

The core influencing factors of housing rent difference in Guangzhou's urban district
WANG Yang

通讯作者:
收稿日期:2020-05-6修回日期:2021-03-26
基金资助: |
Received:2020-05-6Revised:2021-03-26
Fund supported: |
作者简介 About authors
王洋(1984-), 男, 黑龙江黑河人, 博士, 研究员, 硕士生导师, 研究领域为城市地理、城市与区域规划。E-mail:

摘要
关键词:
Abstract
Keywords:
PDF (2550KB)元数据多维度评价相关文章导出EndNote|Ris|Bibtex收藏本文
本文引用格式
王洋, 吴康敏, 张虹鸥. 广州中心城区住宅租金差异的核心影响因素. 地理学报, 2021, 76(8): 1924-1938 doi:10.11821/dlxb202108008
WANG Yang, WU Kangmin, ZHANG Hong'ou.
1 引言
全世界2010年约有12亿人通过租房解决居住问题[1]。2000年以来,随着城市化进程的推进,中国住房租赁需求迅速增长[2],住房租赁市场成为住房市场中不可或缺的重要组成部分。租房已成为中国特大城市居民的重要居住方式。2015年人口抽样调查数据显示北京、上海、广州、深圳的租房户占比分别达到33.58%、32.75%、40.58%、72.68%。其中,不少中心区的租房户占比超过一半。高房价下,中国特大城市无房户获得住房产权的难度越来越大,很多新市民或新移民通过租房解决居住问题已成为“常态”。近几年国家出台了一系列住房政策措施,例如限购限售、增大租赁住房比例、土地出让时规定开发商自持租赁比例等,意图通过保障和鼓励租赁住房的方式解决特大城市住房问题,也表明了中国特大城市“租房时代”的全面到来。但目前学术界对租房市场的研究还相对薄弱,租金是租房市场的核心指标,因而有必要对城市内部租金问题开展研究。城市内部不同住宅之间的租金水平存在很大差异[3,4,5,6,7],这是由住宅特征的异质性所决定[8,9,10,11,12]。分析住宅租金差异的影响因素是理解租房市场的重要前提。住宅租金是由多种因素共同决定的,特征价格理论模型是当前分析住宅租金因素最常用的思路。这些因素可归纳为以下3个方面:① 建筑特征因素,例如住房的建筑面积、楼龄、设施、内部设计、室内质量、建筑抗震质量等[13,14,15,16,17],以及住房所在小区的容积率、绿化率[18]和物业管理水平[19];② 邻里特征因素,例如公共交通便利性(尤其是地铁可达性)[2, 20-21]、道路可达性[6, 22]、就业中心便利性[2]、公共服务设施便利性[5]、商业中心可达性[17]、艺术和娱乐可达性[23]等,也包括噪音[24]、污水排水渠气味[25]等环境因素;③ 区位条件因素,距CBD距离是最为常见的评价方式[23]。理论上影响住宅租金的因素可包括方方面面。但在学术研究或现实的居民租房选择过程中,由于数据或信息获取的限制,不可能考虑到全部因素。因此,对租金核心影响因素的筛选显得尤为重要[26]。对住宅租金核心因素的分析是深入理解租金形成机制的重要切入点,也可为租房政策的精准化制定提供决策依据,具有现实意义。
更为重要的是房租和房价的形成机制不完全相同。房价不仅是居住效用的体现,还是产权福利(例如学区)和资产属性(投资价值)的体现,而房租主要受到实际居住效用的影响。例如,地铁站的规划预期可提升其附近房价,但难以提升房租(地铁建成投入使用后才能提升);同一片区相似特征的住宅,是否拥有优质学区对房价影响非常大,但对房租的影响有限。更为重要的是,购房者和租房者的住房选择机制也有所不同。正因如此,城市内部房价和房租之间的形成逻辑和主要影响因素必然存在差异[27,28]。近年来已有一些研究分析了城市内部住宅价格分异的影响因素[29,30,31,32],这为住宅租金的研究提供了参考和基础。但目前对房租的研究成果仍远远少于房价。因此,有必要进一步加强对城市内部住宅租金差异因素的理论与实证研究。尤其需在特征价格理论(适用于房价)模型的基础上,研究适用于住宅租金影响因素的特征租金理论与模型框架,这是本文的主要理论价值。
广州是中国租房户占比较高的特大城市之一,同时也是中国房地产市场化发展较早的具有“风向标式”的城市,其租房政策的制定也具有开拓性和创新性。另外,广州本身也是中国城市地理学关注的典型案例城市。因此,广州非常适合作为房租核心因素研究的典型案例城市。在影响房租的众多因素中,探索出核心因素是科学理解城市租房市场的关键问题,也是本文实证研究的价值所在。
鉴于此,本文构建了适用于城市内部住宅租金影响因素研究的特征租金理论框架及特征租金模型。以广州中心城区23126套待租住宅为基本研究单元,以2020年3月的整套住宅挂牌租金单价为价格数据,分析住宅租金空间差异格局与空间关联特征。基于广州租房市场特点和数据可获得性,构建广州中心城区住宅租金的影响因素指标体系,根据适宜的空间回归模型筛选房租的核心影响因素。研究在理论框架、样本数量和租金因素分析方面均有一定的进展,可为中国城市内部租房市场的研究提供理论与实证分析参考,具有学术意义。
2 理论框架
2.1 构建城市住宅的特征租金理论框架
对城市住宅租金影响因素的分析需要与之相适应的理论框架。尽管已有大多数研究成果都参考了特征价格理论的基本思路,但住宅买卖与租赁的逻辑特点存在差别,仍需要构建专门适用于住宅租金研究的理论框架。本文借鉴特征价格理论(适用于研究住宅价格)的基本思路和出发点,结合住宅租金形成的基本特点,提出适用于城市住宅租赁价格研究的特征租金(Hedonic Rent,也可称为享乐租金)理论框架。特征租金理论框架可借鉴特征价格理论。特征价格理论是当前住宅价格影响因素研究中最常用的理论[33,34],源于1966年以Lancaster为代表的新消费者理论,认为消费者并非从消费产品本身得到效用,真正的效用来自于产品所拥有的特征及其提供的服务[35]。Rosen基于供需理论,从需求方和供应方对产品特征的市场均衡展开分析,完成了特征价格分析的技术框架[36]。同类产品,其包含的特征组合不同,价格也不同[37]。在该理论框架下,住房特征通常可划分为结构(建筑)特征、邻里特征及区位特征三方面[38,39]。本文的理论框架可借鉴特征价格理论的基本思路,但住宅租金相比于住宅价格,更加注重居住的使用效用。
特征租金理论的假设包括:① 待出租住宅存在异质性特征;② 租房市场是完全竞争的市场;③ 出租方(房东)和承租方(租户)可以自由进出市场而不受约束;④ 出租方和承租方对待租房源的基本特征和租金拥有完全信息;⑤ 所有出租方和承租方对于每个住宅各类特征所带来的效用理解一致;⑥ 假定特征租金函数是曲线。
