
RESEARCH ON INVERSION METHOD OF HYPERELASTIC CONSTITUTIVE PARAMETERS OF SKELETAL MUSCLES BASED ON SIMULATION AND INTELLIGENT ALGORITHM1)
Li Yang, Sang Jianbing
通讯作者: 2)桑建兵, 教授, 主要研究方向: 工程结构分析与智能算法研究. E-mail:sangjianbing@hebut.edu.cn
收稿日期:2021-01-22接受日期:2021-03-18网络出版日期:2021-05-19
基金资助: |
Received:2021-01-22Accepted:2021-03-18Online:2021-05-19
作者简介 About authors

摘要
从事高强度的体力工作者经常会发生肌肉软组织的损伤, 因此对骨骼肌的变形特性和应力分布的研究受到了越来越多的重视. 获取正确的本构参数对于生物软组织的力学行为的研究至关重要, 而本构参数的确定本质上是一个逆过程, 具有很大的挑战性. 本文分别采用K近邻(K-nearest neighbor, KNN)模型和支持向量机回归(support vector machine regression, SVR)模型并结合非线性有限元仿真, 提出了两种确定骨骼肌本构参数的反演方法. 首先建立了骨骼肌压缩的有限元模型, 对其压缩条件下的变形特性进行了有限元仿真, 得到了相应的变形特性及应力分布规律, 同时也建立了骨骼肌组织的名义应力和主伸长之间非线性关系的数据集. 其次, 分别利用KNN模型和SVR模型搭建了针对骨骼肌组织进行本构参数反演的机器学习智能算法, 对相应的数据集进行训练, 结合单轴压缩实验的实验数据预测了材料的本构参数. 最后, 对分别基于KNN模型和SVR模型对骨骼肌超弹性本构参数的误差结果进行了分析, 通过引入相关系数$R$和决定系数$R^{2}$对采用两种反演方法的有效性进行数值上的验证. 结果表明, 利用KNN模型和SVR模型结合有限元仿真是确定骨骼肌超弹性本构参数的有效、准确的方法, 该方法也可进一步推广到其他类型的非线性软组织的本构参数反演.
关键词:
Abstract
Muscle injury and other diseases often occurs in high-intensity physical workers, so the research on the deformation characteristics and the stress distribution of skeletal muscles are of increasing importance. It is important to obtain the correct constitutive parameters for the study of mechanical behavior of biological soft tissues, and the determination of the constitutive parameters is essentially an inverse process, which possesses challenges. In this paper, two inverse methods based on machine learning are proposed to determine the constitutive parameters, which are k-nearest neighbor (KNN) model and support vector machine regression (SVR) model combined with nonlinear finite element simulation. Firstly, based on the principle of nonlinear mechanics, a finite element model is established to simulate the nonlinear deformation of skeletal muscles under compression, and the corresponding deformation characteristics and stress distribution. At the same time, the dataset of nonlinear relationship between nominal stress and principal stretch of skeletal muscles is established by using the finite element model. Then KNN model and SVR model are used to build the machine learning intelligent algorithms for the inversion of constitutive parameters of skeletal muscle tissues, and the corresponding datasets are trained. Combined with the experimental data of uniaxial compression experiment, the constitutive parameters of skeletal muscles are predicted. Finally, intensive studies also have been carried out to compare the performance of KNN model with SVR model to identify the hyperelastic material parameters of skeletal muscles. And the validity of two inversion methods were verified numerically by introducing the correlation coefficient $(R)$ and the decision coefficient ($R^{2})$. The results show that KNN model and SVR model combined with finite element method are effective and accurate method to identify the hyperelastic material parameters of skeletal muscles. This method can also be further extended for the predictions of constitutive parameters of other types of nonlinear soft materials.
Keywords:
PDF (2408KB)元数据多维度评价相关文章导出EndNote|Ris|Bibtex收藏本文
本文引用格式
李洋, 桑建兵, 敖日汗, 马钰, 魏新宇. 基于仿真和智能算法骨骼肌超弹性本构参数的反演方法研究1). 力学学报, 2021, 53(5): 1449-1456 DOI:10.6052/0459-1879-21-038
Li Yang, Sang Jianbing, Ao Rihan, Ma Yu, Wei Xinyu.
