HTML
--> --> -->Measurements of economic risk are science-based tools used by governments to make important decisions related to climate change. They are also core components of previous IPCC scientific assessment reports. To better measure the relationship between different socioeconomic development models and climate change risks, the IPCC developed scenario A (SA90) for the first assessment report (FAR) in 1990 (IPCC, 1990), IS92 for the third assessment report (TAR) in 1992 (IPCC, 1992), the SRE scenario for the TAR’s special report on emissions and the fourth assessment report (AR4; IPCC, 2000), and the Representative Concentration Pathway (RCP) for the fifth assessment report (van Vuuren et al., 2011). Phase 6 of the Coupled Model Intercomparison Project (CMIP6) uses six integrated assessment models (IAMs), various shared socioeconomic paths (SSPs), and the latest trends in anthropogenic emissions and land-use changes to generate new prediction scenarios. These scenarios form part of CMIP6 and are referred to as the Scenario Model Intercomparison Project (ScenarioMIP; O’Neill et al., 2016).
ScenarioMIP is a matrix combination of different SSPs and radiative forcing. An SSP describes possible future social development without the effects of climate change or climate policy (Zhang et al., 2019). O’Neill et al. (2016) gave a complete description of ScenarioMIP for CMIP6. For the analysis presented here, we briefly describe each SSP. A total of five pathways (i.e., SSP1, SSP2, SSP3, SSP4, and SSP5) are included in CMIP6, which consider the effects of population changes, economic growth, and urbanization (Calvin et al., 2017; Kriegler et al., 2017; Fricko et al., 2017; Fujimori et al., 2017; van Vuuren et al., 2017). Among the pathways, SSP1 is the most optimistic scenario and maintains sustainable development. In contrast, SSP5 assumes an energy intensive, fossil-fuel-based economy, although it also assumes relatively optimistic development. SSP2 is a middle pathway, which assumes current development trends continue in the future. SSP3 and SSP4 are the most undesirable pathways and assume unsustainable development trends, involving less investment in education and health, fast-growing populations, and increasing inequality. ScenarioMIP uses the IAMs to generate quantitative predictions of greenhouse gas emissions, atmospheric component concentrations, and land-use changes that may occur under different SSP energy scenarios. ScenarioMIP divides the experiments into two groups: Tier 1 and Tier 2. Tier 1 includes new SSP-based scenarios (SSP1-2.6, SSP2-4.5, SSP5-8.5) as continuations of the RCP2.6, RCP4.5, and RCP8.5 forcing levels, and an additional unmitigated forcing scenario (SSP3-7.0) with particularly high aerosol emissions and land-use change. Tier 2 includes additional scenarios of interest as well as additional ensemble members and long-term extensions (SSP1-1.9, SSP4-3.4, SSP4-6.0, SSP5-3.4-over) (O’Neill et al., 2016).
The Chinese Academy of Sciences Flexible Global Ocean–Atmosphere–Land System Model, GridPoint version 3 (CAS FGOALS-g3), developed by the State Key Laboratory of Numerical Modeling for Atmospheric Sciences and Geophysical Fluid Dynamics (LASG), Institute of Atmospheric Physics (IAP), Chinese Academy of Sciences (CAS), has completed the Tier 1 and 2 experiments of ScenarioMIP (Li et al., 2020a). Simulation results have been submitted to the Earth System Grid (ESG) data server (
2.1. Model description
CAS FGOALS-g3 comprises the following five components:(1) Atmospheric general circulation model (AGCM). The Gridpoint Atmospheric Model of IAP LASG, version 3 (GAMIL3) (Li et al. 2020b), is an updated version of GAMIL2 (Li et al., 2013).
(2) Oceanic general circulation model (OGCM). The LASG/IAP Climate Ocean Model (LICOM3) has been updated from LICOM2 (Liu et al., 2012; Lin et al., 2016). LICOM3 has performed the OMIP simulations and a detailed description of the results is given by Lin et al. (2020).
(3) Land model. The Land Surface Model of the Chinese Academy of Sciences (CAS-LSM), the land component of FGOALS-g3 with the same horizontal resolution as the atmospheric model, is based on the Community Land Model, version 4.5 (CLM4.5).
(4) Sea ice model. The sea ice model is the improved Los Alamos sea ice model, version 4.0, which uses the same grid as the oceanic model.
(5) Coupler. In FGOALS-g3, there are two optional couplers: CPL7, developed by the National Center for Atmospheric Research (NCAR) (Craig et al., 2012), and the Community Coupler, version 2 (C-Coupler2), developed by Tsinghua University (Liu et al., 2018).
A detailed description of CAS FGOALS-g3 is given in Li et al. (2020a).
