

Clean energy power generation potential and value in Qinghai Province
MAO Aihan

通讯作者:
收稿日期:2020-07-17修回日期:2020-12-18网络出版日期:2021-01-25
基金资助: |
Received:2020-07-17Revised:2020-12-18Online:2021-01-25
作者简介 About authors
毛爱涵,女,内蒙古赤峰人,硕士生,研究方向为资源生态与自然资源保护。E-mail:

摘要
关键词:
Abstract
Keywords:
PDF (10773KB)元数据多维度评价相关文章导出EndNote|Ris|Bibtex收藏本文
本文引用格式
毛爱涵, 李发祥, 杨思源, 黄婷, 郝蕊芳, 李思函, 于德永. 青海省清洁能源发电潜力及价值分析. 资源科学[J], 2021, 43(1): 104-121 doi:10.18402/resci.2021.01.09
MAO Aihan, LI Faxiang, YANG Siyuan, HUANG Ting, HAO Ruifang, LI Sihan, YU Deyong.
1 引言
根据2019年《BP世界能源统计年鉴》[1],受经济发展、科技进步和人口增长影响,世界一次能源消费量不断增加,全球一次能源需求在2017年达到135.11亿t油当量,且在2018年增长了2.9%,其中2/3的增长来自中国、美国和印度3国,是2010年以来的最快增速。根据美国能源信息署预测结果,在当前能源发展情景下,如果不更改能源消费策略,2040年以前全球能源需求年平均增速为1.3%[2]。在这种情况下,以煤、石油、天然气等传统能源为主的一次能源将面临短缺和枯竭的风险。另外传统能源利用会释放CO2等温室气体到大气中,根据2014年4月正式发布的IPCC第五次评估报告(AR5)第三工作组(WGIII)报告,在2000—2010年间,人为温室气体排放量增加了100亿t CO2当量,其中47%来自能源供应部门[3]。温室气体排放的增加,加剧了全球气候变化,尤其是全球变暖的进程。为了缓解能源紧张和遏制全球气候变暖问题,人类正寻求新的能源解决方案。水电势能、太阳能、风能、地热能、生物质能、潮汐能等新型能源由于利用潜力巨大、环境污染少、可再生性强等特点,日益受到世界的广泛关注[4]。根据国家能源局发布的《中华人民共和国能源法(征求意见稿)》,清洁能源定义为开发利用、使用过程中环境污染物和CO2等温室气体零排放或者低排放的能源[5]。因此,评估清洁能源潜力,逐渐提高清洁能源在能源消费中的比重是节能减排、缓解全球环境压力的重要途径之一[6]。国外****大多通过模型模拟和空间分析等方法对清洁能源潜力进行研究。对于水电资源,许多****利用不同的水文模型,如ARIMA[7]、SWAT[8]或水文模型与GIS分析工具相结合[9,10]对水资源蕴藏量、地表径流量等参数进行估计,从而评估水电资源潜力。对于太阳能资源,****们计算了一些国 家[11,12]的太阳能发电潜力及太阳能碳减排潜力[13],他们主要依据遥感数据[14],利用地理信息系统(GIS)相关工具[15]对太阳能的能源量和空间分布进行评估。对于风能资源,研究者通常依据风速数据的统计特征[16,17,18],在此基础上根据资源、地形、环境和经济可利用性,利用GIS工具进行风能潜力研究[19]。对于生物质资源,针对不同国家的生物质蕴藏情况[20,21],研究者主要从已经充分利用的生物质资源和剩余的生物质潜力两方面进行评估,除此之外还对生物质碳减排能力进行了研究[22]。对于地热能资源,由于所处地理位置不同,并非所有国家都具有地热能,因此相关研究主要针对不同地区的地理条件和地热田分布情况[23,24],对地热能潜力进行了评估。上述研究工作对于不同清洁能源的潜力研究均有所涉及,但国外****的研究成果大多针对单一种类的清洁能源进行研究,或者针对不同国家的清洁能源发展情况进行研究,研究尺度大多数为国家,少有针对区域尺度的研究,内容上仍存在针对性不强或考虑因素不够全面等问题。
国内****对于清洁能源的潜力也展开了众多研究。对于水电势能发电潜力,研究者主要针对不同地区,如沃尔特河流域[25]、卡扬河[26]等,评估了水资源理论蕴藏量和水电资源开发潜力,通过与其他国家水资源开发程度的比较证明中国水电资源潜力巨大[27]。对于太阳能发电潜力,研究者在很多空间尺度上进行了研究,如全国范围[28]、黄河流域[29]、黔西南州[30]、华南地区[31]等,这些研究利用GIS等工具,主要从太阳辐射量的计算[32]、时空分布特征[33,34]、开发利用潜力[35,36]等方面评估太阳能应用潜力。对于风能发电潜力,研究人员大多选取风功率密度、风能可用时数等参数,从风能资源的丰富度、稳定度、开发适宜度等方面[37]对不同地区的风能潜力进行评估,如西藏地区[38]、京津冀及周边地区[39]。对于生物质能发电潜力,有研究者针对生物质某种资源禀赋开展了研究,如桉树[40]、人工湿地植物[41]等,还有研究者从不同尺度的生物质空间分布和蕴藏量等方面开展了生物质能应用潜力研究,如全国[42]、黑龙江[43]、黔西南州[44]等。对于地热能资源,中国****主要针对不同类型地热资源,即浅层地热能、水热型地热资源和干热岩地热资源进行了潜力评估[45],此外还评估了地热资源的分布、开发利用现状和前景[46,47,48]。国内****在清洁能源潜力方面的研究主要侧重于清洁能源的蕴藏量和开发程度,且能源种类通常比较单一,对于区域清洁能源的多种类综合分析相对较少,且少有研究定量评估清洁能源潜力价值。
国内外对于清洁能源价值的研究通常将能源本身与许多因素结合在一起进行分析,如清洁能源与碳价格的调控[49],清洁能源经济制度与碳排放交易[50],清洁能源消费与股票市场和经济增长的关系[51]。清洁能源碳减排价值受到越来越多的关注,主要原因在于清洁能源利用能够有效缓解CO2和污染物的排放[52]。尽管很多研究都涉及清洁能源的价值及碳减排价值,但很少有****将二者结合从定量角度衡量清洁能源潜力的经济价值。
青海省是青藏高原的重要组成部分,是中国重要的生态安全屏障、战略资源储备基地,生态环境在全国及全球都具有十分重要的战略地位。2018年青海省委十三届四次全会作出了坚持生态保护优先,推动高质量发展,创造高品质生活,即“一优两高”的战略部署。青海省作为中国西部地区资源丰富的省份之一,在发展清洁能源方面具有得天独厚的条件。由于其独特的地理位置和气候条件,青海省具有丰富的水资源、太阳能资源、风能资源、地热资源、动植物资源等,有巨大的开发利用潜力。在保护青海省生态环境的基础上,充分开发利用生态潜力是青海省实施区域可持续发展战略的重要支柱。
本文在研究对象上将青海省的5种清洁能源进行综合研究,对青海省2000—2018年太阳能、风能、水电势能、地热能、生物质能进行定量分析,并将其太阳能、风能、水电势能资源的时空分布可视化,对清洁能源发电潜力价值和碳减排价值进行评估,以期直观全面地了解青海省清洁能源潜力概况,为国家和青海省清洁能源开发利用设施布局及相关利用政策制定提供科学支撑,也为同类研究提供方法上的参考。
2 研究区概况、研究方法与数据来源
2.1 研究区概况
青海省地理坐标范围为89°35′E—103°04′E,31°36′N—39°19′N之间。中国七大地理分区将全国划分为华北、东北、华东、华中、华南、西南、西北,青海省位于七大地理分区的西北地区,深居内陆,远离海洋,地处青藏高原,属于高原大陆性气候。青海省地势总体呈西高东低,南北高中部低的态势,西部海拔高峻,向东倾斜,呈梯型下降,东部地区为青藏高原向黄土高原过渡地带,地形复杂,地貌多样。青海省是长江、黄河、澜沧江等重要水系的发源地,降水较多,水系发育,河网密集,河流比降大,境内多海拔5000米以上的高大山体,山上终年积雪,广布冰川,形成许多天然巨型固体水库。青海省地势高、空气稀薄、干燥少云,太阳辐射透过大气层的距离较海拔低的地区短,被大气层所反射和吸收的部分也较少,因而到达地面的辐射量相对增多,是中国太阳辐射量最多的地区之一。青藏高原地势开阔,海拔较高,对风的阻挡小,冬季东南部盛行偏南风,东北部多为东北风,风能资源丰富。青海省位于欧亚板块的交接地区,地壳运动较频繁,频繁的造山运动使得活跃地壳的热运动频繁,地热资源丰富。青海省蕴藏丰富的水能、太阳能、风能等可再生能源,是中国的能源资源储备大省,且具备大规模开发条件,是国家重要的清洁能源基地。
2.2 清洁能源潜力评估方法
2.2.1 水电资源潜力评估方法CLM(Community Land Model)陆面过程模式是国家大气研究中心(National Center of Atmospheric Research, NCAR)发展推广的陆面过程模式。CLM在综合了LSM(Land Surface Model)、IAP94(Institute of Atmospheric Physics Land Surface model)以及BATS(Biosphere-Atmosphere Transfer Scheme)等陆面模式优点的基础上,改进了一些物理过程参数化方案,并且加入了水文、生物地球化学以及动态植被等过程,是目前世界上发展最为完善而且也是最具发展潜力的陆面过程模式之一。因此,本文采用最新发布的CLM5.0[53],使用其尺度自适应河流运输模型(MOSART),通过运动波方法对河道汇流、随时间变化的河道径流速度、水深以及河道地表水储量进行模拟,进而得到青海省地表径流量,由地表径流的势能产生的最大水电势能作为水电开发利用潜力评估的依据。本文根据水资源量和水位差(高程)计算水电势能理论蕴藏量,计算方法如公式(1)所示:
式中:
首先,利用ArcGIS水文分析工具,基于青海省90 m数字高程模型(DEM)数据,以当年的地表径流为权重计算青海省汇流累积分布,并生成集水区分布图。水资源只有达到一定汇集量才具有发电能力。参考已有研究[54],确定该汇集量为1.28×106 m3,即达到或超过此阈值点具备建设水库使水势能转化为电能的条件。此外,由于当代可开发水电技术条件限制以及水能不能完全转变为电能,存在一定损失,因此真正能够被利用的水能资源为可开发水电势资源。全国技术可开发水能资源量占全国水能理论蕴藏量的40.67%[50],以此比例计算青海省水电势能发电量及其经济价值。
本文驱动CLM模型所用数据集如表1所示,水电势能潜力评估的空间分辨率为1 km,时间为2000—2018年。
Table 1
表1
表1本文CLM模型所需的基础数据及来源
Table 1
类型 | 名称 | 时空分辨率 | 时间范围 | 来源 |
---|---|---|---|---|
气象数据 | CLDAS东亚区域大气驱动场再分析数据集 | 0.0625°/hour | 2008—2018年 | 国家气象信息中心[55] |
中国大气强迫数据集 | 5 km/3hour | 2000—2010年 | Li等[56],Huang等[57] | |
下垫面数据 | 土地利用/土地覆盖数据 | 30 m | 2000、2005、2010、2015年 | 中国西部环境与生态科学数据中心[58] |
中国植被功能型数据 | 1 km | — | 中国科学院寒区旱区环境与工程研究所[59] | |
全球湖泊数据(GLDBv.2) | 1 km | — | Kourzeneva等[60],Choulga等[61] | |
DEM | 90 m | — | 地理空间数据云[62] | |
CLASS 叶面积指数 | 1 km/8day | 2000—2018年 | 国家地球系统科学数据中心[63] | |
河道数据 | 全球河道模块数据集 | 0.125° | — | Li等[64] |
新窗口打开|下载CSV