引言
骨骼肌约占生物体重的40%[1], 是维持生物基本运动最重要的生理软组织, 而骨骼肌的损伤会导致人类行为严重受限, 如常见的临床病例: 中风、截瘫等[2]. 在对骨骼肌研究中, 软组织的本构关系可能是最基本和最关键的, 因为其可以建立可靠的数学或计算模型. 由于骨骼肌组织的特殊特性(各向异性[3]、 非线性[4]、 超弹性[5]、 时间依赖性[6])的存在, 骨骼肌组织的力学特性的表征具有很大的挑战性. 全面了解骨骼肌的组成行为, 对于更好地了解骨骼肌的生物学特性, 提高肌肉疾病和损伤的治疗水平具有重要意义. 许多****进行了大量的实验来研究骨骼肌的生物力学特性, 包括压缩[7]、拉伸[8]、应力松弛[9]等. 王宝珍等[10]对猪后腿肌肉进行冲击压缩实验, 并利用改进后的分离式Hopkinson压杆技术获取了不同加载方向和不同应变率下的应力应变数据. Mohammadkhah等[11]对新鲜鸡胸大肌进行了加载方向平行于纤维方向和垂直于纤维方向以及加载方向与纤维方向成45$^\circ$ 的单轴准静态压缩和拉伸实验, 证明鸡肌肉的力学行为是非线性和各向异性的. B?l等[12]使用3种不同的加载模式对肌肉组织进行了一系列实验. 结果表明, 纤维方向对压缩应力有各向异性贡献. 如果有可靠的正问题模型, 反问题分析是系统的识别材料性质的有效手段[13]. Takaza等[14]证明了反向有限元方法在确定材料性能方面的有效性. Silva等[15]通过逆有限元方法并结合优化算法获得了女性耻骨联合肌肉的主动和被动力学特性. 虽然现有的研究在识别本构参数方面取得了进展, 但现有预测的准确性还有待进一步提高.随着人工智能技术(AI)的发展, 机器学习(ML) 作为AI的一个分支已被广泛应用于生活中的各个领域, 如数据融合和识别[16-17]、 医学检测[18-19]、 流体力学[20-22]和固体力学中[23-24]. ML在模拟输入和输出变量之间的非线性相关性方面具有很高的效率, 并且已经被用于预测生物软组织的力学特性. Liang等[25]利用支持向量机模型和有限元方法相结合来预测主动脉瘤的风险, 并取得了良好的效果. Lu等[26]提出了一种基于动态多体方法和神经网络分析的创新方法, 建立了基于有限元的软骨神经网络模型. Liu等[27]对牛的肌肉组织进行了无约束压缩实验, 并利用了改进后的小生境遗传算法预测了肌肉组织的本构参数. 通常AI的成功归结于大规模的数据集, 然而许多现实应用的场景例如医学, 军事等领域, 因为隐私或者安全等因素不允许收集足够多的数据集, 所以对于小样本的学习便成了当今AI领域的热点问题. Koch等[28]将深度学习与小样本学习结合起来, 提出了一种新的卷积神经网络模式来学习成对的样本中与类别无关的变量. Liu等[29]基于BP神经网络, 建立了一个可靠的正问题神经网络来代替有限元方法收集样本, 来缩短收集样本的时间, 也为解决小样本学习问题提供了新的想法. 而简单的机器学习模型对小样本学习也会有良好的效果. 因此, 本研究考虑将机器学习的方法应用于骨骼肌的本构参数的预测, 分别提出了两种反问题求解方法, 一种是将K近邻模型(KNN)与有限元方法(FEM)相结合, 另一种是将支持向量机回归模型(SVR)与有限元方法相结合. 在本研究中, 通过训练和测试Liu等[27]收集的数据集, 证明了所提出的两种反问题求解方法的有效性. 本研究还进一步对比了所提出的两种方法, 对比结果表明, 利用K近邻模型结合有限元方法是预测骨骼肌本构参数的更精确有效的方法.