2
2.2. Experimental design
Following the requirements for ScenarioMIP experiments (O’Neill et al., 2016), we carried out simulations for eight scenarios (Experiment ID in Table 1). In these experiments, the external forcings, including greenhouse gas concentrations, ozone concentrations, anthropogenic aerosol optical properties and an associated Twomey effect, land-use changes, and solar irradiance, are all based on the SSP scenario. All experiments were initialized from 1 January 2015 (branch run from the end of the historical runs, which ended on 31 December 2014) and share the same physical scheme settings, which are exactly same as those of the historical run. Experiment variants are labelled; e.g., r1i1p1f1, indicating the realization, initialization, physical, and forcing indices. We used the branch run method for the Tier 1 and 2 SSP scenario simulations. For example, the label r1i1p1f1 indicates that the initial conditions are the outputs from the historical r1i1p1f1 branch run. Table 1 gives detailed descriptions of each experiment.Experiment ID | Variant Label | Description | |
Tier 1 | SSP1-2.6 doi:10.22033/ESGF/CMIP6.3465 | r1i1p1f1 | Initialized from the historical r1i1p1f1 branch run. All external forcings were from the SSP1-2.6 scenario. |
r2i1p1f1 | Initialized from the historical r2i1p1f1 branch run. | ||
r3i1p1f1 | Initialized from the historical r3i1p1f1 branch run. | ||
r4i1p1f1 | Initialized from the historical r4i1p1f1 branch run. | ||
SSP2-4.5 doi:10.22033/ESGF/CMIP6.3469 | r1i1p1f1 | Initialized from the historical r1i1p1f1 branch run. All external forcings were from the SSP2-4.5 scenario. | |
r2i1p1f1 | Initialized from the historical r2i1p1f1 branch run. | ||
r3i1p1f1 | Initialized from the historical r3i1p1f1 branch run. | ||
r4i1p1f1 | Initialized from the historical r4i1p1f1 branch run. | ||
SSP3-7.0 doi:10.22033/ESGF/CMIP6.3480 | r1i1p1f1 | Initialized from the historical r1i1p1f1 branch run. All external forcings were from the SSP3-7.0 scenario. | |
r2i1p1f1 | Initialized from the historical r2i1p1f1 branch run. | ||
r3i1p1f1 | Initialized from the historical r3i1p1f1 branch run. | ||
r4i1p1f1 | Initialized from the historical r4i1p1f1 branch run. | ||
r5i1p1f1 | Initialized from the historical r5i1p1f1 branch run. | ||
SSP5-8.5 doi:10.22033/ESGF/CMIP6.3503 | r1i1p1f1 | Initialized from the historical r1i1p1f1 branch run. All external forcings were from the SSP5-8.5 scenario. | |
r2i1p1f1 | Initialized from the historical r2i1p1f1 branch run. | ||
r3i1p1f1 | Initialized from the historical r3i1p1f1 branch run. | ||
r4i1p1f1 | Initialized from the historical r4i1p1f1 branch run. | ||
Tier 2 | SSP1-1.9 doi:10.22033/ESGF/CMIP6.3462 | r1i1p1f1 | Initialized from the historical r1i1p1f1 branch run. All external forcings were from the SSP1-1.9 scenario. |
SSP4-3.4 doi:10.22033/ESGF/CMIP6.3493 | r1i1p1f1 | Initialized from the historical r1i1p1f1 branch run. All external forcings were from the SSP4-3.4 scenario. | |
SSP5-3.4-over doi:10.22033/ESGF/CMIP6.3499 | r1i1p1f1 | Initialized from the historical r1i1p1f1 branch run. All external forcings were from the SSP5-3.4-over scenario. | |
SSP4-6.0 doi:10.22033/ESGF/CMIP6.3496 | r1i1p1f1 | Initialized from the historical r1i1p1f1 branch run. All external forcings were from the SSP4-6.0 scenario. |
Table1. ScenarioMIP experiment descriptions.
We used the model outputs for the period 2015–2100 in our analysis. Following the requirements of CMIP6 (Martin et al., 2020), monthly mean values for the primary variables of each component model were output. To investigate predicted extreme weather events in each scenario, the atmospheric component also provides additional 6-h and 3-h high-frequency outputs for some variables, including precipitation, specific humidity, and near-surface air temperature, for both future predictions and the historical runs. Details of the primary outputs and diagnostic variables for each component model are given in Tables 2–5.
