
Pixel-Based and Object-Oriented Classification of Jujube and Cotton Based on High Resolution Satellite Imagery over Alear, Xinjiang
JI XuSheng1,2,3,4, LI Xu1,5, WAN ZeFu1,2,3,4, YAO Xia1,2,3,4, ZHU Yan1,2,3, CHENG Tao
通讯作者:
责任编辑: 杨鑫浩
收稿日期:2018-09-19接受日期:2019-01-17网络出版日期:2019-03-16
基金资助: |
Received:2018-09-19Accepted:2019-01-17Online:2019-03-16
作者简介 About authors
姬旭升,E-mail: 2016101011@njau.edu.cn。

摘要
关键词:
Abstract
Keywords:
PDF (2161KB)元数据多维度评价相关文章导出EndNote|Ris|Bibtex收藏本文
本文引用格式
姬旭升, 李旭, 万泽福, 姚霞, 朱艳, 程涛. 基于高空间分辨率卫星影像的新疆阿拉尔市棉花与枣树分类[J]. 中国农业科学, 2019, 52(6): 997-1008 doi:10.3864/j.issn.0578-1752.2019.06.004
JI XuSheng, LI Xu, WAN ZeFu, YAO Xia, ZHU Yan, CHENG Tao.
0 引言
【研究意义】新疆是我国重要的农产品种植区,棉花和红枣在当地农业种植结构中占据主体地位,据统计2015年新疆棉花和红枣的种植面积分别达到2273 110和495 548 hm2,占农作物和水果总种植面积的37.11%和50.99%,均位列第一位[1]。准确、快速地提取棉花和枣树的种植面积对了解当地农作物种植结构,调整当地农业经济增长模式,实现农作物的精确管理具有重要意义。传统的农作物种植面积获取往往是通过实地调研和逐级上报的方式来完成,然而该方法费时费力,已不能满足现代农业的生产需要,所以寻求一种快速、准确的棉花及红枣面积提取方法已经刻不容缓。【前人研究进展】卫星遥感作为新型的地物探测技术可对地物信息进行大范围、实时地获取,现已在作物种植面积调查中起到了重要作用[2,3,4]。目前农作物种植区遥感识别主要通过监督分类的方式来实现,如最大似然(Maximum Likelihood)[5]、光谱角制图(Spectral Angle Mapper)[6]、最邻近采样(Nearest Neighbors)[7]、穗帽变换(Tasseled Cap Transformation)[8]、决策树算法(Decision Tree Algorithm)[9]、随机森林(Random Forest)[10]、支持向量机(Support Vector Machine)[11]、神经网络(Neural Network)[12]、仿生算法(Biologically Inspired Algorithm)[13]等监督分类算法先后被应用于作物种植面积调查。随着信息提取技术的快速发展,时间序列克里格(Time-series Kriging)[14]、马可夫逻辑模型(Markov Logic Models)[15]、动态时间规整(Dynamic Time Warping)[16]等不依赖实地采样的作物分类算法开始出现,随着MARIANA等[17]利用Sentinel-2影像和动态时间权重分析(time-weighted dynamic time warping analysis),对作物种植区进行识别(总体分类精度高达92%)等一系列相关研究的开展,此类算法开始日趋成熟。