeit with poor temporal coverage (Poore et al., 1995; Zhang et al., 2010). Therefore, the present study aims to investigate the impact of the CBH on the estimated shortwave CRF (SWCRF) by using a high-resolution dataset from a radiosonde network in China that spanned nine years (2010?2018). As a first step, the climatology of SWCRF at the surface (SWCRFSUR), at the top of the atmosphere (SWCRFTOA), and in the atmosphere (SWCRFATM) is compiled, by combining the CBH from the radiosonde measurements and the CTH and cloud properties from MODIS for the summers spanning 2010 to 2018. The accuracy of the SWCRF climatology is established through comparisons with the Clouds and the Earth's Radiant Energy System (CERES) product. Then, the SWCRFs calculated under four scenarios which vary the vertical profile of cloud optical depth (COD) are compared to deduce the role of the CBH on the estimation of the SWCRF. In addition, we will test the sensitivity of both the CBH and solar zenith angle on the SWCRF.
2.1. Data
32.1.1. Radiosonde derived CBH
Radiosonde data provide in situ measurements of atmospheric environmental variables, including temperature, pressure, RH, wind speed, and wind direction, which is recognized to be able to derive the overlapping pattern of cloud in the vertical direction. The China radiosonde network (CRN) consists of 120 radiosonde sites across China, in which the high-resolution (5?8 m) sounding balloon is launched twice per day at 0800 LST (LST = UTC + 8) and 2000 LST (Guo et al., 2016, 2019). Based on these fine-resolution radiosonde measurements, the CBHs are calculated for the summer (June?August) during the period from 2010 to 2018 using the improved relative humidity (RH) threshold method (Zhang et al., 2018). Coincidently, up to two additional soundings are launched occasionally at 1400 LST and 0200 LST in summer during certain intensive observing periods at selected stations (Guo et al., 2016; Lou et al., 2019). Given the spatial inhomogeneity, the CBHs from all sites are interpolated onto a regular 5° × 5° grid, following the method by Zhang et al. (2018). The significant diurnal variation of summertime clouds in China (Chen et al., 2018) justifies the use of the 1400 LST soundings to derive CBHs unless otherwise noted. This is expected to better match with the CTH products from MODIS onboard Aqua, one of the polar-orbiting satellites.3
2.1.2. MODIS level-3 daily cloud products
The MODIS instrument, an important payload of both Terra and Aqua satellites, can observe the Earth with a total swath width of 2300 km, with a revisit cycle of one to two days, depending on the location (Remer et al., 2005). The MODIS has 36 spectral bands ranging from 0.4 to 14.2 μm (Salomonson et al., 1989), providing global coverage of cloud optical and physical parameters at both granule and grid levels (Platnick et al., 2003). As one of important cloud optical properties, cloud optical depth (τ) can affects the shortwave CRF by reflecting the attenuation of radiation intensity by cloud in the transmission path (Chen et al., 2019b). As the global gridded product, the level-3 cloud products of MODIS are made publicly available on a grid size of 1° × 1° at daily, 8-day, and monthly timescales (Menzel et al., 2008).Therefore, the MODIS level-3 daily cloud products onboard Aqua (MYD08_D3) are used in this study to characterize the optical and physical properties of cloud tops. In order to be collocated with the CBHs derived from the 1400 LST radiosonde data, the MODIS cloud products, including the CTH (ht), the daily averaged liquid and ice COD (τc), liquid and ice cloud effective radius (rc), as well as cloud fraction (?c), are resampled to 5° latitude× 5°longitude grid by simply averaging out the original MODIS products.