Variable Name | Description | Output Frequency |
cl | Percentage Cloud Cover | Monthly |
cli | Mass Fraction of Cloud Ice | Monthly |
clivi | Ice Water Path | Monthly |
clt | Total Cloud Cover Percentage | 3-h*, Daily, Monthly |
clw | Mass Fraction of Cloud Liquid Water | Monthly |
clwvi | Condensed Water Path | Monthly |
evspsbl | Evaporation Including Sublimation and Transpiration | Monthly |
hfls | Surface Upward Latent Heat Flux | 3-h*, Daily, Monthly |
hfss | Surface Upward Sensible Heat Flux | 3-h*, Daily, Monthly |
hur | Relative Humidity | Daily, Monthly |
hurs | Near-Surface Relative Humidity | 6-h*, Daily, Monthly |
hursmax | Daily Maximum Near-Surface Relative Humidity | Daily |
hursmin | Daily Minimum Near-Surface Relative Humidity | Daily |
hus | Specific Humidity | 6-h*, Daily, Monthly |
huss | Near-Surface Specific Humidity | 3-h*, Daily, Monthly |
mc | Convective Mass Flux | Monthly |
o3 | Mole Fraction of O3 | Monthly |
pfull | Pressure at Model Full-Levels | 6-h*, Monthly |
phalf | Pressure on Model Half-Levels | Monthly |
pr | Precipitation | 3-h*, 6-h*, Daily, Monthly |
prc | Convective Precipitation | 3-h*, Daily, Monthly |
prhmax | Maximum Hourly Precipitation Rate | 6-h* |
prsn | Snowfall Flux | 3-h*, Daily, Monthly |
prw | Water Vapor Path | Monthly |
ps | Surface Air Pressure | 3-h*, 6-h*, Monthly |
psl | Sea Level Pressure | 6-h*, Daily, Monthly |
rlds | Surface Downwelling Longwave Radiation | 3-h*, Daily, Monthly |
rldscs | Surface Downwelling Clear-Sky Longwave Radiation | 3-h*, Monthly |
rls | Net Longwave Surface Radiation | Daily |
rlus | Surface Upwelling Longwave Radiation | 3-h*, Daily, Monthly |
rlut | TOA Outgoing Longwave Radiation | Daily, Monthly |
rlutcs | TOA Outgoing Clear-Sky Longwave Radiation | Monthly |
rsds | Surface Downwelling Shortwave Radiation | 3-h*, Daily, Monthly |
rsdscs | Surface Downwelling Clear-Sky Shortwave Radiation | 3-h*, Monthly |
rsdsdiff | Surface Diffuse Downwelling Shortwave Radiation | 3-h* |
rsdt | TOA Incident Shortwave Radiation | Monthly |
rss | Net Shortwave Surface Radiation | Daily |
rsus | Surface Upwelling Shortwave Radiation | 3-h*, Daily, Monthly |
rsuscs | Surface Upwelling Clear-Sky Shortwave Radiation | 3-h*, Monthly |
rsut | TOA Outgoing Shortwave Radiation | Monthly |
rsutcs | TOA Outgoing Clear-Sky Shortwave Radiation | Monthly |
rtmt | Net Downward Radiative Flux at Top of Model | Monthly |
sfcWind | Near-Surface Wind Speed | 6-h*, Daily, Monthly |
sfcWindmax | Daily Maximum Near-Surface Wind Speed | Daily |
ta | Air Temperature | 6-h*, Daily, Monthly |
tas | Near-Surface Air Temperature | 3-h*, 6-h*, Daily, Monthly |
tasmax | Daily Maximum Near-Surface Air Temperature | Daily, Monthly |
tasmin | Daily Minimum Near-Surface Air Temperature | Daily, Monthly |
tauu | Surface Downward Eastward Wind Stress | Monthly |
tauv | Surface Downward Northward Wind Stress | Monthly |
ts | Surface Temperature | Monthly |
ua | Eastward Wind | 6-h*, Daily, Monthly |
va | Northward Wind | 6-h*, Daily, Monthly |
wap | Omega (= dp/ dt) | 6-h*, Daily, Monthly |
zg | Geopotential Height | Daily, Monthly |
Table2. AGCM output variables from FGOALS-g3 for the ScenarioMIP experiments. TOA means top of atmosphere; * represents additional high-frequency output variables.
Variable Name | Description | Output Frequency |
friver | Water Flux into Sea Water from Rivers | Monthly |
hfbasin | Northward Ocean Heat Transport | Monthly |
hfds | Downward Heat Flux at Sea Water Surface | Monthly |
hflso | Surface Downward Latent Heat Flux | Monthly |
hfsso | Surface Downward Sensible Heat Flux | Monthly |
mlotst | Ocean Mixed Layer Thickness Defined by Sigma T | Monthly |
msftbarot | Ocean Barotropic Mass Stream Function | Monthly |
msftmz | Ocean Meridional Overturning Mass Stream Function | Monthly |
msftmzmpa | Ocean Meridional Overturning Mass Stream Function Due to Parameterized Mesoscale Advection | Monthly |
rlntds | Surface Net Downward Longwave Radiation | Monthly |
rsntds | Net Downward Shortwave Radiation at Sea Water Surface | Monthly |
so | Sea Water Salinity | Monthly |
soga | Global Mean Sea Water Salinity | Monthly |
sos | Sea Surface Salinity | Monthly |
thetao | Sea Water Potential Temperature | Monthly |
thetaoga | Global Average Sea Water Potential Temperature | Monthly |
tos | Sea Surface Temperature | Monthly |
tossq | Square of Sea Surface Temperature | Monthly |
umo | Ocean Mass X Transport | Monthly |
uo | Sea Water X Velocity | Monthly |
vmo | Ocean Mass Y Transport | Monthly |
vo | Sea Water Y Velocity | Monthly |
vsf | Virtual Salt Flux into Sea Water | Monthly |
wfo | Water Flux into Sea Water | Monthly |
wmo | Upward Ocean Mass Transport | Monthly |
wo | Sea Water Vertical Velocity | Monthly |
zos | Sea Surface Height Above Geoid | Monthly |
zossq | Square of Sea Surface Height Above Geoid | Monthly |
Table3. OGCM output variables from FGOALS-g3 for the ScenarioMIP experiments.