但是,由于全自动作物分类算法对卫星数据的时空分辨率要求极高,目前的农作物面积遥感调查方法仍以监督分类算法为主。受卫星传感器性能的限制,长期以来农作物识别的相关研究均以中、低分辨率卫星影像为基础,以中、低分辨率卫星影像为数据源,以农作物制图和面积提取为目的的研究已经在各个地区广泛开展[18,19],对遥感技术在农业领域的广泛应用起到了巨大的推动作用。随着空间探测技术的发展,光学传感器性能不断改善,在过去的数十年间QuickBird、IKONOS、WorldView-2/WorldView-3等多颗高空间分辨率卫星已经先后投入商业运营,遥感影像开始步入米级、亚米级时代。在相关技术发展和现实需求的双重推动下,高分遥感影像已被广泛应用于农作物生产管理、农作物面积提取等诸多领域,并取得了良好的应用效果[20,21],农作物面积提取开始进入“精细化”时代。如刘克宝等[22]以RapidEye 遥感影像为数据源,使用最大似然分类算法对肇东市农作物种植面积进行获取,作物种植面积识别精度达到97%。在相当长的一段内,参与作物分类的特征通常保持在反射率水平[23];随着高光谱卫星影像的出现,相关****开始开展以主成分分析为基础的农作物提取,推动作物识别研究的发展[24];近年来随着植物指数时间序列物候学信息的应用,基于卫星影像的农作物识别研究内涵更加丰富[25]。与中分或低分遥感影像相比,高分影像包含更加丰富的空间信息。根据高分影像的这种特性我们可以提取更加完善的地物信息,但是随着相关****对其分析的逐渐深入,高分影像中同类地物内部的光谱差异增大(“同物异谱”和“异物同谱”现象)等新问题逐渐显现出来,并成为制约提高高分影像农作物面积提取精度的主要因素之一[26]。因此,探索更加精细的信息提取方法成为高分辨遥感影像处理中亟待解决的重要问题。许多研究已经表明,面向对象的信息提取技术方法比传统的以像元为基础的方法更适合高分辨率影像的处理和分析[27,28]。LI等[29]将高分影像的空间信息与中分影像的光谱信息相结合,获得了更加精细的作物分布图,并使分类精度从原来的93.83%提高到94.67%。面向对象的分类方法可以综合利用光谱、纹理及空间信息[30],为基于高分影像的农作物面积提取提供了新的解决途径,对农作物面积提取精度的进一步提高具有重要的意义。我国大部分地区地块面积普遍较小,且不同地块种植作物类型多样化。新疆地区地块形状和大小多样性丰富,用Landsat等中分辨率影像精细识别某些枣树及棉花地块存在困难。高分影像的爆炸性增长为新疆枣树及棉花分类的相关研究及应用提供了新的发展机遇,精确识别棉花及枣树的分布及种植面积对农作物的精确管理具有重要意义。【本研究切入点】现有作物识别及面积提取的相关研究多集中于对中分辨率时序影像的综合利用,单个时期或关键生育期高分辨率遥感影像相互比较的研究少有涉及,且面向对象作物分类方法在高分影像作物分类和识别中的优势还未加以明确,仍需进一步的研究和探索。本文采用基于像素与面向对象的信息提取手段对研究区2016-5-10(SPOT-6)、2016-09-07(Pleiades-1)、2016-10-08(WorldView-3)等3个特定时期的高分遥感影像进行分类,探究影像获取时期对作物分类精度的影响,最终建立起针对高空间分辨率遥感影像的主要农作物分类技术体系。【拟解决的关键问题】比较不同时期高分辨率影像的棉花、枣树分类精度,探明高分影像获取时间对该研究区主要农作物分类精度的影响;比较基于像素和面向对象信息提取及不同分类算法方法在高分影像作物识别中的差异,明确面向对象作物分类方法在高分影像作物识别中优势,找到合适的棉花、枣树分类方法。1 材料与方法
