
天津工业大学经济与管理学院, 天津 300387
收稿日期:
2021-01-12发布日期:
2021-07-22通讯作者:
李稚E-mail:lizhi@tiangong.edu.cn基金资助:
国家自然科学基金青年项目(72002153);全国统计科学研究项目(2019LY41);国家自然科学基金面上项目(41971249)The marketing strategy of online video based on danmaku-video: A bimodal analysis
LI Zhi(
School of economics and management, Tiangong university, Tianjin 300387, China
Received:
2021-01-12Published:
2021-07-22Contact:
LI Zhi E-mail:lizhi@tiangong.edu.cn摘要/Abstract
摘要: 随着互联网飞跃发展, 弹幕视频应运而生。这种新型的用户与视频交互方式具有新特性, 如用户情感表达实时动态性、情感分布多峰性。同时, 新特性也给实际研究工作带来挑战, 如用户画像刻画难度增大, 视频推荐和广告推送所需精度提高。现有研究尚未对弹幕视频的新特性进行深入分析, 也没有充分挖掘其本身所蕴含的学术研究价值。本文利用深度学习、自然语言处理技术、系统动力学方法, 结合心理学、市场营销学等多学科交叉前沿知识, 从数据驱动角度对弹幕视频数据进行分析和建模, 深度挖掘视频大数据潜在的商业价值。重点研究弹幕与视频双模态融合的情感识别方法; 构建带有用户情感特征的动态用户画像, 并建立基于用户画像的网络视频粘性营销机制; 分析用户情感与视频广告插播方式的相关性, 提出视频广告动态插播策略。丰富现有研究的同时, 为网络视频企业准确定位与分析用户需求, 创建高品质的智能营销平台供理论与决策支持。
图/表 10
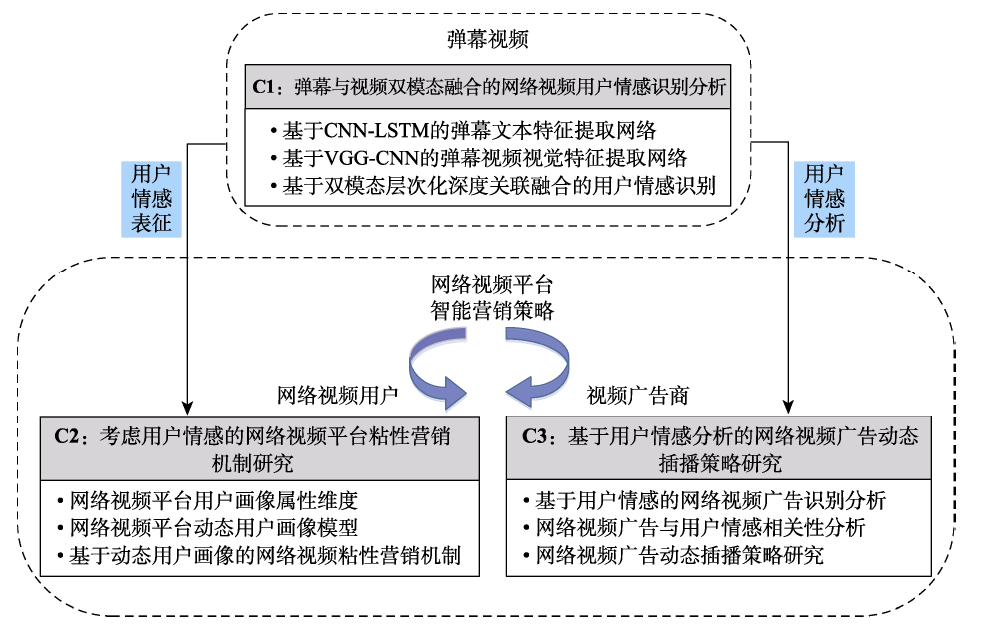
图1本文研究内容框架图
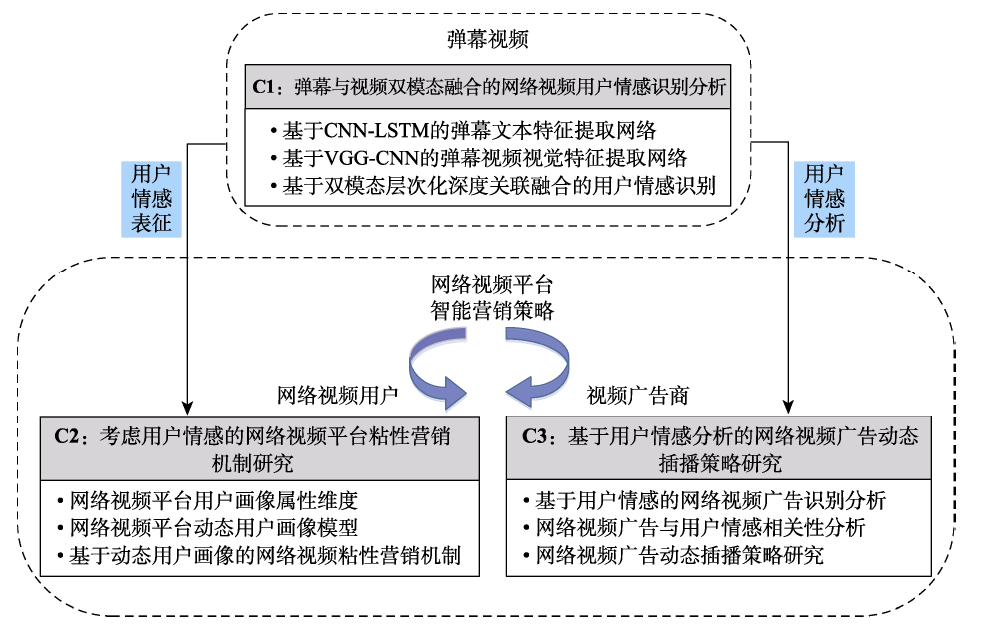

图2弹幕文本模态特征提取网络图

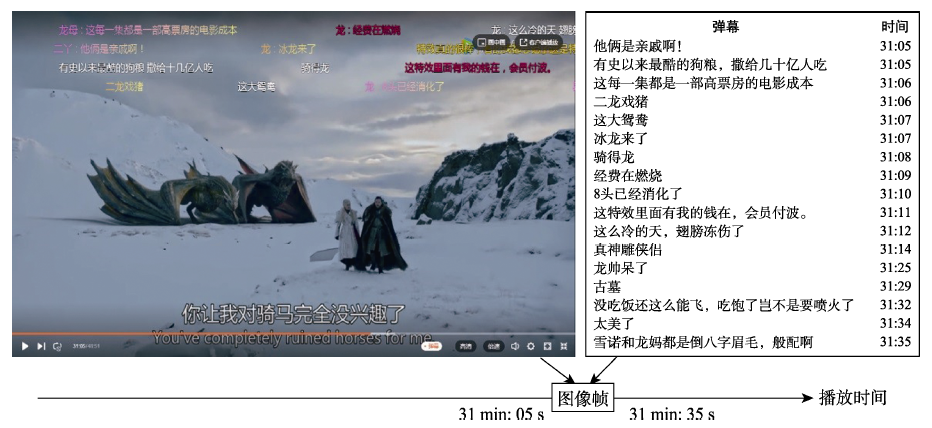
图3弹幕评论与视频画面相关性示意图
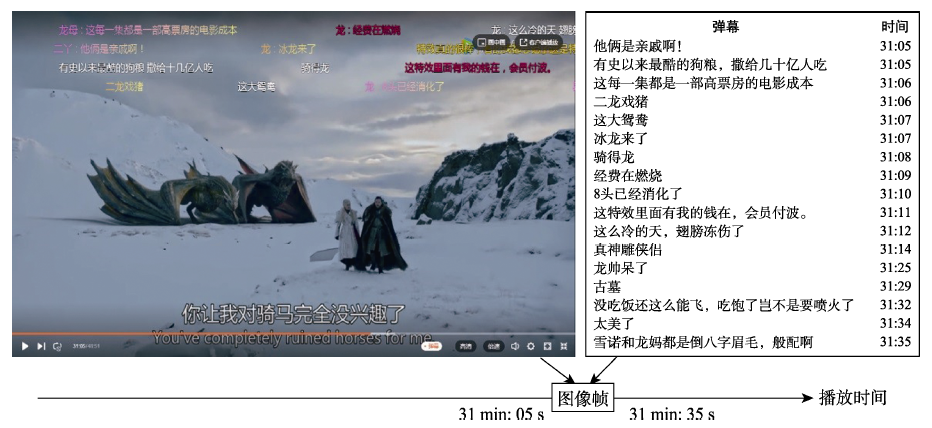
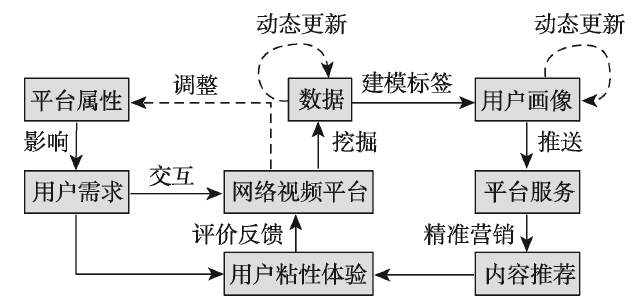
图4网络视频平台粘性营销机制模型
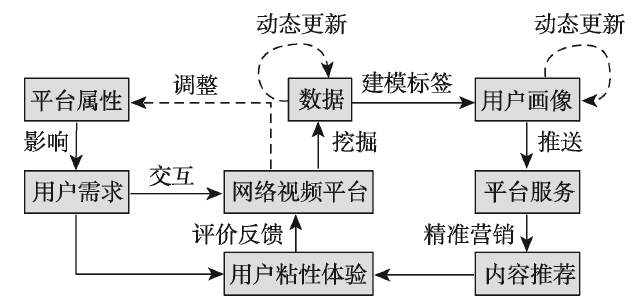
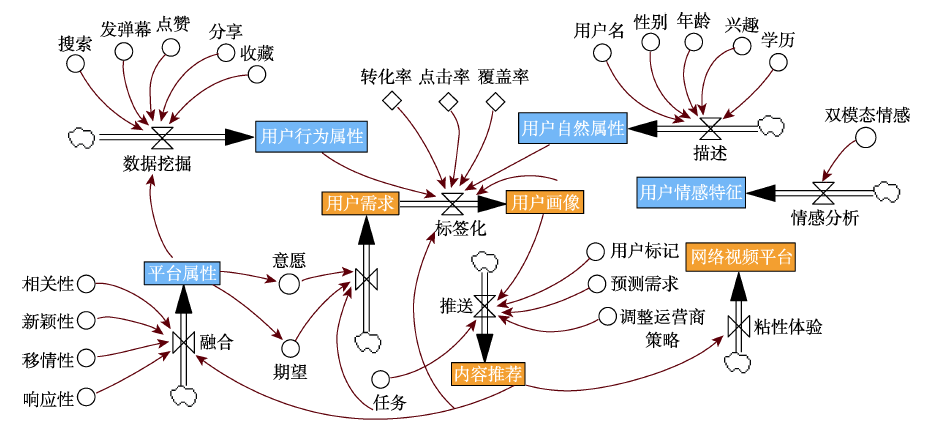
图5基于动态用户画像的网络视频平台粘性营销机制模型
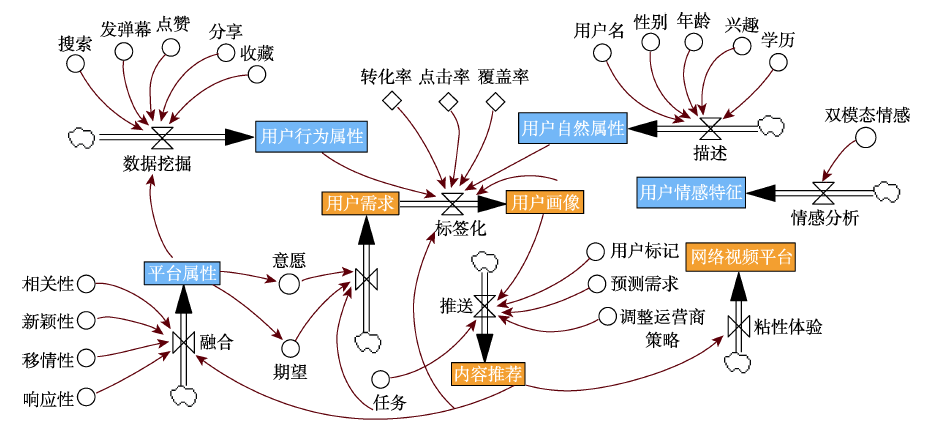
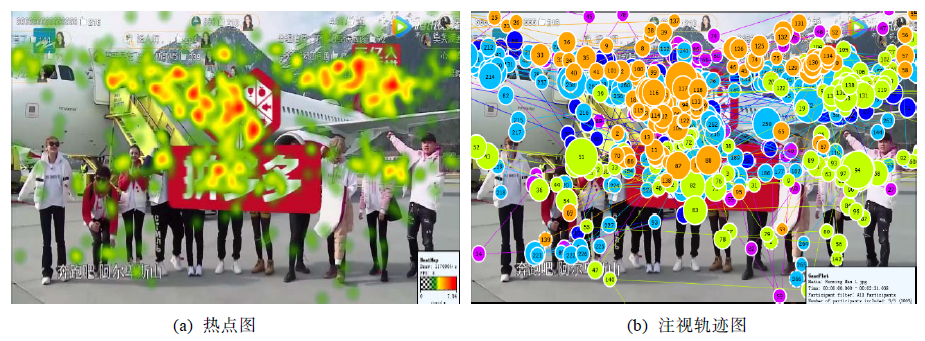
图6“拼多多”广告眼动实验结果图
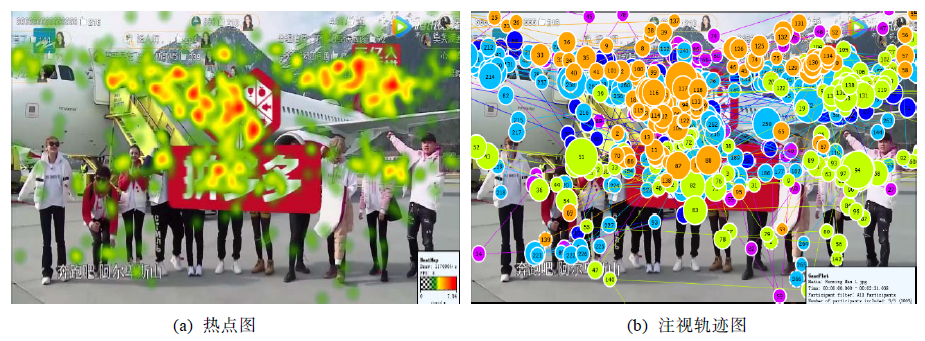
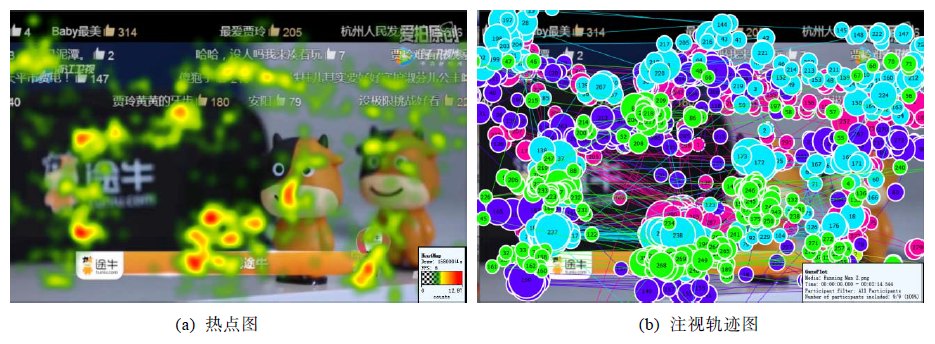
图7“途牛”广告眼动实验结果图
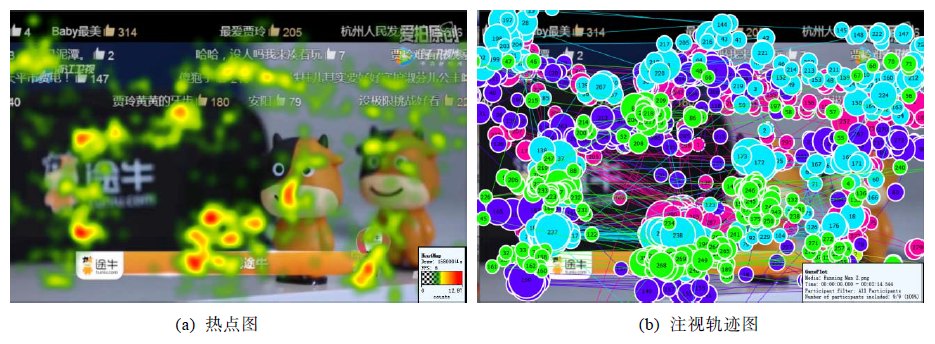

图8弹幕文本与广告品牌的一致性示意图

表1网络视频主题分类——广告类型归纳
主题 | 节目举例 | 广告类型 |
---|---|---|
综艺 | 奇葩说第六集(爱奇艺) | 口播广告、视频浮层广告 |
演员请就位(腾讯) | 口播广告、视频浮层广告、创意中插广告、中播广告 | |
明星大侦探5(芒果TV) | 口播广告、创意中插广告、视频浮层广告 | |
电视剧 | 小欢喜(爱奇艺) | 创意中插广告、中播广告、视频浮层广告 |
三生三世十里桃花(腾讯) | 中播广告、创意中插广告 | |
海棠经雨胭脂透(芒果TV) | 中播广告、视频浮层广告 | |
电影 | 狙击手(爱奇艺) | 中播广告 |
中国机长(腾讯) | ||
动漫 | 海贼王(爱奇艺) | 中播广告、视频浮层广告 |
秦时明月之君临天下(优酷) | 中播广告、视频浮层广告、创意中插广告 | |