3
2.1.3. Land surface albedo
The MODIS albedo product (MCD43) could provide both white-sky albedos and black-sky albedos for spectral and broad bands. The MCD43 is a daily product, obtained by the surface reflectance observations built up over a 16-day period (Schaaf et al., 2002). As a climate modeling grid product, MCD43C3 provides albedo d ata at a spatial resolution of 0.05° latitude× 0.05° longitude grid. In the present study, a climatology of daily-averaged surface albedo (α) data was built using nine years (2010?18) of MCD43C3 black-sky visible albedo, in which only those α values with a quality flag less than or equal to two were used.3
2.1.4. CERES radiative flux data
The Clouds and the Earth's Radiant Energy System (CERES) instrument directly measures global radiance at the TOA. By combining the MODIS and GEOS-derived cloud properties, CERES could also provide the radiance at the surface (Wielicki et al., 1996). The high accuracy and robustness of the CERES radiance products in revealing Earth’s energy imbalance have been adequately verified (Loeb et al., 2012; Allan et al., 2014; Trenberth et al., 2014). As such, the CERES Synoptic TOA and surface fluxes and clouds (SYN) products under the clear-sky and all-sky conditions with a spatial resolution of 1° × 1° are used here as “ground-truth” to validate the SWCRF estimated by the radiative transfer model. Prior to its application in the following analysis, we first calculate the CERES SWCRFTOA and SWCRFSUR using the clear-sky and all-sky incoming and outgoing SW flux at the TOA and surface, respectively. Then, the CERES-derived SWCRF are resampled from a 1° × 1° to 5° × 5° latitude/longitude grid.3
2.1.5. Radiative transfer model RRTM_SW
The shortwave rapid radiative transfer model (RRTM_SW) has been used to calculate both atmospheric radiative fluxes and heating rates for the spectral wavenumber of 820?50 000 cm?1 (Clough et al., 2005). By utilizing the correlated-k approach, the fluxes provided by RRTM SW are accurate, computationally efficient, and fast. Due to its high accuracy and efficiency, the RRTM_SW has been widely applied to those studies associated with atmospheric radiative transfer and general circulation models (GCMs) (e.g., Thampi and Roca, 2014; Verlinden and de Szoek, 2018).2
2.2. Methods
32.2.1. Calculation of SWCRF
Here, the SWCRF (ΔF; W m?2) is defined as the difference of net solar flux between an environment with clouds and one without clouds present at the surface, at the TOA, or in the atmosphere, which can be expressed as:where i represents the surface, TOA, and the ATM, respectively,





Similarly,



Combining Eqs. (1)?(3), we obtain the following expression:
Since the downward flux at the top of the atmosphere is independent of the presence of clouds (i.e.,

Meanwhile, the SWCRF in the atmosphere (ΔFATM) is defined as:
3
2.2.2. Assumption concerning the vertical profile of COD
Normally, the vertical distribution of COD is assumed to be uniform in the current climate model. Here, we define four scenarios regarding the vertical distribution of COD, including a two-point distribution (Scenario 1), a uniform distribution (Scenario 0 & Scenario 2), and a normal distribution (Scenario 3), which are described as follows:(1)Two-point distribution
The two-point distribution assumes the total COD is concentrated in only two cloud layers. Thus, the COD for each cloud layer is 1/2 of the total COD. In addition, we define the two heights of the cloud layer to be 1/4 and 3/4 of the total cloud height. The distribution can be formulated as follows:
where the


(2)Uniform distribution
The uniform distribution assumes that the total COD is uniformly distributed across the cloud layers in the vertical, and the COD of the ith cloud layer is expressed as follows:
where the hi,t, and hi,b stand for the top and base height for the ith cloud layer, respectively, and N represents the number of the cloud layer.
(3)Normal distribution
The symmetrical distribution of many natural processes and phenomena is the normal distribution. In this study, we assume the vertical distribution of COD is a normal distribution. For the given interval [μ ± 3σ], the probability of the actual COD falling within that interval is nearly 1.0 (Mishra and Datta-Gupta, 2018). Thus, the COD of the ith cloud layer can be described as follows:
3
2.2.3. Radiative transfer calculations
To calculate the upward flux reaching the top-of-atmosphere (



Besides the CBH, it is well known that the SWCRF is influenced by the vertical profile of the COD. In order to investigate the impact of CBH on the SWCRF, we assume two main scenarios: (1) the SWCRF is calculated from only the CTH with the CBH unknown (Scenario 0), and (2) the SWCRF is calculated from both the CTH and CBH (Scenarios 1?3), the details of which are summarized in Table 1. Schematically shown in Fig. 1 is an example of the vertical profile of the COD as a function of the normalized total COD.