1.1 试验区概况
该研究区地处天山南麓,塔克拉玛干沙漠北部边缘,紧靠塔里木河,属塔里木河冲积细土平原;地理位置为81°18′0″—81°22′30″E,40°39′30″—40°42′30″N(图1)。该地区属暖温带极端大陆性干旱荒漠气候,雨量稀少,年均日照时间长,地表蒸发强烈,年均降水量为40.1—82.5 mm,年均蒸发量1 876.6— 2 558.9 mm;年均日照2 556.3—2 991.8 h,年均太阳辐射133.7—146.3 kcal/cm2,日照率为58.69%,非常适合光学卫星遥感影像获取。图1
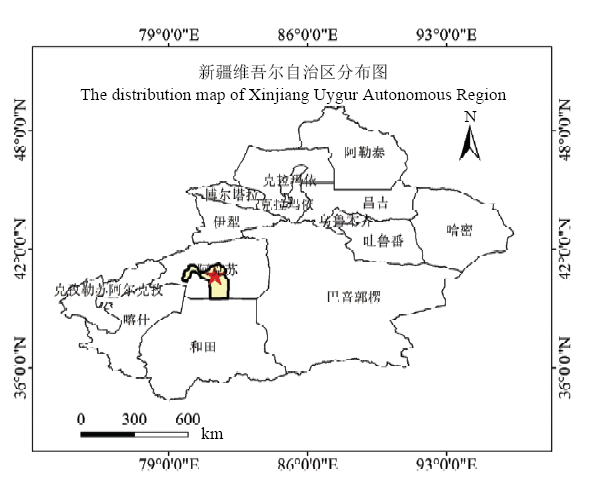
图1研究区位置图
Fig. 1Location of the study area
棉花和枣树为该研究区的两种主要作物,种植面积所占比达95%以上,塔里木河和胜利水库为该地区作物灌溉的主要水系。研究区为新疆建设兵团第十师十团农场(阿拉尔农场)的代表性区域,该农场土壤肥沃、土地平坦、耕地连片、田块整齐、灌溉体系完善,可以很好反映新疆阿拉尔地区的作物种植模式,具有重要的研究意义。
1.2 数据
1.2.1 遥感影像获取及预处理 根据本研究的目的,我们获取到2016年高空间分辨率卫星影像,分别对应枣树的展叶期(2016-05-10)、果实膨大期(2016-09-07)、果实成熟期(2016-10-08),以及棉花的苗期(2016-05-10)、成熟吐絮前期(2016-09-07)、成熟吐絮后期(2016-10-08),其具体参数如表1所示。Table 1
表1
表1获取的卫星影像
Table 1
卫星 Satellite | 波段类型 Band type | 空间分辨率 Spatial resolution (m) | 波段 Band | 获取时间 Date of image acquisition |
---|---|---|---|---|
SPOT-6 | 全色Panchromatic | 1.5 | 全色Panchromatic | 2016-05-10 |
多光谱Multispectral | 6 | 蓝Blue | ||
绿Green | ||||
红Red | ||||
近红外Near-infrared | ||||
Pleiades-1 | 全色Panchromatic | 0.5 | 全色Panchromatic | 2016-09-17 |
多光谱Multispectral | 2 | 蓝Blue | ||
绿Green | ||||
红Red | ||||
近红外Near-infrared | ||||
Worldview-3 | 全色Panchromatic | 0.5 | 全色Panchromatic | 2016-10-08 |
多光谱Multispectral | 2 | 海岸Coastal | ||
蓝Blue | ||||
绿Green | ||||
黄色Yellow | ||||
红Red | ||||
红边Red edge | ||||
近红外Near-infrared | ||||
近红外2 Near-infrared 2 |
新窗口打开|下载CSV