名侦探柯南(芒果TV) | 视频浮层广告 | |
体育 | 2019ATP男单总决赛—蒂姆VS贝雷蒂尼(爱奇艺) | 中播广告、口播广告 |
NBA凯尔特人VS勇士(腾讯) | 中播广告 | |
CBA 辽宁本钢VS八一(优酷) | 视频浮层广告 |
表1网络视频主题分类——广告类型归纳
主题 | 节目举例 | 广告类型 |
---|---|---|
综艺 | 奇葩说第六集(爱奇艺) | 口播广告、视频浮层广告 |
演员请就位(腾讯) | 口播广告、视频浮层广告、创意中插广告、中播广告 | |
明星大侦探5(芒果TV) | 口播广告、创意中插广告、视频浮层广告 | |
电视剧 | 小欢喜(爱奇艺) | 创意中插广告、中播广告、视频浮层广告 |
三生三世十里桃花(腾讯) | 中播广告、创意中插广告 | |
海棠经雨胭脂透(芒果TV) | 中播广告、视频浮层广告 | |
电影 | 狙击手(爱奇艺) | 中播广告 |
中国机长(腾讯) | ||
动漫 | 海贼王(爱奇艺) | 中播广告、视频浮层广告 |
秦时明月之君临天下(优酷) | 中播广告、视频浮层广告、创意中插广告 | |
名侦探柯南(芒果TV) | 视频浮层广告 | |
体育 | 2019ATP男单总决赛—蒂姆VS贝雷蒂尼(爱奇艺) | 中播广告、口播广告 |
NBA凯尔特人VS勇士(腾讯) | 中播广告 | |
CBA 辽宁本钢VS八一(优酷) | 视频浮层广告 |
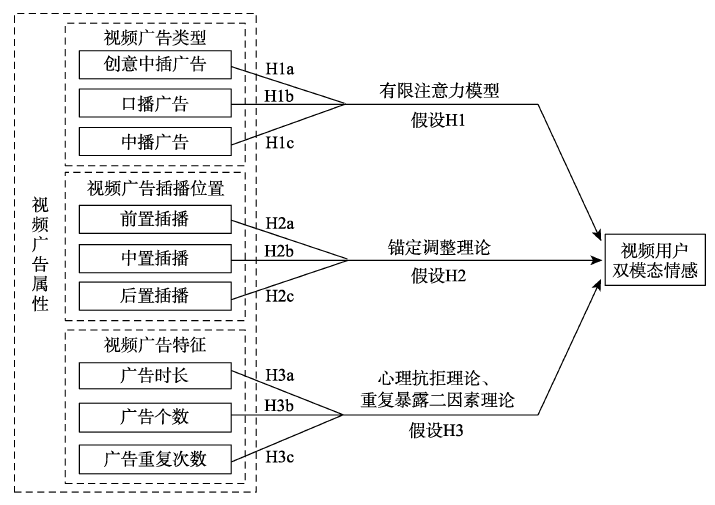
图9理论假设模型框架图
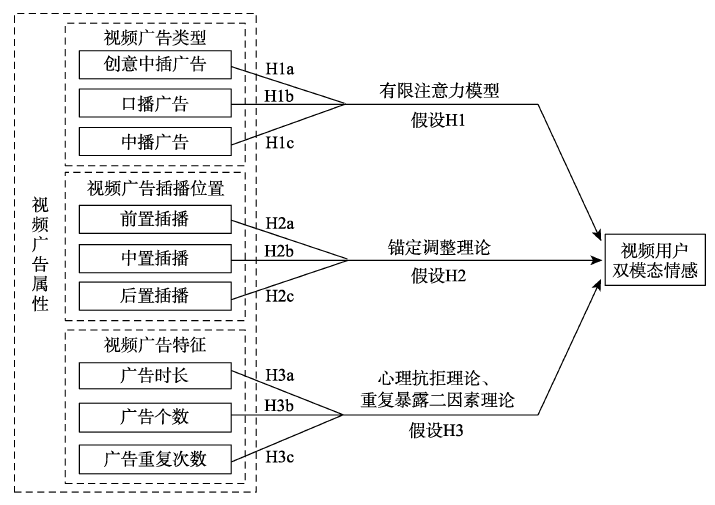
参考文献 71
[1] | 陈丹, 罗烨, 吴智勤. (2019). 基于大数据挖掘和用户画像的高校图书馆个性化服务研究. 图书馆研究与工作, 4, 50-59. |
[2] | 邓扬, 张晨曦, 李江峰. (2017). 基于弹幕情感分析的视频片段推荐模型. 计算机应用, 37(4), 1065-1070. |
[3] | 范钧, 潘健军. (2016). 剧情式视频广告中品牌—情节信息对受众传播意愿的影响. 营销科学学报, 12(2), 111-123. |
[4] | 高茂庭, 杨涛. (2020). 融合协同过滤和主题模型的弹幕视频推荐算法. 计算机应用研究, 37(12), 3565-3568+ 3577. |
[5] | 龚诗阳, 李倩, 余承锬. (2017). 在线社交对消费者需求的影响研究—基于网络视频产业的实证分析. 中国软科学, 7, 39-48. |
[6] | 郭伏, 叶国全, 李明明, 吕伟. (2017). 植入式广告显著度对广告效果影响的眼动研究—以电影植入式广告为例. 营销科学学报, 4, 18-33. |
[7] | 洪庆, 王思尧, 赵钦佩, 李江峰, 饶卫雄. (2018). 基于弹幕情感分析和聚类算法的视频用户群体分类. 计算机工程与科学, 40(6), 1125-1139. |
[8] | 勒伯夫 G.(2012). 粘性营销: 新时代的营销圣经(派力译译). 北京: 中国商业出版社. |
[9] | 李泽中, 张海涛, 张鑫蕊, 王兴鲁, 孙鸿飞. (2020). 融合用户社交与情境信息的虚拟知识社区个性化知识推荐研究. 情报理论与实践, 43(4), 152-158 |
[10] | 刘海鸥, 刘旭, 姚苏梅. 谢姝琳. (2019). 基于舆情画像的在线社交用户信息传播特征统计分析. 现代情报, 39(9):64-73. |
[11] | 刘启元, 张栋, 吴良庆, 李寿山. (2019). 基于上下文增强LSTM的多模态情感分析. 计算机科学, 46(11), 181-185. |
[12] | 刘婷艳, 王晰巍, 贾若男, 王微. (2020). 视频网站用户生成内容国内外发展动态及发展趋势. 情报科学, 38(10), 133-140. |
[13] | 王敏, 徐健. (2019). 视频弹幕与字幕的情感分析与比较研究. 图书情报知识, 5, 109-119. |
[14] | 王贇芝, 王雪, 查先进. (2020). 弹幕视频网站用户从众信息评论行为驱动因素探索. 信息资源管理学报, 10(4), 60-69. |