在上述理论假设的基础上,住宅承租方(从效用最大化出发)的最高出价和出租方(从利益最大化出发)的最低要价都是由该住宅所含的一系列特征综合决定的。承租方最高出价与出租方最低要价之间交汇形成的均衡租金就是该住宅的特征租金。多个住宅的特征租金点共同估计出了特征租金曲线。在城市住宅中,区位不同、便利性不同、面积不同、房龄不同,租金显然不会相同。租房市场双方对住宅的每一个特征都会做出估价,所有特征的价格集合形成住宅的市场租金。但是这一过程并不能在住宅租赁市场中显性的显示出来,而是隐含在住宅租金当中,所以该特征租金也称为隐含租金,可通过特征租金模型(例如回归模型)估计出来。
因此,特征租金理论可简要表述为:住宅具有多种特征(如面积、房龄、便利性、区位条件等)差异,是一种异质性的可出租商品。这些特征共同决定了该住宅的租金,住宅租金就是其拥有各类特征的综合体现(货币体现)。在该理论视角下,住宅特征对住宅租金的影响机制可简述为:在租房市场中,住宅特征决定了承租方的居住效用,进而影响了承租方对该住宅的租金认可价。同样,住宅特征也决定了出租方对住宅拥有的居住价值(租金)的判断,从而影响了出租方的租金要价。当承租方的认可价和出租方的要价出现市场均衡状态时,即形成了住宅租金(图1)。当住宅的某一特征变化时,住宅租金也相应随之变化。这就是特征租金理论的核心框架。该理论可作为解释住宅租金影响因素和形成机制的重要切入点。
图1
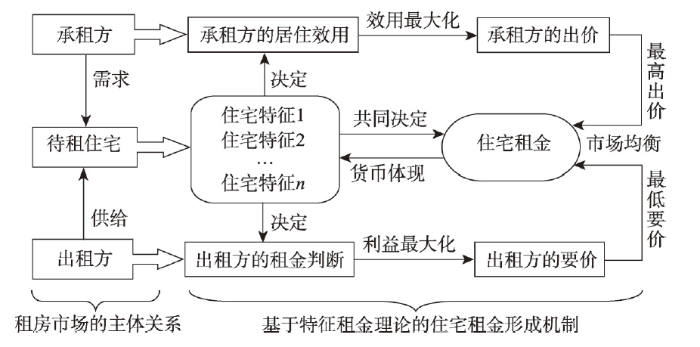
图1特征租金理论的核心框架
Fig. 1The core framework of hedonic rent theory
从特征租金的理论角度理解,租房者租赁住房的效用来自于该住宅所拥有的特征及租住感受,出租者的要价也受到住宅特征影响。这样,影响住宅租金的因素实际上就是住宅拥有的各类特征。这些特征在多大程度上影响住宅租金,就需要构建相应的特征租金模型进行定量分析。
2.2 建立特征租金模型及其要素构成体系
传统特征价格模型通常将住宅特征划分为结构(或建筑)特征、邻里特征及区位特征3方面,并且也广泛应用到住宅租金因素的实证研究中[3, 6]。但住宅买卖与租赁的逻辑存在差别,仍需要构建专门适用于特征租金理论特点的住宅特征要素构成体系。在特征租金理论视角下,本文提出将住宅特征划分为建筑特征、便利性特征、环境特征、区位特征4个方面,形成特征租金模型的“四分法”要素框架。其中,建筑特征可进一步划分为单户住宅特有特征和整栋住宅(或小区)共有特征;便利性是租房者的重要导向性因素,便利性特征主要包括交通出行便利性、就业便利性、就学便利性、商业服务便利性、公共服务与休闲游憩便利性等。对上述便利性的判断往往可以采用上述设施的可达性进行评价;环境特征可划分为两个方面:一是亲近优质环境或景观(例如绿地、水域,优质的环境等),二是远离消极的环境或景观(例如环境污染设施、厌恶型设施、混乱不安全的环境等)。这里的环境既包括自然环境和建成环境,也包括社会环境;区位特征取决于住宅所在的地理位置。通常可根据距市中心距离、所在板块、所属圈层地域等方式判断。因此,在构建特征租金模型时,可从上述方面选取相应的变量。本文构建的特征租金模型“四分法”要素框架与传统特征价格模型的“三分法”有所区别。特征租金模型突出便利性特征,并且将环境特征单列。原因在于:① 在传统的特征价格模型中,便利性相关因素往往被归入邻里特征或区位特征中。与住房买卖相比,便利性是租房户关注的重要方面。租房户在居住选择时,一般有“为了工作方便”“为了孩子上学方便”“为了消费方便”3种驱动导向,这都与便利性相关。因此,将便利性单独划分出来,符合租房的特点。② 居住环境是居民租房选择过程中的重要方面,往往倾向于亲近优质的环境景观,远离消极(污染)的环境景观。而在传统特征价格模型的“三分法”中,环境因素从属于邻里特征或区位特征。③ 本文提出的特征租金模型不再保留邻里特征,原因是,在中国城市,邻里特征可以有两种理解,一种是具有一定规模居住小区的自身特征,一种是住宅周边的设施(例如公共服务设施、公园、地铁)情况。前者也可被划分至结构(建筑)特征,后者也可被划分至区位特征。因此,为了避免歧义,将传统特征价格模型中邻里特征的要素进行拆分,这更适用于租房市场情形。
建筑特征与住宅及其所在楼盘(或小区)自身相关,租房者对建筑特征的选择属于“人房关系”研究范畴;便利性特征、环境特征、区位特征都由住宅所处的地理位置决定,租房者与上述3类特征的关系属于“人地关系”研究范畴,其影响机制也更加复杂,是地理学关注的重点。根据该特征租金模型框架,在分析住宅租金的影响因素时,可从如下方面构建其影响因素体系(表1)。该指标体系既包括实体特征因素,也包括社会文化因素。在实证研究中,可根据所在城市的特点和数据的可获得性,在该影响因素指标体系中遴选部分因素开展研究。
Tab. 1
表1
表1基于特征租金模型“四要素”的城市住宅租金影响因素分析体系框架
Tab. 1
要素 | 细分特征领域 | 涉及的主要因素 |
---|---|---|
建筑特征 | 单户住宅特有特征 | 建筑面积、所在楼层、朝向、装修程度、视野、通风、采光、住宅设施、户型格局 |
整栋住宅(或小区)共有特征 | 房龄、是否有电梯、物业管理水平、楼盘(或小区)档次、绿化水平、容积率、小区设施配套、停车位、开发商品牌、社会文化特征 | |
便利性特征 | 交通出行便利性 | 地铁便利性、公交站点便利性、道路交通便利性 |
就业便利性 | 主要就业地点的可达性 | |
就学便利性 | 幼儿园、小学、中学、大学的可达性 | |
商业服务便利性 | 商场、购物中心、超市、餐饮、娱乐、市场等商业服务场所的可达性 | |
公共服务与休闲游憩便利性 | 文化、体育、医疗等各类公共服务设施可达性、各类休闲游憩场所可达性 | |
环境特征 | 优质环境或景观 | 公园、水域、自然景区可达性,位于或亲近优质的建成环境或社会环境区域 |
消极环境或景观 | 受到工厂、物流中心、批发中心、厌恶型交通设施(例如飞机场、港口、火车站、汽车站、高速公路或高架路、加油站)、厌恶型市政设施(例如污水处理厂、垃圾处理场、燃气站、发电厂、变电站、高压走廊、殡仪馆、墓地、信号发射塔)的影响程度,受到环境污染、较差的建成环境、不安全的社会环境影响 | |
区位特征 | 地理位置 | 距市中心距离、所处圈层、板块、区域、方位 |
新窗口打开|下载CSV
基于特征租金模型的要素构成框架,特征租金模型的基本形式可表示如下:
式中:HR代表特征租金;f(B, C, E, L)代表多元回归模型,可以是传统OLS线性回归模型,也可以是空间回归模型;B、C、E、L分别代表建筑特征、便利性特征、环境特征、区位特征的指标集合,其中,B ={b1, b2, …, bn},C ={c1, c2, …, cm},E ={e1, e2, …, ep},L ={l1, l 2, …, l q};b、c、e、l分别为各自大类要素的具体特征构成指标,是租金的具体影响因素;n、m、p、q分别为相应大类要素的具体特征构成指标数量。