Scenarios | Vertical profile pattern of COD | Number of cloud layers (N) |
Scenario 0 | Uniform | 1 |
Scenario 1 | two-point | 2 |
Scenario 2 | Uniform | true cloud layer |
Scenario 3 | Normal | true cloud layer |
Table1. Summary for the vertical profile scenarios of cloud optical depth (COD) assumed in the calculation of cloud optical depth, which is an important input for the calculation of cloud radiative forcing.


3
2.2.4. Sensitivity analysis
We perform a sensitivity analysis to better understand the impact of CBH on the SWCRF. This consisted of a series of experiments on the CRF estimation, that have been conducted by running the RRTM_SW with varying CBH values, under different cloud vertical profile patterns of COD. In an ideal experiment, the liquid effective particle radius is set to be uniform at 15 μm, whereas the solar zenith angles (SZA) are set to 0°, 30°, and 60°, respectively. The CBH values are set to increase from 0.5 km upwards until 5.0 km at 0.5 km intervals, and the increase in CBH each time is defined as Δhb. The total COD is constant, and the vertical profile of COD is calculated using the cloud-top height (ht) and the changed cloud-base height (hb + Δhb).3.1. Distribution of CTH, CBH and cloud thickness in China
Figure 2 presents the geographic distributions of summertime cloud properties in China within 5° latitude × 5° longitude grid boxes during the period from 2010 to 2018, including the CTH derived from the MODIS/Aqua, the CBH derived from the radiosonde measurements, and the cloud thickness (CT). The mean CTH, CBH, and CT correspond to 7.23 km, 3.56 km, and 4.71 km above ground level (AGL), respectively. Overall, the CTH exhibits a noticeable geographical variability in summer across China. For instance, the cloud top tends to develop much higher over the Tibetan Plateau (TP) and South China Sea (SCS), where the South Asian Monsoon and mesoscale convective systems most frequently occur (Wang and Ho, 2002; Chen et al., 2019a). In the latitude zone between 30°N and 45°N, western China has higher CTHs than eastern China, which is likely due to the terrain difference between eastern and western China. By comparison, the summertime CBHs exhibit a “northwest-high-southeast-low” pattern: CBHs as high as 4.0 km AGL or higher are found in Northwest China versus much smaller CBHs (less than 3.0 km AGL) in Southeast China. The lowest CBH tends to occur in the coastal region of East China, and the northern part of the South China Sea, which is lower than 2.0 km AGL. This could be attributed to a lower lifting condensation level due to higher dew points near the coast. Another pronounced signal is evidenced by the decreasing trend of CT with increasing latitude, indicating that the CT spatial pattern exhibits a strong south-to-north gradient, with greater CT in southern China.
Figure 3 shows the spatial distributions of height-resolved occurrence frequency for the CTH, CBH, and CT, respectively. The probability density function (PDF) of CTH peaks at around 6?8 km AGL and features a heavy tail. Specifically, most of the CTHs (~80%) are mainly located above 5 km, indicating the dominance of middle- and high-level clouds in China. By comparison, the tail shifts towards low CBHs, and the CBHs falling within 0?3 km are responsible for approximately 65% of all cases. Interestingly, the PDF of cloud thickness exhibits a roughly normal distribution, with more than half of the CTs falling within 2?5 km. Overall, the frequency distributions of CTH and CBH revealed here are broadly consistent with the previous remote sensing studies (e.g., Yin et al., 2015; Chen et al., 2018). Note that the PDFs of CTH, CBH, and CT given in 0.1 km vertical range bins are also shown in Fig. 3. The peak in the PDF of CBH is generally located at 1?2 km, and then gradually decreases with height above 2 km, indicating that the bases of cloud layers are lower than 2 km AGL under most circumstances.