通过对所获取的3幅遥感影像进行一系列预处理,构建起该研究区高质量的高分辨率时序影像集。其中,预处理主要包括:(1)辐射定标。通过ENVI自带的定标工具,自动解析遥感影像的头文件进行定标,得到辐射亮度值。(2)大气校正。使用FLAASH大气校正模块去除大气及观测角等因素的影响,同时将辐射亮度值转化为反射率,提高遥感影像的精度及一致性。(3)空间重采样。由于SPOT-6、Pleiades-1、Worldview-3卫星影像的光谱及空间分辨率不同,本文利用重采样方法将所有影像统一重采样到6 m分辨率,且仅保留3个卫星传感器共有的红、绿、蓝、近红外4个波段,以保证卫星影像间的可比性。(4)影像配准。运用ENVI影像配准工具对不同时期的卫星影像进行配准,使三景影像的配准误差保持在0.5个像素以内[31]。
1.2.2 训练及检验数据 为了获取更具代表性的作物样本,笔者于2016 年 10月期间,对研究区的主要作物类型(棉花、枣树)进行了实地调查,并用便携式GPS定位仪记录每个样本的位置信息,此次实地调查共收集到样本点600个,以供下一步训练、验证之用,其中棉花200个,枣树200个,其他类别(行道树、建筑、水体等)200个。笔者将获取到的每类实测样本随机地分为训练样本和验证样本两组,每组样本均为100个(训练样本﹕验证样本=1﹕1)。图2展示了各类样点及实测田块的空间分布。
图2
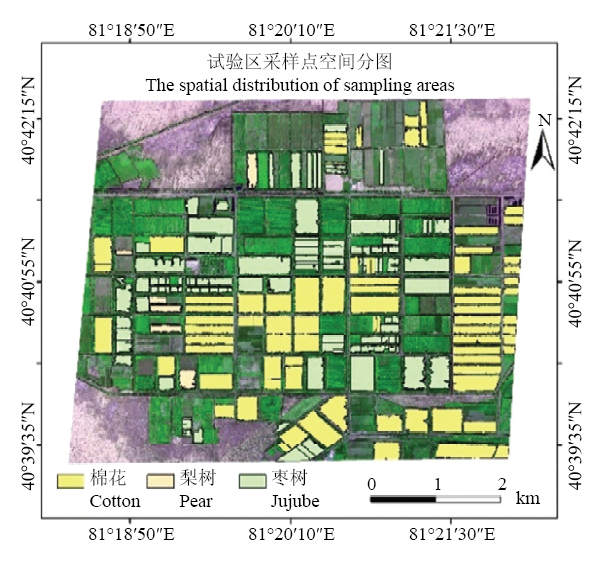
图2采样区空间分布
底图为研究区假彩色影像,R:Red;G:NIR;B:Green,矢量数据为采样区
Fig. 2The spatial distribution of field samples
The base image iWWs a false color image of the study area, R: Red; G: NIR; B: Green, the vector data is the sampling area
1.3 研究方法
图3为本文的技术路线图,本文的研究方法主要包括以下步骤:(1)影像分割。运用基于Sobel算子的分水岭(watershed)分割算法,进行研究区影像的分割及对象特征的获取。(2)作物分类。将传统的光谱角制图(spectral angle mapping,SAM)和3种机器学习方法应用于该研究,进行研究区主要作物的分类和制图。(3)分类精度评价。通过对混淆矩阵中的总体分类精度(overall accuracy,OA)、生产者精度(producer’s accuracies,PA)、用户精度(user’s accuracies,UA)和Kappa系数等指标进行分析,比较生育期对作物分类精度的影响;明确面向对象与基于像素分类方法的差异。图3
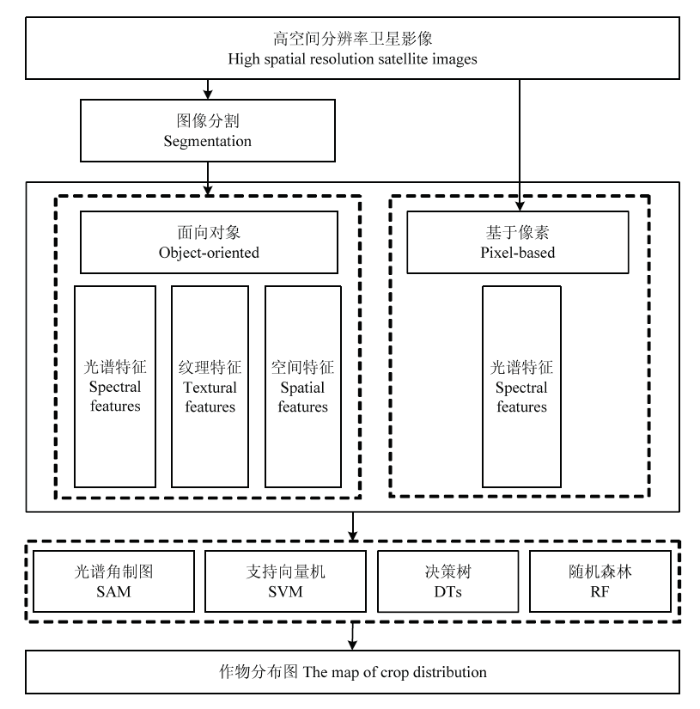
图3作物分类流程图
Fig. 3The flowchart of crop classification
1.3.1 影像分割 图像分割是面向对象图像分析的前提和决定性步骤[11],本研究以ENVI FX(feature extraction)模块中内置的分水岭(watershed)分割算法为基础进行对象获取。分水岭分割是图像分割领域应用最广泛的分割算法之一,在该算法中图像被看作若干相连的盆地,图像的梯度值(gradient)被看作高程,从图像高程局部最低点开始“淹没”图像,在不同盆地水面交汇处(局部最高点)构建水坝,当水平面到达高程最高点时,分割过程停止,该区域被水坝分成若干小块,最终产生一个分割图像,并得到一系列的对象特征(光谱、纹理、空间特征)[32,33]。为了消除分割尺度对地物分类结果的影响,本研究采用单尺度的Sobel-Watershed分割算法(Scale:0;Merge:0)对研究区影像进行分割,以获取棉花和枣树的光谱、纹理、空间特征。