3 研究区域、数据与研究方法
3.1 研究区域与研究单元
以广州中心城区为研究区域,范围为西至广州市界,南至海珠区区界,东至天河区的前进街道、黄村街道、新塘街道、龙洞街道,北至白云区的京溪街道、同和街道、黄石街道、新市街道、棠景街道、松洲街道,总体上包括了广州环城高速公路围合区域及其附近街道。该范围参考了之前相关研究成果对广州功能地域的划分方式[40,41]。研究区面积为379.71 km2,常住人口596万人(广州市第六次人口普查数据)。从行政区划看,该中心城区范围包括越秀区、荔湾区、海珠区全部范围,天河区绝大部分范围以及白云区南部范围,是“真正意义上广州”。该范围可进一步划分为旧城(面积18.54 km2)、核心区(面积53.93 km2)和城区(面积307.24 km2)3个地域(图2)。以单套整租住宅为基本研究单元,共23126套。其中,旧城2680套,核心区8940套,城区11506套。租金以单套住宅每月的租金单价(元/m2)表示。图2展示的是单套住宅所在的小区/楼盘位置。图2
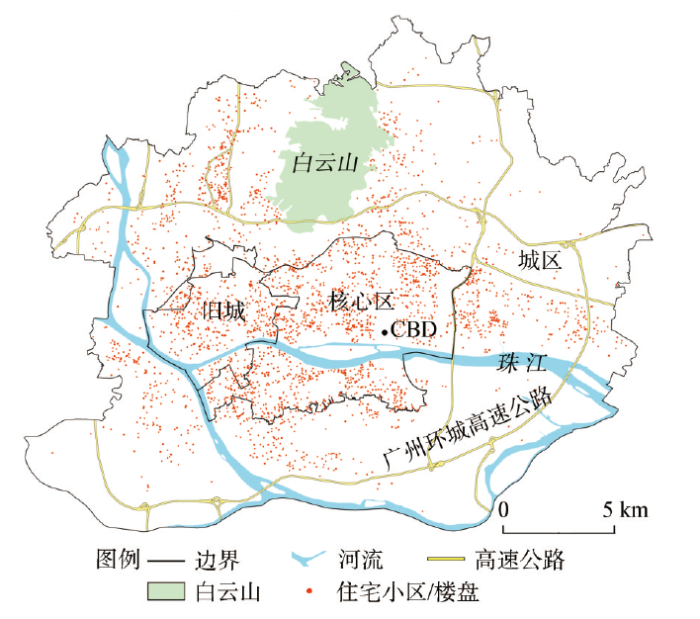
图2研究区范围及其单套住宅所在小区分布
Fig. 2The scope of the study area and the distribution of its estate of single house
3.2 影响因素的指标体系选取
基于本文提出的特征租金理论框架及其特征租金模型,从数据的可获得性和广州中心城区特点,选择12个影响因素指标。① 在建筑特征方面,选取建筑面积、朝向与楼层、房龄、物业与电梯4个因素,其中,前2项指标是单户住宅本身特有的特征,后2项指标是整栋住宅楼的共有特征,也可认为是居住小区(或居住组团)的共有特征。理论上,房龄与租金负相关,朝向与楼层、物业与电梯两项因素与租金正相关。建筑面积与租金相关关系的理论预期不明确,但从中国城市租房市场特征看,由于中小户型房源更受租户青睐,因此建筑面积越大,租金单价往往越低;② 在便利性特征方面,从公共交通、就业、教育、商业服务4个方面出发,选取地铁便利性、办公便利性、基础教育便利性、商业服务便利性4个因素,其中,地铁便利性代表住房的公共交通方便程度,其他3项因素分别代表租房选择时“为了工作”“为了孩子”“为了消费方便”的3种驱动导向。理论上,上述4种因素的便利程度越高,租金越高;③ 在环境特征方面,从接近优质环境设施和远离污染设施来考虑。分别选取公园可达性、厌恶型市政设施影响、工业污染影响3个因素指标。其中,公园可达性代表优质的环境设施,理论上对租金有正向促进作用。后两个因素代表负面的污染型设施,理论上对租金有负向影响;④ 在区位特征(指狭义的区位)方面,珠江新城是广州的CBD,其中的广州国际金融中心大厦是核心建筑,可以认为是广州的城市中心,以住宅与城市中心的直线距离代表区位条件。理论上,距离越大,住宅租金越低。每项因素的指标、计算方法或过程及对房租的预期影响方向如表2所示。Tab. 2
表2
表2广州中心城区住宅租金差异影响因素指标体系及其计算方法
Tab. 2
特征租金因素视角 | 影响因素指标 | 计算方法(分数赋值标准) | 预期影响方向 |
---|---|---|---|
HR1建筑特征 | F1 建筑面积 | 住宅的建筑面积数值 | 负向 |
F2 朝向与楼层 | 好朝向且优越楼层(9分);好朝向且一般楼层,或者一般朝向且优越楼层(7分);一般朝向且一般楼层(5分);差朝向且优越楼层(3分),差朝向且一般楼层(1分)。对朝向定义:好朝向为南、东南、西南,差朝向为北,其他情况为一般朝向;对楼层定义:对于楼梯楼,处于低楼层为优越楼层,中高层为一般楼层;对于电梯楼,中高层为优越楼层,低层为一般楼层。 | 正向 | |
F3 房龄 | 2020年减去住房建成时的年份 | 负向 | |
F4 电梯与物业 | 有电梯且有物业公司管理(9分);有电梯且为业主自筹/私人承办/单位代管物业(7分);有电梯但无物业管理,或者无电梯但有物业公司管理(5分);无电梯且为业主自筹/私人承办/单位代管物业(3分);无电梯且无物业管理(1分) | 正向 | |
HR2 便利性特征 | F5 地铁便利性 | 距地铁站200 m范围内(9分),距地铁站200~400 m范围内(7分),距地铁站400~800 m范围内(5分),距地铁站800~1500 m范围内(3分),距1500 m范围外(1分) | 正向 |
F6 办公便利性 | 将主要办公场所(写字楼、政府机关、事业单位、科技园)点数据生成核密度,并根据标准差均值面进行分级。住宅位于核密度3个标准差以上(含3个标准差)范围内(9分),位于2~3个标准差之间(7分),位于1~2个标准差之间(5分),位于0~1个标准差之间(3分),其他,即位于低于标准差均值的范围内(1分) | 正向 | |
F7 基础教育便利性 | 将小学、中学的点数据生成核密度,并根据标准差均值面进行分级。赋分方式与办公便利性相同 | 正向 | |
F8 商业服务便利性 | 将主要商业服务网点(市场、超市、商场、餐饮场所、娱乐场所)的点数据生成核密度,并根据标准差均值面进行分级。赋分方式与办公便利性相同 | 正向 | |
HR3环境特征 | F9 公园可达性 | 位于公园边界100 m范围内(9分),位于公园边界100~200 m范围内(8分),…,距公园800m范围外(1分) | 正向 |
F10 厌恶型市政设施影响 | 各类交通或市政类厌恶型设施及其影响范围设定如下:机场(半径5000 m),火车站(半径500 m),长途汽车站(半径500 m),高速公路和高架路(单侧200 m),铁路(单侧80 m),加油站(半径80 m),殡仪馆(半径1000 m),污水处理厂(半径2000 m),垃圾处理场(半径4000 m),变电站(半径500 m),高压走廊(单侧100 m)。在此基础上,赋分如下:未受到厌恶型设施影响(1分),受到1种设施影响(3分),受到2种设施影响(5分),受到3种设施影响(7分),受到4种及4种以上设施影响(9分) | 负向 | |
F11 工业污染影响 | 将工厂点数据生成核密度,并根据标准差均值面进行分级。赋分方式与办公便利性相同 | 负向 | |
HR4区位特征 | F12 距城市中心的距离 | 距广州国际金融中心大厦(珠江新城西塔)的距离 | 负向 |
新窗口打开|下载CSV
3.3 数据来源与处理