1 有限元模型的建立
骨骼肌的在生理学上的数学表述是一项复杂的任务, 考虑到其大变形行为, 骨骼肌的材料特性常被模拟为各向异性, 非线性和超弹性. 各向异性超弹性模型可以很好模拟骨骼肌的力学行为, 其本构方程如下[30]式中, $U$代表单位体积应变能, $C_{10} $, $k_{1} $, $k_{2} $和$\kappa $是与温度无关的材料常数; ${N}$是纤维的族数; $\overline {I}_{1} $是$\overline {{C}} $的第一应变不变量, 被定义为$\overline I_{1} =J^{-2/3}\left( {\lambda_{1}^{2}+\lambda_{2}^{2}+\lambda_{3}^{2}} \right)$ $(\lambda_{i} $表示主伸长); $J^{\rm el}$是弹性体积比; $\overline I _{4(\alpha \alpha )} $是$\overline {{C}} $和$A_{\alpha }$的应变伪不变量, $A_{\alpha } $是纤维束的方向向量. 从式(1)可以看出, 参数$C_{10} $和$k_{1} $是具有量纲的参数; 参数$k_{2}>0$是一个无量纲的参数; 参数$\kappa$ ($0\leqslant \kappa \leqslant 1/3$) 描述了纤维方向的分散度, 表征了纤维方向对组织力学性质的影响, 当$\kappa =0$时, 纤维沿着同一方向排列, 当$\kappa =1/3$时, 这意味着纤维是均匀分布的, 与纤维方向有关的应变能函数项为常数为0, 此时的应变能密度函数只与材料的变形有关. $D$反应的是材料受温度影响变化的程度, 因此在不考虑温度变化的不压缩条件下, 其本构方程表示如下[31]
各向异性骨骼肌中本构参数的确定是一项具有挑战性的任务, 由于骨骼肌组织的高含水量, 骨骼肌通常被认为是不可压缩的物质[32]. 所以本文提出的反演程序预测的本构参数包括: $C_{10} $, $k_{1} $, $k_{2} $和$\kappa $.
图1给出了为模拟骨骼肌单轴压缩试验的有限元模型. 骨骼肌被建模为超弹性各向异性材料. 有限元网格由3375个八节点六面体单元和4096个节点组成. 八节点六面体单元相对于四面体单元, 增加了节点的数目, 可以显著的提高计算精度. 因为拉伸试验机顶部和底部的压板是由钢制成的, 相比于新鲜的骨骼肌组织要硬的多, 所以在有限元仿真中他们被定义为刚体. 在压板与骨骼肌组织样本之间设置了摩擦系数以更准确的模拟实验.
图1
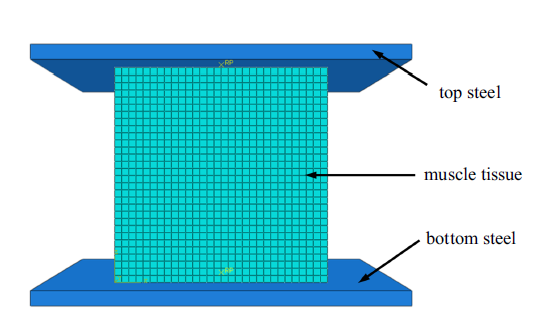
图1模拟实验设置的骨骼肌有限元模型
Fig.1Finite element model of skeletal muscles for simulating experiment
2 K近邻与支持向量机预测模型构建
KNN是一种常用的有监督学习的机器学习算法, 因为其具有从高维特征空间中恢复相似实例的简单性和直观性, 所以具有广泛的应用前景. 在KNN模型中, KNN对于给定测试样本, 基于距离度量找出训练集中与其最靠近的$K$个训练样本, 然后基于这$K$个``邻居''的信息来进行预测[33]. KNN模型的核心问题是$K$值的选取以及距离函数的选择. 在本研究中, 通过大量的数值实验, 最终确定$K=3$. 待求样本的状态向量与已知样本的状态向量之间的距离量度表示二者之间的相似程度. 常见的KNN模型的距离函数有欧式距离、曼哈顿距离、马氏距离、切比雪夫距离等. 在本研究中使用的欧氏距离公式如下: 假设待求样本的状态向量为 $x=\left( {x_{1} ,x_{2} ,\cdots,x_{n} } \right)$, 已知样本的状态向量为$y=\left( {y_{1} ,y_{2} ,\cdots,y_{n} } \right)$, 则其之间的距离$d$为支持向量机(SVM)也是一种常用的机器学习算法, 具有很强的非线性数据处理能力. 而SVR是支持向量机算法通过非线性回归分析的延伸[34], 是解决回归问题的一种有效方法. 支持向量机回归模型利用核函数将非线性问题转化为高维空间的线性问题, 是替代高维空间复杂计算的一种非常有效的方法[35]. 常见的核函数有线性核函数、多项式核函数、径向基核函数(radical basis function, RBF). 其中应用最广泛的是径向基核函数, 其已广泛应用在机械设备故障识别预测[36]. RBF定义为
这是一个从0变化到1的钟形函数. 其中$a$和$b$代表样本向量, $\gamma $为超参数.