Variable Name | Description | Output Frequency |
evspsblsoi | Water Evaporation from Soil | Monthly |
evspsblveg | Evaporation from Canopy | Monthly |
gwt | Groundwater Intake | Monthly |
mrfso | Soil Frozen Water Content | Monthly |
mrro | Total Runoff | Monthly |
mrros | Surface Runoff | Monthly |
mrso | Total Soil Moisture Content | Monthly |
mrsos | Moisture in Upper Portion of Soil Column | Monthly |
prveg | Precipitation onto Canopy | Monthly |
tsl | Temperature of Soil | Monthly |
frostdp | Frost Deep | Monthly |
snc | Snow Area Percentage | Monthly |
snd | Snow Depth | Monthly |
thawdp | Thaw Depth | Monthly |
Table4. Land model output variables from FGOALS-g3 for the ScenarioMIP experiments.
Variable Name | Description | Output Frequency |
sfdsi | Downward Sea Ice Basal Salt Flux | Monthly |
siconc | Sea-Ice Area Percentage (Ocean Grid) | Monthly |
sidconcdyn | Sea-Ice Area Percentage Tendency Due to Dynamics | Monthly |
sidconcth | Sea-Ice Area Percentage Tendency Due to Thermodynamics | Monthly |
sidivvel | Divergence of the Sea-Ice Velocity Field | Monthly |
sidmassdyn | Sea-Ice Mass Change from Dynamics | Monthly |
sidmassgrowthbot | Sea-Ice Mass Change Through Basal Growth | Monthly |
sidmassgrowthwat | Sea-Ice Mass Change Through Growth in Supercooled Open Water (Frazil) | Monthly |
sidmasslat | Lateral Sea-Ice Melt Rate | Monthly |
sidmassmeltbot | Sea-Ice Mass Change Through Bottom Melting | Monthly |
sidmassmelttop | Sea-Ice Mass Change Through Surface Melting | Monthly |
sidmasssi | Sea-Ice Mass Change Through Snow-to-Ice Conversion | Monthly |
sidmassth | Sea-Ice Mass Change from Thermodynamics | Monthly |
siflcondtop | Net Conductive Heat Flux in Ice at the Surface | Monthly |
sifllatstop | Net Latent Heat Flux over Sea Ice | Monthly |
sifllwdtop | Downwelling Longwave Flux over Sea Ice | Monthly |
sifllwutop | Upwelling Longwave Flux over Sea Ice | Monthly |
siflsenstop | Net Upward Sensible Heat Flux over Sea Ice | Monthly |
siflsensupbot | Net Upward Sensible Heat Flux Under Sea Ice | Monthly |
siflswdbot | Downwelling Shortwave Flux Under Sea Ice | Monthly |
siflswdtop | Downwelling Shortwave Flux over Sea Ice | Monthly |
siflswutop | Upwelling Shortwave Flux over Sea Ice | Monthly |
siforcecoriolx | Coriolis Force Term in Force Balance (X-Component) | Monthly |
siforcecorioly | Coriolis Force Term in Force Balance (Y-Component) | Monthly |
siforceintstrx | Internal Stress Term in Force Balance (X-Component) | Monthly |
siforceintstry | Internal Stress Term in Force Balance (Y-Component) | Monthly |
sipr | Rainfall Rate over Sea Ice | Monthly |
sishevel | Maximum Shear of Sea-Ice Velocity Field | Monthly |
sisnconc | Snow Area Percentage | Monthly |
sistrxdtop | X-Component of Atmospheric Stress on Sea Ice | Monthly |
sistrxubot | X-Component of Ocean Stress on Sea Ice | Monthly |
sistrydtop | Y-Component of Atmospheric Stress on Sea Ice | Monthly |
sistryubot | Y-Component of Ocean Stress on Sea Ice | Monthly |
sitemptop | Surface Temperature of Sea Ice | Monthly |
sitimefrac | Fraction of Time Steps with Sea Ice | Monthly |
siu | X-Component of Sea-Ice Velocity | Monthly |
siv | Y-Component of Sea-Ice Velocity | Monthly |
sndmassmelt | Snow Mass Rate of Change Through Melt | Monthly |
sndmasssi | Snow Mass Rate of Change Through Snow-to-Ice Conversion | Monthly |
sndmasssnf | Snow Mass Change Through Snowfall | Monthly |
Table5. Sea ice model output variables from FGOALS-g3 for the ScenarioMIP experiments.