单套住宅(为挂牌待租房源)租金单价数据通过“贝壳租房”(广州)(建筑特征的4个因素(建筑面积、朝向与楼层、房龄、物业与电梯)数据来源于“贝壳租房”(广州)并进行整理。便利性特征和环境特征因素涉及的相关数据来源于百度地图POI点数据,线状和面状数据根据百度地图绘制而成(数据时间是2019年8月,其中地铁数据时间是2020年2月)。其中,污水处理厂、垃圾处理场、变电站、高压走廊的空间位置数据参考广州市城市总体规划的现状图绘制。由于便利性特征和环境特征因素的相关数据相对稳定,因而,存在一定的时间滞后性不会对研究结果产生影响。距市中心距离(区位特征)根据GIS量取每套住宅与广州国际金融中心大厦(珠江新城西塔)的直线距离得出。
3.4 研究方法
特征租金模型的基本形式就是回归模型,普通最小二乘法(OLS)是最常用的方法。如果住宅租金存在显著的空间相关效应,就需要采用空间回归模型,例如空间滞后模型(SLM)和空间误差模型(SEM)[44,45]。本文通过以上3种模型比选,选择最优模型,进而分析住宅租金的影响因素和影响方向。OLS是线性模型,可用于研究住宅租金(因变量)与一系列影响因素(自变量)之间的线性关系。OLS模型的假设前提是变量间相互独立。该模型中,变量的空间信息被忽略。OLS模型可表示为:
式中:s为广州中心城区待出租的住宅样本,共23126套;ys是第s套住宅的租金;Xs为租金影响因素i维行向量(i = 1, 2,…, 12),表示第i个影响因素变量在第s套住宅的观测值;β为i维列向量,是这些因素变量相对应的回归系数;ε是模型的误差项,εs~N(0, δ2I)表示误差项服从正态分布,并且方差一致,即误差与协方差矩阵的积为0;I代表单位矩阵。根据研究需要,本文将ys(因变量)和Xs向量的各自变量值取自然对数(即全对数模型),以消除量纲的影响,便于比较不同因素对住宅租金的影响程度。
在上述基本线性模型的基础上,空间滞后模型(SLM)考虑到了某住宅的租金对其他邻近住宅租金的影响,即空间溢出效应,是空间回归模型的一种形式。SLM模型可表示为[45,46,47,48]:
式中:ρ为空间自回归的系数值;Wsj表示空间权重矩阵。
在空间回归分析中,模型的独立误差项可能存在空间自相关。空间误差模型(SEM)可考虑到独立误差项的空间溢出效应。SEM的基本形式是[46, 48-49]:
式中:φ代表空间自相关误差项;λ是误差项的空间自相关系数。
4 结果与分析
4.1 住宅租金的空间差异格局与空间关联特征
根据数据分布特征情况和居民对租金的感受,将住宅租金由高到低划分为5个等级,阈值分别为100元/(m2·月)、80元/(m2·月)、60元/(m2·月)、40元/(m2·月)。不同租金等级的单套住宅数量和比例如表3所示。总体上,中低房租住宅数量最多,占全部住宅的36.43%,其次为中等房租住宅,占比也超过25%。不同地域范围的房租数量和比例特征有所差异:在外围城区,中低房租住宅占比高达50.88%;在核心区,高房租和中高房租住宅的占比分别高达25.21%和21.13%,体现出该区域整体租金水平较高;在旧城,其租金等级特征与外围城区类似,中低房租住宅占比最高。Tab. 3
表3
表32020年3月广州中心城区不同等级住宅租金的数量和比例
Tab. 3
租金等级 | 租金区间 (元/(m2·月)) | 研究区全部 | 旧城 | 核心区 | 外围城区 | |||||||
---|---|---|---|---|---|---|---|---|---|---|---|---|
数量(套) | 占比(%) | 数量(套) | 占比(%) | 数量(套) | 占比(%) | 数量(套) | 占比(%) | |||||
高房租 | >100.00 | 2834 | 12.25 | 306 | 11.42 | 2254 | 25.21 | 274 | 2.38 | |||
中高房租 | 80.01~100.00 | 3199 | 13.83 | 513 | 19.14 | 1889 | 21.13 | 797 | 6.93 | |||
中等房租 | 60.01~80.00 | 5898 | 25.50 | 923 | 34.44 | 2903 | 32.47 | 2072 | 18.01 | |||
中低房租 | 40.01~60.00 | 8424 | 36.43 | 835 | 31.16 | 1735 | 19.41 | 5854 | 50.88 | |||
低房租 | ≤ 40.00 | 2771 | 11.98 | 103 | 3.84 | 159 | 1.78 | 2509 | 21.81 | |||
总计 | 全部 | 23126 | 100.00 | 2680 | 100.00 | 8940 | 100.00 | 11506 | 100.00 |
新窗口打开|下载CSV
由于所处相同住宅小区/楼盘的单套住宅在空间上重合,为了便于租金的空间格局展示,本文计算了每个住宅小区/楼盘的平均租金单价(采用单套住宅租金的算术平均数计算),并通过GIS进行空间格局展示(图3)。广州中心城区住宅租金呈现明显的空间集聚性差异特征。高租金小区集聚分布在核心区的珠江北岸和旧城东部。低租金小区主要分布在外围城区。总体上,住宅租金水平呈现核心高,外围低的空间分异格局。
图3
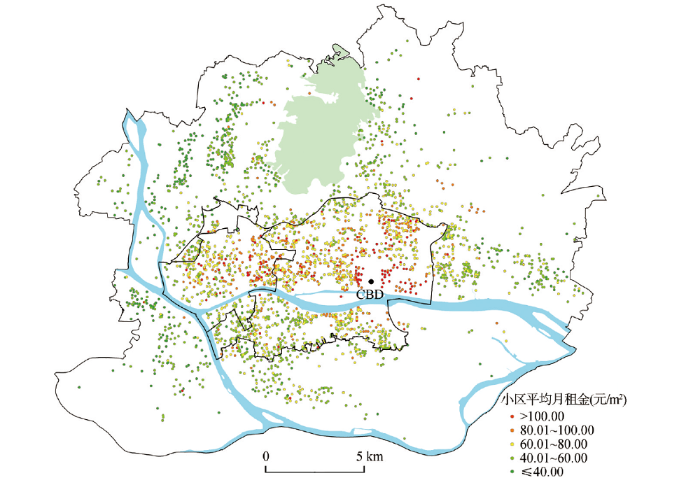
图32020年3月广州中心城区住宅租金的空间分布格局
Fig. 3The spatial pattern of housing rents in Guangzhou's urban district in March 2020
城市内部的住房市场往往存在较为显著的空间相互作用与空间关联特征[50],因此采用Moran's I指数[51,52]测度研究区内23126套住宅租金的空间自相关特征。空间权重矩阵以FD法为判断根据,门槛距离设为1000 m,得出Moran's I指数为0.8871,p值0.0000,Z统计值是558.2517,这说明广州中心城区住宅租金呈现显著的空间关联特征。因此,在后续影响因素回归模型选择时,就需要考虑到住宅租金间的空间相互作用,采用空间回归模型进行比选分析。
4.2 住宅租金差异的核心影响因素分析
对前述选择的12个影响因素指标(自变量)和住宅租金(因变量)数据进行对数标准化处理,以便消除量纲差异对结果带来的影响。首先对这12个指标进行共线性检验,检验结果表明,12个指标VIF值最大的因素(办公便利性)也仅3.429,表明这12个因素之间不存在明显的共线性,全部可以纳入回归模型进行因素分析。分别采用3种回归方式(OLS、SLM、SEM)的特征租金模型进行分析并筛选最优模型。结果(表4)表明,OLS、SLM、SEM这3个模型中,SEM模型的调整R2最大,达0.7354,Log likelihood值最高,为2033.11,AIC值最低,为-4040.23。由此判断,空间误差模型(SEM)的拟合程度明显优于其他两个模型,这也再次说明广州中心城区住宅间的租金水平具有显著的空间溢出效应。因此,采用空间误差模型的方法构建广州中心城区的特征租金模型,进而分析其影响因素。
Tab. 4
表4
表4广州中心城区3种回归模型的主要参数对比