3 预测结果与讨论
在本研究中, Liu等[27]对骨骼肌进行单轴压缩实验中获得的名义应力和主伸长数据集被分成两个子数据集, 分别对应于加载方向平行于纤维方向和加载方向垂直于纤维方向. 参数空间的必要参数为4个, 分别为$C_{10}$ (取值范围0.2$\sim$1.5 kPa), $k_{1}$ (取值范围0.05$\sim$0.4 MPa), $k_2$ (取值范围10$\sim$60), $\kappa$ (取值范围0.04$\sim$0.32). 本研究在参数空间中分别以固定增量采集了250组骨骼肌超弹性本构参数以使得采集到的本构参数能够充分代表整个参数空间, 其中$C_{10} $增量为$5.0\times 10^{-3}$ kPa, $k_{1}$增量为$1.4\times 10^{-3}$ MPa, $k_{2} $增量为0.2, $\kappa $增量为$1.12\times 10^{-3}$. 将收集到的骨骼肌组织的本构参数通过建立的有限元模型得到了相应的名义应力和主伸长. 之后以有限元模型收集的名义应力作为输入, 以相应的本构参数作为输出分别构建KNN模型和SVR模型. 为了更准确, 快速地进行回归, 本研究分别对名义应力和骨骼肌组织的本构参数进行归一化处理其中, $X'$是归一化后的取值, $X$是实际取值; $X_{\max } $, $X_{\min }$分别对应于名义应力和骨骼肌组织的本构参数的最大值和最小值. 当训练完成后, 将骨骼肌压缩实验中获得的名义应力数据集分别输入两个模型, 最终得到实验样本的本构参数.
为了进行监督学习, 需要选择合适的训练和测试数据进行预处理. 训练集用于构建KNN和SVR模型, 测试集用于验证模型的泛化能力. 训练集的大小必须代表整个数据集的特征. 训练集数目太少可能导致模型的学习能力差, 而训练集数目太多很可能导致验证困难并导致计算时间过长. 在本研究中, 80%的有限元收集到的数据被分给训练集, 其余20%被分配给测试集[37]. 图2给出了本文中提出的两个反向识别本构参数反演方法的流程图.
图2
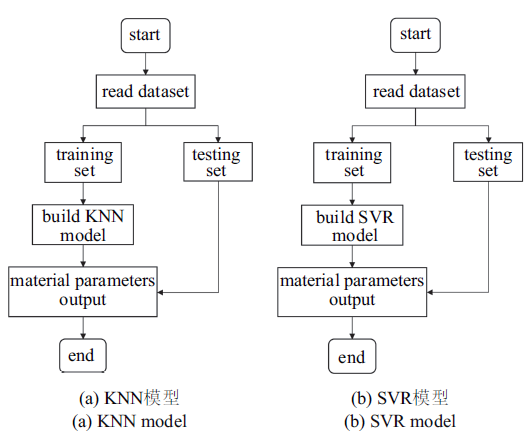
图2本研究中提出的本构参数识别方法流程图
Fig.2Flow chart of the parameter identification method proposed in this study
基于Liu 等[27]得到的骨骼肌压缩实验数据, 本研究对利用提出的参数识别程序得到的本构参数进行处理, 以获得一组本构参数, 这些参数既可以表示加载方向平行于纤维方向, 又可以很好地表示加载方向垂直于纤维方向. 在训练集完成之后, 测试集的数据用来评估KNN模型和SVR模型的准确性, 并比较本构参数的真实值和预测值. 在本研究中, 选用了决定系数($R^{2})$作为预测值准确率的指标. $R^{2}$反映了因变量的所有变化可以通过回归关系由自变量所解释的比例, 即利用数据的平均值作为误差基准, 观察预测误差与平均参考误差的偏离程度. $R^{2}$为
式中, $N$是样本的数量, $y_{i} $代表真实值, $y_{\rm pred} $代表预测值, $\overline y $代表真实值的平均值. 图3描绘了测试集中本构参数的真实值与预测值准确率的直方图. 从直方图中可以看出, 无论是KNN模型还是SVR模型测试集中各本构参数的准确率都很好.