[15] | 吴剑云, 胥明珠. (2021). 基于用户画像和视频兴趣标签的个性化推荐. 情报科学, 39(1), 128-134. |
[16] | 徐海玲, 张海涛, 魏明珠, 尹慧子. (2019). 社交媒体用户画像的构建及资源聚合模型研究. 图书情报工作, 63(9), 109-115. |
[17] | 俞淑平, 陈刚. (2011). 一种高效的行为定向广告投放算法. 计算机应用与软件, 28(1), 4-7. |
[18] | 袁海霞, 方青青, 白琳. (2020). 弹幕对在线消费行为过程影响的时变效应研究. 管理学报, 17(7), 1059-1066. |
[19] | 张海涛, 崔阳, 王丹, 宋拓. (2018). 基于概念格的在线健康社区用户画像研究. 情报学报, 37(9), 912-922. |
[20] | 张帅, 王文韬, 周华任, 占南. (2018). 基于扎根理论的弹幕视频网站用户使用行为驱动因素研究. 情报理论与实践, 41(7), 117-122. |
[21] | 张涛, 翁康年, 邓悦, 杨满, 张玥杰. (2021). 基于网络浏览行为的小众领域用户画像建模. 系统工程理论与实践, 40(3), 641-652. |
[22] | 庄须强, 刘方爱. (2018). 基于AT-LSTM的弹幕评论情感分析. 数字技术与应用, 36(2), 210-212. |
[23] | Bansal T., Das M., & Bhattacharyya C. (2015). Content driven user profiling for comment-worthy recommendations of news and blog articles. ACM Conference on Recommender Systems, 195-202. |
[24] | Bao L. N., Le D.-N., van Chung L., & Nguyen G. N.(2016). Performance evaluation of video-based face recognition approaches for online video contextual advertisement user-oriented system. Proceedings paper: Information Systems Design and Intelligent Applications Conference, 3, 287-295. |
[25] | Barnett S. B., & Cerf M. (2017). A ticket for your thoughts: Method for predicting content recall and sales using neural similarity of moviegoers. Journal of Consumer Research, 44(1):160-181. |
[26] | Belanche D., Flavián C., & Pérez-Rueda A. (2017). Understanding interactive online advertising: congruence and product involvement in highly and lowly arousing, skippable video ads. Journal of Interactive Marketing, 37, 75-88. doi: 10.1016/j.intmar.2016.06.004URL |
[27] | Bellman S., Nenycz-Thiel M., Kennedy R., Larguinat L., McColl B., & Varan D. (2017). What makes a television commercial sell? Using biometrics to identify successful ads. Journal of Advertising Research, 57(1), 53-66. doi: 10.2501/JAR-2016-051URL |