目前没有统一的划分标准来确定水电势能潜力的高低,参考中华人民共和国水利部批准的《水利水电工程等级划分及洪水标准》(SL252-2017)[65]、《水力发电工程地质勘察规范》(GB50287-2016)[66],本文拟将水电势能潜力划分为4个等级,如表2。
Table 2
表2
表2水电势能资源潜力分级
Table 2
指标 等级 | 发电装机容量(MW) | 水电势能发电量 (亿kW·h/km2) | 潜力 分级 |
---|---|---|---|
1 | ≤50.0 | ≤0.0010 | 贫乏 |
2 | 50.1~300.0 | 0.0011~0.0030 | 一般 |
3 | 300.1~1200.0 | 0.0031~0.0050 | 丰富 |
4 | >1200.0 | >0.0050 | 极丰富 |
新窗口打开|下载CSV
2.2.2 太阳能发电潜力评估方法
本文以太阳辐射数据为基础评估太阳能发电潜力,根据太阳辐射年等效利用小时数、理论装机量及其理论发电量量化青海省太阳能发电潜力。在计算青海省太阳能水平面总辐射、散射辐射的基础上,根据公式(2)计算倾斜面上月平均太阳辐照量[67]:
式中:
(1)年等效太阳能利用小时数
年等效太阳能利用小时数表示光伏电站发电量按照额定功率满发所计算的小时数,是电站选址的重要因素[57]。本文按照固定式斜面太阳能进行评估,根据固定斜面总辐射年总量,计算年等效利用小时数,计算方法如公式(3)所示:
式中:
(2)太阳能理论装机量
在考虑地形地貌对太阳能发电装机的约束后,计算一定区域太阳能电站的可装机量,计算方法如公式(4)所示:
式中:
Table 3
表3
表3不同坡向、坡度下的土地利用率
Table 3
坡向类型 | 坡度 | 土地利用率 |
---|---|---|
315°~45° | [0,10) | 1.0 |
315°~45° | [10,90) | 0.0 |
45°~135°,135°~225°,225°~315° | [0,10) | 1.0 |
45°~135°,135°~225°,225°~315° | [10,20) | 0.8 |
45°~135°,135°~225°,225°~315° | [20,30) | 0.6 |
45°~135°,135°~225°,225°~315° | [30,90) | 0.0 |
新窗口打开|下载CSV
Table 4
表4
表4不同土地利用类型的利用率
Table 4
土地利用类型 | 土地利用率 |
---|---|
湿地、森林、水体等 | 0.00 |
耕地、人造地表 | 0.05 |
灌木 | 0.10 |
草地 | 0.20 |
裸地 | 1.00 |
新窗口打开|下载CSV
(3)青海省太阳能发电潜力计算方法
太阳能发电潜力,即理论发电量,表示在单位面积下,考虑地形地貌和土地利用等约束条件,基于年等效利用小时数和理论装机量计算得到的年总发电量,计算方法如公式(5)所示:
式中:
本文2000—2007年短波辐射数据来自中国大陆地表强迫场的数据集,2008—2018年来自CLDAS东亚区域大气驱动场再分析数据集(表1)。
本文以国家气象科学研究院对总辐射的分级标准[70]对太阳年总辐射潜力进行划分,由高到低分为4级;对年日照时数和年有效日照天数的划分,参照针对太阳能光电利用区划制定的分级标准,由高到低分为4级[71];在此基础上,为进一步区分中国太阳能资源极端丰富区的分布范围,以各评价指标最高等级区内最大的一个四分位数作为分割阈值,将最高等级区再分为两级,即每项指标总共分5级[72](表5)。分级赋值之后,将各评价因子分值相加,其平均值即为各地太阳能资源开发潜力总分值,计算方法如公式(6):
Table 5
表5
表5太阳能资源潜力分级
Table 5
指标等级 | 分值 | 年总辐射(MJ/m2) | 年日照时数 | 年有效日照天数 | 潜力分级 |
---|---|---|---|---|---|
1 | 20 | ≤4200.0 | ≤1600.0 | ≤210.0 | 贫乏 |
2 | 40 | 4200.1~5200.0 | 1600.1~2000.0 | 210.1~270.0 | 一般 |
3 | 60 | 5200.1~6200.0 | 2000.1~2400.0 | 270.1~330.0 | 较丰富 |
4 | 80 | 6200.1~7000.0 | 2400.1~3200.0 | 330.1~350.0 | 丰富 |
5 | 100 | >7000.0 | >3200.0 | >350.0 | 极丰富 |
新窗口打开|下载CSV
式中:
2.2.3 风能发电资源潜力评估方法
风能资源分析的关键参数之一为风功率密度。风功率密度指的是气流在单位时间里垂直通过单位截面积的风功率[73],是评价一个地区风能资源潜力的重要参数[74]。本文根据国家标准(GB/T18170-2002)《风电场风能资源评估方法》计算风功率密度,如公式(7)所示:
式中:
式中:
式中:
2000—2007年风速、气压、气温数据来自中国大陆地表强迫场的数据集,2008—2018年来自第三次青藏高原科学考察气象数据中的东亚区域大气驱动场再分析数据集(表1)。
国家标准《风电场风能资源评估方法》(GB/T18710-2002)对风能资源的风功率密度等级进行了划分。只要风能资源达到3级及以上就能满足风电并网及大规模开发的基本要求,而2级风能资源的区域目前只适于发展中小型或离网型风电。在进行风能资源开发潜力分析时,既要考虑当前中国大规模开发风电的需要,也要考虑随着技术水平的提高及在风能资源等级较低的内陆地区发展小型离网风电的需要。
本文的风能资源评估参考了美国可再生能源实验室(NREL)的方法,从技术、经济及国家和地方政策角度综合考虑,中国陆地风能资源不可开发区域主要包括,海拔高度≥3500 m的地区,GIS坡度>4%的地区,以及水体、湿地、沼泽地等。因此根据年平均风速、并网条件与地形条件,将风能资源潜力划分为4个等级(表6)。
Table 6
表6
表6风能资源潜力分级
Table 6
指标 等级 | 年平均风速 参考值/(m/s) | 应用于并网风力 发电条件 | 潜力 分级 |
---|---|---|---|
1 | 5.1 | 不好 | 贫乏 |
2 | 5.6 | 较好 | 一般 |
3 | 6.0 | 好 | 丰富 |
4 | 6.4 | 很好 | 极丰富 |
新窗口打开|下载CSV
2.2.4 生物质能发电潜力评估方法
依据青海省统计数据,计算生物质能蕴藏量,并折算能源量。青海省生物质分为5类,分别是秸秆资源、畜禽粪便、薪柴林木、城市垃圾与废水。
(1)生物质蕴藏量
①农作物秸秆:主要包括粮食作物、油料作物、棉花、麻类和糖料作物5大类[75]。其用途广泛,除可以作为工业原料和畜牧饲料外,剩余部分都可以作为燃料使用。由于秸秆产量未列入国家有关部门的统计范围,其产量通常依据农作物的产量计算而得。计算方法如公式(10)所示:
式中:
Table 7
表7
表7不同农作物谷草比系数
Table 7
秸秆资源种类 | 草谷比系数 |
---|---|
小麦 | 1.1 |
杂粮 | 1.6 |
薯类 | 1.0 |
油菜籽 | 3.0 |
胡麻 | 2.0 |
新窗口打开|下载CSV
②畜禽粪便:是一种重要的生物质资源。畜禽粪便经干燥可直接燃烧供应热能,若经厌氧处理还可产生甲烷和肥料。根据各类畜禽每日粪便产生量和畜禽的饲养周期可以估算畜禽粪便排放量,计算方法如公式(11)所示:
式中:
Table 8
表8
表8单位畜禽饲养期内的粪便排泄量
Table 8
畜禽种类 | 饲养期内的粪便排泄量/kg |
---|---|
肉猪 | 1050.0 |
存栏猪 | 1460.0 |
牛 | 8200.0 |
羊 | 632.0 |
马 | 5237.0 |
肉禽 | 4.5 |
蛋禽 | 55.0 |
新窗口打开|下载CSV
③林木生物质:可以通过薪炭林,林业生产和更新剩余物以及灌木林、竹林等其他林木生物质资源来计算[80]。本文根据青海省统计年鉴数据计算了林业生产采伐剩余物、林木抚育间伐量、四旁树种植量3种林木生物质资源。计算方法如式(12)所示:
式中:F表示林木生物质资源实物量(t);
Table 9
表9
表9林木生物质能计算参数[81]
Table 9
种类 | bj | 折重 |
---|---|---|
采伐剩余物 | 40% | 1.17 t/m3 |
抚育间量 | 8 m/hm2 | 0.90 t/m3 |
四旁树 | 100% | 2.00 kg/株 |
新窗口打开|下载CSV
④城市垃圾:通过青海省统计年鉴中每年的城市垃圾清运量得到。
⑤城市污水:可以用于生产沼气,分为工业废水和生活废水两部分,计算方法如公式(13)所示:
式中:
(2)生物质折标能源量
根据以上各类生物质能资源的实物量,乘相应的折标系数,就可以得到不同种类生物质能折合成标准能源的总量。例如,在秸秆资源总量的基础上引入折标系数
式中:ES为农作物秸秆的折标能源总量;
畜禽粪便资源能源潜力量ET,林木资源能源潜力量
Table 10
表10
表10不同种类生物质能源的折标系数[82,83,84]
Table 10
生物质类型 | 折标能源系数 |
---|---|
麦秆、麻类秆 | 0.500 |
杂粮秆 | 0.050 |
薯类秆 | 0.486 |
油料秆 | 0.529 |
薪柴 | 0.571 |
牛粪 | 0.429 |
猪粪 | 0.471 |
马、羊、驴、骡粪 | 0.529 |
鸡粪 | 0.643 |
生活垃圾 | 0.143 |
工业沼 | 0.857 |
农业沼 | 0.714 |
新窗口打开|下载CSV
本文所使用的农作物秸秆产量、畜禽养殖数据、林木数据、城市垃圾清运量、城市污水数据、废水中COD平均含量,来自2000—2018年《青海省统计年鉴》、2000—2018年《中国统计年鉴》、2000—2018年《中国环境统计年报》。
2.2.5 地热能发电潜力评估方法
中国地质科学院水文地质环境地质研究所在青海省开展的“青海省地热资源调查评价”“贵德盆地深部水文地质调查”项目完成了青海省地热资源现状评价与区划工作[85]。由于数据获取的局限性,本文2000—2018年地热能发电潜力依据该研究探明的2012—2014年地热资源量平均值代替。
2.3 清洁能源价值研究方法
2.3.1 清洁能源发电价值为了便于比较,清洁能源价值计算参考2018年的价值指数。根据国家能源局发布《2018年度全国电力价格情况监管通报》[86],水电机组平均上网电价为267.19元/千kW·h,光伏发电平均上网电价为859.79元/千kW·h,风电机组平均上网电价为529.01元/千kW·h,生物质发电平均上网电价为677.99元/千kW·h,由于地热能目前没有统一的价格标准,故将地热能折算的标准煤完全燃烧释放的能量作为理论发电量,以2018年燃煤机组平均上网电价为370.52元/千kW·h对地热能潜力价值进行评估,计算方法如公式(15)所示:
式中:
2.3.2 清洁能源碳减排价值
根据2006年IPCC制定的《国家温室气体清单指南》中提供的参考方法,CO2排放量可以根据各种能源消费导致的CO2排放估算量加和得到[87]。根据中华人民共和国生态环境部发表的《2006—2017年度减排项目中中国区域电网基准线排放因子》可知,青海省属于西北区域电网,根据文件中的排放因子计算方法和原理,取2006—2017年平均值作为青海省CO2排放系数,即1.0012(tCO2/MW·h)。依据美国能源部发布的清洁能源碳排量数据,即生命周期内,不同清洁能源发电方式1 kW·h的发电量平均产生的CO2量[88],将其从与清洁能源量等值的传统能源利用的碳排放中扣除即可以得到清洁能源碳减排量。