图3
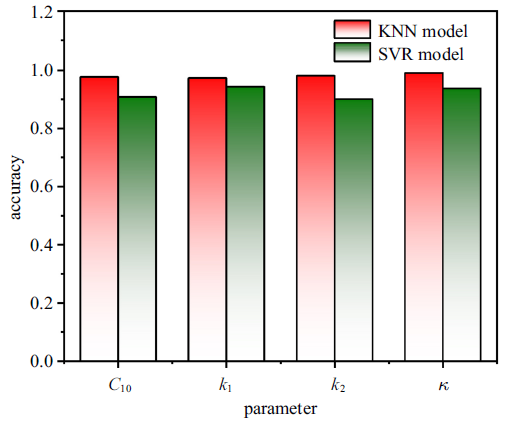
图3KNN模型和SVR模型测试集预测准确率比较
Fig.3Comparison of test set prediction accuracy between KNN] model and SVR model
图4给出了当加载方向平行于纤维方向和加载方向垂直于纤维方向时, 利用KNN模型获得的本构参数的计算响应曲线和实验曲线的比较以及仿真加载过程的名义应力云图. 从图4可以看出名义应力和主伸长之间存在非线性关系, 并且名义应力随着主伸长的增大而增大, 无论加载方向平行于纤维方向还是垂直于纤维方向, 利用KNN模型预测的骨骼肌本构参数的响应曲线与实验曲线吻合良好. 通过比较图4(a)和图4(b)可以发现, 当加载方向垂直于纤维方向时, 骨骼肌组织对压缩变形表现出更强的抵抗力.
图4
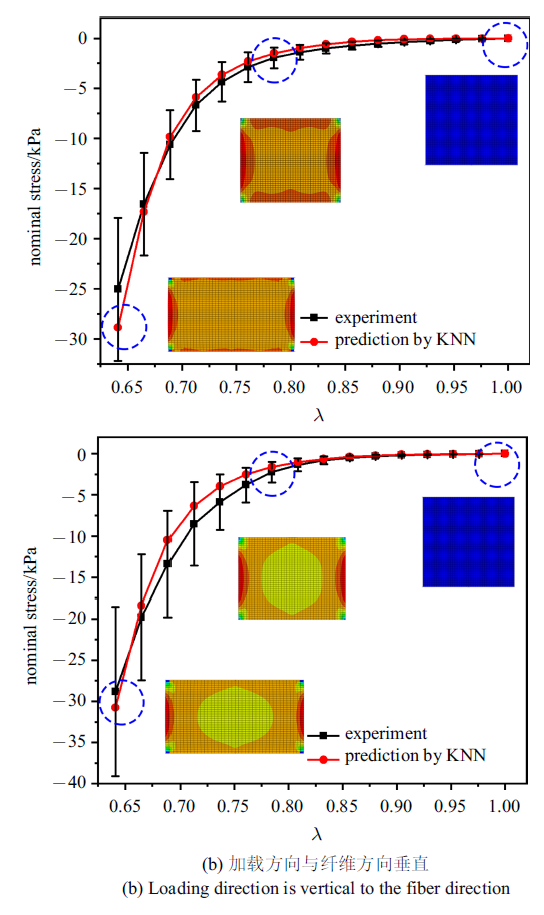
图4利用KNN模型获得的计算响应以及仿真加载过程名义应力云图
Fig.4Calculated response of KNN model and the nominal stress diagram of simulation loading process
图5给出了当加载方向平行于纤维方向和加载方向垂直于纤维方向时, 利用SVR模型获得的本构参数的计算响应曲线与利用KNN模型获得的本构参数的计算响应曲线和实验曲线的比较. 从图5中可以看出, 无论加载方向平行于纤维方向还是垂直于纤维方向, 利用SVR模型和KNN模型预测的骨骼肌本构参数的响应曲线与实验曲线吻合良好. 但在加载方向垂直于纤维方向时, 利用KNN模型预测的骨骼肌本构参数的响应曲线比利用SVR模型与实验曲线吻合更好.
图5
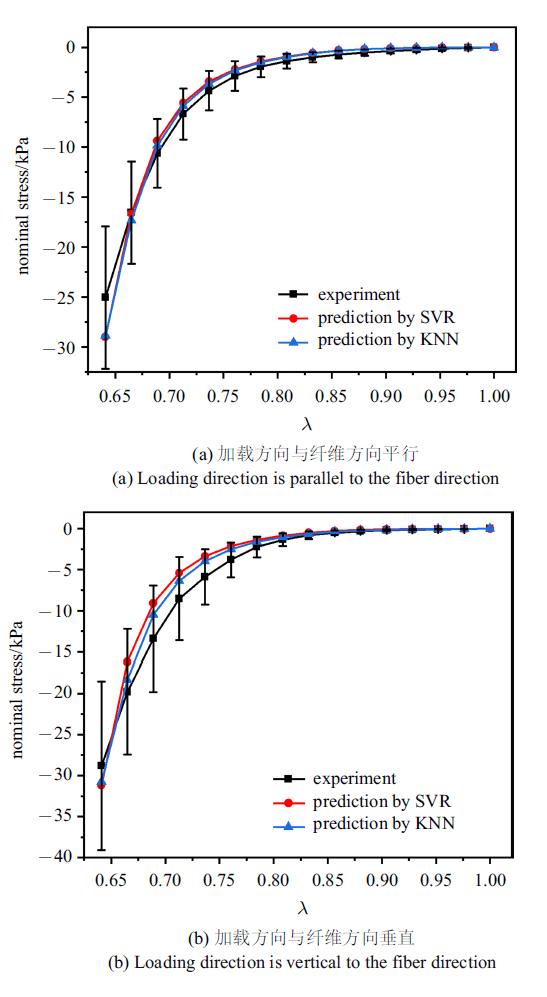
图5利用SVR模型获得的本构参数的计算响应与实验数据以及与利用KNN模型获得的计算响应对比
Fig.5Comparison of calculated response between KNN model and SVR model
为了更直观地观察真实值与预测值的拟合程度, 引入了2种统计指标, 包括相关系数($R)$和决定系数($R^{2})$. $R$是一个相关性指标, 可以用来衡量真实值与预测值之间的关系有多强, $R^{2}$是一个效率指标如前所述. $R$为
其中$\overline {y_{\rm pred} } $代表预测值的平均值. 表1给出了从统计指标方面比较了利用SVR模型参数识别方法和利用KNN模型参数识别方法.