[28] | Bendak S., & Al-Saleh K. (2010). The role of roadside advertising signs in distracting drivers. International Journal of Industrial Ergonomics, 40(3), 233-236. doi: 10.1016/j.ergon.2009.12.001URL |
[29] | Berlyne D. E. (1970). Novelty, complexity, and hedonic value. Perception & Psychophysics, 8, 279-286. doi: 10.3758/BF03212593URL |
[30] | Brehm J. W. A. (1966). A theory of psychological reactance. New York: Academic Press Inc. |
[31] | Bruce N. I., BeckerM, & Reinartz W. (2020). Communicating brands in television advertising. Journal of Marketing Research, 57(2), 236-256. doi: 10.1177/0022243719892576URL |
[32] | Chen X., Nguyen T. V., Shen Z. Q., & Kankanhalli M. (2019). Livesense:Contextual advertising in live streaming videos. Proceedings paper of 27th ACM International Conference on Multimedia (MM), 19, 392-400. |
[33] | Cheng Z. -Q., Liu Y., Wu X., & Hua X. -S. (2016). Video ecommerce: Towards online video advertising. Proceedings of the 24th ACM Multimedia Conference, 16, 1365-1374. |
[34] | Chiang H. S., & Hsiao K. L. (2015). Youtube stickiness: The needs, personal, and environmental perspective. Internet Research, 25(1), 85-106. doi: 10.1108/IntR-11-2013-0236URL |
[35] | CostaM, BonettiL, Vignali V., Bichicchi A., Lantieri C., & Simone A. (2019). Driver's visual attention to different categories of roadside advertising signs. Applied Ergonomics, 78, 127-136. doi: 10.1016/j.apergo.2019.03.001URL |
[36] | Fossen B. L., & Schweidel D. A. (2017). Television advertising and online word-of-mouth: An empirical investigation of social TV activity. Marketing Science, 36(1), 105-123. doi: 10.1287/mksc.2016.1002URL |
[37] | Gorodnichenko Y., & Weber M. (2016). Are sticky prices costly? Evidence from the stock market. American Economic Review, 106(1), 165-199. |