1.3.2 研究区作物分类 在进行作物分类之前,我们运用Jeffries-Matusita算法对获取到的3类训练样本(棉花、枣树、其他)进行了分离度计算,以确定3类样本间的差异程度。计算结果表明,不同样本之间的分离度都大于1.9,所获取的样本之间差异度较大,符合各分类方法的基本要求。
为了比较对象与像素两种空间尺度上信息提取结果的异同,本文将光谱角制图(SAM)、支持向量机(SVM)、决策树(DTs)、随机森林(RF)这4种监督分类方法应用于研究区作物分类,由其对面向对象信息提取过程的优缺点进行客观呈现。为保证作物分类精度,提高分类方法的普适性,SVM分类方法使用目前最为流行的高斯核函数(radial basis function,RBF)作为基础函数,DTs分类方法以CART为基础算法。
本研究立足于面向对象的图像分析技术,通过对研究区棉花、枣树的光谱(16个)、纹理(16个)和空间特征(14个)进行分类,并与以光谱信息为基础的像素尺度的地物分类结果进行对比,探讨面向对象的遥感影像分析技术在作物识别方面的作用。
1.3.3 分类精度评价 分类精度评价分别从像素及对象尺度进行。通过构建混淆矩阵,可以运用生产者精度(producer’s accuracy,PA),用户精度(user’s accuracy,UA),总体精度(overall accuracy,OA)和kappa系数(kappa coefficient,K)对面向对象和基于像素的作物识别精度进行比较,评判各分类方法的优劣。
2 结果
2.1 不同时期作物分类精度的比较
表2总结了面向对象及基于像素的光谱角制图(SAM)、支持向量机(SVM)、决策树(DTs)、随机森林(RF)分类方法对不同时期作物的总体分类精度。从表2可以看出不同时期的作物总体分类精度呈现出显著的差异,作物的总体分类精度在64.62%—93.52%,跨度为28.90%;Kappa系数在0.47—0.90,跨度为0.43。4种分类方法的最高精度均出现在第一时期(2016-05-10),分别为87.47%、90.25%、92.12%、95.35%;最大Kappa系数分别为0.81、0.85、0.88、0.90,作物分类效果较好。图4总结了枣树及棉花不同时期的用户精度和生产者精度。如图4-a和4-b所示,除了SAM之外,其他3种方法的枣树用户精度在不同时期均保持在较高水平,不存在显著性差异;然而,4种方法的棉花用户精度在不同时期的差异较大,且表现出相同的变化趋势,即第一个时期最高(分别为92.05%、70.42%、81.58%、82.29%),第三个时期次之(分别为44.21%、52.51%、54.55%、57.59%),第二个时期最低(分别为69.31%、5.37%、58.84%、62.21%)。与研究区各种作物的用户精度变化趋势相反,枣树的生产者精度在各个时期变化幅度较小,即第一时期最大,第三个时期次之,第二个时期最好;棉花的生产者精度除SAM外基本一致,为86.41%—95.64%,分类效果较好(图4-c和4-d)。Table 2
表2
表2不同时期作物分类总体精度
Table 2
获取时间 Date of image acquisition | 光谱角制图SAM | 支持向量机SVM | 决策树DTs | 随机森林RF | ||||||||||||
---|---|---|---|---|---|---|---|---|---|---|---|---|---|---|---|---|
