

2.
Current Status and Prospects of Genomics Data Analysis Methods
Chen Meili1,#, Ma Yingke1,#, Li Rujiao

2.
通讯作者: * 李茹姣(lirj@big.ac.cn);鲍一明(baoym@big.ac.cn)
第一联系人:
收稿日期:2020-01-21网络出版日期:2020-04-20
基金资助: |
Received:2020-01-21Online:2020-04-20
作者简介 About authors
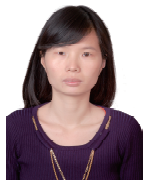
陈梅丽,中国科学院北京基因组研究所(国家生物信息中心),国家基因组科学数据中心,助理研究员,博士,主要从事基因组、转录组等组学数据整合和挖掘工作。
参与完成文献调研和论文撰写,与马英克贡献相同。
Chen Meili, PhD., is a research assistant of National Genomics Data Center, Beijing Institute of Genomics (China National Center for Bioinformation), Chinese Academy of Sciences. Her research interests include integration and data mining of genomics and transcriptomics data.
For this paper she surveyed the literatures and drafted the manuscript.She and Ma Yingke contributed equally.
E-mail:chenml@big.ac.cn
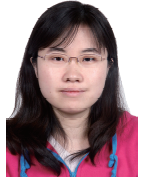
马英克,中国科学院北京基因组研究所(国家生物信息中心),国家基因组科学数据中心,助理研究员,博士,主要从事数据库开发、生物信息学软件开发相关研究。
参与完成文献调研和论文撰写,与陈梅丽贡献相同。
Ma Yingke, PhD., is a research assistant of National Genomics Data Center, Beijing Institute of Genomics (China National Center for Bioinformation), Chinese Academy of Sciences. Her research interests include database development and bioinformatics software development.
For this paper she surveyed the literatures and drafted the manuscript.She and ChenMeili contributed equally.
E-mail:mayk@big.ac.cn
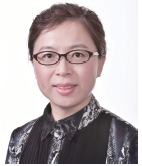
李茹姣,中国科学院北京基因组研究所(国家生物信息中心),国家基因组科学数据中心,高级工程师,博士,主要从事组学大数据整合和挖掘,2019年入选中国科学院关键技术人才。
参与完成文献调研和论文撰写,修改全文。
Li Rujiao, PhD., is a senior engineer of National Genomics Data Center, Beijing Institute of Genomics (China National Center for Bioinformation), Chinese Academy of Sciences. Her research interests include omics big data integration and mining. She was named one of 'CAS Key Technology Talent Program 2019'.
For this paper she surveyed the literatures, drafted and revised the manuscript. E-mail:lirj@big.ac.cn
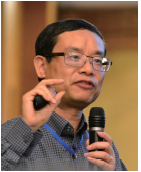
鲍一明,现任中国科学院北京基因组研究所(国家生物信息中心)国家基因组科学数据中心主任、研究员、博士生导师。主要从事生物大数据整合与信息挖掘、病毒基因组注释和病毒进化与分类等研究。于1987年获得北京大学生物化学专业学士学位,1994年于英国John Innes中心(通过East Anglia大学)获遗传学博士学位。现为中国科学院大学健康医疗大数据国家研究院副院长,中国生物工程学会计算生物学与生物信息学专委会委员。
参与文章整体构思和设计,修改全文。
Bao Yiming, is the director and professor of National Genomics Data Center, Beijing Institute of Genomics (China National Center for Bioinformation), Chinese Academy of Sciences. His research interests include biology big data integration and mining, virus genome annotation and virus evolution and classification. He received B.S. degree from Peking University, Beijing, China in 1987, and Ph.D. degree from John Innes Centre (through University of East Anglia), UK, in 1994. Currently Dr. Bao is the deputy director of National Institute of Data Science in Health and Medicine, University of Chinese Academy of Sciences and a member of computational biology and bioinformatics specialized committee, Chinese Society of Biotechnology.
For this paper he conceived , designed and revised the paper.E-mail:baoym@big.ac.cn