[38] | He M., Ge Y., WuL. Chen E., & Tan C. (2016). Predicting the popularity of Dan Mu-enabled videos: A multi-factor view. Database Systems for Advanced Applications, 351-366. |
[39] | Hu A., & Flaxman S. (2018). Multimodal sentiment analysis to explore the structure of emotions. Proceedings of the 24th ACM SIGKDD International Conference on Knowledge Discovery and Data Mining, KDD, 350-358. |
[40] | Huang F., Zhang X., Zhao Z., Xu J., & Li Z. (2019). Image-text sentiment analysis via deep multimodal attentive fusion. Knowledge-Based Systems, 167, 26-37. doi: 10.1016/j.knosys.2019.01.019URL |
[41] | Hui S. K., Meyvis T., & Assael H. (2014). Analyzing moment-to-moment data using a bayesian functional linear model: Application to TV show pilot testing. Marketing Science, 33(2), 222-240. doi: 10.1287/mksc.2013.0835URL |
[42] | Hogarth R. M., & Einhorn H. J. (1992). Order effects in belief updating the belief adjustment model. Cognitive Psychology, 24(1), 1-55. doi: 10.1016/0010-0285(92)90002-JURL |
[43] | Kim H., & Bruce N. I. (2018). Should sequels differ from original movies in pre-launch advertising schedule? Lessons from consumers’ online search activity. International Journal of Research in Marketing, 35(1), 116-143. doi: 10.1016/j.ijresmar.2017.12.006URL |
[44] | Kumar A., Srinivasan K., Cheng W. -H., & Zomaya A. Y. (2020). Hybrid context enriched deep learning model for fine-grained sentiment analysis in textual and visual semiotic modality social data. Information Processing & Management, 57(1), 1-25. |
[45] | Lau R. Y. K., Zhang W., & Xu W. (2018). Parallel aspect-oriented sentiment analysis for sales forecasting with big data. Production and Operations Management, 27(10), 1775-1794. doi: 10.1111/poms.2018.27.issue-10URL |
[46] | Li D. F., Hu B. T., Chen Q. C., Wang X., Qi Q., Wang L., & Liu H. (2021). Attentive capsule network for click-through rate and conversion rate prediction in online advertising. Knowledge-Based Systems, 211, 106522. doi: 10.1016/j.knosys.2020.106522URL |