Tab. 4
模型 | R2 | 调整R2 | AIC | 对数似然数 |
---|---|---|---|---|
普通最小二乘法回归模型(OLS) | 0.4324 | 0.4321 | 10734.50 | -5354.27 |
空间滞后模型(SLM) | 0.4864 | 0.4862 | 8485.33 | -4228.67 |
空间误差模型(SEM) | 0.7356 | 0.7354 | -4040.23 | 2033.11 |
新窗口打开|下载CSV
本文采用空间误差模型,得出广州中心城区住宅租金影响因素的回归系数及显著性等指标(表5)。结果表明,12个因素中,除了商业服务便利性和厌恶型市政设施影响2项因素外,其余10项因素对住宅租金的影响全部显著,且影响方向都符合理论预期。这其中,基础教育便利性在0.05水平上显著,其他9项因素都在0.01水平上显著。也印证了本文选择的影响因素指标体系基本合理。
Tab. 5
表5
表5基于空间误差模型的广州中心城区住宅租金影响因素回归系数
Tab. 5
因素类别(自变量) | 回归系数 | 标准差 | z统计值 | P值 |
---|---|---|---|---|
F1建筑面积 | -0.3494** | 0.0034 | -101.4040 | 0.0000 |
F2朝向与楼层 | 0.0196** | 0.0029 | 6.8631 | 0.0000 |
F3房龄 | -0.2807** | 0.0126 | -22.2924 | 0.0000 |
F4电梯与物业 | 0.1191** | 0.0094 | 12.6365 | 0.0000 |
F5地铁便利性 | 0.0484** | 0.0092 | 5.2342 | 0.0000 |
F6办公便利性 | 0.0983** | 0.0174 | 5.6511 | 0.0000 |
F7基础教育便利性 | 0.0391* | 0.0157 | 2.4926 | 0.0127 |
F8商业服务便利性 | -0.0246 | 0.0131 | -1.8787 | 0.0603 |
F9公园可达性 | 0.0240** | 0.0057 | 4.2099 | 0.0000 |
F10厌恶型市政设施影响 | 0.0130 | 0.0070 | 1.8574 | 0.0633 |
F11工业污染影响 | -0.0555** | 0.0070 | -7.9185 | 0.0000 |
F12距城市中心的距离 | -0.2060** | 0.0088 | -23.3809 | 0.0000 |
CONSTANT | 7.7150** | 0.0974 | 79.2189 | 0.0000 |
LAMBDA | 0.7314** | 0.0041 | 176.5310 | 0.0000 |
R2:0.7356;调整R2:0.7354;AIC:-4040.23;对数似然数:2033.11 |
新窗口打开|下载CSV
由于对自变量和因变量都进行了对数标准化处理,因此可根据回归系数绝对值大小判断各因素对住宅租金的影响弹性,进而探索对住宅租金有显著影响的核心因素。总体上建筑特征和区位特征对住宅租金的影响最强,便利性特征和环境特征的影响相对较弱。
在建筑特征方面,有3个因素的回归系数绝对值大于0.1,其中,建筑面积的回归系数绝对值最高,达-0.3494,说明住宅建筑面积每增加1%,租金单价降低0.3494%。建筑特征的4个因素对住宅租金的影响由强到弱分别为:建筑面积>房龄>电梯与物业>朝向与楼层。这说明,整套住宅的面积越小,租金单价越高。租户在租房选择过程中,在租金支付能力一定的前提下,更倾向于租住“中小户型住宅”,这符合中国城市租房市场的特征。其次,租户对电梯和物业管理情况也较关注,电梯代表了垂直交通的便利性,物业管理情况可在一定程度上反映其住宅所在小区/楼盘的建设档次。朝向和楼层对房租的影响相对较弱,其回归系数绝对值远低于建筑特征的其他3个因素。
在便利性特征方面,办公便利性的系数最高,其次为地铁便利性。而商业服务便利性不显著,基础教育便利性的显著性P值大于0.01。这说明,广州中心城区租户租房选择是以就业和通勤为核心导向,教育其次,而商业服务的影响不明显。就业和日常通勤主要都是“为了工作”。因此,从居民租房区位选择的3个驱动导向上看,“为了工作”占据主导,其次是“为了孩子”,而“为了消费方便”则不显著。商业服务便利性对住宅租金影响不显著的原因在于,商业服务设施对居住舒适程度来说是“双刃剑”,商业设施为日常生活带来消费方便的同时,也带来嘈杂、混乱和不安全感。尤其是过于接近较多的商业服务设施会降低居住品质。
在环境特征方面,厌恶型市政设施对租金的影响不显著。这说明随着城市人居环境的日益改善,通过各类防护措施,厌恶型设施对居住质量的影响逐渐减轻。另外,在一些区位条件较好的区域也不可避免地分布着一些厌恶型设施(如广州内环路、广深铁路、广州站、广州东站等),而租房户在租房选择过程中首先看重区位和便利性,厌恶型设施在租房决策过程中的权重相对较轻。公园可达性和工业污染影响对租金有显著影响。公园可为附近区域带来良好的环境和景观感受,也提供休闲空间,还可改善城市小气候。工厂则为周边环境和景观带来较多负面影响。工业污染影响的回归系数绝对值高于公园可达性,因此工业污染是影响租金不可忽略的环境因素。
在区位特征方面,距市中心距离对住宅租金有非常显著的负向影响。与市中心的距离每增加1%,租金下降0.2060%。该数值高于便利性特征和环境特征的所有因素,仅低于建筑特征的建筑面积和房龄,这印证了区位条件对住房租金的核心影响作用。
上述10个显著影响的因素中,建筑面积、房龄和距市中心距离是住宅租金的3个最关键核心影响因素,电梯与物业、办公便利性也是2个核心影响因素,而朝向与楼层、地铁便利性、基础教育便利性、公园可达性、工业污染影响是5个一般影响因素(图4)。鉴于此,在分析广州中心城区住宅租金差异的影响因素时,可重点关注建筑面积、房龄、距市中心距离、电梯与物业、办公便利性这5个核心因素。这其中,建筑特征的因素最多,达到3个,办公便利性和距市中心距离分别代表了便利性特征和区位特征。值得注意的是,环境特征中没有核心影响因素,说明环境特征对住宅租金的影响相对较弱。
图4
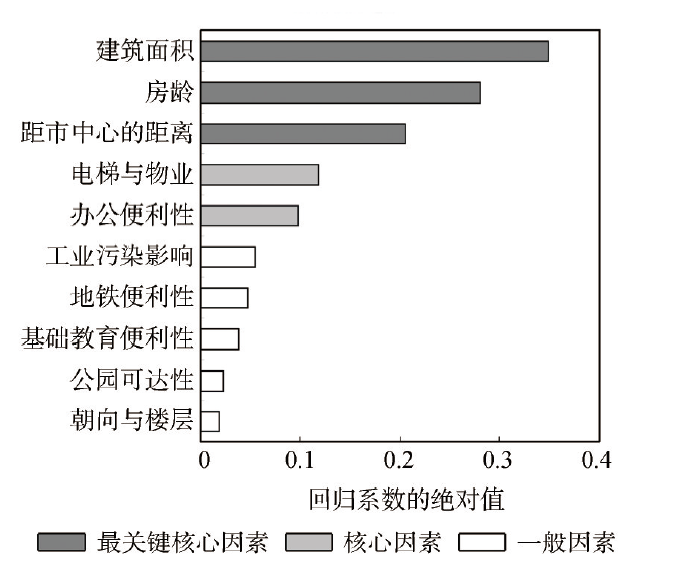
图4广州中心城区住宅租金影响因素的影响程度分级模式
Fig. 4Classification model of 10 influencing factors of housing rents in Guangzhou's urban district
5 结论与讨论