摘要
【目的】全面阐述基因组学数据分析方法的现状和未来发展趋势,为精准医学、精准育种、生物安全、生物多样性、分子进化等的相关组学数据分析算法的研究与工具开发提供参考。【结果】基因组学数据分析主要包括基因组、转录组、表观组数据分析,当前基因组学数据主要面临着海量、多维、异构等挑战。本文详细地阐述了基因组学数据分析算法和工具开发的现状、应用、存在的问题和面临的挑战。【结论】充分利用人工智能、统计模型、知识图谱等先进技术,不断地优化和开发更先进的算法和更鲁棒的模型,使其兼具高容错、高准确、高效、计算资源低耗等优点,匹配海量、多维、异构基因组学大数据分析的需求,是未来基因组学数据分析算法和工具开发的方向。
关键词:
Abstract
[Objective] Through a comprehensive review of the current status and future development of genomics data analysis methods, we provide suggestions for the improvement of algorithm and tool development of related omics data analysis in precision medicine, precision breeding, biosafety, biodiversity and molecular evolution. [Results] The analysis of genomics data mainly includes that of genomic, transcriptomic and epigenomic data. At present, the analysis of genomics data faces challenges primarily because the data are massive, multidimensional and heterogeneous. This review will elaborate on the current status, applications, challenges, and prospects of algorithm and tool development for genomics data analysis. [Conclusions] The future directions of algorithm and tool development for genomics data analysis are to make full use of advanced technologies such as artificial intelligence, statistical models, and knowledge graphs, and to continuously optimize and develop more advanced algorithms and robust models that are of error tolerance, high accuracy, and high efficiency with low cost of computing resources.
Keywords:
PDF (21169KB)元数据多维度评价相关文章导出EndNote|Ris|Bibtex收藏本文
本文引用格式
陈梅丽, 马英克, 李茹姣, 鲍一明. 基因组学数据分析方法现状和展望. 数据与计算发展前沿[J], 2020, 2(2): 1-19 doi:10.11871/jfdc.issn.2096-742X.2020.02.001
Chen Meili.
引言
随着人类基因组测序计划的完成,基因组学的影响力迅速扩大,数以万计的动物、植物、微生物基因组被组装[1,2,3,4],游离DNA应用于无创产前检测、通过基因检测指导靶向药物治疗成为可能[5,6]、单细胞技术应用于辅助生殖[7]、DNA编辑技术广泛应用[8]、体外提供造血干细胞[9]、长寿基因被找到[10]、抗病虫害和高产的农业作物新品种被不断培育[11,12]。这些基因组科学领域的巨大进展,一方面来自于测序和实验技术的革新,同时也依赖于为适应实验和测序技术进步而不断发展的分析手段和方法[4, 13-14]。基因组学测序数据增长迅猛,加快数据分析速度,提高数据处理效率,是对大数据整合分析工具和算法开发的迫切需求。如何用好多源海量基因组学数据,去除异质性,并对其进行整合分析和深度挖掘,是对数据分析工具和算法开发另一个层面的新要求。科学家们也在不断地开发各种算法和工具来提高计算效率,比如第三代测序数据组装算法wtdbg2,将拼接的分析速度提高5倍,少于数据产出时间[4]。而在此基础上,人工智能等先进技术也被广泛应用于基因组学大数据分析的工具开发[15]。本文将详细地阐述随着测序技术的发展,基因组、转录组、表观组数据的分析算法和工具开发的现状,以及大数据时代基因组学数据算法和工具开发在未来将面临的问题和挑战。
1 基因组测序数据分析
随着测序技术的发展,各类基因组相关研究计划接踵而来[16,17],为生物多样性、物种进化、分子育种、临床治疗等研究提供了宝贵的数据资源。基因组数据分析主要包括基因组组装、基因组注释、基因组变异分析等。基因组序列和注释信息包含了生物体的所有遗传信息和功能信息,是多种组学研究的重要基础数据[18]。通过基因组变异分析可以解析基因组变异对表型、物种进化、疾病等的影响[19]。但是测序数据分析时间往往远大于测序数据产出时间,无法匹配数据爆发式增长的趋势,是基因组数据分析面临的最大挑战之一。1.1 基因组组装
基因组组装是将测序产生的读段(read)片段经过序列组装成完整的基因组序列。基因组组装方法主要有两类:(1)基于参考基因组序列比对的有参组装,常用于重测序和线粒体/叶绿体等保守细胞器基因组[20,21];(2)从头(de novo)组装,目前主要有纯二代测序组装[22]、二代和三代测序混合组装[23]、纯三代测序组装[24]等组装策略。对于动植物等大基因组可以利用遗传图谱、Bionano光学图谱、Hi-C、10X Genomics等图谱信息进行整合辅助组装,将基因组组装提升到染色体级别[25,26,27,28]。动植物基因组因存在基因组大、杂合度高、GC含量高、重复序列多和多倍化水平较高等复杂因素,给组装带来了很大的挑战,需要开发出兼顾效率和计算资源消耗,并且在重复区域获得连续性和完整性都表现很好的高质量基因组组装结果的算法和工具。目前常用的软件有SOAPdenovo2[22]、ALLPATHS-LG[29]、Canu[30]、Falcon[31]等。为了解决大型基因组组装效率、准确性要求高和计算资源消耗大的问题,阮珏团队提出了三代数据组装wtdbg2算法,该算法遵循overlap-layout-consensus模式,以快速的读段“全部对全部”比对实现方式和基于模糊布鲁因图这种新组装图理论——一种与稀疏布鲁因图和其变体A-Bruijn图有关的序列组装的新数据结构,来改进现有的组装程序并提高组装效率[4]。在一台计算机上,可在2天内完成4个~30X的人类基因组数据集的组装,极大提高三代测序数据的分析效率。与此前提出的Flye算法[32]相比,wtdbg2的分析速度提升了5倍,内存使用仅为Flye的一半,组装连续性和精度可与Canu、Falcon、Flye等其他算法相媲美。wtdbg2首次将测序数据分析时间降低到少于测序数据产出时间,是一种兼具高容错和高准确的高效算法,并可扩展到超大基因组,如32 Gb大小的蝾螈基因组[4]。
当前Hi-C技术越来越多地应用于辅助染色体水平二倍体基因组组装[25],Hi-C技术是基于将线性距离远、空间结构近的DNA片段进行交联,并将交联的DNA片段富集后进行高通量测序,对测序数据分析揭示染色质的远程相互作用,明确基因组草图中scaffold和染色体对应关系、scaffold之间的连接顺序和方向,从而将scaffold挂载到染色体上。但是对于同源多倍体和近期加倍的异源多倍体来说,其同源染色体之间的Hi-C交联信号会将序列相似的等位片段连接在一起,导致同源染色体被错误地连接到一起,形成大量嵌合的组装,给组装造成了较大困难。针对该问题明瑞光团队提出了ALLHiC算法,该算法包括pruning、partition、rescue、optimization、building 5个步骤。ALLHiC算法一方面通过修剪Hi-C平行信号和弱信号进行等位基因分型,减少了同源染色体间的嵌合连接;另一方面通过遗传算法随机优化,极大地提高了contig序列的排序和定向准确性,成功解决了同源高倍体甘蔗、菠萝和异源四倍体栽培花生等多倍体组装难题[33,34]。
而针对于二倍体或者是多倍体,单体型基因组组装是基因组组装的最终目标,目前已提出单体型区块组装策略,如FALCON-Unzip[31]、trio binning[35]等方法,尝试将两个或多个配子来源的染色体进行分别组装。但是当前已有的单体型区块组装策略依然无法很好地解决单条染色体长度的单体型基因组组装问题。针对该问题,Kronenberg团队提出了一种单体型基因组组装的新技术FALCON-Phase,可把杂合基因组的父本母本分型组装,并可将其应用于野外采集的样本或缺乏谱系信息的生物。其主要原理和流程为:将基因组区域中显示出高水平杂合性的区域鉴定为单体型区块contig,基于鉴定结果对所有contig拆分和打断,通过将Hi-C数据集成到图形数据结构中构建标准化的互作矩阵,最后经过Hi-C辅助组装挂载获得单条染色体长度的单体型基因组组装[36]。
优化算法解决非桥式重复等重复序列导致的组装连续性问题[32],通过开发出对杂合子感知的一致性算法和分型组装方法,兼具高容错、高准确、高效、计算资源低耗的优点,解决超大基因组组装和大规模基因组组装的计算困境,是未来基因组组装工具开发需要继续改进优化的方向。同时高效低耗的组装算法也能解决真核生物泛基因组(pan-genome)构建的困境[37]。在单体型基因组组装方面,未来的发展可以优化算法将单体型组装扩大到高杂合性样品、更高倍性物种的应用上,将分型算法整合到组装中,并通过基于组装图的方法提高单体型区块contig放置的准确率,提高算法性能[36]。
1.2 基因组注释
基因组注释是对组装的基因组进行基因结构和功能注释。基因预测方法依赖于利用两种类型的基因信息:(1)内容——局部位点,如剪接位点、起始密码子、终止密码子等,预测编码和非编码区域;(2)信号——蛋白质功能位点,检测功能性位点是否存在。原核生物的基因结构简单,各种局部位点特异性强、易识别,基因预测方法基本成熟,如GeneMarkS[38]、Glimmer[39]等。而真核生物的基因存在内含子结构,要经过RNA剪接步骤才能形成成熟的RNA,使得真核基因的预测更为复杂和困难,需要采用更加复杂的算法来预测真核生物的基因结构[18]。如何准确地识别可变剪切位点是真核基因预测的技术难点,目前有三种策略来预测真核基因结构:(1)同源预测:有一些基因蛋白在相近物种间是高度保守的,可以使用已有的高质量近缘物种注释信息通过比对的方式确定外显子边界和剪切位点,该方法最可靠,但是对数据的依赖性很高,所选取物种间的亲缘关系和进化距离对结果影响较大[40];(2)从头预测:通过已有的概率模型(如隐马尔科夫模型)来预测基因结构,再预测剪切位点和非翻译区,这类方法需要预先训练获得预测参数,准确性较低,尤其是对于研究较少的物种,这种情况建议利用近缘物种的同源基因数据以训练基因结构预测参数[18];(3)基于转录组预测:通过物种的RNA-Seq/EST等转录组数据辅助注释,能够较为准确地确定剪切位点和外显子区域[41],但是低表达基因和转录组组装错误也是该方法的主要瓶颈。可以开发出综合上述三种策略的算法,兼具准确性和特异性地识别可变剪切位点。基于可靠的基因结构注释,后续可以进行基因功能注释,目前普遍采用比对的方法[40, 42],但是序列相似与实际生物学功能相似无法完全等价,需考虑引入其它方法,如引入基因表达、调控网络等信息,开发算法进行信息整合进一步完善基因功能注释工作。1.3 基因组变异分析
基因组变异是指与参考序列相比,基因组中发生的单碱基变异、DNA序列片段插入、缺失、扩增和复杂结构变异等。目前基于测序方法进行单核苷酸多态性(Single Nucleotide Polymorphism, SNP)、短的插入缺失(Insertion-Deletion, InDel)等变异检测的策略主要有:(1)基于比对结果直接检测变异信息;(2)基于从头组装结果比对检测变异信息。而在结构变异(Structural Variation, SV)检测方面目前主要有四种策略:(1)基于插入片段长度的异常分布(Read Pair, RP);(2)基于读段覆盖度的异常分布(Read Depth, RD);(3)基于未比对上的读段进行分割比对(Split Read, SR);(4)长读段或者从头组装[43]。不同策略可检测到的变异类型不同,可将多个策略整合在一起,从而大大降低假阳性率[43]。基于拼接的方法是当前获得全基因组范围内各类型变异最好的方法,但是该方法的结果准确性取决于高质量组装,有待高效准确组装方法的发展。GATK是当前公认的最主流的基因组变异分析软件之一,可应用于人和其它物种[44]。但是当前基因组数据变异分析在计算处理能力的瓶颈限制了这些技术的广泛使用,针对该问题已有用FPGA进行硬件加速、搭载GPU加速的Parabricks软件等方案来加速GATK的运行效率。测序读长加长可以提高变异分析的准确度,尤其是大的结构变异分析。未来测序技术的发展,兼具测序读长长和测序准确性,也将极大的改变变异检测方法的现状[45]。如何解读这些变异信息,从海量的变异位点中筛选出真正具有生物学意义的基因位点是变异组学运用的主要瓶颈之一。目前可以通过GWAS分析获得基因型和表型之间的关系[46]。在GWAS分析中需要考虑适合样本的关联模型。样本的表型分两种:(1)数量性状的表型,这种关联分析主要采用线性回归模型,包括一般线性模型和混合线性模型,对于受到多因素共同影响的复杂数量性状常采用混合线性模型或者改进后的模型进行分析,仔细选择模型或者整合互补模型可以对复杂性状的遗传基础有更深入的了解[47,48];(2)case-control二元的表型,case和control比例失衡时,可能会导致较高的假阳性率,针对该问题提出的SAIGE模型显示出在样品比例极其失衡时结果仍较为正常的特点[49]。当前GWAS分析对于低频基因的挖掘能力有待提高,可以通过改进开发新的模型和新的群体设计揭示表型变异的确定原因[47]。而将GWAS关联结合转录组、甲基化组等组学研究的数据分析,即eQTL、meQTL等分析,则可以将表型与基因组变异,再到基因表达、甲基化等之间的关系联系起来。整合多组学数据进行联合分析揭示复杂性状的分子机制,这也是目前变异解析的主要挑战[50]。在识别各类QTLs位点的时候,需要进行多重假设检验和校正,常用的多重假设检验校正方法有Bonferroni校正、BH校正和随机打乱后进行Storey校正等[51]。当前的多重假设检验校正方法难以兼具准确性和速度,保证准确度需要耗费大量的内存和时间,尤其是对于trans-eQTLs位点识别时用随机打乱后进行Storey校正方法,通常的超级计算机集群是基本不可能完成的,亟需新的模型和算法优化来攻克这道难关[52]。
2 转录组测序数据分析
转录组是细胞内所有RNA分子的总和。细胞中的DNA记录的遗传信息通过转录表达,决定生物体的性状。转录组中,mRNA是DNA和蛋白质之间的中间产物,而非编码RNA则有多种多样的功能。转录组技术可以捕捉到细胞内所有RNA分子瞬时的存在情况。转录组数据分析技术对转录组技术产生的数据进行分析和挖掘,得到的结果和发现对基础研究、临床诊断等都有很大的推动。比如Hammond等[53]对小鼠发育过程中、老年和大脑受伤后的76 000个小神经胶质细胞进行了单细胞测序,发现年轻小鼠的小神经胶质细胞的异质性最高,老年小鼠小神经胶质细胞中的炎性细胞最多,并鉴定出受伤后发生反应的小神经胶质细胞亚型,为今后鉴定和操作健康和疾病状态的各种亚型的小神经胶质细胞奠定了基础。而在临床诊断中,转录组数据分析结果可以得出超出生理范围的基因表达情况、等位基因的特异表达和异常的选择性剪切等[54],对基因组测序数据是一个重要的补充。2.1 RNA-Seq数据分析
RNA-Seq技术是目前科研中最常用的转录组技术[55]。由于RNA-Seq读长短,一般无法测出全长的转录本,为了检测哪些转录本在样品中表达,在所研究物种有高质量的参考基因组的情况下,通常会先把测序短读段比对到参考基因组,再组装成全长转录本[56]。在研究的物种没有参考基因组或参考基因组质量较差的情况下,需要对转录本进行从头组装[41]。如果物种的参考转录组注释质量高或使用从头组装的转录组,则可以将读段比对到转录组上直接定量[57]。对基因表达的定量一般是通过直接计算比对到基因上的读段数,然后对基因长度和测序深度做归一化,得到基因表达的RPKM(Reads Per Kilobase per Million mapped reads),FPKM(FragmentsPer Kilobase per Million mapped reads)或TPM(Transcripts Per Million mapped reads)值[56]。也有一些定量方法不需要将读段比对到基因序列上,比如通过对读段中k-mer计数的方法获得基因的表达量[58]。在对基因的表达数据做样本间、样本内归一化、去除批次效应和考虑其它可能产生偏差的因素后,可以基于对表达量统计分布的推断(一般假设基因的表达量服从负二项分布或泊松分布),通过统计检验确定在分组间差异表达的基因[59]。对转录本水平上的差异表达分析有两种策略:基于转录异构体(isoform)的策略和基于外显子的策略。基于转录异构体的策略对转录异构体的表达进行定量,然后检测差异表达[56];基于外显子的策略通过对比对到外显子上和跨剪切位点的读段进行计数来检测选择性剪切的信号[60]。融合基因的鉴定类似于新转录异构体的鉴定,但由于融合的两个基因可能位于不同的染色体上,搜索空间更大,并且要考虑到读段比对的错误和生物学上的重要性对找到的融合基因进行筛选[61]。对小RNA测序数据的分析一般是把读段直接比对到已知的小RNA序列上进行定量,或者把读段比对到参考基因组,提取上下游序列进行茎环结构预测,预测miRNA前体序列和可能的新miRNA[62]。2.2 三代转录组测序数据分析
三代转录组测序数据的特点是读长长、错误率高和通量较低。因其读段长,大多数情况下可以直接得到全长的转录本序列,不需要组装。但因为其错误率较高,需要在转录本鉴定前对读段进行错误校正[63]。一般错误校正的方法分为两类:(1)基于混合读段的错误校正,即利用对同一个样品的RNA-Seq短读段序列对易出错的长读段进行校正[64];(2)基于读段重叠区的错误校正,即通过比对不同读段间重叠的序列来校正这段被多次测到的序列[65]。最新的三代转录组的建库和测序策略(如PacBio公司的ISO-Seq技术[66]和Oxford Nanopore公司的R2C2方法[67]),充分利用目前测序读段长度远长于转录本长度的特点,在一个长读段中对同一转录本连续进行多次测序,将每次测到的全长转录本(称为subread)合在一起比对得到转录本的一致序列(consensus sequence),有效排除了测序错误的影响。由于之前三代测序技术的通量较低,一般使用二代和三代混合测序数据进行转录本定量。但目前主流三代测序技术的通量都有很大提高,如PacBio Sequel II测序仪的一个SMRT Cell的通量已达到8百万个长读段,达到了可以对转录本进行定量的标准,此时只需要对相应的长读段进行简单计数就可以得到基因或转录本的TPM值。三代测序数据由于读长长的优势,在转录异构体鉴定[68]、融合基因转录本鉴定[69]、转录本单体型鉴定(transcript haplotype phasing)[70]、转录本重复序列鉴定等应用上无论是准确度还是分析方法的简便性对比RNA-Seq技术都有很大优势,加上三代的RNA直测技术[71]的兴起可以去除建库时反转录步骤带来的人为干扰,使转录本的鉴定和定量更准确,三代转录组测序技术是未来转录组技术进步的一个方向。2.3 单细胞RNA-Seq数据分析