Figure 4 illustrates the percentile analysis for CBH, CTH, and CT as a function of latitude. It is clear that CBHs tend to increase with latitude, reaching the peaks in the latitude range between 37.5°N and 42.5°N. Coincidently, the planetary boundary layer height (PBLH) exhibits an interesting spatial pattern of “northwest high southeast low” in China (i.e., Zhang et al., 2018; Guo et al., 2019; Xu et al., 2021). Therefore, the dependence of CBH on latitude resembles the spatial pattern of PBLH in China, to some extent. This could be attributed to the spatial discrepancy of soil moisture (Xu et al., 2021) since the dominant factor driving the development of the boundary layer comes from sensible heat flux that is closely associated with soil moisture. In particular, as the latitude increases, the gap of CBH shifts towards larger values particularly when the CBH is below the 50th percentile, suggesting a patchier CBH distribution at high altitude regions in China (under lower cloud base circumstances). As for the linear meridional trends in CBH percentiles, the lower percentiles of CBH increase slowly, in contrast to the higher percentiles of CBH. The trends of high CBHs (e.g., 80th and 90th percentile), on average, are much larger than those of low CBHs (e.g., 50th percentile and below). Regarding the CTHs, low percentiles of CTHs do not show any pronounced latitudinal dependence, while high percentiles of CTHs tend to decrease with increasing latitudes. The average trend of high CTHs (90th percentile) is far less than twice as large as the median CTHs (50th percentile). This is also the case for CT, since the high percentiles of CTs decrease much more rapidly with the increasing latitude compared to the median percentile of CT.
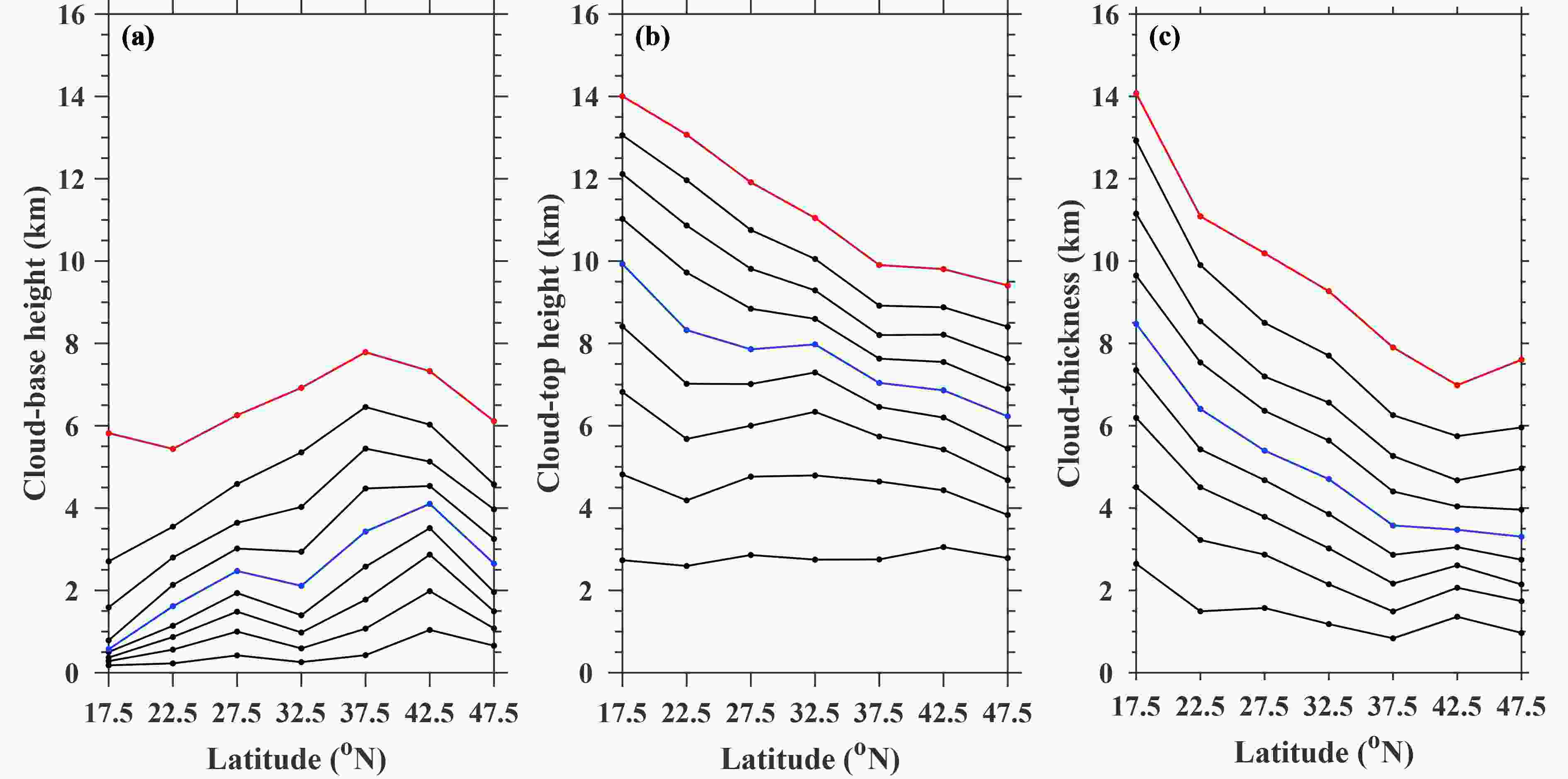
2
3.2. Evaluation of the simulated SWCRF climatology