通过对广州中心城区住宅租金影响因素的实证研究,本文得出以下主要结论:① 在研究城市内部住宅租金影响因素时,可采用本文构建的特征租金理论及其特征租金模型。该模型将住宅特征划分为建筑特征、便利性特征、环境特征、区位特征4方面,形成了“四分法”要素框架,更适合租房市场的特点;② 广州中心城区中低租金水平的住宅数量最多,住宅租金呈现核心区高、外围城区低的空间分异格局,并具有显著的空间集聚和空间关联特征;③ 建筑特征(建筑面积、朝向与楼层、房龄、电梯与物业)、便利性特征(地铁便利性、办公便利性、基础教育便利性)、环境特征(公园可达性、工业污染影响)和区位特征(距市中心的距离)共4个方面的10个因素对广州中心城区住宅租金差异有显著影响,影响方向符合理论预期;④ 建筑面积、房龄和距市中心距离是住宅租金的3个最关键核心影响因素,电梯与物业、办公便利性也是核心影响因素。本文提出了特征租金理论框架和特征租金模型。相比于传统特征价格理论,特征租金理论更适用于解释和分析城市住宅租金的影响因素。在租房导向下,将住宅特征的构成要素进行“四分法”划分,突出了便利性特征和环境特征,这比传统特征价格模型(“三分法”)更符合中国城市租房市场的特点。未来研究城市住宅租金影响因素时,可采用本文提出的理论框架和住宅特征“四分法”划分视角,从中选取相应的指标进行研究。
实证研究结论厘清了广州租房市场的核心因素。建筑特征仍然是租房户租房选择过程中最看重的因素,说明住房的基本居住品质是租房户首先考虑的因素。这与张若曦等[17]对广州的研究结论一致。除了住房自身因素外,区位条件(距市中心距离)依然是租房户租房时考虑的关键因素,这与城市经济学的经典理论和基本认知相符,也表明区位条件在租房市场起到不可或缺的作用。办公便利性是租房市场中另一个重要因素,这在实证研究的角度回答了西方理论中关于居住区位选择的3个观点,即“为了工作”“为了孩子(基础教育方便)”“为了享受生活(消费方便)”。本文表明,在中国像广州一样的特大城市,“为了工作方便”是租房的最核心动机,是“三个导向”中的核心。该结论与已有基于南京[18]、武汉[19]的实证研究结果基本相符。在住宅租金研究领域,当数据获得受限,仅需粗略研究或开展基本理论分析(尽可能简化模型)时,可重点从这5个核心影响因素切入,进而将复杂问题简单化。住宅租金空间差异格局的研究结论表明,在分析住宅租金的影响因素时,需要考虑到变量或误差项的空间自相关效应对回归结果的影响,也说明了广州租房市场的空间相互作用关系。
值得注意的是,这些因素对住宅租金的影响可能存在空间异质性效应。本文尚未考虑到该效应,这是不足之处。未来可采用地理加权回归进一步分析影响因素的空间差异特征。社会与文化因素也对房租有不可忽视的影响,但由于数据获取限制,本文的实证研究没有纳入该类型因素,未来应进一步补充完善。另外,不同类型人群的住房租赁需求不同,未来可进一步采用问卷调查或访谈的方式分析租房选择的人群差异特征,这有助于加强对租金因素形成机制的理解。还可从住房子市场的视角出发,进一步研究不同类别住宅的租金影响因素差异,从多角度甄别核心影响因素。
本文的结论对中国特大城市租房市场管理和租赁住房(尤其是公共租赁住房)的精准化供应提供了参考依据。① 建议新建商品房须按一定比例配建租赁住房,并在租房需求旺盛的区域适当提高配建比例要求,以满足租赁市场需求;② 在新建公共租赁住房时,宜加大对整套中小户型租赁房源的供应,以便满足多数租房户的基本居住需求并减轻租房成本压力;③ 可通过增加优势区位用地收储力度、推进城市更新和直管公房改造等多种方式,更多地在优势区位或临近主要就业集聚区供应公共租赁房源,以满足租房户的工作便利性需求;④ 出台或细化相关法规及管理办法,在建筑质量、房屋设施、物业管理、通风采光、安全等方面明确租赁住房的入市标准,以保障租房户享有符合基本要求的居住品质和居住体验。
参考文献 原文顺序
文献年度倒序
文中引用次数倒序
被引期刊影响因子
DOI:10.1016/j.habitatint.2015.11.025URL [本文引用: 1]
URL [本文引用: 3]
DOI:10.1108/IJHMA-09-2018-0067URL [本文引用: 2]
[本文引用: 1]
[本文引用: 1]
DOI:10.2307/142337URL [本文引用: 2]
[本文引用: 2]
DOI:10.18306/dlkxjz.2019.12.001 [本文引用: 3]

Higher housing demand leads to housing price increasing rapidly, with lower housing affordability. Under these circumstances, renting a place has progressively become an alternative way for residents of cities. Urban transportation is one of the most significant influencing factors for housing rent, which needs to be examined at greater depth. This study used complex network analysis to explore how transportation centrality impacts housing rent. The conclusions are: transportation network shows significant impacts on housing rent, with higher impacts of public transport network than street network. Closeness in public transport has the highest impact on housing rent. Each centrality aspect influences housing rent differently, and relative accessibility in public transport network and transfer capacity in street network have the greatest impacts on housing rent. This article discussed two modes of urban transportation and their spatial characteristics. By refining the impacts of different transportation modes by network analysis, it provides a new perspective of urban transportation research and its spatial effects.