单细胞RNA-Seq技术由于把瞬时基因表达谱的刻画提升到了单个细胞的精度,极大地推进了人们对生命系统的认识,促成了很多新的科学发现,因此是当下最热门和发展最快的转录组技术。单细胞RNA-Seq产生的读段除了转录本的片段序列外,一般会带有一段细胞特异的(实际上是井或者液滴特异的)条形码和一段转录本特异的唯一分子标识(Unique Molecular Identifier,UMI),首先需要根据这两段标记序列给读段分类,得到一个计数矩阵(count matrix),每一行代表一个细胞,每一列是一个转录本在各个细胞中表达的读段数[13]。在做基因表达分析之前,需要综合考虑每个条形码下的读段数(计数深度,count depth)、每个条形码下表达的基因数和每个条形码下的线粒体基因的读段数三个特征来对测序数据进行质量控制,去除死细胞、细胞膜破裂的细胞和一个井/液滴中含有两个或多个细胞的情况[72]。由于建库和测序过程中化学反应的复杂性,即使对于完全相同的细胞,都可能获得不同的计数深度,因此在比较细胞间基因表达差异之前,需要在细胞水平上对转录本表达计数进行归一化。单细胞RNA-Seq计数矩阵中由于零值较多,需要专门设计归一化的方法。单细胞RNA-Seq计数矩阵归一化的方法分为线性和非线性两种:线性方法对一个细胞内的所有转录本使用全局统一的缩放因子[73];而非线性方法则可以去掉其它的偏差,比如批次因素[74]。在归一化之后,计数矩阵会进行log(x+1)变换。为了减少下游分析的复杂度和减少数据中的噪声,避免“维数灾难”,需要对计数矩阵进行特征选择和降维。特征选择步骤选出对于下游分析信息量最大的基因,通常会选择表达量波动最大的1 000-5 000个基因(Highly Variable Genes, HVGs),作为降维算法的输入[75]。降维算法在尽量保持数据结构的基础上,进一步将高维空间的数据映射到低维空间。最常用的降维方法分为两类:(1)线性降维方法,主要关注在映射后保持表达差异点的距离,如主成分分析法(Principal Component Analysis, PCA)和多维标度法(Multidimensional Scaling, MDS);(2)非线性降维方法,主要关注在映射后保持表达模式相似的点足够接近、保持数据的局部结构,同时也兼顾保持全局结构,比如曲线成分分析(Curvilinear Components Analysis, CCA)、t-SNE(t-Distributed Stochastic Neighbor Embedding)、UMAP(Uniform Manifold Approximation and Projection)、扩散映射(Diffusion maps)等。在数据降维后,可以实现对单细胞RNA-Seq数据的可视化。
对经上述步骤处理好的表达数据进行模式识别是单细胞RNA-Seq数据分析中的核心步骤。单细胞RNA-Seq常见的下游分析有聚类分析、聚类注释、组成分析、轨迹推断和差异表达分析等。聚类分析根据基因表达谱定义的细胞间的距离对细胞聚类。细胞间距离的度量方式包括欧几里得距离、余弦相似度、基于相关性的距离度量和通过对每个数据集用高斯核学习得到的距离度量等,后三种方式由于具有标度不变性,考虑值之间的相对差异,对于建库大小、细胞大小不同的细胞之间的比较更健壮。传统上,聚类分析方法主要有:(1)k-均值法,其中最常用的是RaceID和SIMLR,这两种方法针对识别罕见细胞类型做了特殊处理;(2)层次聚类法,常用的有CIDR和PCAReduce,分别针对测序深度低导致的dropout事件和罕见细胞类型做了优化;(3)社区发现法(community detection),该类方法的思路是把密集连接的点,而非距离近的点归为一类,这类方法被认为针对大数据集有更快的速度和更好的聚类效果,其中基于k-近邻图[76]的Louvain算法[77]在被Seurat和SCANPY工具包采用后,在单细胞RNA-Seq数据聚类分析中得到了最广泛的应用。近期针对单细胞RNA-Seq数据,出现了一些新的聚类分析思路,比如基于概率模型的CellAssign、BAMM-SC、基于深度神经网络的GOAE、GONN、ACTINN、scScope和scDeepCluster,这些方法在一些数据集上显示出具有更高的聚类准确度,未来有望得到更广泛的应用。对聚类的注释需要依靠Mouse Brain Atlas和Human Cell Atlas[78]这样的参考数据库,或者先找出研究的一类细胞与所有其它细胞差异表达的基因(称为标记基因)与数据库中细胞的标记基因对照,或者用这类细胞的整个基因表达谱与数据库中的细胞表达谱对照,推断这类细胞的类型。对聚类进行注释之后,可以对细胞组成进行统计建模,用统计检验对不同数据集间的细胞组成差异进行分析。测序样品中的细胞如果处于连续变化的过程中,比如样品中同时存在处于发育、疾病进展、对处理条件响应等过程中不同阶段的细胞时,旨在寻找离散的分组的聚类分析技术无法准确刻画样品中细胞的组成结构,而轨迹推断或伪时序分析技术则可以对细胞变化的路径进行解析,把每类细胞在进程中的先后顺序表示为线性的、二叉的、复杂图的、树的或多叉的拓扑结构[79]。在基因层面上,可以对细胞间差异表达的基因进行分析。根据测试,为普通RNA-Seq设计的差异表达分析方法加上对基因进行加权的方法的效果要好于目前专门为单细胞RNA-Seq设计的差异表达分析方法[80]。
转录组分析技术已成为科学研究、临床应用中不可缺少的关键技术环节。一方面,现有转录组技术的不断完善和新兴的单细胞三代测序技术、空间转录组测序技术等新转录组技术的不断涌现和发展为转录组数据分析技术的发展提出了更高的要求;另一方面,人们对科学问题理解的逐步加深又要求转录组分析技术不断创新,能更充分更深入地挖掘基因表达数据中蕴含的现象和规律。可以预见,转录组分析技术在未来将发挥更大的作用。
3 表观组测序数据分析
随着测序技术的发展,表观组学相关研究已经成为生命科学领域炙手可热的研究方向。科学家们发现,表观组学对许多重要的细胞过程的调控起着关键作用,在精准医学、生长发育、分子育种、公共安全等领域研究中占有不可忽视的地位。表观组研究主要包括组蛋白修饰、DNA甲基化、染色质可及性,以及染色质三维结构等。表观组测序实验技术的特殊性决定了用于数据分析的各种计算方法的特征和特异性。随着表观组研究技术发展的突飞猛进,必将开发出更多新的算法,产生更多解决问题的新工具用于数据整合和深度挖掘,也必将使得更多的生命科学秘密被发现,更多的改变生命过程的表观遗传标记被发现并应用于提高国计民生。3.1 组蛋白修饰
目前,组蛋白修饰研究主要通过免疫共沉淀技术与深度测序技术相结合的办法(ChIP-Seq)获取实验数据。ChIP-Seq数据分析中的核心步骤是富集峰识别(peak calling)。首先是通过整合比对到特定基因组区域的读段数来生成信号图谱。然后,依靠滑动窗口方法将读段计数的离散分布平滑为连续的信号轮廓分布,估计超出预定义的峰的读段数量[81],进一步考虑正链和负链中读段计数的对应关系[82,83],以提高峰的分离度。还有其他一些工具使用更复杂的方法在序列窗口中整合信号,例如使用局部泊松模型来识别基因组位置的局部偏差[84]、依赖核密度估计[85]、使用贝叶斯分层t-混合模型用于平滑基因组信号图谱中的读段计数[86]。在与样本的比较分析中,背景分布的选择也是识别富集峰中必不可少的步骤,多使用非结合蛋白质的对照抗体实验的ChIP-Seq数据。大多数峰识别算法通过估计其相应的p值和q值来对更重要的峰进行排序和选择[87]。通常,不同的峰识别工具产生的结果会有所不同,因此,使用者需根据研究的靶向蛋白和实验的情况,比如阴性对照样本的选择、是否有生物学重复等来选择峰识别的工具。3.2 DNA甲基化
通常情况下,DNA甲基化是指胞嘧啶甲基化。检测DNA甲基化状态的实验主要包括重亚硫酸盐芯片、MeDIP-Seq、重亚硫酸盐测序BS-Seq等等。Illumina公司的Infinium Methylation Assay能够对全基因组范围内的单碱基分辨度的甲基化水平进行定量测量。在处理Infinium芯片测定的数据时,要进行背景校正,以消除非特异性信号和重复样品之间的差异[88],完成归一化[88]和进行批次效应校正[89]等。MeDIP-Seq是利用5-甲基胞嘧啶特异性抗体识别甲基化DNA的免疫沉淀技术结合高通量测序的方法。MeDIP-Seq数据可以采用与ChIP-Seq数据相同的生物信息学方法识别富集区域,也可以使用专门为甲基化富集数据量身定制的方法[89]。在重亚硫酸盐测序数据中,甲基化的胞嘧啶不与重亚硫酸盐发生化学反应,而未甲基化的胞嘧啶则在重亚硫酸盐处理和测序后变成胸腺嘧啶。对于BS-Seq数据,将已经发生碱基变化的读段比对到基因组,是甲基化数据分析的第一个难点。目前,已开发出多种比对策略来解决这个问题。一类是利用已有通用比对软件[90,91],采用三碱基(即T,G和A或者A,T和C)方式进行序列比对[92,93,94]。由于三碱基比对降低了序列的特异性,在比对到大的参考基因组序列时,一方面增加了比对时间,另一方面减少了唯一比对的读段数量,造成测序读段利用率变低。一旦重亚硫酸盐测序读段与参考基因组唯一比对,就可以估计特定基因组区域的甲基化水平,定量Cs和Ts的频率。另一种比对策略是在比对过程中,不断调整标记比对评分的矩阵,以适应碱基不匹配的情况[94]。这类比对工具往往高估了高甲基化的区域,但比对效率较高。在为BS-Seq数据选择比对工具时,要考虑测序数据是来自于定向还是非定向建库测序。在BS-Seq建库时往往会加入非甲基化的Lambda DNA,用于比对后评估重亚硫酸盐反应的转化效率。近年来,单细胞实验技术和测序技术迅猛发展,单细胞甲基化研究多采用重亚硫酸盐建库方式,测序数据的比对率一直都比较低。2019年一项研究发现[95],在单细胞BS-Seq文库制备时,通过重亚硫酸盐转化后基因组近端序列与微同源区(MR)重组产生嵌合分子,而且MR内的DNA甲基化高度可变,必须删除这些区域以准确估算DNA甲基化水平。而常规比对工具采用end-to-end比对,难以达到较高的比对率。于是科学家们开发出支持单细胞甲基化测序数据局部比对的比对工具,在执行质控和重亚硫酸盐测序数据局部比对的同时,进行嵌合分子测定和MR去除,大大提高了单细胞重亚硫酸盐测序数据的比对率和功能元件的回收率。对于DNA甲基化测序数据的分析,如何在全基因组水平上准确识别DNA甲基化差异区域(Differentially Methylated Region, DMR)仍然是一个挑战。DMR的识别通常采用以下几种方法,第一种使用二项分布、负二项分布或具有过度分散参数的离散分布对甲基化/未甲基化读段的数量进行建模[96,97,98]。一种是应用平滑算子(smoothing operator),考虑相邻CpG位点之间甲基化模式的相关性[98]。Metilene[99]采用分割算法来检测单个或者一组重复实验之间的DMR,不需要对数据生成机制进行任何模型假设。虽然识别DMR的方法很多,但是往往存在参数的依赖性,选择不同的参数,会得到不同的DMR识别结果。为了解决参数依赖和缺乏通用性的问题,科学家们采用完全贝叶斯方法开发工具ABBA[14]自动平滑甲基化图谱,并可靠地识别DMR。ABBA基于潜在的高斯模型(Latent Gaussian Model, LGM)和集成嵌套拉普拉斯近似(Integrated Nested Laplace Approximation, INLA),对WGBS实验的随机采样过程(甲基化和非甲基化的读段数量分布作为非高斯响应变量)进行建模,采用概率分布指定所有未知量。ABBA通过对平滑的未观测到的甲基化图谱的后验概率的评估来计算每个CpG位点的后验概率,并对两组数据之间的全基因组后验甲基化概率进行差异比较,进而识别DMRs。ABBA考虑了WGBS数据的一些内在特征,比如通过具有特定组内差异的随机效应进行建模以消除组内实验重复之间的DNA甲基化差异;通过潜在的高斯场方程反映模型的邻域结构,并自动适应数据的变化,进而明确DNA甲基化模式的相关性。ABBA为潜在的高斯场方程的参数分配先验分布,从而充分考虑了这些量的不确定性。所有这些功能使ABBA的模型能够根据实际情况进行调整,而无需任何用户定义的参数。尽管此方法实现了DMR识别的无参数,但是算法繁琐复杂,并且对于大样本间的差异甲基化识别仍有很大的困难和挑战。
3.3 染色质可及性
目前,研究染色质可及性的实验手段主要是通过酶解或者物理化学手段对开放区域的DNA进行片段化处理,进一步对DNA片段进行基因组定位,明确富集区域,并对富集区域进行功能分析。目前研究染色质可及性的方法主要包括DNase-Seq、FAIRE-Seq、MNase-Seq和ATAC-Seq。MNase-Seq是研究核小体定位的方法,通过对核小体保护区域的DNA序列进行定位,进而反映出失去核小体保护的DNA的区域,其他三种方法则是通过对染色质开放区域的直接定位,反映染色质开放性。分析这几种实验类型测序数据的思路与ChIP-Seq测序数据的分析思路基本上是一致的,即识别富集区域,并对富集区域进行功能分析。但是染色质开放性峰信号通常是宽序列读取峰,需要区分真实峰和假象峰,也要对峰进行分类[100,101,102]。科学家们不断开发和优化算法,使染色质可及性测序数据的分析越来越准确。近年来,单细胞染色质可及性研究越来越多[103],单细胞染色质可及性对于在单细胞水平了解细胞核内染色质动态变化、揭示染色质可及性在细胞间的异质性、鉴定细胞特异性的染色质活性区域等具有重要意义。3.4 染色质三维结构
三维基因组结构研究利用的染色质构象技术在经历了3C、4C和5C技术后,又开发出了Hi-C技术。通过Hi-C数据分析揭示染色质的远程相互作用,推导出基因组的三维空间结构和可能的基因之间的调控关系。Hi-C数据的上游分析包括:(1)比对基因组,Hi-C数据需要用特定的策略来比对基因组[104,105];(2)基因组的一定区间范围(bin)的选择,bin的大小直接决定了最终分析结果的分辨度。由此,也产生了一些确定最佳bin大小的方法,使选择的bin尽可能的小[106];(3)归一化,如迭代校正和特征向量分解归一化,顺序分量归一化等[104-105, 107-108],并生成数据矩阵。Hi-C数据的下游分析是从Hi-C数据矩阵中提取有生物学意义的结果,包括:(1)隔室(A/B Compartments)识别,隔室识别多通过主成分分析获得,由第一主成分值的正负来表示A Compartment或者B Compartment,也有依靠一种更快且内存效率更高的方法来定义隔室得分,以反映出任何给定的bin进入“A”隔室的可能性[105, 109];(2)拓扑相关结构域(TAD)识别,即识别沿着接触矩阵的对角线显示为高度自关联的连续区域[106, 110-112];(3)相互作用点识别,也就是识别距离较远的染色质区域之间相互联系的特定位点。相互作用的计算识别需要定义背景模型,以便于识别相互作用频率高于预期的相互作用联系[113,114]。另外,数据的可视化在Hi-C数据的分析中显得尤为重要,可视化可以直观地帮助研究者描述TAD模式,也可以揭示分析产生的基因组结构特征性信息。因此,科学家们不断地开发一些可视化工具用于Hi-C数据的分析,比如Hi-Browse[115]、HicPlotter[116]、3D-GNOME[117]、HiC-3Dviewer[118]和3D genome browser[119]等以热图的方式可视化染色质相互作用矩阵数据,并大多采用web形式实现人机交互。近两年开发的,如Delta[120]通过使用4个可视化视图模块,即Virtual 4C绘图、线性基因组视图、基因组环形视图和物理视图,实现对不同类型的特征数据进行全面的可视化展示;GITAR[121]除了可实现Hi-C数据的全面分析和可视化,还提供大量人类和小鼠的数据集,便于用户进行数据集之间的比较;HiCcompare[122]能够可视化分析两个Hi-C数据集之间的差异;GenomeFlow[123]在实时可视化Hi-C数据3D基因组模型的同时,还允许用户在3D基因组模型上附上基因注释、基因表达数据和基因组甲基化数据;SilkDB 3.0[124]专门用于家蚕数据的交互式可视化分析。总体而言,Hi-C技术的迅速发展,带来了染色质三维结构数据集的快速增加,这也加快了数据分析方法的迅速兴起,然而,虽然可以使用大量的方法学解决方案来识别TAD,但尚未完全了解TAD的结构和功能,尤其是TAD的内部结构仍然难以捉摸[125]。因此,全面了解染色质三维结构的功能和作用,面临着生物学和技术上的双重挑战。4 挑战和展望
从本世纪初高通量组学测序技术开始得到广泛应用至今,随着测序花费的下降和测序通量的不断提高,各国政府和企业资助的大型基因组、转录组、表观组计划相继开展[16-17, 78, 126],对基因组学数据分析技术处理海量数据的速度提出了极高的要求。为了应对基因组学数据的飞速增长,一方面,算法技术不断改进,如数据分析中耗时步骤是将读段比对到基因组的算法,从线性比对[127],到对基因组建立散列索引[128],到建立后缀数组/Burrows-Wheeler变换索引,到建立更加复杂的FM索引[129],到更高效的层次化图结构的FM索引(Hierarchical Graph FM index,HGFM)[130],一系列改进使得序列比对速度有了上千倍的提高;另一方面,专门针对基因组数据分析设计的并行计算和高性能计算方案也开始涌现,比如使用多核共享内存的超级计算机、特殊的硬件设备(FPGA、GPU、TPU等)、多节点的高性能计算机、云计算、容器部署等[45]。已有文章针对上述硬件开发了应用于多种基因组学数据分析的并行算法,比如基于Apache Spark的GATK RNA-Seq流程、基于迭代随机森林的基因表达网络构建、基于MapReduce的应用于年龄预测的CpG岛筛选、基于Apache Spark的对BWA-MEM的加速等,都达到了很好的效果。尽管有上述这些进展,现有的算法和硬件技术发展对处理数据效率的提升仍然远远赶不上组学数据增长的速度,很多分析流程的复杂性决定了计算过程必须是并行和串行混合的,并且有很难突破的限速步骤,并行计算和分布式计算本身也存在理论上的极限[131]。因此,如何开发新的方法,提高组学大数据的处理效率,仍是目前亟待解决的问题。另外,实践“功能即服务”的理念——把复杂的数据分析流程分割成微服务,部署在商业云或专业云上,可以增加每部分服务的可伸缩性,对于世界各地需要进行组学数据分析的中小实验室,可以减去其购买高性能服务器等基础设施建设和搭建软件环境的压力,提高其数据分析的效率,也是未来的发展趋势之一。海量组学数据分析除了数据量大带来的计算问题,数据来源广产生的异构性、不完整、尺度大、质量参差不齐,也给组学大数据分析带来了极大的挑战。目前已有很多去除组学大数据批次效应的方法和模型[132,133],但是统计建模过程从特定数据类型,转变为多组学整合交叉研究的自动化建模是未来发展的方向。针对异构性和数据缺失问题,虽然已提出了多核学习(multiple kernel learning)算法、DARTS计算框架、有参模型、无参模型等大数据机器学习、人工智能、统计建模技术,为多源异构组学数据构建预测模型,但是算法的性能、精度和可靠性仍有很大的提升空间[15, 134-135]。测序技术的发展也将推动组学数据分析方法的发展,但是如何整合分析多组学数据全面解读生物系统也成了一项挑战[13]。多组学数据整合分析将带来维度灾难、扩展性、归一化等问题,有待优化和开发一些深度学习方法促进多维组学大数据整合分析[136,137]。此外,临床上将多组学数据与环境暴露数据、健康医疗档案信息、临床影像信息联合分析将能更好的服务于精准医学的发展,虽然已经有了一些研究范式[17, 135],但是制定数据标准化体系、建设生物医学大数据共享平台、开发多维生物医学数据分析的新算法、建立多维知识图谱等都是亟待解决的问题[138]。我国于2019年成立国家基因组科学数据中心来存储、质控、管理、发布、共享这些多维、异构基因组学数据[139],期待该中心未来也能开发出能解决数据多维、异构问题的算法和工具。
利益冲突声明
所有作者声明不存在利益冲突关系。参考文献 原文顺序
文献年度倒序
文中引用次数倒序
被引期刊影响因子
[J].
[本文引用: 1]
[J].
[本文引用: 1]
[J].
[本文引用: 1]
[J].
[本文引用: 5]
[J].
[本文引用: 1]
[J].
[本文引用: 1]
[J].
[本文引用: 1]
[J].
[本文引用: 1]
[J].
[本文引用: 1]
[J].
[本文引用: 1]
[J].
[本文引用: 1]
[J]. Cell
[本文引用: 1]
[J].
[本文引用: 3]
[J].
[本文引用: 2]
[J].
DOI:10.1038/s41592-019-0351-9URL [本文引用: 2]
[J].
[本文引用: 2]
[J].
[本文引用: 3]
[J].
[本文引用: 3]
[J].
[本文引用: 1]
[J].
[本文引用: 1]
[J].
[本文引用: 1]
[J].
[本文引用: 2]
[J].
[本文引用: 1]
[J].
DOI:10.1371/journal.pone.0132881URL [本文引用: 1]
[J].
DOI:10.1038/nbt.2727URL [本文引用: 2]