[47] | Li G., Park S. T., & Jin H. (2017). Antecedents of WeChat group chatting user’s stickiness and organizational commitment. Journal of Engineering and Applied Sciences, 12(1), 5708-5713. |
[48] | Li Z., Li R., & Jin G. (2020). Sentiment analysis of danmaku videos based on naïve bayes and sentiment dictionary. IEEE Access, 5, 75073-75084. |
[49] | Liu D. -., Liao Y. -., Chung Y. -., & Chen K. -Y. (2019). Advertisement recommendation based on personal interests and ad push fairness. Kybernetes, 48(8), 1-21. |
[50] | Lu H. -P., & Lee M. -R. (2010). Demographic differences and the antecedents of blog stickiness. Online Information Review, 34 (1), 21-38. doi: 10.1108/14684521011024100URL |
[51] | Lv G., Xu T., Chen E., Liu Q., & Zheng Y. (2016). Reading the videos:temporal labeling for crowdsourced time-sync videos based on semantic embedding. Thirtieth AAAI Conference on Artificial Intelligence, 3000-3006. |
[52] | Majumder N., Hazarika D., Gelbukh A., Cambria E., & Poria S. (2018). Multimodal sentiment analysis using hierarchical fusion with context modeling. Knowledge- Based Systems, 161, 124-133. doi: 10.1016/j.knosys.2018.07.041URL |
[53] | Olbrich R., & Holsing C. (2011). Modeling consumer purchasing behavior in social shopping communities with clickstream data. International Journal of Electronic Commerce, 16(2), 15-40. doi: 10.2753/JEC1086-4415160202URL |
[54] | Peng D., Tian W., Zhu M., Ren Y., Lin X., & Li M. (2020). TargetingVis: Visual exploration and analysis of targeted advertising data. Journal of Visualization, 23(6), 1113-1127. doi: 10.1007/s12650-020-00671-wURL |
[55] | Piao G., & Breslin J. G. (2016). Exploring dynamics and semantics of user interests for user modeling on Twitter for link recommendations. ACM International conference on semantic systems, 81-88. |