Using the radiosonde-derived CBH and the MODIS-derived CTH as the two main input parameters, the SWCRF climatology is simulated in summer over China. In order to confirm the reliability of this climatology, the simulated SWCRF is compared with its counterpart from CERES in Fig. 5. In general, the SWCRFSUR and SWCRFTOA are negative, whereas the SWCRFATM is positive. This means that clouds tend to exhibit a cooling effect at the SUR and TOA, but exhibit a warming effect in the ATM during summer over China. A high correlation (R = 0.8) is found between the simulated SWCRFTOA and CERES observation. Similarly, the SWCRFSUR agrees well with CERES (R = 0.79). These robust relationships indicate that the simulated SWCRF climatology is consistent with the CERES measurements at the TOA and the surface.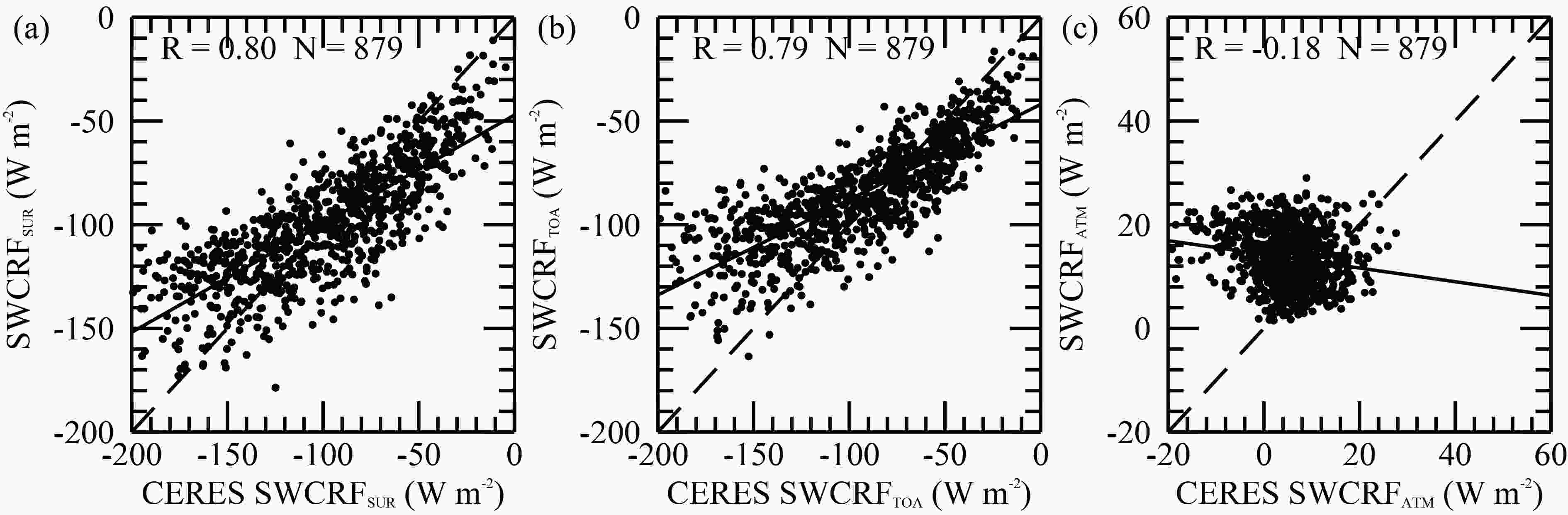
However, there are large differences between the simulated and the CERES-derived SWCRFATM. We hypothesize that the probable reason for this could be the inability of the satellite observation of CERES to capture the cloud bottom. To confirm our hypothesis, we conducted a correlation analysis concerning the absolute difference between the CERES-derived and the simulated SWCRFATM (ΔSWCRFATM) versus cloud height. Figure 6a shows that the biases which exist in the CERES-derived SWCRFATM positively correlate with cloud thickness, while it negatively correlates with cloud bottom (Fig. 6b). This indicates that the biases of CERES SWCRFATM tend to increase when thick clouds with low base height are present. Also, the negative SWCRFATM from the CERES product (red points in Fig. 6) further demonstrates that large biases from satellites often occur for low and thick clouds. The reason for this could be that the satellite observation could not provide an accurate estimation of cloud bottom, especially in the presence of thick clouds with low bottoms.