为了便于比较,清洁能源碳减排价值计算均按2018年的价值指数进行计算。根据《2018年中国碳价调查》中的试点碳价范围[89]和中央财经大学绿色金融国际研究院发布的2018年试点碳市场价格得到2018年中国试点碳市场年度成交均价为23.445 元/tCO2。依据此对清洁能源的碳减排价值进行计算,如公式(16):
式中:
3 结果与分析
3.1 清洁能源发电潜力
3.1.1 水电资源潜力2000—2018年青海省水电势能潜力呈增加趋势(n=19, R2=0.49),且2016年之后,水电势能潜力增长较快,年平均增量为52.157亿kW·h,但空间分布格局未发生明显变化,高值区主要分布在青海省南部和北部部分区域。
2000—2018年青海省平均水电势能潜力的空间分布如图1所示。从全省整体空间分布情况来看,水电势能资源潜力在空间上呈不均匀分布,水电势能高值区分布在青海省南部及东部,低值区分布在西北部,由东南向西北逐渐递减。从州(市)尺度来看,玉树州和海西州是水电势能潜力的高值区,多年平均水电势能潜力最高值分别达到896亿kW·h/年、645亿kW·h/年,共占全省水电势能潜力总量的66%;果洛州、海南州、海北州为水电势能潜力的中值区,共占全省水电势能潜力总量的27%;黄南州、海东市、西宁市为水电势能潜力的低值区。从生态功能区尺度来看,三江源地区是水电势能潜力的高值区,多年平均水电势能潜力最高值达2502.96亿kW·h/年,占全省水电势能潜力总量的74%;东部干旱山区为水电势能潜力的中值区,占全省水电势能潜力总量的13%;柴达木地区、青海湖流域和祁连山地区为水电势能潜力的低值区。
图1
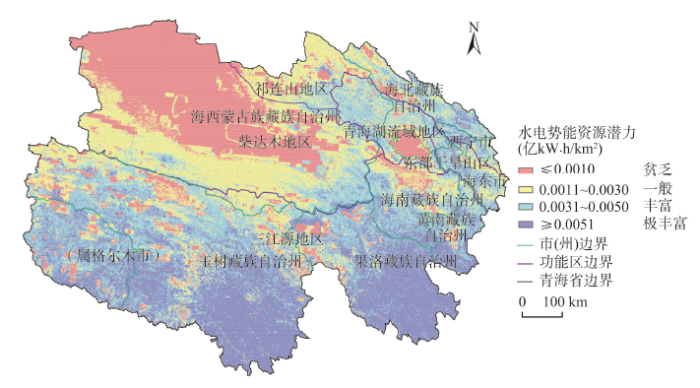
图12000—2018年青海省多年平均水电势能潜力的空间分布
Figure 1Spatial distribution of the average hydropower potential energy in Qinghai Province, 2000-2018
水电势能潜力在时间上呈增加趋势,首先是年际降水量呈增加趋势,使得地表水资源量增加,进而影响水电势能变化;其次是在全球气候变暖的大背景下,虽然蒸发量在增大,但同时会使冰川冻土融水量增加,从而使液态水资源总量在一定时期内增加[90]。水电势能潜力在空间分布上呈现东南高西北低的格局。首先是降水的空间分布差异,由于所处地理位置,青海省东南部靠近横断山区,南北走向的横断山可以阻隔东南季风,但西南季风会沿河谷抬升,在青海南部形成降水,降水分布格局决定了径流的大体变化趋势,形成东南地区水电势能资源潜力较大的空间格局;此外,地表径流上下断面水位差也是重要影响因素,青海省东部地区为青藏高原向黄土高原过渡地带,地形复杂,河流比降大,从而形成东南高西北低的水电势能潜力分布。
3.1.2 太阳能发电潜力
2000—2018年的太阳能发电潜力进行拟合(n=19, R2=0.89),结果表明,2000—2005年太阳能发电潜力呈现减少趋势,2005—2015年呈现增加趋势,2015—2018年呈减少趋势,但2000—2018年整体呈增加趋势,空间分布格局变化不大,且太阳能发电潜力较稳定。
2000—2018年青海省平均太阳能发电潜力的空间分布如图2所示。从全省整体空间分布来看,太阳能资源在空间上呈现不均匀分布,从西北到东南呈递减趋势。从州(市)尺度来看,海西州是太阳能发电潜力的高值区,多年平均最高发电潜力达150.66亿kW·h/年,占全省太阳能发电潜力总量的的65%;玉树州是太阳能发电潜力的中值区,占全省太阳能发电潜力总量的22%;果洛州、海南州、海北州、黄南州、海东市、西宁市是太阳能发电潜力的低值区。从生态功能区尺度来看柴达木地区是太阳能发电潜力的高值区,多年平均最高发电潜力达123.52亿kW·h/年,占全省太阳能发电潜力总量的53%;三江源地区是太阳能发电潜力的中值区,占全省太阳能发电潜力总量的39%;祁连山地区、青海湖流域、东部干旱山区是太阳能发电潜力的低值区。
图2
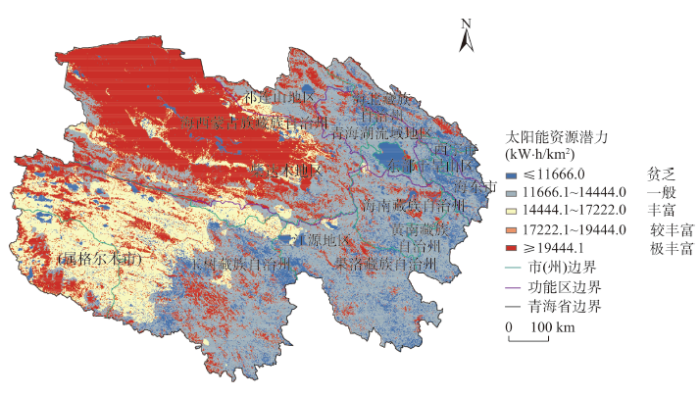
图22000—2018年青海省多年平均太阳能发电潜力的空间分布
Figure 2Spatial distribution of the average solar power generation potential in Qinghai Province, 2000-2018
太阳能发电潜力在时间上呈现增加的趋势主要受年际天气状况影响,年等效利用小时数增加,进而带来太阳能发电潜力呈增加趋势。太阳能发电潜力在空间分布上呈现西北高东南低分布的格局主要受纬度位置影响,青海省地处中高纬地带,太阳辐射强度大,光照时间长;其次是受海拔高度影响,青海省海拔高,空气稀薄,大气对太阳辐射的阻挡作用弱,到达地面的太阳辐射强。此外受地形和土地利用类型影响,青海省兼具青藏高原、内陆干旱盆地、黄土高原三种地形地貌,地形和土地利用类型影响太阳能装机情况,进而形成太阳能发电潜力呈西北高东南低的空间格局。
3.1.3 风能发电潜力
2000—2018年青海省风能发电潜力呈下降趋势(n=19, R2=0.449),平均每年下降约0.384亿kW·h,但空间分布格局变化较小。
2000—2018年青海省平均风能发电潜力的空间分布如图3所示。从全省空间分布来看,风能发电潜力在空间上呈不均匀分布,整体上为西部高,东部低。从州(市)尺度来看,海西州是风能发电潜力的高值区,最高每年达1.82×106 W/m2,占全省风能发电潜力总量的48%;玉树州是风能发电潜力的中值区,占全省风能发电潜力总量的30%;果洛州、海南州、海北州、黄南州、海东市、西宁市是风能发电潜力的低值区。从生态功能区尺度来看,三江源地区始终处于风能发电潜力的高值区,多年平均最高发电潜力达1.77×106 W/m2 ,占全省风能发电潜力总量的56%;柴达木地区是风能发电潜力的中值区,占全省风能发电潜力总量的32%;祁连山地区、青海湖流域、东部干旱山区是风能发电潜力的低值区。
图3
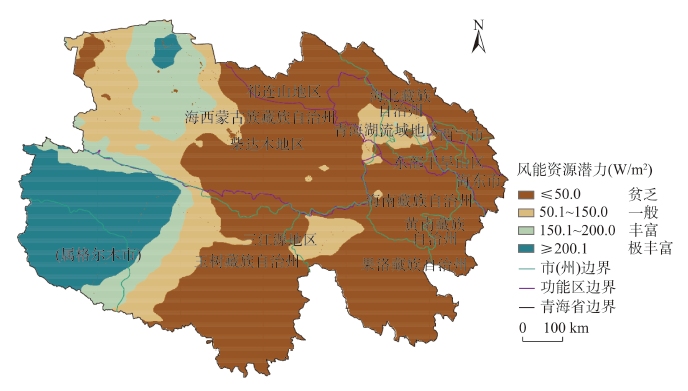
图32000—2018年青海省多年平均风能发电潜力的空间分布
Figure 3Spatial distribution of the average wind power generation potential in Qinghai Province, 2000-2018
风能发电潜力在空间上呈现西高东低的格局,主要受地形等因素的影响,青海省西部海拔较高,由于地形的抬升更易形成大风,因此海西州西部、玉树州西部、柴达木地区风能资源丰富。青海湖东北面、西南面均有山体,气流通过这种特殊地形条件时会产生狭管效应而导致风速增加,同时由于东南端地势较高,气流会在此产生堆积作用而加速,另外由于气流流经光滑的湖面时也会加速,因此青海湖周边地区风能潜力较大。
3.1.4 生物质能发电潜力
2000—2018年青海省生物质资源折合标准煤能源总量如表11所示。
Table 11
表11
表112000—2018年青海省生物质资源折合标准煤总量
Table 11
年份 | 秸秆/万t | 畜禽粪便/万t | 林木/万t | 城市垃圾/万t | 废水/万t | 总和/万t |
---|---|---|---|---|---|---|
2000 | 89.74 | 2157.24 | 2876.32 | 13.69 | 2.51 | 5139.50 |
2001 | 77.77 | 2535.06 | 3380.08 | 10.84 | 2.54 | 6006.30 |
2002 | 71.86 | 2578.78 | 3438.37 | 11.30 | 2.51 | 6102.82 |
2003 | 74.23 | 2500.90 | 3334.53 | 7.99 | 2.48 | 5920.13 |
2004 | 80.91 | 2717.14 | 3622.85 | 8.25 | 3.03 | 6432.18 |
2005 | 90.47 | 2755.69 | 3674.26 | 7.78 | 5.60 | 6533.80 |
2006 | 70.45 | 2787.66 | 3716.88 | 8.55 | 5.75 | 6589.30 |
2007 | 86.55 | 2897.62 | 3863.49 | 9.25 | 5.91 | 6862.81 |
2008 | 92.84 | 2478.19 | 3304.26 | 9.09 | 5.80 | 5890.19 |
2009 | 98.31 | 2969.49 | 3959.31 | 12.50 | 5.92 | 7045.53 |
2010 | 96.41 | 3006.90 | 4009.20 | 11.88 | 8.01 | 7132.39 |
2011 | 92.62 | 3067.77 | 4090.36 | 11.88 | 8.01 | 7270.65 |
2012 | 90.49 | 2964.33 | 3952.44 | 9.48 | 8.07 | 7024.82 |
2013 | 89.60 | 2924.25 | 3899.00 | 10.60 | 8.04 | 6931.48 |
2014 | 87.27 | 3025.99 | 4034.65 | 11.10 | 8.16 | 7167.17 |
2015 | 84.69 | 3172.70 | 4230.27 | 11.75 | 8.11 | 7507.52 |
2016 | 84.20 | 3279.38 | 4372.50 | 11.73 | 5.46 | 7753.27 |
2017 | 82.44 | 3357.87 | 4477.17 | 11.11 | 4.47 | 7933.06 |
2018 | 87.95 | 3138.24 | 4184.32 | 16.23 | 4.47 | 7431.21 |
新窗口打开|下载CSV
2000—2018年的青海省生物质资源呈上升趋势(n=19, R2=0.807),林木资源和畜禽粪便资源总量占比较高,其次是秸秆资源、城市垃圾、废水。影响生物质资源的因素有很多,如光热组合、自然地理条件、种植情况等。生物质能源呈上升趋势与人口数量增多有密切关系,2000—2018年青海省常住人口数量由503万增长到603.23万,随着人口数量增多,城市垃圾、生活污水和工业污水的排放量增加,作物种植、畜禽饲养的水平也有所提高,使生物质能源成为青海省具有较大发展潜力的的清洁能源之一[84]。
3.1.5 地热能资源潜力
青海省地热资源总储量为1.43×1020 J,折合标准煤为8.10×109 t;可开采地热资源总量为1.40×1017 J/年,折合标准煤为7.98× 106 t/年[85]。青海省地热资源类型主要分为隆起断裂型和沉降盆地型。隆起断裂型地热资源,大多分布在盆地周边山区地带,常以温泉形式沿断裂带排泄于地表,具有温度高、分布面积小的特点,主要分布在西宁盆地南缘药水滩地热区、贵德热水沟地热区、兴海县温泉地热区及唐古拉山口温泉地热区等。青海省沉降盆地型地热资源主要分布在青海东部西宁、贵德、共和等盆地内,该地区地热资源丰富,开发利用价值较高,同时勘查研究程度较高。青海省地热资源的热源特征与底部岩浆活动历史和特征密切相关,由于我国青藏高原地区具有强烈的构造运动,新生代以来,受欧亚板块和印度洋板块的挤压,青藏高原逐渐隆升,局部有岩浆入侵的存在,故产生沉积盆地型干热岩资源和隆起断裂型地热资源[91]。根据青海省地热资源广泛分布的现状,未来在政策措施引导下,能更好地推动地热资源开发利用,为中国地热发电战略部署做出贡献。
3.2 清洁能源价值
2000—2018年青海省5种清洁能源价值汇总如表12所示。Table 12
表12
表122000—2018年青海省清洁能源价值/亿元
Table 12