[56] | Ramanathan S., & McGill A. L. (2007). Consuming with others: Social influences on moment-to-moment and retrospective evaluations of an experience. Journal of Consumer Research, 34(4), 506-524. doi: 10.1086/520074URL |
[57] | Rong K., Xiao F., Zhang X., & Wang J. (2019). Platform strategies and user stickiness in the online video industry. Technological Forecasting & Social Change, 143, 249-259. |
[58] | ,, & Sánchez P., & Bellogín A.(2019). Building user profiles based on sequences for content and collaborative filtering. Information Processing & Management, 56(1), 192-211. doi: 10.1016/j.ipm.2018.10.003URL |
[59] | Simmonds L., Bellman S., Kennedy R., Nenycz-Thiel M., & Bogomolova S. (2020). Moderating effects of prior brand usage on visual attention to video advertising and recall: An eye-tracking investigation. Journal of Business Research, 111, 241-248. doi: 10.1016/j.jbusres.2019.02.062URL |
[60] | Skehan P. (1996). A Framework for the Implementation of task-based instruction. Applied Linguistics, 17(1), 38-62. doi: 10.1093/applin/17.1.38URL |
[61] | Sun Q., Niu J., Yao Z., & Yan H. (2019). Exploring eWOM in online customer reviews: Sentiment analysis at a fine-grained level. Engineering Applications of Artificial Intelligence, 81, 68-78. doi: 10.1016/j.engappai.2019.02.004URL |
[62] | Teixeira T. S., Wedel M., & Pieters R. (2010). Moment- to-moment optimal branding in TV commercials: Preventing avoidance by pulsing. Marketing Science, 29(5), 783-804. doi: 10.1287/mksc.1100.0567URL |
[63] | Thompson J., Leung B., Blair M. R., & Taboada M. (2017). Sentiment analysis of player chat messaging in the video game StarCraft 2: Extending a lexicon-based model. Knowledge-Based Systems, 137, 149-162. doi: 10.1016/j.knosys.2017.09.022URL |