2
3.3. Spatial pattern of SWCRF
Figure 7 presents the spatial distribution of summertime SWCRFSUR, SWCRFTOA, and SWCRFATM in China during the period from 2010 to 2018. The mean values of SWCRFSUR, SWCRFTOA and SWCRFATM are ?97.14 W m?2, ?84.35 W m?2, and 12.79 W m?2, respectively. This result is consistent with previous studies. For example, Yu et al. (2001) estimated SWCRFTOA values as large as ?85.0 W m?2 using the observations from the Earth Radiation Budget Experiment (ERBE) experiment during summertime in eastern China. Similarly, Wang et al. (2004) obtained the mean SWCRFTOA over the Yangtze River?Huaihe River valley at about ?110.0, ?100.0, and ?70.0 W m?2 in June, July, and August, respectively. In particular, relatively small SWCRFSUR is observed in Northwest China (Fig. 7a), which may be explained by the thin cloud layer caused by the high CBH and low CTH common to this region (Fig. 2). By comparison, the SWCRFSUR in central and eastern China is high, which is attributed to the large cloud thickness in this region. This result demonstrates that the SWCRFSUR largely depends on the cloud thickness but has no relation with the cloud base. Our results are consistent with previous findings (e.g., Zhang et al., 2017; George et al., 2018), which suggests that cloud depth contributes significantly to the estimate of SWCRFSUR.
The spatial distribution of SWCRFTOA (Fig. 7b), to some extent, is roughly similar to that of the SWCRFSUR. This indicates that SWCRFTOA is also influenced by cloud depth but also has no relation with the cloud base. A probable reason for this could be that SWCRFTOA is highly dependent on cloud optical depth, which is positively correlated with cloud depth.
Also noteworthy is that the spatial distribution of SWCRFATM and CBH exhibits an obvious negative correlation. As shown in Fig. 7c, the SWCRFATM is low in northwest China where high clouds always occur. In contrast, the SWCRFATM in southeast China is high, which is attributed to the low cloud base in this region. A possible reason may be that low clouds tend to increase the multiple scattering and absorption of solar radiation between the clouds and the surface, which enhances the solar radiation absorbed by the cloud, thus, resulting in a marked increase in the SWCRFATM. This result demonstrates that the height of the cloud base significantly contributes to the estimate of the SWCRFATM. By comparison, the frequencies of the SWCRFATM as a function of latitude are consistent with the height of cloud base, which increases first and then decreases as the latitude increases. This further highlights the importance of the cloud base in modulating SWCRFATM.
2
3.4. Correlation analyses between SWCRF and CBH
To further examine the relationship between the CBH and SWCRF, the SWCRFSUR, SWCRFTOA, and SWCRFATM are calculated by differentiating between the various vertical distribution patterns of COD, which has already been clarified in Table 1 and Fig. 1. Figure 8 shows the comparison of the CBH and SWCRF at the surface, top-of-atmosphere, and in the atmosphere. As expected, the SWCRFATM negatively correlated with cloud base, while the SWCRFSUR and SWCRFTOA have no relation to cloud base. Under Scenarios 2 and 3, the correlation of SWCRFATM with the height of cloud base is strong, with the R values as high as 0.76. However, under Scenario 0, the relationship between SWCRFATM estimates and cloud base is weak, since it does not consider the cloud base at all.