We used the following observational datasets for the model validation: Global Precipitation Climatology Project (GPCP, version 2.3) monthly data (Adler et al., 2003), HadCRUT4 monthly mean near-surface temperatures (Morice et al., 2012), China Merged Surface Temperature data (Yun et al., 2019), and the Arctic and Antarctic sea ice area records provided by the National Snow and Ice Data Center (NSIDC; http://nsidc.org/arcticseaicenews/sea-ice-tools/). The ensemble means from the historical runs (six members) and Tier 1 SSP experiments (see Table 1 for ensemble sizes) were used in our analysis. The base period for each anomaly analysis was 1980–2009.
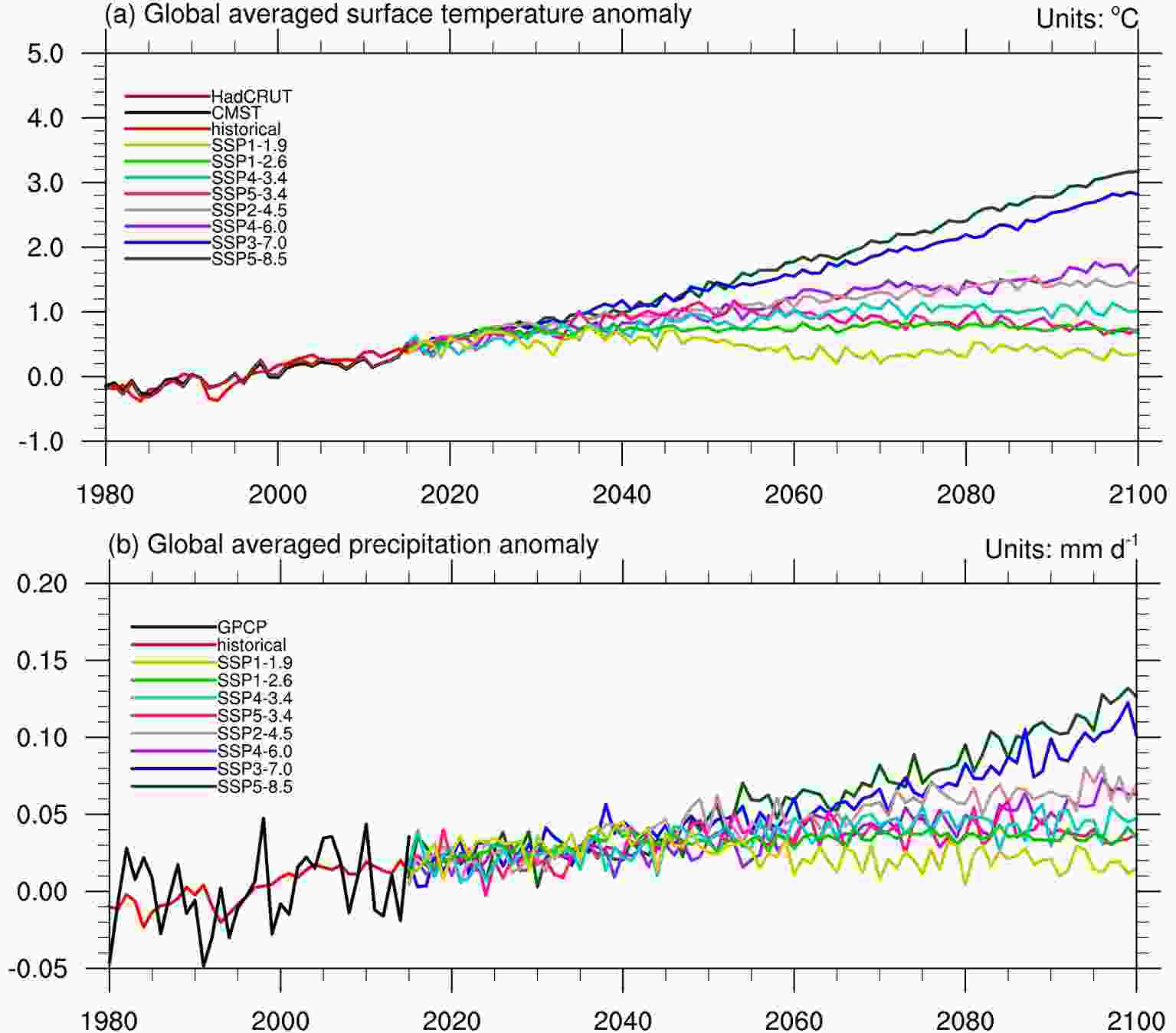
During the period 1980–2016, the observed global precipitation (GPCP) follows an increasing trend but with large annual fluctuations (Fig. 1b). The FGOALS-g3 model captures this increasing trend, but with less pronounced annual fluctuations. This is reasonable because the result of FGOALS-g3 is the ensemble mean, which smooths some model internal variability. Under all scenarios, the precipitation increases until 2050 when precipitation variability increases and results diverge among the scenarios, as was the case for the surface temperature trends (Fig. 1a). For SSP1-1.9, the precipitation decreases after 2050, and eventually returns to the values of the 2000s and 2010s. For SSP4-3.4 and SSP5-3.4-over, the increasing tends are not significant. By 2100, the anomaly reaches 0.06 mm d?1 for scenarios SSP2-4.5 and SSP4-6.0, and exceeds 0.10 mm d?1 for scenarios SSP3-7.0 and SSP5-8.5.