Table 1
表1
表1KNN模型与SVR模型统计指标比较
Table 1
![]() |
新窗口打开|下载CSV
从表1可以更直观看出, 无论是加载方向平行与纤维方向还是垂直于纤维方向, KNN模型和SVR模型的相关系数$R$和决定系数$R^{2}$相差不大, 在加载方向垂直于纤维方向时, KNN模型的相关系数$R$和决定系数$R^{2}$比SVR模型好一些. 所以KNN模型和SVR模型都是预测本研究中骨骼肌组织的本构参数的准确有效手段. 本研究对利用KNN模型得到的骨骼肌组织的本构参数进行处理, 表2给出了骨骼肌组织本构参数的均值和标准差.
Table 2
表2
表2利用KNN模型获得的骨骼肌组织本构参数范围
Table 2
![]() |
新窗口打开|下载CSV
从表2可以看出$C_{10}$在0.508$\sim$0.684 kPa之间, $k_{1}$在373.361$\sim$422.881 kPa之间, $k_{2}$在31.235$\sim$36.394之间, $\kappa $在0.261$\sim$0.299之间.
4 结论
在本研究中提出了两种有效的反演方法来识别骨骼肌的本构参数. 一种是利用K近邻模型并结合有限元方法来识别骨骼肌的本构参数, 另一种是利用支持向量机回归模型并结合有限元方法来识别骨骼肌的本构参数. 本研究中的数据集由有限元方法来生成, 从骨骼肌组织的单轴压缩实验获得的实验数据用来验证本文提出的反演方法的准确性. 最后, 用相关系数$R$和决定系数$R^{2}$来直观地衡量本文提出的两种模型的准确性, 得出如下结论:(1)本文利用K近邻模型和SVR模型确定了可靠的骨骼肌组织的本构参数的范围, 所得到的本构参数的计算响应与骨骼肌组织的实验曲线吻合良好.
(2)利用K近邻模型和SVR模型在预测骨骼肌组织的本构参数都具有很大的潜力. 本文提出的两种模型和有限元相结合的反求方法也可以应用于其他具有超弹性结构的模型的软组织.
参考文献 原文顺序
文献年度倒序
文中引用次数倒序
被引期刊影响因子
PMID [本文引用: 1]

We employed a whole body magnetic resonance imaging protocol to examine the influence of age, gender, body weight, and height on skeletal muscle (SM) mass and distribution in a large and heterogeneous sample of 468 men and women. Men had significantly (P < 0.001) more SM in comparison to women in both absolute terms (33.0 vs. 21.0 kg) and relative to body mass (38.4 vs. 30.6%). The gender differences were greater in the upper (40%) than lower (33%) body (P < 0.01). We observed a reduction in relative SM mass starting in the third decade; however, a noticeable decrease in absolute SM mass was not observed until the end of the fifth decade. This decrease was primarily attributed to a decrease in lower body SM. Weight and height explained approximately 50% of the variance in SM mass in men and women. Although a linear relationship existed between SM and height, the relationship between SM and body weight was curvilinear because the contribution of SM to weight gain decreased with increasing body weight. These findings indicate that men have more SM than women and that these gender differences are greater in the upper body. Independent of gender, aging is associated with a decrease in SM mass that is explained, in large measure, by a decrease in lower body SM occurring after the fifth decade.