年份 | 水电势能 | 太阳能 | 风能 | 生物质能 | 地热能 | 总价值 | |||||||||
---|---|---|---|---|---|---|---|---|---|---|---|---|---|---|---|
发电 | 碳减排 | 发电 | 碳减排 | 发电 | 碳减排 | 发电 | 碳减排 | 发电 | 碳减排 | ||||||
2000 | 685.26 | 45.59 | 184.00 | 4.48 | 16.33 | 0.71 | 411.97 | 29.63 | 221.37 | 4.67 | 1604.00 | ||||
2001 | 657.25 | 43.71 | 182.46 | 4.44 | 17.00 | 0.74 | 481.45 | 34.63 | 221.37 | 4.67 | 1647.72 | ||||
2002 | 706.91 | 47.02 | 180.55 | 4.39 | 16.80 | 1.07 | 489.18 | 35.18 | 221.37 | 4.67 | 1707.14 | ||||
2003 | 739.99 | 49.22 | 180.74 | 4.40 | 20.92 | 0.91 | 474.54 | 34.13 | 221.37 | 4.67 | 1730.89 | ||||
2004 | 722.07 | 48.03 | 183.67 | 4.47 | 20.30 | 0.89 | 515.58 | 37.08 | 221.37 | 4.67 | 1758.11 | ||||
2005 | 838.46 | 55.78 | 179.41 | 4.36 | 17.43 | 0.76 | 523.73 | 37.67 | 221.37 | 4.67 | 1883.63 | ||||
2006 | 626.18 | 41.65 | 176.31 | 4.29 | 17.94 | 0.78 | 528.18 | 37.99 | 221.37 | 4.67 | 1659.36 | ||||
2007 | 831.34 | 55.30 | 181.30 | 4.41 | 17.12 | 0.75 | 550.10 | 39.56 | 221.37 | 4.67 | 1905.91 | ||||
2008 | 813.57 | 54.11 | 188.13 | 4.58 | 15.41 | 0.67 | 472.14 | 33.96 | 221.37 | 4.67 | 1808.61 | ||||
2009 | 887.52 | 59.03 | 185.39 | 4.51 | 15.95 | 0.70 | 564.75 | 40.62 | 221.37 | 4.67 | 1984.50 | ||||
2010 | 813.71 | 54.12 | 190.46 | 4.63 | 16.46 | 0.72 | 571.71 | 41.12 | 221.37 | 4.67 | 1918.97 | ||||
2011 | 844.55 | 56.17 | 193.72 | 4.71 | 16.56 | 0.72 | 582.79 | 41.91 | 221.37 | 4.67 | 1967.17 | ||||
2012 | 853.20 | 56.75 | 192.46 | 4.68 | 16.67 | 0.73 | 563.09 | 40.50 | 221.37 | 4.67 | 1954.12 | ||||
2013 | 749.83 | 49.88 | 198.47 | 4.83 | 15.26 | 0.67 | 555.61 | 39.96 | 221.37 | 4.67 | 1840.54 | ||||
2014 | 860.02 | 57.21 | 198.06 | 4.82 | 15.22 | 0.66 | 574.50 | 41.32 | 221.37 | 4.67 | 1977.85 | ||||
2015 | 653.91 | 43.49 | 190.47 | 4.63 | 15.90 | 0.69 | 601.78 | 43.28 | 221.37 | 4.67 | 1780.20 | ||||
2016 | 956.05 | 63.59 | 195.06 | 4.74 | 14.55 | 0.63 | 621.48 | 44.70 | 221.37 | 4.67 | 2126.85 | ||||
2017 | 1107.23 | 73.65 | 179.82 | 4.37 | 14.77 | 0.64 | 635.89 | 45.73 | 221.37 | 4.67 | 2288.15 | ||||
2018 | 1189.14 | 79.10 | 171.18 | 4.16 | 14.77 | 0.64 | 595.66 | 42.84 | 221.37 | 4.67 | 2323.53 |
新窗口打开|下载CSV
清洁能源潜力总价值由本身价值和其具有的碳减排价值两部分组成,本文对5种清洁能源的本身价值及碳减排价值进行整理分析,得出以下结果:
(1)从总价值的变化趋势来看,2000—2018年,清洁能源价值呈增加趋势(n=19,R2=0.69),平均增量为29.61亿元/年。其中,水电势能、太阳能、生物质能价值呈增加趋势,平均增量分别为19.47亿元/年、0.44亿元/年、9.91亿元/年,风能呈减少趋势,平均减量为0.21亿元/年。清洁能源的价值与国际碳交易市场的碳交易定价关系密切,国际碳市场发展迅速且价格波动较大。中国处在碳交易产业链的最低端,只是作为市场的参加者和规则的执行者进行碳市场定价[92]。因此未来清洁能源潜力的价值变化取决于未来中国的碳交易市场发展情况,完善碳交易市场体系,加强对清洁能源发展机制(Clean Development Mechanism,CDM)项目开发的研究、制定统一交易标准等,对中国碳交易市场的完善具有重要意义。
(2) 从能源结构来看,2000—2018年水电势能平均价值占清洁能源总价值的比重最大(46.20%),其次是生物质能(30.82%)、地热能(11.97%)、太阳能(10.09%)和风能(0.92%);从能源价值构成来看,清洁能源发电价值占总价值的95%,碳减排价值占总价值的5%。根据《青海清洁能源发展白皮书2019》[93],截至2018年底,青海省清洁能源装机规模已达2420万kW,占全省电源总装机的86.5%,其中,水力发电占青海省电源结构的42.6%,太阳能发电占34.4%,风力发电占9.5%。由本文对清洁能源的时空分布评估结果可以看出青海省的清洁能源具有巨大开发潜力,增加清洁能源在能源消费中的比重,能够获得更高的发电价值及碳减排价值。
(3)根据青海省发布的《青海能源发展报告(2018)》[94],2018年清洁能源的实际利用量分别为:水力发电量为517亿kW·h,同比增长56.6%;风力发电量为37.6亿kW·h ,同比增长108.9%,弃风率1.6%;太阳能发电量为131.1亿kW·h,同比增长15.6%,弃光率4.75%。根据本文的研究结果,青海省水电、太阳能发电、风力发电的实际应用约占其总能源潜力的26%,且生物质能与地热能有待继续开发,因此,青海省未来的清洁能源开发应用潜力巨大。
4 结论与讨论
4.1 结论
本文基于模型模拟和空间分析等方法,对青海省水电势能、太阳能、风能、生物质能、地热能5种主要清洁能源的发电潜力及价值进行评估,得到了青海省主要清洁能源发电潜力的时空分布格局和数量统计特征。主要结论有:(1)青海省清洁能源蕴藏量丰富,开发利用潜力巨大,具有时间和空间变化特征。2000—2018年青海省清洁能源潜力整体呈逐年增加的趋势,其中水电势能、太阳能、生物质能呈增加趋势,风能呈减少趋势;在空间上,青海省西部清洁能源潜力相对较高,东部较低,西部具有丰富的太阳能和风能,东部具有丰富的水电势能。能源总量和单位面积能源产出效率排序并不完全一致,因此在能源开发时应综合考虑不同地区的生态环境现状、能源产出效率、能源蕴藏总量等因素,可以把长期处于清洁能源潜力的高值区作为优先开发区,如在西部地区开发太阳能与风能,在东部地区开发水电势能等。
(2)从清洁能源结构来看,青海省水电资源潜力最为丰富,其次是生物质能、地热能、太阳能和风能。随着科学技术的进步,青海省水电站、光伏电站、风电站的装机量都在逐年增加,生物质能源的使用量也在不断提高,但是地热能利用仍然处于初级阶段,开发利用量较少。针对清洁能源利用现状,应充分考虑清洁能源的蕴藏量和开发条件,在合理保护生态环境的前提下,在适宜地区进一步建设水力发电站、太阳能发电站、风力发电站,提高生物质资源的利用效率,对可用于发电的地热资源进行勘探和开发。
(3)从清洁能源价值来看,2000—2018年清洁能源价值呈增加趋势,水电势能平均价值占清洁能源总价值的比重最大,其次是生物质能、地热能、太阳能和风能。根据相关统计数据与本文研究结果比较后发现,全省清洁能源实际应用程度与清洁电力应用潜力尚有较大差距,未来青海省清洁能源利用水平存在较大提升空间。在未来清洁能源开发利用过程中应结合能源潜力总量、不同地区的能源产出效率与能源需求,建立与清洁能源生产相适应的产业体系、消费体系,加大推广清洁能源开发利用的力度。
4.2 讨论
本文评估的对象为青海省清洁能源发电潜力及潜在价值,因此对评估结果和开发利用清洁能源的意义展开讨论:(1)本文考虑了青海省气候、植被、水文、地形地貌、土壤等自然地理要素时空分异特征,通过对2000—2018年青海省清洁能源的量化评估,进一步证实青海省的最大价值在生态这一科学判断。此外,水电势能、太阳能、风能发电潜力影响因素众多,由于气候、植被等因素的变化使其时空分布具有动态变化特征和一定的不确定性,这需要进一步的长期跟踪研究。
(2)本文根据模型模拟和数据统计结果核算了2000—2018年青海省清洁能源发电潜力及碳减排潜力。清洁能源潜力价值依据平均上网电价进行核算,碳减排潜力价值依据中国试点碳市场年度成交均价进行核算,结果具有可靠性,为同类研究提供了方法上的参考。由本文核算过程可知,清洁能源的价值与中国的上网电价和国际碳交易市场的碳交易定价关系密切,因此在未来发展中,确定合理的国内上网电价、制定统一的中国碳交易标准等措施对开发清洁能源、提升宏观经济、改善民生福祉具有积极影响。
(3)根据本文核算结果,青海省水力发电、太阳能发电、风力发电的实际应用约占其总能源潜力的26%,且生物质能与地热能有待继续开发,印证了青海省清洁能源具有巨大的开发潜力。因此,充分利用水能、风能、太阳能、生物质能、地热等清洁能源,可以逐步改善并优化青海省乃至全国的能源消费结构;制定具有吸引力的清洁能源开发利用政策,吸收各种资本,增加清洁能源开发与利用方面的投入,对于确保中国的能源安全,治理环境污染问题,建设“美丽中国”和落实生态文明建设都具有十分重要的战略意义。
参考文献 原文顺序
文献年度倒序
文中引用次数倒序
被引期刊影响因子
[R/OL]. (
URL [本文引用: 1]
[R/OL]. (
URL [本文引用: 1]
[本文引用: 1]
[J].
[本文引用: 1]
[EB/OL]. (
URL [本文引用: 1]
[EB/OL]. (
URL [本文引用: 1]
[J].
[本文引用: 1]
[J].
[本文引用: 1]
[J].
[本文引用: 1]
[J].
DOI:10.1007/s11269-015-0975-1URL [本文引用: 1]
[J].
DOI:10.3178/hrl.11.6URL [本文引用: 1]
[J].
DOI:10.1080/00908310490451385URL [本文引用: 1]
[J].
[本文引用: 1]
[J].
DOI:10.1007/s40808-015-0041-2URLPMID:26870835 [本文引用: 1]