Genomes assembled de novo from short reads are highly fragmented relative to the finished chromosomes of Homo sapiens and key model organisms generated by the Human Genome Project. To address this problem, we need scalable, cost-effective methods to obtain assemblies with chromosome-scale contiguity. Here we show that genome-wide chromatin interaction data sets, such as those generated by Hi-C, are a rich source of long-range information for assigning, ordering and orienting genomic sequences to chromosomes, including across centromeres. To exploit this finding, we developed an algorithm that uses Hi-C data for ultra-long-range scaffolding of de novo genome assemblies. We demonstrate the approach by combining shotgun fragment and short jump mate-pair sequences with Hi-C data to generate chromosome-scale de novo assemblies of the human, mouse and Drosophila genomes, achieving-for the human genome-98% accuracy in assigning scaffolds to chromosome groups and 99% accuracy in ordering and orienting scaffolds within chromosome groups. Hi-C data can also be used to validate chromosomal translocations in cancer genomes.
[J].
[本文引用: 1]
[J].
DOI:10.1126/science.aal3327URL [本文引用: 1]
[J].
DOI:10.1038/nature22971URL [本文引用: 1]
[J].
DOI:10.1101/gr.141515.112URL [本文引用: 1]

Exceptionally accurate genome reference sequences have proven to be of great value to microbial researchers. Thus, to date, about 1800 bacterial genome assemblies have been "finished'' at great expense with the aid of manual laboratory and computational processes that typically iterate over a period of months or even years. By applying a new laboratory design and new assembly algorithm to 16 samples, we demonstrate that assemblies exceeding finished quality can be obtained from whole-genome shotgun data and automated computation. Cost and time requirements are thus dramatically reduced.
[J].
DOI:10.1101/gr.215087.116URL [本文引用: 1]
[J].
DOI:10.1038/nmeth.4035URL [本文引用: 2]
[J].
DOI:10.1038/s41587-019-0072-8URL [本文引用: 2]
[J].
DOI:10.1038/s41477-019-0487-8URL [本文引用: 1]
[J].
DOI:10.1038/s41588-018-0237-2URL [本文引用: 1]
[J].
DOI:10.1038/nbt.4277URL [本文引用: 1]
[本文引用: 2]
[J].
DOI:10.1186/s13059-019-1751-yURL [本文引用: 1]
[J].
DOI:10.1093/nar/29.12.2607URL [本文引用: 1]
[J].
DOI:10.1093/bioinformatics/btm009URL [本文引用: 1]
[J].
DOI:10.1186/1745-6150-7-12URL [本文引用: 2]
[J].
DOI:10.1038/nprot.2013.084URL [本文引用: 2]
DOI:10.1093/bioinformatics/btu031URL [本文引用: 1]