Consistent with the results shown in Fig. 1a, the annual mean global surface temperature follows an overall increasing trend over the period 2070–99 relative to the base period, as radiative forcing increases. However, large spatial discrepancies for the same emission and land-use scenarios exist in the simulations from each experiment (Fig. 2). In general, the surface temperature over the Arctic and high-latitude regions of the NH presents the strongest warming signals, with amplitudes of 1.0°C to >5.0°C for the SSP1-1.9 to SSP5-8.5 scenarios. The surface warming over the continents is generally higher than over the oceans, particularly for the Tibetan and Brazilian plateaus. Although ocean surface warming remains relatively weak, the equatorial East–Central Pacific shows an El Ni?o-like warm tongue for SSP4-3.4 and the last four scenario simulations (Figs. 2c and e–h). The differences in regional patterns of warming are consistent with expectations and previous results. Note that although a global warming trend exists under each scenario, the North Atlantic Ocean is an exception. The northwest–southeast belt-shaped “warm hole” (i.e., cooling anomaly) in this region even strengthens with increased radiative forcing and reaches ?5.0°C for SSP4-6.0 (Fig. 2f). This phenomenon has been observed in other simulation studies (Gervais et al., 2019), and may be related to Arctic sea ice melting and resulting changes to ocean circulation patterns, such as the Atlantic Meridional Overturning Circulation (AMOC). We will describe them in the following contents.

The projected annual mean precipitation shows large variations in the tropical and subtropical regions of both the NH and SH (Fig. 3). Between 2070 and 2099, there is a narrow quasi-east–west belt of increased rainfall over the equatorial Pacific with large amounts of precipitation to the east of the Maritime Continent. The positive rainfall anomalies increase from 0.5 mm d?1 to >3.0 mm d?1 as the scenario varies from lower to higher future forcing. In contrast, the tropical Indian Ocean, subtropical southwestern Pacific, tropical western Atlantic, and northern South America all show decreases in rainfall of ?0.5 to ?1.5 mm d?1. Rainfall anomalies present an obvious dipole feature in the tropical Indian ocean in all scenarios. Lower rainfall intensities in these regions are associated with greater radiative forcing.
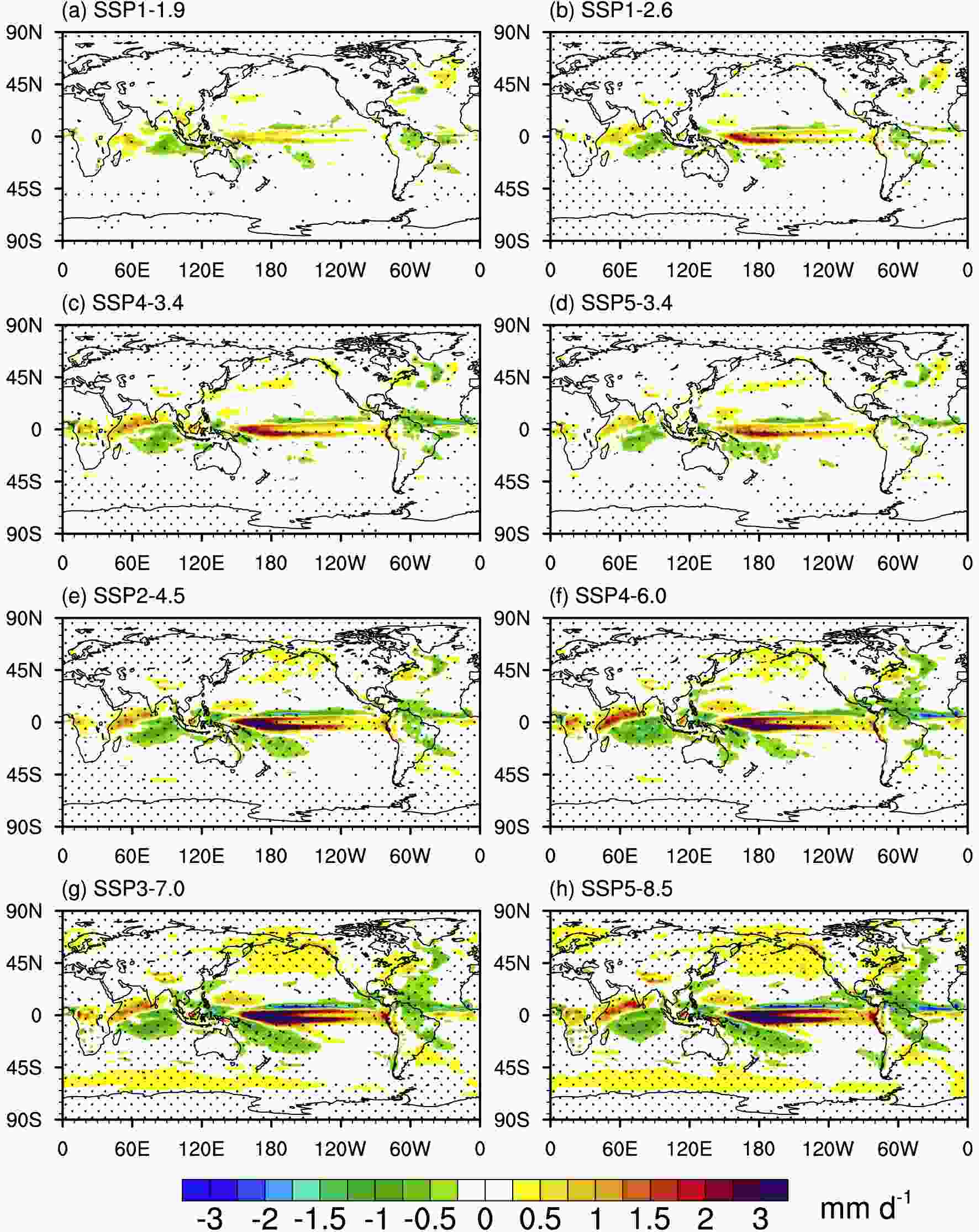
The spatial distributions of winter snow cover over the NH for the period 2070–2099 relative to the base period for eight scenarios are shown in Fig. 4. Clear negative anomalies are evident in the NH under the various emission scenarios. Western Europe and southern North America experience the most significant decrease. With increasing carbon dioxide concentrations and anthropogenic radiative forcing, these negative anomalies grow. For SSP3-7.0 and SSP5-8.5, most areas north of 30°N show negative anomalies, with values less than ?0.2 over Eurasia and North America (Figs. 4g and h). Results suggest that to maintain snow cover over the NH, it will be important to control greenhouse gas emissions in the future.