[本文引用: 3]
[本文引用: 1]
[本文引用: 1]
[本文引用: 1]
[本文引用: 1]
[本文引用: 1]
[本文引用: 1]
[本文引用: 1]
[本文引用: 1]
[本文引用: 1]
[本文引用: 1]
[本文引用: 1]
DOI:10.1080/24694452.2018.1470925URL [本文引用: 1]
DOI:10.1016/j.habitatint.2016.10.007URL [本文引用: 1]
DOI:10.1007/s10901-018-9607-4 [本文引用: 1]

This paper examines the rent determinants of sub-divided units (SDUs) in Hong Kong by hedonic pricing models. The supply of low-cost housing by SDUs is achieved at the expense of tenants' living quality and tenure security. Tenants have to make trade-offs of living space and housing facilities for lower rent, resulting in overcrowdedness and poor living environment. Some SDUs even lack basic facilities for sanitation and ventilation. As most previous property price studies presumed the presence of these basic facilities, SDUs provide a rare opportunity to examine the effects of their deficiencies on rent. Meanwhile, due to the illegality of the building works, most rental agreements are arranged informally. Relatively higher information and search costs are thus involved. Observable attributes are expected to exert significant impacts on rent as tenants mainly rely on their availabilities when they assess the SDUs. For SDUs with shared sanitation facilities, the inconvenience escalates with the subdivision density, which is expected to cause negative impact on rent. The results confirm the hypotheses that basic and observable housing facilities are important rent determinants and tenants have to pay higher rent to mitigate the inconvenience of shared facilities. This study is a pioneer attempt to give a fuller account of the SDU market landscape, providing empirical evidence about the effects of housing facility trade-offs of this informal rental market. Tenants are expected to make more compromises on housing qualities if the shortage of affordable housing worsens. The findings provide far-reaching implications for future research on housing values and affordable housing design.
DOI:10.1016/j.regsciurbeco.2006.06.009URL [本文引用: 1]
[本文引用: 3]
[本文引用: 3]
DOI:10.10282/dqxxkx.2018.180072 [本文引用: 2]

Urban residential rent space differentiation has always been one of the core contents of urban geography and urban economics. The residential rent has the inherent regularity in the spatial distribution, and explores this law information and its influence mechanism, which helps the government to formulate reasonable price regulation and land use policy. Taking Nanjing "one main city and three subsidiary cities" residential quarters as research unit, this paper simulates and estimates the spatial distribution of "one main city and three subsidiary cities" residential rents by using fishnet analysis and Exploratory Spatial Data Analysis (ESDA), and explores the influence mechanism of residential rent space difference from three aspects of residential area's location feature, architectural feature and neighborhood feature by using the geographical detector model. The results show that: (1) Residential rent in Nanjing generally shows an upward trend, showing a peripheral pattern of decentering from the main city to the vice city in space, and the spatial structure of residential rent gradually develops from single nuclear to dual nuclear with significant spatial heterogeneity in residential rent. (2) The residential rent shows obvious positive spatial correlation and regional agglomeration. The hot spots tend to weaken from the inner core to the vice cities, and the hot and cold spot spatial patterns are in a circle. (3) The traffic trend and central tendency are the factors that explain the rent of one house and three houses the most, and the second one is the explanation power of commercial facilities, financial facilities and residential houses. The influence intensity of the characteristic factors on the rents of the main city and vice-cities varies. Therefore, research on urban residential rental space distribution can promote the differentiation of space and an important driving mechanism, and can predict the basic trend in the future time city residential rental space distribution pattern evolution, can provide a forward-looking perspective and analysis tools to the study of urban social space.
[本文引用: 2]
[本文引用: 2]
[本文引用: 2]
DOI:10.1016/j.tra.2013.04.002URL [本文引用: 1]
[本文引用: 1]
[本文引用: 1]
DOI:10.1016/j.tranpol.2015.07.004URL [本文引用: 1]
DOI:10.1006/jhec.1996.0018URL [本文引用: 2]
URL [本文引用: 1]
DOI:10.1007/s10018-015-0136-zURL [本文引用: 1]
[本文引用: 1]
DOI:10.11821/dlyj020180352 [本文引用: 1]

At present, the development of China's housing sales market and housing rental market are unbalanced. The study on the spatial differentiation and relationship between housing prices and housing rents is of important reference value for the establishment of the "renting and purchasing" housing system. Using the data of housing prices and rental prices of residential units in 2016, and the 2006-2016 monthly housing sales price index and rental price index in Beijing, the paper explores the spatial differentiation and relationship between housing prices and rents from space-time scales, as well as the difference mechanism. The results show that: (1) The housing prices and rents both diminish from the multi-center to the outside. There are great differences in the distribution of housing prices and rents between the northern and southern parts of Beijing. Compared with the housing prices, rents show weaker spatial differentiation. (2) Housing prices have a moderate spatial correlation, which is mainly caused by uncertain factors (such as policy factors, and human factors), while rents have a strong spatial correlation, which is mainly caused by certain factors (such as location, and public facilities). (3) The profiles of housing prices and rents are different, since housing prices are sensitive to the school districts and urban large parks, but rents are more sensitive to the employment center and building age. The price-to-rent ratio is relatively homogeneous across the region, and the higher the housing price, the higher the price-to-rent ratio. (4) Beijing's housing sales market and housing rental market is in mutually independent development, in line with the characteristics of the dual market theory. The main reason for the above differences is that the market characteristics, the oriented population as well as the market development of the housing sales market and housing rental market are different.
[本文引用: 1]
DOI:10.18306/dlkxjz.2018.09.009 [本文引用: 1]

Under the background of rapid urbanization and housing market reform, urban housing and housing price have become a major issue related to the national economy and people's livelihood in China. The spatial differentiation of housing prices has become an important topic of research, which has been concerned by Chinese urban geographer and economic geographer in recent years. Few of the existing studies in China relied on long time series data to compare the spatial pattern, location influencing factors, and mechanism of change of urban housing selling and rental prices. As Nanjing City can represent the general development path of China's big cities, this study took 4560 residential communities in Nanjing as the total sample, and collected the average housing selling and rental prices for 30 quarters of 2009-2017. Data analyses show that there is a causality between the selling and rental prices, and the selling price shows a clear pattern that "fast rise" alternates with "relatively smooth," price levels whereas the rental price shows a more stable trend. The study chose areas where the sample communities are concentrated as focused research area and 6 characteristic time intervals to analyze the spatiotemporal differentiation and characteristics of change of housing selling and rental prices by employing the Kriging interpolation method. The results show that the spatial differentiation of housing selling price significantly intensified and high-value areas are more concentrated in Hexi New Town, Jiangxinzhou, and elite school districts in Gulou District; and based on the current situation of development, the high-value areas continue to transfer to the periphery of the city. Meanwhile, the spatial pattern of rental price shifted from gradually decreasing from city center toward outskirts to a more balanced structure of multi-centers both in the old and the new city areas. On this basis, an indicator system of location influence coefficient on housing selling and rental prices was built, focusing on the characteristics of houses and the "location" property. Through multiple stepwise regression analysis, it was found that central geographical variable has the highest degree of explanation power on selling and rental prices, while the explanatory power of supporting services on selling and rental prices is decreasing. With rapidly rising housing selling price in Nanjing, the significance of normal "location" coefficient on housing price differentiation is continually abating, but the decisive effect of some special "location" coefficients (such as school district and policy preferences) on selling price is becoming more prominent, further expanding the price-to-rent ratio, which also indicates the overall increase of housing price "risk" in Nanjing.
[本文引用: 1]
[本文引用: 1]
[本文引用: 1]
DOI:10.11821/dlxb201610006 [本文引用: 1]

Existing studies on the heterogeneities and determinants of urban housing price have put overwhelming emphasis on the 'location theory', which is generally descriptive rather than modeling in nature. More research which can systematically explore the spatial heterogeneities of urban housing price is needed. Given that housing price is, to some extent, the reflection of household activity demand, the paper therefore attempts to model urban housing price from this perspective on the basis of urban transport-land use interaction model. Taking Beijing as an empirical case, this research first develops a new measurement of accessibility which can directly depict the cost and possibilities to access opportunities of different activities such as employments, educational, shopping and medical services. According to the composition of different households and their different demands for activities, the paper then analyzes the relations between urban housing price and these demands. The empirical results demonstrate that the spatial pattern of housing price can be relatively well represented by the regression model. Among the four kinds of accessibilities, employment accessibility is found to be the most profound factor influencing housing price, while the next is followed by shopping, education accessibility. Medical service accessibility demonstrates the least influences on housing price. The approach and method proposed in this paper can well demonstrate how the distributions of different activities influence the spatial pattern of urban housing price and therefore have the potential to simulate the results of various urban land use policies, such as 'Decentralization Policies'. Finally, the policy implications of the model are discussed at the end of the paper.