Motivation: Robust large-scale sequence analysis is a major challenge in modern genomic science, where biologists are frequently trying to characterize many millions of sequences. Here, we describe a new Java-based architecture for the widely used protein function prediction software package InterProScan. Developments include improvements and additions to the outputs of the software and the complete reimplementation of the software framework, resulting in a flexible and stable system that is able to use both multiprocessor machines and/or conventional clusters to achieve scalable distributed data analysis. InterProScan is freely available for download from the EMBl-EBI FTP site and the open source code is hosted at Google Code.
[J].
DOI:10.1038/nrg2958URL [本文引用: 2]
[J].
DOI:10.1101/gr.107524.110URL [本文引用: 1]
[J].
DOI:10.1186/s13059-019-1858-1URL [本文引用: 2]
[J].
DOI:10.1016/j.cell.2015.07.003URL [本文引用: 1]
[J].
DOI:10.1016/j.molp.2016.12.008URL [本文引用: 2]
[J].
DOI:10.1371/journal.pgen.1007309URL [本文引用: 1]
[J].
DOI:10.1038/s41588-018-0184-yURL [本文引用: 1]
[J].
DOI:10.1093/nar/gky814URL [本文引用: 1]
[J].
DOI:10.1111/rssb.2002.64.issue-3URL [本文引用: 1]
[J].
DOI:10.1093/bioinformatics/btv722URL [本文引用: 1]
[J].
DOI:10.1016/j.immuni.2018.11.004URL [本文引用: 1]
[J].
DOI:10.3389/fgene.2019.01152URL [本文引用: 1]
[J].
DOI:10.1038/s41576-019-0150-2URL [本文引用: 1]
[J].
DOI:10.1038/nprot.2012.016URL [本文引用: 3]