0.97). This makes the model suitable for the application on sites, where the information on soil temperature is discontinuous or scarce.]]>
[J].
[本文引用: 1]
[J].
DOI:10.1016/j.energy.2010.03.025URL [本文引用: 1]

AbstractThe objects of the article are to determine the profits for solar energy integrating remote sensing data: the optimal locations of photovoltaic and the base price of electricity resulting from solar energy. An illustrated experiment with five European countries data sets is taken. Results indicate that Germany is the only optimal region to set up photovoltaic so as to satisfy the electricity demand of the five considered. Results also show that solar energy is a promising energy source since the highest base price of electricity resulting from solar energy is only 0.35 $/kWh. The base electricity price for Germany is the lowest whereas the base electricity price for Italy is the highest. Moreover, the results further indicate that the photovoltaic module price plays a key role in determining the best appropriate region(s) to install photovoltaic and the base electricity price.]]>
[J].
[本文引用: 1]
[J].
[本文引用: 1]
[J].
DOI:10.1080/15435070902784947URL [本文引用: 1]
[J].
[本文引用: 1]
[J].
DOI:10.1016/S0960-1481(00)00169-5URL [本文引用: 1]
[J].
DOI:10.1016/j.renene.2017.02.008URL [本文引用: 1]
[J].
DOI:10.1016/S0961-9534(00)00004-0URL [本文引用: 1]
[J].
DOI:10.1016/j.biombioe.2013.05.004URL [本文引用: 1]
[J].
[本文引用: 1]
[J].
DOI:10.1016/j.renene.2014.01.044URL [本文引用: 1]
[J].
[本文引用: 1]
[J].
[本文引用: 1]
[J].
[本文引用: 1]
[J].
[本文引用: 1]
[J].
[本文引用: 1]
[J].
[本文引用: 1]
[J].
DOI:10.11820/dlkxjz.2010.09.004URL [本文引用: 1]