2
3.5. Impact of CBH on SWCRF
In this section, we will perform a quantitative analysis to deduce how the SWCRFATM is dependent on cloud base. The SWCRF estimates, under different vertical profile scenarios of COD, are shown in Fig. 9. Overall, the estimates of SWCRFSUR do not differ with the vertical distribution of COD (Fig. 9a). On average, the relative deviations of the SWCRFSUR with (Scenarios 1?3) and without (Scenario 0) considering cloud bottom is very small (only 0.32%), which indicates that the impact of cloud base on the SWCRFSUR can be ignored. This result is consistent with previous studies pointing out that the SWCRF at the surface is independent of the cloud bottom height (e.g., George et al., 2018).
Similar to the SWCRFSUR, the error in the SWCRFTOA estimates considering no cloud bottom is not as obvious (Fig. 9b). However, it is inferred that the SWCRFTOA tends to be overestimated if the cloud base is not considered in the estimation of cloud forcing, especially under Scenario 1. The relative deviations of the SWCRFTOA under Scenario 1 from that under Scenario 0 is nearly 6.1%, which demonstrates that an accurate cloud base is more essential to the SWCRFTOA than to the SWCRFSUR.
In stark contrast to the SWCRFSUR and the SWCRFTOA, the magnitudes of estimated SWCRFATM under different profile scenarios of COD differ greatly (Fig. 9c). Given the lack of consideration of an accurately described cloud bottom, the SWCRFATM is expected to be underestimated. The possible reason is that the true cloud bottom would be overestimated if the cloud base is not considered in the calculation of the SWCRFATM, which would reduce the multiple scattering and absorption of solar radiation between the surface and cloud layer, ultimately resulting in an underestimate of the SWCRFATM.
The absolute (relative) discrepancy of the SWCRFATM between Scenario 0 and Scenarios 1?3 reaches up to 9.61 (57%), 5.43 (42%), and 4.82 (40%) W m-2, suggesting that the influence of cloud base on the SWCRFATM estimate under Scenario 1 is much larger compared to that under Scenario 2 and 3. This could be likely associated with the multiple scattering and absorption of solar radiation. With the assumption of a two-point COD vertical distribution, the multiple scattering and absorption of solar radiation become strongest, which allows more solar radiation to be absorbed by the clouds, resulting in a commensurate increase of the SWCRFATM. Overall, the SWCRFATM estimates without using cloud base as input are considerably lower than those that use the cloud base, indicating the importance of using an in situ observed cloud base for an accurate calculation of the SWCRFATM.
2
3.6. Sensitivity of SWCRF to CBH and SZA
Provided that the solar zenith angle (SZA) can greatly modulate the total solar radiation that is available to interact with cloud layers, this subsection will elaborate on the sensitivity of the SWCRF to CBH and SZA in an attempt to further quantify how the SWCRF changes with the cloud base. As shown in Figs. 10a-c, the SWCRFSUR estimates are nearly unaffected by increases in the height of cloud base, irrespective of SZA and the vertical profile of COD. This indicates that the calculation of SWCRFSUR does not change appreciably with the cloud base and/or the SZA, which has been well documented in previous studies. For example, Meloni et al. (2005) reported on the modest dependency of the shortwave radiative forcing on the vertical profile of the cloud. The reasons behind this may be that the total COD and other cloud properties do not change much, and thus the capability for the cloud to scatter and absorb solar radiation is kept nearly constant.
The sensitivity of the SWCRFTOA to CBH and the SZA exhibits similar behavior as those for the SWCRFSUR (Figs. 10d-f). The sensitivity of the SWCRFTOA to both cloud base and the SZA is weak. As the cloud base increases, the magnitude of SWCRFTOA tends to increase. This may be related to the expectation that a higher cloud base corresponds to a larger COD for the top of the cloud, which enhances the scattering of the solar radiation, and thus results in an increased SWCRF at the top of the atmosphere.