Recent advances in high-throughput cDNA sequencing (RNA-seq) can reveal new genes and splice variants and quantify expression genome-wide in a single assay. The volume and complexity of data from RNA-seq experiments necessitate scalable, fast and mathematically principled analysis software. TopHat and Cufflinks are free, open-source software tools for gene discovery and comprehensive expression analysis of high-throughput mRNA sequencing (RNA-seq) data. Together, they allow biologists to identify new genes and new splice variants of known ones, as well as compare gene and transcript expression under two or more conditions. This protocol describes in detail how to use TopHat and Cufflinks to perform such analyses. It also covers several accessory tools and utilities that aid in managing data, including CummeRbund, a tool for visualizing RNA-seq analysis results. Although the procedure assumes basic informatics skills, these tools assume little to no background with RNA-seq analysis and are meant for novices and experts alike. The protocol begins with raw sequencing reads and produces a transcriptome assembly, lists of differentially expressed and regulated genes and transcripts, and publication-quality visualizations of analysis results. The protocol's execution time depends on the volume of transcriptome sequencing data and available computing resources but takes less than 1 d of computer time for typical experiments and similar to 1 h of hands-on time.
[J].
DOI:10.1186/1471-2105-12-323URL [本文引用: 1]
[J].
DOI:10.1038/nmeth.4197URL [本文引用: 1]
[J].
DOI:10.1186/s13059-014-0550-8URL [本文引用: 1]
[J].
DOI:10.1073/pnas.1419161111URL [本文引用: 1]