从资源丰富度、稳定度和保障度3个方面,分别选取了较有代表性的太阳总辐射、日照时数和有效日照天数3项量化因子,利用多指标评分法对中国陆地太阳能资源开发潜力进行了综合评价和分析。结果表明:①中国青藏高原大部、日一肃北部、新疆东部和内蒙中西部地区,太阳能资源极为丰富,最适于进行大规模光电开发;②日一肃中部、新疆、青海东部和南部、内蒙古中东部、东北西部、河北与宁夏北部、四川西部,太阳能资源比较丰富,也适于规模化的太阳能发电;③东北东部和北部、华北平原北部、黄上高原大部、青藏高原东南缘、云南大部、雷州半岛和海南岛,以及新疆北部,太阳能可小规模或季节性开发利用;④其余地区太阳能资源比较贫乏,规模性开发潜力低。
[J].
DOI:10.11820/dlkxjz.2010.09.004URL [本文引用: 1]

With rapid population growing and dramatic industrial economy developing, the world faces the dual challenges of massive energy requirements and environmental issuescaused by extensive fossil fuel depletion. One of the main candidates for faxing these challenges are renewable sources of energy (including solar, wind, biomass, geothermal, hydro,wave and tidal energies). Among these sources, solar energy comes at the top of the list due to its abundance, and more even distribution in nature than any other renewable energy types. However, analyses of China's solar energy potential so far have generally relied onclimatological empirical formula which is hard to spread and undeveloped drawing technique. Therefore, based on general solar radiation observations taken from 99 China's radiation stations and sunshine duration observations taken from 387 of China's 752 meteorological stations, this textcpunted average annual solar total radiation as the factor of abundance, sunshine duration as the factor of stabilization and available sunshine days(≥3 hours) as the factor of guarantee from the years 1995-2004. Using ArcGIS cartography software and the aforementioned data, the following text depicted a series of maps of mainland China's solar energy distribution. Then these maps were overlaid to present a more accurate picture of mainland China's solar energy potential. An analysis of these maps leads to the following conclusions. The greatest solar energy potential is in the Qingzang Plateau, Northern Gansu, Eastern Xinjiang and Mid-Western Inner Mongolia, where are the best locations for extensive solar energy utilization; solar energy potential in Xinjiang, Eastern Inner Mongolia, western part of Northeast Plain, Northern Hebei and Ningxia, Southeast Qinghai and Western Sichuan, is the second best, also perfect for solar energy development. In the areas of the northeast part of Northeast Plain, most parts of Loess Plateau, Yunnan and Hainan, Northern Huabei Plain, and Northern Xinjiang, solar energy potential is at average level. The other areas of mainland China have small solar energy potentials due to the lack of solar radiation.
[J].
URL [本文引用: 1]

本文利用非参数统计检验方法(MannKendall法)分析了黄河流域77个气象站1958年~2001年日照时数序列的长期变化趋势,并用参数检验法对其结果进行了相互验证。结果表明,黄河流域1958年~2001年的年、典型月日照时数序列均大致存在一定的下降趋势,年序列所选77个站中有53个表现出下降趋势,只有24个表现出一定的上升趋势;在有意义(拒绝零假设)的34个站点中,28个站点表现出下降趋势,6个显示出上升趋势。为了进一步阐明日照时数长期变化趋势的时空分布特点,根据研究区的气候特点,将黄河流域分为5个子区域。结果表明子区域Ⅰ,Ⅱ和Ⅲ的日照时间均表现出了明显的下降趋势,但这一趋势在子区域Ⅳ和Ⅴ表现并不明显。这一结果说明在过去的40年到50年期间,黄河流域的日照时间总体上呈下降趋势,这一趋势在黄河流域中下游地区尤其明显。在此基础上,选择1月、4月、7月和10月作为一年四季的代表时段,对日照时数的时间分布特点进行了分析。其结果表明,对年日照时数下降趋势贡献最大的月份是1月、6月、10月和12月;日照时数的长期变化趋势既存在一定的时间差异,也存在着一定的地区差异。
[J].
URL [本文引用: 1]

The MannKendall statistic test is a rankbased nonparametric method, which has been widely used to detect trends in hydrometeorological time series such as streamflow, temperature, precipitation and sunlight radiation hours, etc. Sunlight radiation duration is one of the portant climatic factors. The possible trend of annual and monthly sunlight radiation duration in the Yellow River basin was detected by using the annKendall test in this paper. The data used in this study were observed at 77 meteorological stations in the Yellow River basin. The MannKendall test was applied to annual and monthly time series from 1958 to 2001. At the same time, a parametric test was also applied to make the trend analysis. The results shown that there is an obvious downward trend in the annual and monthly sunlight radiation durations in the subregion of Ⅰ,Ⅱ, and Ⅲ, but subregion of Ⅳ and Ⅴ did not exhibit significant longterm trend. The results from 53 stations shown a decreasing trend and only 24 stations exhibited an increasing trend. 34 stations rejected the hypothesis, among which 28 stations shown significant decreasing trend, and only 6 stations shown significant increasing trend. In other words, most area of the middle and downstream of the Yellow River basin exhibited downward trend of sunlight radiation duration, but the upstream did not show obvious longterm trend. This demonstrated that decrea sing trend dominated the longterm trend of the sunlight radiation duration in the study area during the past fifty years. On the basis of above analysis, January, April, July, and October were selected as typical months to represent four seasons of the year, and the corresponding longterm trend for these representative months were further investigated. The results shown that in January, June, October and December there is a greater longterm trend and a greater contribution made by annual sunlight radiation duration. The temporal and spatial distribution of the longterm trend for the sunlight radiation duration in the study area shows that there is a difference for the trend of sunlight radiation duration over different area during different periods in the Yellow River basin from 1958 to 2001.
[J].
[本文引用: 1]
[J].
[本文引用: 1]
[J].
[本文引用: 1]
[J].
[本文引用: 1]
[J].
[本文引用: 1]
[J].
[本文引用: 1]
[J].
[本文引用: 1]
[J].
[本文引用: 1]
[J].
[本文引用: 1]
[J].
[本文引用: 1]
[J].
[本文引用: 1]
[J].
[本文引用: 1]
[J].
[本文引用: 1]
[J].
[本文引用: 1]
[J].
URL [本文引用: 1]

本文从风能资源的丰富度、稳定度、人文环境对风能资源开发适宜度和自然环境适宜度等四个方面,分别选取了较有代表性的有效风能密度、风能可用时数、人口密度、森林郁闭度四项量化指标,利用综合指数法对我国陆地10m高度风能资源开发潜力进行了综合评价和分析。结果表明:内蒙古中部和西部、河西走廊、青藏高原中北部、东北平原南部、山东和辽东半岛,以及江苏、浙江、福建和海南的沿海地带,风力资源优越,环境因子限制小,是风能规模开发潜力最高的地区;内蒙古锡林郭勒高原、呼伦贝尔高原、新疆东部和西北克拉玛依地区、东北平原、河北北部和东部沿海(除了最高潜力地区)为次高潜力区;大兴安岭、山东西北部、河南北部、江苏大部、安徽中部、新疆北部和东南部、西藏中西部和云南东部属中级开发潜力区;其它地区因风能资源贫乏或环境因子限制大,风能开发潜力较低。
[J].
URL [本文引用: 1]

With rapid population growing and industrial economy development, the world facesdual challenges of massive energy requirement and environmental issues caused by extensive fossilfuel depletion. One of possible measures for addressing such issues is exploitation and utilizationof renewable sources of energy, e.g., solar, wind, biomass, geothermal, combustible ice and oceantidal energy. Compared to other renewable energy, development of wind power has become apriority all over the globe today due to its mature technology, relatively low cost and fewenvironmental problems. However, analyses of China’s wind power potential have rarelyconsidered social or physical factors limiting actual wind utilization. Based on wind speedobservations (10 meters above the surface) taken from 387 of China's 752 meteorological stations,and using the American Renewable Resources Laboratory wind energy evaluation methods, theauthors analyzed in detail meteorological observations of mean annual wind power density (as thefactor of abundance) and mean available time of wind utilization (as the factor of stabilization)from 1995-2004. In the meanwhile, we took the population density and forest canopy density as thesocial and physical limiting environmental factors of wind power potential. Using ArcGIStechniques and the aforementioned data, the authors made a series of maps of wind power density,available time of wind utilization, population density and forest canopy density distribution inmainland China. Furthermore, overlapping analysis was performed to derive more reasonablespatial representation of wind power potential over mainland China. It was concluded that thegreatest potential is in roughly the mid-western Inner Mongolia, Hexi Corridor, northwesternQinghai, Shandong and Liaodong Peninsula, foreland of Fujian, Zhejiang, Jiangsu and Hainan, andpart of the Northeast Plain, which have great wind power with few limitations. The Xilinguole andHulunbeier Plateau in Inner Mongolia, Northeast Plain, eastern and northwest Xinjiang show thesecond largest wind power potential. Areas with great natural wind power but whose wind potentialis significantly constrained by large population densities are along the Pacific coast (except thegreatest potential areas) and northwestern Shandong. The range of Daxingan and Xiaoxingan innortheastern China are limited most by forested land. The wind power potential in northern Henan,most part of Jiangsu, center Anhui, northern and southeast Xinjiang, western Tibet, and easternYunnan either limited by poor wind resources or environmental factors is at an average level. Theother areas of mainland China have small potential to exploit wind power energy.
[J].
[本文引用: 1]
[J].
[本文引用: 1]
[J].
[本文引用: 1]
[J].
[本文引用: 1]
[J].
[本文引用: 1]
[J].
[本文引用: 1]
[J].
[本文引用: 1]
[J].
[本文引用: 1]
[J].
[本文引用: 1]
[J].
[本文引用: 1]
[J].
[本文引用: 1]
[J].
[本文引用: 1]
[J].
[本文引用: 1]
[J].
[本文引用: 1]
[J].
[本文引用: 1]
[J].
[本文引用: 1]
[J].
[本文引用: 1]
[J].
[本文引用: 1]
[J].
[本文引用: 1]
[J].
[本文引用: 1]
[J].
[本文引用: 1]
[J].
DOI:10.1108/IJCCSM-03-2018-0024URLPMID:33408756 [本文引用: 1]