DOIURL [本文引用: 1]
DOIPMID [本文引用: 1]

Of the plethora of work performed analyzing skeletal muscle tissue, relatively little has been done in the examination of its passive material properties. Previous studies of the passive properties of skeletal muscle have been primarily performed along the longitudinal material direction. In order to ensure the accuracy of the predictions of computational models of skeletal muscles, a better understanding of the tensile three-dimensional material properties of muscle tissue is necessary. To that end, the purpose of this study was to collect a comprehensive set of tensile stress-strain data from skeletal muscle tissue. Load-deformation data was collected from eighteen extensor digitorum longus muscles, dissected free of aponeuroses, from nine New Zealand White rabbits tested under longitudinal extension (LE), transverse extension (TE), or longitudinal shear (LS). The linear modulus, ultimate stress, and failure strain were calculated from stress-strain results. Results indicate that the linear modulus under LE is significantly higher than the modulus of either TE or LS. Additionally, the ultimate stress of muscle was seen to be significantly higher under LE than TE. Conversely, the failure strain was significantly higher under TE than under LE.
DOIURL [本文引用: 1]
DOIURL [本文引用: 1]
DOIPMID [本文引用: 1]

Skeletal muscle relaxation behaviour in compression has been previously reported, but the anisotropic behaviour at higher loading rates remains poorly understood. In this paper, uniaxial unconfined cyclic compression tests were performed on fresh porcine muscle samples at various fibre orientations to determine muscle viscoelastic behaviour. Mean compression level of 25% was applied and cycles of 2% and 10% amplitude were performed at 0.2-80Hz. Under cycles of low frequency and amplitude, linear viscoelastic cyclic relaxation was observed. Fibre/cross-fibre results were qualitatively similar, but the cross-fibre direction was stiffer (ratio of 1.2). In higher amplitude tests nonlinear viscoelastic behaviour with a frequency dependent increase in the stress cycles amplitude was found (factor of 4.1 from 0.2 to 80Hz). The predictive capability of an anisotropic quasi-linear viscoelastic model previously fitted to stress-relaxation data from similar tissue samples was investigated. Good qualitative results were obtained for low amplitude cycles but differences were observed in the stress cycle amplitudes (errors of 7.5% and 31.8%, respectively, in the fibre/cross-fibre directions). At higher amplitudes significant qualitative and quantitative differences were evident. A nonlinear model formulation was therefore developed which provided a good fit and predictions to high amplitude low frequency cyclic tests performed in the fibre/cross-fibre directions. However, this model gave a poorer fit to high frequency cyclic tests and to relaxation tests. Neither model adequately predicts the stiffness increase observed at frequencies above 5Hz. Together with data previously presented, the experimental data presented here provide a unique dataset for validation of future constitutive models for skeletal muscle in compression.
DOIPMID [本文引用: 1]

The objective of this study is to identify the dynamic material properties of human passive muscle tissues for the strain rates relevant to automobile crashes. A novel methodology involving genetic algorithm (GA) and finite element method is implemented to estimate the material parameters by inverse mapping the impact test data. Isolated unconfined impact tests for average strain rates ranging from 136 s(-1) to 262 s(-1) are performed on muscle tissues. Passive muscle tissues are modelled as isotropic, linear and viscoelastic material using three-element Zener model available in PAMCRASH(TM) explicit finite element software. In the GA based identification process, fitness values are calculated by comparing the estimated finite element forces with the measured experimental forces. Linear viscoelastic material parameters (bulk modulus, short term shear modulus and long term shear modulus) are thus identified at strain rates 136 s(-1), 183 s(-1) and 262 s(-1) for modelling muscles. Extracted optimal parameters from this study are comparable with reported parameters in literature. Bulk modulus and short term shear modulus are found to be more influential in predicting the stress-strain response than long term shear modulus for the considered strain rates. Variations within the set of parameters identified at different strain rates indicate the need for new or improved material model, which is capable of capturing the strain rate dependency of passive muscle response with single set of material parameters for wide range of strain rates.