[64] | Travis D. (2003). E-commerce usability: Tools and techniques to perfect the on-line experience. CRC Press, London. |
[65] | Trusov M., Ma L., & Jamal Z. (2016). Crumbs of the cookie: User profiling in customer-base analysis and behavioral targeting. Marketing Science, 35(3), 405-426. doi: 10.1287/mksc.2015.0956URL |
[66] | Wang G., Zhuo L., Li J., Ren D., & Zhang J. (2018). An efficient method of content-targeted online video advertising. Journal of Visual Communication and Image Representation, 50, 40-48. doi: 10.1016/j.jvcir.2017.11.001URL |
[67] | Young C., Gillespie B., & Otto C. (2019). The impact of rational, emotional, and physiological advertising images on purchase intention: how TV ads influence brand memory. Journal of Advertising Research, 59(3), 329-341. doi: 10.2501/JAR-2019-010URL |
[68] | Yu S., Christakopoulou E., & Gupta A. (2016). Identifying decision makers from professional social networks. ACM SIGKDD International Conference on Knowledge Discovery and Data mining, New York: ACM, 333-342. |
[69] | Zhang Q., Wang W., & Chen Y. (2020). Frontiers: In-consumption social listening with moment-to-moment unstructured data: The case of movie appreciation and live comments. Marketing Science, 39(2), 1-11. doi: 10.1287/mksc.2019.1205URL |
[70] | Zhao F., Zhu Y., Jin H., & Yang L. T. (2016). A personalized hashtag recommendation approach using LDA-based topic model in microblog environment. Future Generation Computer Systems, 65, 196-206. doi: 10.1016/j.future.2015.10.012URL |
[71] | Zhou J. L., Zhou J., Ding Y., & Wang H. (2019). The magic of danmaku: A social interaction perspective of gift sending on live streaming platforms. Electronic Commerce Research and Applications, 34, 1-9. |
相关文章 0
No related articles found! |
PDF全文下载地址:
http://journal.psych.ac.cn/xlkxjz/CN/article/downloadArticleFile.do?attachType=PDF&id=5567