Purpose: Productivity and production risks affect the use of agricultural production practices and inputs, particularly in developing countries. This paper aims to investigate the effects of adopting drought-tolerant maize varieties (DTMVs) on farm productivity, yield variance and downside risk exposure of maize growing households of Zambia. Design/methodology/approach: The study uses household survey data collected from 11 maize producing districts of Eastern, Southern and Copperbelt provinces of Zambia using a structured questionnaire. The Antle's flexible moment-based approach was used in specifying, estimating and testing a stochastic production function. The study further applied an endogenous switching regression model to control for both observable and unobservable sources of bias. Findings: The study revealed that DTMV adoption increases maize yield by 15 per cent and reduces the risk of crop failure: reducing yield variance by 38 per cent and exposure to downside risk by 36 per cent. Originality/value: This study establishes the benefits of DTMV adoption in Zambia with regards to productivity, yield stability and downside risk in the face of climate change. Results from this study underscore the need for more concerted efforts to scale-out DTMVs for both maize productivity enhancement and for risk mitigation against weather shocks.
[J].
DOI:10.1016/j.jclepro.2017.05.123URL [本文引用: 2]
[J].
DOI:10.1016/j.renene.2005.08.034URLPMID:32288364 [本文引用: 1]

Water is in short supply with countries vying for access to river heads. Hong Kong (HK) is no different. It relies on supply from Mainland China with other cities rivalling access. Yet there is still no great impetus for water conservation. This paper reports on progress in setting up a pilot biological wastewater treatment plant and a PV-powered UV-LED disinfection system plus a feasibility study carried out in conjunction with a local developer investigating the application of the treated water for irrigation. The objective is to incorporate the systems within a small-scale community for its contribution to water and energy conservation as well as establish the parameters for replication in other countries with potential up-scaling for urban application.
[J].
DOI:10.1016/j.jclepro.2017.01.034URL [本文引用: 1]
[J].
DOI:10.1029/2019MS001689URLPMID:32999700 [本文引用: 1]

In the atmosphere, microphysics refers to the microscale processes that affect cloud and precipitation particles and is a key linkage among the various components of Earth's atmospheric water and energy cycles. The representation of microphysical processes in models continues to pose a major challenge leading to uncertainty in numerical weather forecasts and climate simulations. In this paper, the problem of treating microphysics in models is divided into two parts: (i) how to represent the population of cloud and precipitation particles, given the impossibility of simulating all particles individually within a cloud, and (ii) uncertainties in the microphysical process rates owing to fundamental gaps in knowledge of cloud physics. The recently developed Lagrangian particle-based method is advocated as a way to address several conceptual and practical challenges of representing particle populations using traditional bulk and bin microphysics parameterization schemes. For addressing critical gaps in cloud physics knowledge, sustained investment for observational advances from laboratory experiments, new probe development, and next-generation instruments in space is needed. Greater emphasis on laboratory work, which has apparently declined over the past several decades relative to other areas of cloud physics research, is argued to be an essential ingredient for improving process-level understanding. More systematic use of natural cloud and precipitation observations to constrain microphysics schemes is also advocated. Because it is generally difficult to quantify individual microphysical process rates from these observations directly, this presents an inverse problem that can be viewed from the standpoint of Bayesian statistics. Following this idea, a probabilistic framework is proposed that combines elements from statistical and physical modeling. Besides providing rigorous constraint of schemes, there is an added benefit of quantifying uncertainty systematically. Finally, a broader hierarchical approach is proposed to accelerate improvements in microphysics schemes, leveraging the advances described in this paper related to process modeling (using Lagrangian particle-based schemes), laboratory experimentation, cloud and precipitation observations, and statistical methods.
[本文引用: 1]
[本文引用: 1]
[DB/OL]. (
URL [本文引用: 1]
[DB/OL]. (
URL [本文引用: 1]
[J].
DOI:10.1007/s00376-014-3190-8URL [本文引用: 1]

The proposed approach is applied to observations collected at approximately 700 stations over mainland China. The generated forcing fields are compared with the corresponding components of the National Centers for Environmental Prediction (NCEP) Climate Forecast System Reanalysis dataset and the Princeton meteorological forcing dataset. The comparison shows that, both within the station network and within the resolutions of the two gridded datasets, the interpolation errors of the proposed approach are markedly smaller than the two gridded datasets.]]>
[J].
DOI:10.1016/j.jhydrol.2013.11.022URL [本文引用: 2]
[DB/OL]. (2018-12) [
URL [本文引用: 1]
[DB/OL]. (2018-12) [
URL [本文引用: 1]
[DB/OL]. (
URL [本文引用: 1]
[DB/OL]. (
URL [本文引用: 1]
[J].
[本文引用: 1]
[J].
[本文引用: 1]
[DB/OL]. (
URL [本文引用: 1]
[DB/OL]. (
URL [本文引用: 1]
[DB/OL]. (
URL [本文引用: 1]
[DB/OL]. (
URL [本文引用: 1]
[J].
DOI:10.1175/JHM-D-14-0079.1URL [本文引用: 1]
[EB/OL]. (
URL [本文引用: 1]
[EB/OL]. (
URL [本文引用: 1]
[EB/OL]. (
URL [本文引用: 1]
[EB/OL]. (
URL [本文引用: 1]
[J].
DOI:10.1115/1.3266201URL [本文引用: 1]
[J].
[本文引用: 1]
[J].
[本文引用: 1]
[J].
[本文引用: 1]
[J].
[本文引用: 1]
[J].
[本文引用: 1]
[J].
[本文引用: 1]
[J].
[本文引用: 1]
[J].
[本文引用: 1]
[J].
DOI:10.11820/dlkxjz.2010.09.004URL [本文引用: 1]

从资源丰富度、稳定度和保障度3个方面,分别选取了较有代表性的太阳总辐射、日照时数和有效日照天数3项量化因子,利用多指标评分法对中国陆地太阳能资源开发潜力进行了综合评价和分析。结果表明:①中国青藏高原大部、日一肃北部、新疆东部和内蒙中西部地区,太阳能资源极为丰富,最适于进行大规模光电开发;②日一肃中部、新疆、青海东部和南部、内蒙古中东部、东北西部、河北与宁夏北部、四川西部,太阳能资源比较丰富,也适于规模化的太阳能发电;③东北东部和北部、华北平原北部、黄上高原大部、青藏高原东南缘、云南大部、雷州半岛和海南岛,以及新疆北部,太阳能可小规模或季节性开发利用;④其余地区太阳能资源比较贫乏,规模性开发潜力低。
[J].
DOI:10.11820/dlkxjz.2010.09.004URL [本文引用: 1]

With rapid population growing and dramatic industrial economy developing, the world faces the dual challenges of massive energy requirements and environmental issuescaused by extensive fossil fuel depletion. One of the main candidates for faxing these challenges are renewable sources of energy (including solar, wind, biomass, geothermal, hydro,wave and tidal energies). Among these sources, solar energy comes at the top of the list due to its abundance, and more even distribution in nature than any other renewable energy types. However, analyses of China's solar energy potential so far have generally relied onclimatological empirical formula which is hard to spread and undeveloped drawing technique. Therefore, based on general solar radiation observations taken from 99 China's radiation stations and sunshine duration observations taken from 387 of China's 752 meteorological stations, this textcpunted average annual solar total radiation as the factor of abundance, sunshine duration as the factor of stabilization and available sunshine days(≥3 hours) as the factor of guarantee from the years 1995-2004. Using ArcGIS cartography software and the aforementioned data, the following text depicted a series of maps of mainland China's solar energy distribution. Then these maps were overlaid to present a more accurate picture of mainland China's solar energy potential. An analysis of these maps leads to the following conclusions. The greatest solar energy potential is in the Qingzang Plateau, Northern Gansu, Eastern Xinjiang and Mid-Western Inner Mongolia, where are the best locations for extensive solar energy utilization; solar energy potential in Xinjiang, Eastern Inner Mongolia, western part of Northeast Plain, Northern Hebei and Ningxia, Southeast Qinghai and Western Sichuan, is the second best, also perfect for solar energy development. In the areas of the northeast part of Northeast Plain, most parts of Loess Plateau, Yunnan and Hainan, Northern Huabei Plain, and Northern Xinjiang, solar energy potential is at average level. The other areas of mainland China have small solar energy potentials due to the lack of solar radiation.
[J].
URL [本文引用: 1]