Ultra-deep RNA sequencing (RNA-Seq) has become a powerful approach for genome-wide analysis of pre-mRNA alternative splicing. We previously developed multivariate analysis of transcript splicing (MATS), a statistical method for detecting differential alternative splicing between two RNA-Seq samples. Here we describe a new statistical model and computer program, replicate MATS (rMATS), designed for detection of differential alternative splicing from replicate RNA-Seq data. rMATS uses a hierarchical model to simultaneously account for sampling uncertainty in individual replicates and variability among replicates. In addition to the analysis of unpaired replicates, rMATS also includes a model specifically designed for paired replicates between sample groups. The hypothesis-testing framework of rMATS is flexible and can assess the statistical significance over any user-defined magnitude of splicing change. The performance of rMATS is evaluated by the analysis of simulated and real RNA-Seq data. rMATS outperformed two existing methods for replicate RNA-Seq data in all simulation settings, and RT-PCR yielded a high validation rate (94%) in an RNA-Seq dataset of prostate cancer cell lines. Our data also provide guiding principles for designing RNA-Seq studies of alternative splicing. We demonstrate that it is essential to incorporate biological replicates in the study design. Of note, pooling RNAs or merging RNA-Seq data from multiple replicates is not an effective approach to account for variability, and the result is particularly sensitive to outliers. The rMATS source code is freely available at rnaseq-mats.sourceforge.net/. As the popularity of RNA-Seq continues to grow, we expect rMATS will be useful for studies of alternative splicing in diverse RNA-Seq projects.
[J].
DOI:10.1186/gb-2011-12-8-r72URL [本文引用: 1]
[J].
DOI:10.1093/nar/gks554URL [本文引用: 1]
[J].
DOI:10.1186/s13059-018-1605-zURL [本文引用: 1]
[J].
DOI:10.1371/journal.pone.0046679URL [本文引用: 1]
[J].
DOI:10.1038/nbt.2705URL [本文引用: 1]
[J].
[本文引用: 1]
[J].
[本文引用: 1]
[J].
DOI:10.1093/bioinformatics/bty098URL [本文引用: 1]
[J].
DOI:10.1093/nar/gkv562URL [本文引用: 1]
[J].
DOI:10.1093/nar/gkw1076URL [本文引用: 1]
[J].
DOI:10.1038/nmeth.4577URL [本文引用: 1]
[J].
DOI:10.1186/s13059-016-0888-1URL [本文引用: 1]
[J].
DOI:10.1186/s13059-016-0947-7URL [本文引用: 1]
[J].
DOI:10.1016/j.cels.2019.03.010URL [本文引用: 1]
[J].
DOI:10.1038/NMETH.2645URL [本文引用: 1]

Single-cell RNA-seq can yield valuable insights about the variability within a population of seemingly homogeneous cells. We developed a quantitative statistical method to distinguish true biological variability from the high levels of technical noise in single-cell experiments. Our approach quantifies the statistical significance of observed cell-to-cell variability in expression strength on a gene-by-gene basis. We validate our approach using two independent data sets from Arabidopsis thaliana and Mus musculus.
[J].
DOI:10.1038/nbt.3192URL [本文引用: 1]
[J].
[本文引用: 1]
[J].
DOI:10.1038/550451aURL [本文引用: 2]
[J].
DOI:10.1038/s41587-019-0071-9URL [本文引用: 1]
[J].
DOI:10.1186/s13059-018-1406-4URL [本文引用: 1]
[J].
DOI:10.1038/nbt.1505URL [本文引用: 1]
[J].
DOI:10.1093/nar/gkn488URL [本文引用: 1]
[J].
DOI:10.1093/bioinformatics/btt470URL [本文引用: 1]

Motivation: Chromatin immunoprecipitation coupled to next-generation sequencing (ChIP-seq) is widely used to study the in vivo binding sites of transcription factors (TFs) and their regulatory targets. Recent improvements to ChIP-seq, such as increased resolution, promise deeper insights into transcriptional regulation, yet require novel computational tools to fully leverage their advantages.
Results: To this aim, we have developed peakzilla, which can identify closely spaced TF binding sites at high resolution (i. e. resolves individual binding sites even if spaced closely), as we demonstrate using semisynthetic datasets, performing ChIP-seq for the TF Twist in Drosophila embryos with different experimental fragment sizes, and analyzing ChIP-exo datasets. We show that the increased resolution reached by peakzilla is highly relevant, as closely spaced Twist binding sites are strongly enriched in transcriptional enhancers, suggesting a signature to discriminate functional from abundant non-functional or neutral TF binding. Peakzilla is easy to use, as it estimates all the necessary parameters from the data and is freely available.
[J].
[本文引用: 1]
[J].
DOI:10.1093/bioinformatics/btn480URL [本文引用: 1]
[J].
DOI:10.1111/j.1541-0420.2010.01441.xURL [本文引用: 1]

ChIP-seq combines chromatin immunoprecipitation with massively parallel short-read sequencing. While it can profile genome-wide in vivo transcription factor-DNA association with higher sensitivity, specificity, and spatial resolution than ChIP-chip, it poses new challenges for statistical analysis that derive from the complexity of the biological systems characterized and from variability and biases in its sequence data. We propose a method called PICS (Probabilistic Inference for ChIP-seq) for identifying regions bound by transcription factors from aligned reads. PICS identifies binding event locations by modeling local concentrations of directional reads, and uses DNA fragment length prior information to discriminate closely adjacent binding events via a Bayesian hierarchical t-mixture model. It uses precalculated, whole-genome read mappability profiles and a truncated t-distribution to adjust binding event models for reads that are missing due to local genome repetitiveness. It estimates uncertainties in model parameters that can be used to define confidence regions on binding event locations and to filter estimates. Finally, PICS calculates a per-event enrichment score relative to a control sample, and can use a control sample to estimate a false discovery rate. Using published GABP and FOXA1 data from human cell lines, we show that PICS' predicted binding sites were more consistent with computationally predicted binding motifs than the alternative methods MACS, QuEST, CisGenome, and USeq. We then use a simulation study to confirm that PICS compares favorably to these methods and is robust to model misspecification.
[J].
[本文引用: 1]
[J].
DOI:10.1093/bioinformatics/btn224URL [本文引用: 2]
[J].
DOI:10.1093/bioinformatics/bts124URL [本文引用: 2]