In sharp contrast to the SWCRFSUR and SWCRFTOA, the calculated SWCRFATM is found to be sensitive to both cloud base and SZA, and the slope or sensitivity becomes much stronger (Figs. 10g-i). Interestingly, the magnitude of the SWCRFATM tends to decrease with a commensurate increase of cloud base in a monotonic scaling way.
Also noteworthy is that the scaling rate of the SWCRFATM with cloud base differs greatly according to the scenario. The sensitivity under Scenario 1 and Scenario 3 is much larger than Scenario 2. More striking is that the SZA not only changes the slope, but also the absolute magnitude of SWCRFATM. For instance, the magnitude of SWCRFATM under Scenario 1 is found to decline from 17?23 W m?2 at SZA of 0° (Fig. 9g) to ?7?2 W m?2 at SZA of 60° (Fig. 9i) as the Δhb increases from 0.5 km to 5.0 km, which indicates that the SWCRFATM decreases along with the increase of SZA. Besides, this suggests that the largest discrepancy of SWCRFATM under Scenario 1 preferentially occurs under small SZA conditions, which generally corresponds to late morning, noon, or early afternoon, which is roughly consistent with the results mentioned in section 3.5. By comparison, the largest discrepancy of the SWCRFATM under Scenario 2 preferentially occurs under large SZA conditions, which generally corresponds to early morning and late afternoon. We also notice that the increases in SZA are closely associated with a decreased SWCRFATM that is estimated with CBH as input, further illustrating the important role of cloud base.
Overall, there are good correlations between the simulated and the CERES-derived SWCRFs. However, an obvious disagreement occurs between the simulated and CERES-derived SWCRFATM especially for thick clouds with low cloud bottoms. Also, a series of experiments have been done by assuming two kinds of vertical profiles of COD, Scenario 0 and Scenarios 1?3. The former assumption features only CTH and cloud properties from MODIS as input, and the latter combines both CTH and cloud properties and CBH.
Comparison analyses further suggest that a large discrepancy exists in SWCRFATM between Scenario 0 and Scenarios 1?3. Meanwhile, Scenario 1 produces the largest uncertainties of the SWCRFATM. By comparison, no dramatic difference is observed for the estimate of the SWCRFSUR and SWCRFTOA, under the different scenarios concerning the vertical profiles of the COD. Given the nature of the COD assumed as a two-point distribution under Scenario 1, it is inferred that adequate consideration of the cloud base, would lead to much more realistic estimates of cloud forcing in the atmosphere. Furthermore, the sensitivity analyses also corroborate the findings mentioned above, Scenario 1 produces much more realistic cloud forcing compared with those without consideration of cloud layers in the vertical. As for Scenario 2, the influence of the cloud base on the cloud forcing is largest under large SZA conditions. Therefore, we argue that the impact of CBH depends upon an accurate estimate of the SWCRFATM, especially under Scenario 1, at the time period from late morning through early afternoon when the SZA is relatively small, or under Scenario 2 for the early morning and late afternoon.
Nevertheless, we have to emphasize that our study makes relatively simple assumptions for the vertical profiles of the COD, which inevitably impairs our ability to accurately estimate the cloud forcings. Our understanding and accurate computation of cloud forcing will be greatly improved with the inclusion of additional instrumentation that can resolve the vertical structure of clouds, which will result in more realistic forcing caused by the changing clouds under global warming. Besides, MODIS/Aqua only provides a single snapshot of the clouds, which makes it impossible to characterize the diurnal cycles of cloud properties (e.g., the cloud fraction, COD, and cloud effective radius) and ultimately cloud radiative effect. Improvement in this regard is worthy of separate analysis and should be based on deriving cloud properties from geostationary satellites, like Himawari-8 and Fengyun-4.
Acknowledgments. We acknowledge the support from the National Key R&D Program of China under Grants Nos. 2017YFC1501401 and 2017YFC0212803, the National Natural Science Foundation under Grant No. 41771399, and the Chinese Academy of Meteorological Sciences under Grant No. 2018Y014. Also, we are grateful to NASA for granting access to the level-3 cloud products from MODIS/Aqua. Last but not least, special thanks go to the National Meteorological Information Center, China Meteorological Administration for generously providing the radiosonde datasets used here (