利用模拟20世纪近地层风速变化效果较好的3个全球气候模式和3个区域气候模式预估了21世纪中国区域近地层风功率密度变化。得到如下结论:①区域气候模式CMM5预估21世纪初期中国夏季平均风功率密度比20世纪小;②全球气候模式预估21世纪中期中国年和冬半年平均风功率密度略小于20世纪;夏半年风功率密度变化趋势难以分辨;③区域气候模式(RegCM3和PRECIS)和全球气候模式一致预估21世纪后期中国年和冬半年平均风功率密度比20世纪小,且可信度较大;对于夏半年,全球气候模式预估结论与区域气候模式预估结论不一致,不确定性较大。由于当前科学水平的限制,无论是全球气候模式还是区域气候模式,对区域尺度未来气候变化的预估都存在着较大的不确定性,尤其是在风速和风功率密度的预估方面,才刚刚开始,还需要做大量和长时间的研究试验,才能得到更可靠的结论。
[J].
URL [本文引用: 1]

th century, with the rapid growth of the world economy, energy demand has multiplied and energy industries have been in the process of massive development of wind resources. Wind energy, with the characteristics of being clean, renewable and abundant, can offer pollution-free electricity that could be widely utilized. Wind power projects in China, particularly the wind power generation network are recently experiencing a rapid development. It is expected that the wind power capacity will increase to over 100,000 MW installed capacity by 2030 or 2040, and become the third largest source of energy in the country after thermal power and hydropower. As China’s wind power industries are developing unprecedentedly, the issue on sustainable use of wind energy resources has been a major focus in the context of global and Asia warming. There have been a series of studies on future characteristics of the surface wind and wind resources over the ocean and some other countries but little work has been done in China so far. Therefore, there is an urgent need to investigate characteristics of future wind energy in China. In the present work, three global climate models and three regional climate models which have certain capabilities to simulate changes in wind speed during the 20th century, were used to project changes in wind power density in China for the 21th century. The following conclusions are given. First, the summer mean wind power density in China for the early 21th century decreases slightly, which was projected by the regional climate model CMM5. Second, changes in annual and winter mean wind power density in China for the mid-21th century were projected to be decreasing slightly than that for the 20th century. It was also found that trends in summer mean wind power density for the mid-21th century was difficult to be detected by the models. Third, regional climate models (RegCM3 and PRECIS) and global climate models generated similar results, showing that the annual and winter mean wind power density in China for the late 21th century would decrease slightly and the results were more reliable than that for summer. It is worthwhile to note that due to current limitations in projection capability, both the global climate models and the regional climate models would result in large uncertainties in projections of regional climate changes, particularly in the projection of wind power density. The study on variation trends in wind velocity for the 21st century in China provides meaningful information and reference for further studies.]]>
[J].
[本文引用: 1]
[J].
[本文引用: 1]
[J].
URL [本文引用: 1]

作物秸秆是农作物生产系统中一项重要的生物质资源。中国是世界上秸秆资源最为丰富的国家之一。论文详细论述了中国农作物秸秆的资源量和分布,介绍了其作为工业造纸原料、畜牧饲料、造肥还田以及农村生活能源的利用现状和秸秆综合利用新技术。
[J].
URL [本文引用: 1]

Straw is an important biological resource in agriculture, and China is one of the most abundant countries for straw production in the world. This paper introduces the output and distribution of straw in China and discusses its utilization as the raw material in industry, livestock forage in animal husbandry, fertilizer and energy on farms and households in rural areas. This paper also indicates that great progress was made in integrated utilization based on a number of new technologies, marketing, and support from the Chinese government.
[J].
URL [本文引用: 1]

该文从资源的角度,根据各作物秸秆的经济系数,对我国各类农作物秸秆作了全面的估算,分析了我国作物秸秆资源的发展趋势和分布特点。研究结果表明,目前我国秸秆资源产量达到79454.4×104t的水平,并每年以1251.2×104t的速度稳步增长;我国作物秸秆资源分布存在地域性特点,东部农区是我国作物秸秆资源的主要分布区,黑龙江、河北、山东、江苏和四川是作物秸秆资源的集中分布区。对我国作物秸秆资源利用现状分析认为,每年有95%以上作物秸秆资源通过不同的利用途径转化成其他的形式而被耗散,资源浪费严重。针对作物秸秆资源利用中存在的问题提出了一些政策建议。
[J].
URL [本文引用: 1]
[J].
[本文引用: 1]
[J].
URL [本文引用: 1]

畜禽养殖产生的粪便已成为我国农村面源污染的主要来源,因而畜禽粪便的排放量也越来越引起人们的重视。通过对重庆市畜禽养殖业进行调查,结合国内外有关研究,确定重庆市畜禽粪便年排放量估算方法和各种估算参数。以2001年为基准,估算出重庆市主要畜禽(猪、牛、羊、马、兔、家禽等)年粪尿排放量和粪尿中对环境产生污染的主要物质量(有机质、氮、磷、钾),以便对重庆市畜禽粪便污染作一个定量的认识。估算结果为重庆市2001年畜禽粪尿排放7421万t,其中有机质为806万t,氮42.5万t、磷21.48万t、钾44.98万t。该研究结果可为重庆市工农业生产布局和环境污染治理提供决策依据。
[J].
[本文引用: 1]
[J].
URL [本文引用: 1]

随着我国畜禽养殖业的迅速发展,畜禽粪便排放不断增加。2002年,我国畜禽粪便产生量达27.5亿吨,畜禽粪便农用地的平均负荷为4.19吨/公顷,畜禽粪便每年流失至水体的总氮、总磷、BOD、COD分别为87万吨、34.5万吨、600万吨和647万吨。聚类分析结果显示,如果将我国各省农用地受畜禽粪便污染的风险分为4级;上海受污染的风险等级最高,其次是河南、天津和山东,北京、江苏、河北、安徽和湖南再次之,其余22个省份农用地受污染的风险相对较小。加强畜禽粪便的管理和资源化利用是解决畜禽粪便污染的主要手段。
[J].
[本文引用: 1]
[J].
[本文引用: 1]
[J].
[本文引用: 1]
[J].
[本文引用: 1]
[本文引用: 2]
[本文引用: 1]
[本文引用: 1]
[J].
DOI:10.11849/zrzyxb.2007.01.002URL [本文引用: 2]

8t,主要分布在河南、山东、黑龙江、吉林、四川等省;畜粪39.26×108t,主要分布在河南、山东、四川、河北、湖南等省;林木生物质21.75×108t,主要分布在西藏、四川、云南、黑龙江、内蒙古等省区;城市垃圾1.55×108t,主要分布在广东、山东、黑龙江、湖北、江苏等省;废水482.4×108t,主要分布在广东、江苏、浙江、山东、河南等省;②2004年中国生物质能实物总蕴藏潜力为35.11×108tce,前五位依次为四川、云南、黑龙江、河南和内蒙古;其中理论可获得量为4.6×108tce,前五位为四川、黑龙江、云南、西藏和内蒙古。可获得量中秸秆、薪柴和畜粪所占比例分别达38.9%、36.0%和22.14%;③中国生物质能分布不均,省际差异较大。按农村人口计算,人均理论可获得生物质能最大的西藏自治区达14.17tce,最小的浙江省仅0.15tce。而生物质能蕴藏潜力分布在一定程度上与常规一次能源蕴藏潜力分布呈现互补状态,则更加突出了在一次能源蕴藏量较低的地区开发利用生物质能的巨大潜力。]]>
[J].
DOI:10.11849/zrzyxb.2007.01.002URL [本文引用: 2]

8t,distributed mainly in Henan,Shandong, Heilongjiang,Jilin and Sichuan provincesXdung39.26×108t,distributed mainly in Henan,Shandong, Sichuan,Hebei and Hunan provincesXforest and wood biomass 21.75×108t,distributed mainly in T ibet,Sichuan,Yunnan,Heilongjiang and Inner Mongolia provincesXcity rubbish1.55×108t,distributed mainly in Guangdong,Shandong,Heilongjiang,Hubei and Jiangsu provincesXwastewater482.4×108t, distributed mainly in Guangdong,Jiangsu,Zhejiang,Shandong and Henan provinces.(2)The potential quantity of all the biomass energy in China in 2004 is 35.11×108tce,and the acquirable quantity is 4.6×108tce with the top five provinces of Sichuan,Heilongjiang,Yunan,Tibet and Inner Mongolia.The respective proportion of crop residues,firwood and dung in the acquirable quantity is 38.9%,36.0% and 22.14%,respectively.(3)Biomass energy distribution varies from province to province in China.If computed by the rural population,the biomass energy density varies from the biggest 14.17tce per capita in Tibet Autonomous Region to the smallest 0.15tce per capita in Zhejiang province.And the relation between biomass energy proportion and primary energy proportion of each province in China shows great potential to explore biomass energy especially in the region where there is a shortage of primary energy.]]>
[EB/OL]. (
URL [本文引用: 2]
[EB/OL]. (
URL [本文引用: 2]
[EB/OL]. (
URL [本文引用: 1]
[EB/OL]. (
URL [本文引用: 1]
[本文引用: 1]
[EB/OL]. (
URL [本文引用: 1]
[C].
[本文引用: 1]
[C].
[本文引用: 1]
[J].
[本文引用: 1]
[J].
[本文引用: 1]
[J].
[本文引用: 1]
[J].
[本文引用: 1]
[J].
URL [本文引用: 1]

中国是世界第二大温室气体排放国,是全世界核证减排量(CERs)一级市场上最大供应国,但在碳交易过程中却处在整个碳交易产业链的最低端,只是碳交易市场的参加者,碳交易市场规则的执行者.中国为全球碳交易市场创造的巨大减排量被发达国家以低价购买后,包装、开发成价格更高的金融产品在国外进行交易.作为世界上最大的排放权供应国之一,中国没有一个像欧美那样的国际碳交易市场,不利于争夺碳交易的定价权.本文具体阐述了国际主要的碳交易市场的交易主体、交易类型、交易数量、交易额等发展状况,通过分析归纳出现阶段国际碳交易市场的特点,并以此为基础将国内碳交易市场和国际碳交易市场进行比较得出中国碳交易市场现阶段存在的缺陷,相应的提出了完善中国碳交易市场的启示,即完善碳交易市场的制度建设,完善碳交易体系,加强对CDM项目开发的研究,调动金融机构参与碳交易的主动性,统一国内碳排放标准.
[J].
[本文引用: 1]
[EB/OL]. (
URL [本文引用: 1]
[EB/OL]. (
URL [本文引用: 1]
[EB/OL]. (
URL [本文引用: 1]
[EB/OL]. (
URL [本文引用: 1]