With the increasing availability of high-density methylation microarrays, there has been growing interest in analysis of DNA methylation data. We have developed CpGassoc, an R package that can efficiently perform the statistical analysis needed for increasingly large methylation datasets. CpGassoc is a modular, expandable package with functions to perform rapid analyses of DNA methylation data via fixed or mixed effects models, to perform basic quality control, to carry out permutation tests, and to display results via an array of publication-quality plots.
[J].
DOI:10.1093/bioinformatics/btp324URL [本文引用: 1]
[J].
DOI:10.1186/gb-2009-10-3-r25URL [本文引用: 1]
[J].
DOI:10.1093/bioinformatics/btr167URL [本文引用: 1]

A combination of bisulfite treatment of DNA and high-throughput sequencing (BS-Seq) can capture a snapshot of a cell's epigenomic state by revealing its genome-wide cytosine methylation at single base resolution. Bismark is a flexible tool for the time-efficient analysis of BS-Seq data which performs both read mapping and methylation calling in a single convenient step. Its output discriminates between cytosines in CpG, CHG and CHH context and enables bench scientists to visualize and interpret their methylation data soon after the sequencing run is completed.
[J].
DOI:10.1371/journal.pone.0086707URL [本文引用: 1]
[J].
DOI:10.1186/s12859-018-2120-7URL [本文引用: 2]
[J].
DOI:10.1093/bioinformatics/btz125URL [本文引用: 1]
[J].
DOI:10.1371/journal.pgen.1005650URL [本文引用: 1]
[J].
DOI:10.1186/gb-2012-13-10-r87URL [本文引用: 1]
[J].
[本文引用: 2]
[J].
DOI:10.1101/gr.196394.115URL [本文引用: 1]
[J].
DOI:10.1038/nature11232URL [本文引用: 1]

DNase I hypersensitive sites (DHSs) are markers of regulatory DNA and have underpinned the discovery of all classes of cis-regulatory elements including enhancers, promoters, insulators, silencers and locus control regions. Here we present the first extensive map of human DHSs identified through genome-wide profiling in 125 diverse cell and tissue types. We identify similar to 2.9 million DHSs that encompass virtually all known experimentally validated cis-regulatory sequences and expose a vast trove of novel elements, most with highly cell-selective regulation. Annotating these elements using ENCODE data reveals novel relationships between chromatin accessibility, transcription, DNA methylation and regulatory factor occupancy patterns. We connect similar to 580,000 distal DHSs with their target promoters, revealing systematic pairing of different classes of distal DHSs and specific promoter types. Patterning of chromatin accessibility at many regulatory regions is organized with dozens to hundreds of co-activated elements, and the transcellular DNase I sensitivity pattern at a given region can predict cell-type-specific functional behaviours. The DHS landscape shows signatures of recent functional evolutionary constraint. However, the DHS compartment in pluripotent and immortalized cells exhibits higher mutation rates than that in highly differentiated cells, exposing an unexpected link between chromatin accessibility, proliferative potential and patterns of human variation.
DOI:10.1186/s12864-017-3541-9URL [本文引用: 1]
[J].
DOI:10.1093/nar/gkz759URL [本文引用: 1]
[J].
DOI:10.1016/j.cell.2018.06.052URL [本文引用: 1]
[J].
DOI:10.1038/NMETH.2148URL [本文引用: 2]

Extracting biologically meaningful information from chromosomal interactions obtained with genome-wide chromosome conformation capture (3C) analyses requires the elimination of systematic biases. We present a computational pipeline that integrates a strategy to map sequencing reads with a data-driven method for iterative correction of biases, yielding genome-wide maps of relative contact probabilities. We validate this ICE (iterative correction and eigenvector decomposition) technique on published data obtained by the high-throughput 3C method Hi-C, and we demonstrate that eigenvector decomposition of the obtained maps provides insights into local chromatin states, global patterns of chromosomal interactions, and the conserved organization of human and mouse chromosomes.
[J].
DOI:10.1016/j.cels.2016.07.002URL [本文引用: 3]
[J].
DOI:10.1038/s41467-018-05691-7URL [本文引用: 2]
[J].
[本文引用: 1]
[J].
DOI:10.1093/nar/gky504URL [本文引用: 1]
[J].
DOI:10.1093/bioinformatics/btx802URL [本文引用: 1]
[J].
DOI:10.1038/nmeth.4560URL [本文引用: 1]
[J].
DOI:10.1093/nar/gky789URL
[J].
DOI:10.1038/nature24281URL [本文引用: 1]
[J].
DOI:10.1093/bioinformatics/btw240URL [本文引用: 1]
[J].
DOI:10.1038/s41467-017-02386-3URL [本文引用: 1]
[J].
DOI:10.1093/bioinformatics/btu082URL [本文引用: 1]

Recently developed methods that couple next-generation sequencing with chromosome conformation capture-based techniques, such as Hi-C and ChIA-PET, allow for characterization of genome-wide chromatin 3D structure. Understanding the organization of chromatin in three dimensions is a crucial next step in the unraveling of global gene regulation, and methods for analyzing such data are needed. We have developed HiBrowse, a user-friendly web-tool consisting of a range of hypothesis-based and descriptive statistics, using realistic assumptions in null-models.
[J].
DOI:10.1186/s13059-015-0767-1URL [本文引用: 1]
[J].
DOI:10.1093/nar/gkw437URL [本文引用: 1]
[J].
DOI:10.1007/s40484-017-0091-8URL [本文引用: 1]
[J].
DOI:10.1186/s13059-018-1519-9URL [本文引用: 1]
[J].
DOI:10.1093/bioinformatics/btx805URL [本文引用: 1]
[J].
[本文引用: 1]
[J].
DOI:10.1186/s12859-018-2288-xURL [本文引用: 1]
[J].
DOI:10.1093/bioinformatics/bty802URL [本文引用: 1]
[J].
[本文引用: 1]
[J].
DOI:10.1007/s12551-018-0489-1URL [本文引用: 1]
[J].
DOI:10.1038/nbt1010-1045URL [本文引用: 1]
[J].
DOI:10.1093/nar/25.17.3389URL [本文引用: 1]
[J].
DOI:10.1101/gr.229202URL [本文引用: 1]
[J].
DOI:10.1038/NMETH.1923URL [本文引用: 1]

As the rate of sequencing increases, greater throughput is demanded from read aligners. The full-text minute index is often used to make alignment very fast and memory-efficient, but the approach is ill-suited to finding longer, gapped alignments. Bowtie 2 combines the strengths of the full-text minute index with the flexibility and speed of hardware-accelerated dynamic programming algorithms to achieve a combination of high speed, sensitivity and accuracy.
[J].
DOI:10.1038/s41587-019-0201-4URL [本文引用: 1]
[J].
[本文引用: 1]
[J].
DOI:10.1093/bioinformatics/bts680URL [本文引用: 1]

Motivation: The Illumina Infinium450 k DNA Methylation Beadchip is a prime candidate technology for Epigenome-Wide Association Studies (EWAS). However, a difficulty associated with these beadarrays is that probes come in two different designs, characterized by widely different DNA methylation distributions and dynamic range, which may bias downstream analyses. A key statistical issue is therefore how best to adjust for the two different probe designs.
Results: Here we propose a novel model-based intra-array normalization strategy for 450 k data, called BMIQ (Beta MIxture Quantile dilation), to adjust the beta-values of type2 design probes into a statistical distribution characteristic of type1 probes. The strategy involves application of a three-state beta-mixture model to assign probes to methylation states, subsequent transformation of probabilities into quantiles and finally a methylation-dependent dilation transformation to preserve the monotonicity and continuity of the data. We validate our method on cell-line data, fresh frozen and paraffin-embedded tumour tissue samples and demonstrate that BMIQ compares favourably with two competing methods. Specifically, we show that BMIQ improves the robustness of the normalization procedure, reduces the technical variation and bias of type2 probe values and successfully eliminates the type1 enrichment bias caused by the lower dynamic range of type2 probes. BMIQ will be useful as a preprocessing step for any study using the Illumina Infinium 450 k platform.
[J].
DOI:10.1038/s41592-019-0537-1URL [本文引用: 1]
[J].
DOI:10.1186/s12859-019-2992-1URL [本文引用: 1]
[J].
DOI:10.1371/journal.pone.0157077URL [本文引用: 2]
[J].
DOI:10.1007/s40484-019-0189-2URL [本文引用: 1]
[J].
DOI:10.1038/s41592-018-0176-yURL [本文引用: 1]
[J].
[本文引用: 1]
[J].
[本文引用: 1]