The AMOC plays an important role in regulating the climate by transporting heat northward in the Atlantic and thus maintaining the warmth of the NH. The annual mean maximum volume transport stream function at 26.5°N [units: Sverdrups (Sv)] in the Atlantic is used to measure the intensity of the AMOC. The historical and eight scenario simulations of the AMOC are shown in Fig. 5. From 1980 to 2015, the simulated AMOC from historical runs maintains an intensity of approximately 27.0 Sv, with a weak increase during the 1980s and a decrease in the early 1990s. Similar to surface temperature, the projected AMOC shows an overall weakening trend from 2015 to 2100 for SSP2-4.5, SSP4-6.0, SSP3-7.0, and SSP5-8.5. By 2100, the AMOC shows a decrease in intensity of 26% (37%) for SSP2-4.5 and SSP4-6.0 (SSP3-7.0 and SSP5-8.5). Due to the internal variability of AMOC, the regulation of deep water formation in the Greenland–Iceland–Norwegian Seas and Arctic sea ice melting, the projected AMOC shows large fluctuations under small-to-medium radiative forcing scenarios. This is similar to the results of FGOALS-g2 (Huang et al., 2014). For example, in the 2090s, the AMOC exhibits a strong rebound with a 4% intensity increase relative to 2014 values for SSP1-1.9. For SSP1-2.6, SSP4-3.4, and SSP5-3.4-over, the AMOC also rebounds, but to a lesser extent than for SSP1-1.9.

Figure 6 presents the sea ice area (SIA) anomaly time series over both hemispheres for the NSIDC observations, the historical runs, and the eight ScenarioMIP experiments. Overall, the variation of the SIA over the SH is greater than in the NH in both observations and simulations. The observed SIA over the NH first rises at the end of the 1980s and then gradually decreases over the subsequent 30 years (Fig. 6a). In contrast, the SIA over the SH has continuously increased, with relatively large annual variations, since 1980, and reached a peak around 2014 before decreasing sharply to its lowest point in 2017. The SIA anomalies associated with the historical runs over the NH are more consistent with the observations (i.e., they follow a decreasing trend), but show large discrepancies over the SH, especially between 2008 and 2014 (Fig. 6b). The rate of projected SIA decay over the NH is the largest for SSP5-8.5, followed by SSP3-7.0. The projected SIA over the NH is constant for SSP1-1.9, SSP1-2.6, SSP4-3.4, and SSP5-3.4-over, and even increases in the mid-21st century for SSP1-1.9. Although the SIA over the SH exhibits similar variations to those over the NH for each SSP, the decay rate decreases (e.g., for SSP5-8.5 and SSP3-7.0) and the amplitude of the annual fluctuations increases for all ScenarioMIP experiments.