[本文引用: 1]
[本文引用: 1]
[本文引用: 1]
DOI:10.11821/dlxb201810005 [本文引用: 1]

The growth and spatial differentiation of housing price is a theoretical issue continuously discussed in the field of urban geography, and even a practical problem relating to whether urban residents could realize the goal of living a good life in their own residences. The paper chooses 3761 independent residential districts (or residential clusters) in Nanjing as research objects, and analyzes sample data of 30 quarters in 2009-2017 of each residential district; on this basis, it focuses on the spatial differentiation pattern and evolution process of residential price in Nanjing. It finds that, on the whole, the housing price in Nanjing presents the staged rule of mutually alternative of "fast growth" and "relative stability" in one and a half year; while, high-price residences are mainly in Hexi New Town and the districts of famous schools, as well as high-rise service apartments distributed in inner urban areas, gated communities with landscape at the periphery, and high-grade villas in suburbs. Based on the consideration of the price level, location characteristics and building attributes of residential districts, this paper has comprehensively divided the residences in Nanjing into nine typical types, those are in inner city: (a) the multi-story traditional residences, (b) high-rise apartment residences, and (c) residences within the districts of famous schools; in the peripheral areas: (d) the urban new center residences, (e) landscape-area residences, (f) other gated residences; in the suburbs: g) the residences in core areas of new towns, (h) high-grade villa residences, and i) residences in urban fringe area. The comparison of the price growth process of different residences shows that, a close connection exists between the spatial attribute of residences (centrality, comfort, and scarcity) and the characteristics of housing price growth (rapidity, stability, sustainability). This paper summarizes four models of housing price growth of residences in Nanjing, namely, leading pattern, following pattern, growth-chasing pattern, and independent pattern. In addition, it analyzes the main internal and external driving forces for the differentiation of housing price growth of different types of residences. On this basis, it discusses the deep effects of the rapid and differentiated growth of housing price on the urban social space reconstruction in Nanjing, including enlarging the gap between the rich and the poor, crowding out the middle- and low-income population, solidifying the residential spatial differentiation, etc. The study on the growth of residential housing price and its aftereffects with Nanjing as an example, which is a typical big city in China, would have a certain reference for relevant research on the general fast growth and spatial differentiation of housing prices in China after the global financial crisis in 2008.
[本文引用: 1]
[本文引用: 1]
URL [本文引用: 1]
DOI:10.1086/259131URL [本文引用: 1]
DOI:10.1086/260169URL [本文引用: 1]
[本文引用: 1]
DOI:10.1016/j.habitatint.2019.03.008 [本文引用: 1]

Floor level is an important factor influencing housing price. However, most existing literature just treated floor level as a control variable and simply obtained a linear relationship between floor level and housing price. To fill this gap, this study uses the hedonic price model to deeply investigate floor level premium. The emphasis of this study is on landscape proximity and also the interactive effect of floor level and landscapes. A better understanding between floor level, landscape proximity and housing price is provided. Contrary to most existing studies, the findings suggest that the relationship between floor level and housing price is non-linear and is different in multi-storey buildings and high-rise buildings. As for the interactive effect between floor level and landscape proximity, we find that the amenity value of landscapes shows vertical heterogeneity at different floor levels within a building. On the other hand, landscape proximity significantly affects the relationship between floor level and housing price. This study addresses issues that were not studied before but are crucial to estimate the value of floor level and landscapes. It provides important implications for a better living environment and urban planning.
[本文引用: 1]
[本文引用: 1]
DOI:10.11821/dlyj201712016 [本文引用: 1]

<p>The spatial distribution of inner-city crime rates and crime risks is a topic of great concern in the fields of criminal geography and urban geography. Many empirical studies have shown that inner city criminal behaviors exhibit distinct spatial differentiation characteristics. This is of great importance to pattern analysis of real crime, especially has greater practical significance for its role in predicting patterns of urban crime risk. Because of restrictions related to the difficulty of acquiring real crime data and issues of confidentiality, there are currently few academic summaries of crime risk patterns and model results for Chinese inner-city residents. </p><p>Using social disorganization theory, the criminal risks of 118 neighborhoods were evaluated by the characteristics of social space in Guangzhou. The comprehensive evaluation system of property criminal risks and violent criminal risks are composed of social factors, including a floating population, sex and age, income, unemployment, education, matrimony, occupation, and housing. The scores of criminal risks in terms of neighborhoods were then examined based on information entropy. The heterogeneities of criminal risks in four layers of a circle were subsequently captured by GDI. On this basis, hot spots of criminal risks were explored by methods of spatial autocorrelation (GMI, LMI). Finally, the model and dynamics of spatial patterns were summarized according to the results obtained. </p><p>The results show that: (1) there exists obvious spatial differentiation for criminal risks in Guangzhou. The criminal risk in the old city is the highest, and that in the core area is the lowest. The differentiation of property risks and violent criminal risks in the inner circles are different, and (2) the spatial correlation and agglomeration of criminal risks are obvious in this city. Hot spots of criminal risks are located in the old city and the western fan-shaped area. (3) The curve corresponding to property criminal risk shows a "U" shape from the city center to the periphery. Moreover, the curve of violent criminal risk is a "wave" shape. Their spacial models show the "circle layer + fan shape".</p>
[本文引用: 1]
[本文引用: 1]
[本文引用: 1]
DOI:10.1016/j.jtrangeo.2015.04.008URL [本文引用: 1]
DOI:10.18306/dlkxjz.2016.08.005 [本文引用: 1]

The identification of different functional types of commercial center in a city is of great significance to understanding the spatial structure of business. Compared to the traditional methods, the analysis of big data produces more accurate result and is more convenient. Taking the 59125 POI (Point of Interest) data of Guangzhou City as the basic data, we identified the boundaries of the multi-functional commercial centers and explore their spatial structure and pattern by using the methods of kernel density analysis, statistical analysis, and nearest distance analysis. The result shows that: (1) The commercial area structure presents a clear dual-core distribution, with the Yuexiu District and the Tianhe District constituting the cores. (2) Different types of commercial centers show clear spatial variations. The urban services center and the public service center are located in the Yuexiu District and the business center and the financial center are located in the Tianhe District. Entertainment centers present the characteristics of dispersed clusters. (3) The spatial structure of business is a combination of zones and clusters. The urban services, public service, and business functions are concentrated in the inner zones and the entertainment functions can be found in all areas.
[本文引用: 1]
DOI:10.1016/j.apgeog.2017.09.010URL [本文引用: 1]
DOI:10.1016/j.apgeog.2016.12.003URL [本文引用: 2]
DOI:10.1111/gean.2006.38.issue-1URL [本文引用: 2]
[本文引用: 1]
[本文引用: 2]
[本文引用: 1]
DOI:10.1016/j.regsciurbeco.2003.09.001URL [本文引用: 1]
[本文引用: 1]
DOI:10.1111/gean.1995.27.issue-2URL [本文引用: 1]