[本文引用: 1]
DOIURL [本文引用: 1]
[本文引用: 1]
[本文引用: 1]
DOIPMID [本文引用: 1]

The mechanics of passive skeletal muscle are important in impact biomechanics, surgical simulation, and rehabilitation engineering. Existing data from porcine tissue has shown a significant tension/compression asymmetry, which is not captured by current constitutive modelling approaches using a single set of material parameters, and an adequate explanation for this effect remains elusive. In this paper, the passive elastic deformation properties of chicken pectoralis muscle are assessed for the first time, to provide deformation data on a skeletal muscle which is very different to porcine tissue. Uniaxial, quasi-static compression and tensile tests were performed on fresh chicken pectoralis muscle in the fibre and cross-fibre directions, and at 45° to the fibre direction. Results show that chicken muscle elastic behaviour is nonlinear and anisotropic. The tensile stress-stretch response is two orders of magnitude larger than in compression for all directions tested, which reflects the tension/compression asymmetry previously observed in porcine tissue. In compression the tissue is stiffest in the cross-fibre direction. However, tensile deformation applied at 45° gives the stiffest response, and this is different to previous findings relating to porcine tissue. Chicken muscle tissue is most compliant in the fibre direction for both tensile and compressive applied deformation. Generally, a small percentage of fluid exudation was observed in the compressive samples. In the future these data will be combined with microstructural analysis to assess the architectural basis for the tension/compression asymmetry now observed in two different species of skeletal muscle.Copyright ? 2016 Elsevier Ltd. All rights reserved.
DOIURL [本文引用: 1]
[本文引用: 1]
DOIURL [本文引用: 1]
DOIURL [本文引用: 1]
[本文引用: 1]
[本文引用: 1]
[本文引用: 1]
[本文引用: 1]
[本文引用: 1]
[本文引用: 1]
[本文引用: 1]
[本文引用: 1]
[本文引用: 1]
[本文引用: 1]
[本文引用: 1]
[本文引用: 1]
[本文引用: 1]
[本文引用: 1]
[本文引用: 1]
[本文引用: 1]
DOIPMID [本文引用: 1]

Geometric features of the aorta are linked to patient risk of rupture in the clinical decision to electively repair an ascending aortic aneurysm (AsAA). Previous approaches have focused on relationship between intuitive geometric features (e.g., diameter and curvature) and wall stress. This work investigates the feasibility of a machine learning approach to establish the linkages between shape features and FEA-predicted AsAA rupture risk, and it may serve as a faster surrogate for FEA associated with long simulation time and numerical convergence issues. This method consists of four main steps: (1) constructing a statistical shape model (SSM) from clinical 3D CT images of AsAA patients; (2) generating a dataset of representative aneurysm shapes and obtaining FEA-predicted risk scores defined as systolic pressure divided by rupture pressure (rupture is determined by a threshold criterion); (3) establishing relationship between shape features and risk by using classifiers and regressors; and (4) evaluating such relationship in cross-validation. The results show that SSM parameters can be used as strong shape features to make predictions of risk scores consistent with FEA, which lead to an average risk classification accuracy of 95.58% by using support vector machine and an average regression error of 0.0332 by using support vector regression, while intuitive geometric features have relatively weak performance. Compared to FEA, this machine learning approach is magnitudes faster. In our future studies, material properties and inhomogeneous thickness will be incorporated into the models and learning algorithms, which may lead to a practical system for clinical applications.
DOIURL [本文引用: 1]
DOIURL [本文引用: 4]
[本文引用: 1]
DOIURL [本文引用: 1]
DOIURL [本文引用: 1]
DOIURL [本文引用: 1]
[本文引用: 1]
[本文引用: 1]
DOIURL [本文引用: 1]
DOIURL [本文引用: 1]
[本文引用: 1]
[本文引用: 1]
DOI [本文引用: 1]

Steel fibre reinforced concrete (SFRC) has been increasingly used in the engineering structures subjected to intense dynamic loads. In structural design and analysis, a dynamic increase factor (DIF) has been usually used to characterize strain-rate effect on the dynamic mechanical behaviour of SFRC. At present, several analytical equations that contain one or two variables have been utilised to predict the DIF values for material strengths of SFRC. However, this may lead to unsatisfactory results as the rate sensitivity of SFRC is influenced by multiple variables. In this study, a hybrid model, integrating random forest (RF) technique and firefly algorithm (FA), is proposed for predicting DIF values for SFRC. RF is utilized to discover the non-linear relationship between the influencing variables and DIF, while FA optimizes the hyper-parameters of RF. A total of 193 and 314 DIF data samples for compressive and tensile strengths of SFRC are retrieved from the reported studies to train and verify the proposed model. The input variables for the predictive model include strain rate, matrix strength, fibre dosage, and fibre properties (i.e. fibre shape, fibre aspect ratio and fibre tensile strength). The predicted results denote that the developed model is an efficient and accurate method to predict the DIF values for SFRC. Additionally, the relative importance of each input variable is investigated. It is found that the DIF values of SFRC are most sensitive to the matrix strength.