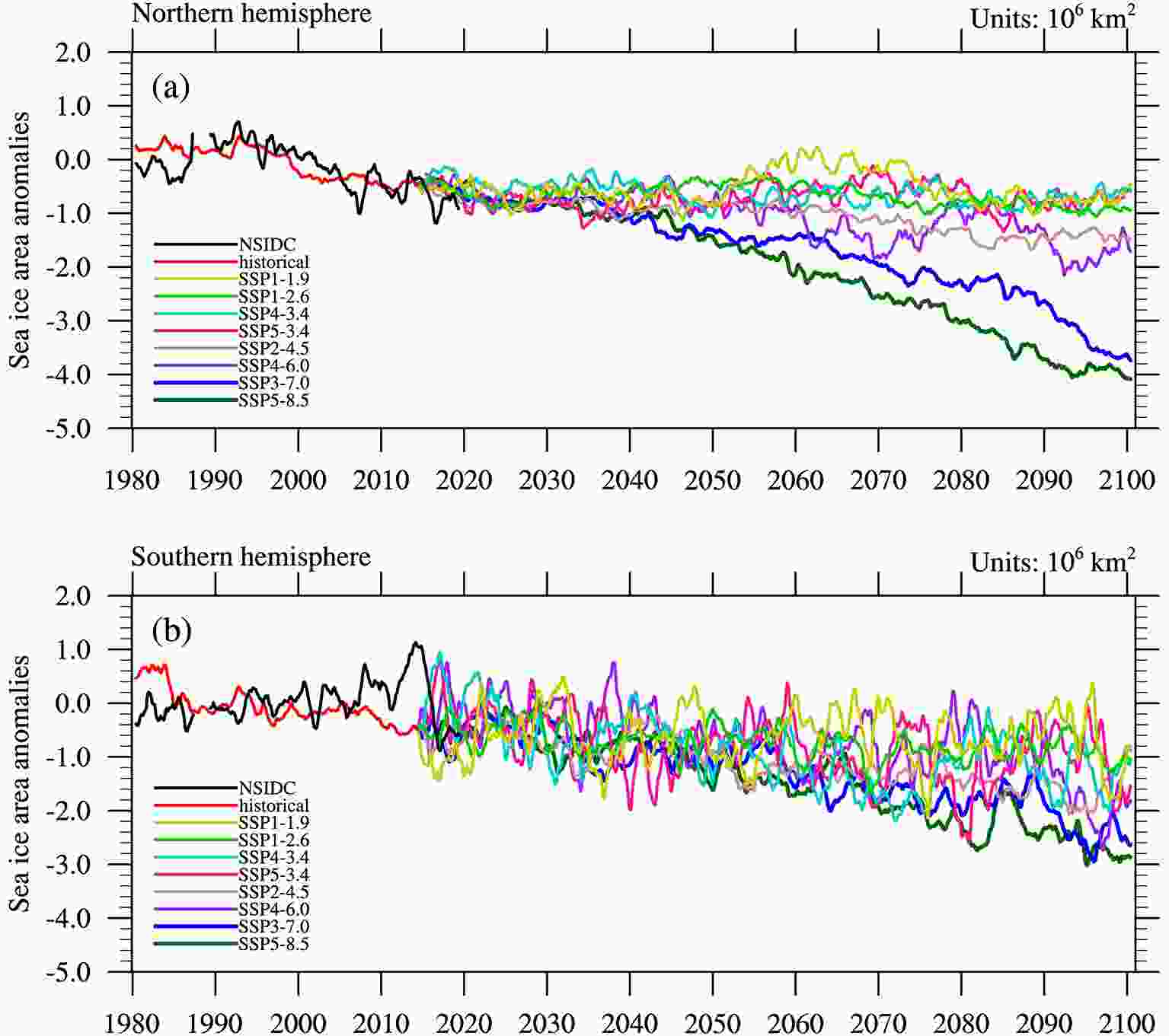
Acknowledgements. This study was supported by the National Key Research and Development Program of China (Grant Nos. 2017YFA0603903, 2017YFA0603901, and 2017YFA0603902), the Strategic Priority Research Program of Chinese Academy of Sciences (Grant No. XDB42010404) and the National Basic Research (973) Program of China (Grant Nos. 2015CB954102).
Open Access This article is distributed under the terms of the Creative Commons Attribution 4.0 International License (