基于像素 Pixel-based | 基于对象 Object-based | 基于像素 Pixel-based | 基于对象 Object-based | 基于像素 Pixel-based | 基于对像 Object-based | 基于像素 Pixel-based | 基于对象 Object-based | |||||||||
OA | K | OA | K | OA | K | OA | K | OA | K | OA | K | OA | K | OA | K | |
2016-05-10 | 87.47 | 0.81 | 64.62 | 0.47 | 78.35 | 0.67 | 90.25 | 0.85 | 90.93 | 0.86 | 92.12 | 0.88 | 91.27 | 0.87 | 93.52 | 0.90 |
2016-09-07 | 68.56 | 0.52 | 67.36 | 0.50 | 74.23 | 0.62 | 84.04 | 0.76 | 76.90 | 0.66 | 85.36 | 0.78 | 79.37 | 0.69 | 84.40 | 0.77 |
2016-10-08 | 78.30 | 0.67 | 74.21 | 0.61 | 77.53 | 0.67 | 88.88 | 0.83 | 80.13 | 0.70 | 88.77 | 0.83 | 82.14 | 0.73 | 83.82 | 0.78 |
新窗口打开|下载CSV
图4
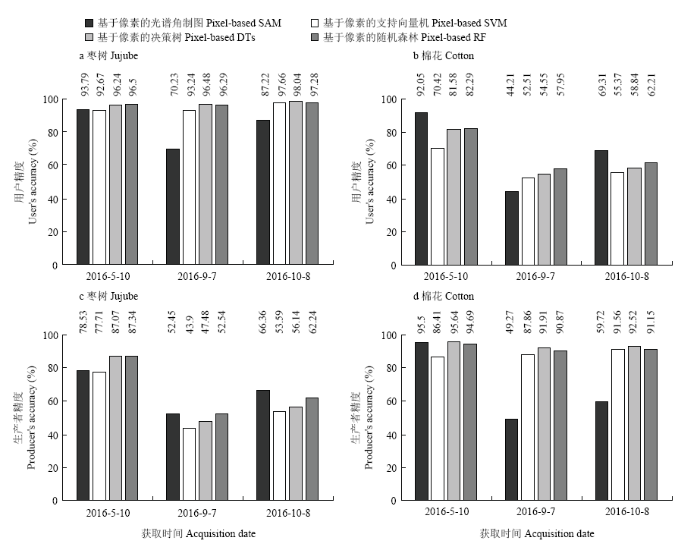
图4基于像素的枣树及棉花各时期用户精度及生产者精度对比
Fig. 4Comparison of user’s accuracies and producer’s accuracies of jujube and cotton for different stages using pixel-based classification methods
2.2 面向对象与基于像素的分类效果比较
除光谱角制图(SAM)外,支持向量机(SVM)、决策树算法(DTs)和随机森林(RF)这3种分类方法在对象水平比在像素水平得到的作物分类精度更高。各时期作物分类总体精度分别提高了9.71%—11.9%(2016-05-10)、1.19%—8.64%(2016-09-07)、1.68%—5.03%(2016-10-08),最高总体分类精度均达到90%以上。就对象水平与像素水平的分类精度差异而言,由随机森林方法得到的增幅最小,支持向量机方法得到的增幅最大,3个时相都表现出同样的趋势。除第二个时期外,其他2个时期的作物精度较高,不便于分析面向对象分类方法对作物分类精度的影响,为了深入分析面向对象分类方法的特点,探究面向对象与基于像素的分类策略对棉花和枣树识别能力的异同,本文选取了分类精度最低的9月份影像进行作物空间分布制图(图5),从而更加深入地理解面向对象分类方法的应用潜力。如表2所示,在使用面向对象的图像分析方法之后,该时期的总体分类精度及Kappa系数均得到了进一步提高(SAM基本保持不变),表3总结了该时期枣树及棉花的分类精度(用户精度及生产者精度),对2种方法的作物分类精度进行对比后发现,面向对象的分类方法(除SAM外)可以显著提升枣树和棉花的分类精度;棉花的用户精度分别提高了12.32%、12.57%、7.71%,棉花的漏分率大幅度降低;枣树的生产者精度也分别提高了16.71%、20.27%、9.65%,制图精度显著提高,枣树的错分率大幅度降低。对作物分布图目视解译后发现,除SAM 外,面向对象的分类可以使枣树的错分率显著降低,枣树的识别面积及空间分布更接近于实际情况。
图5
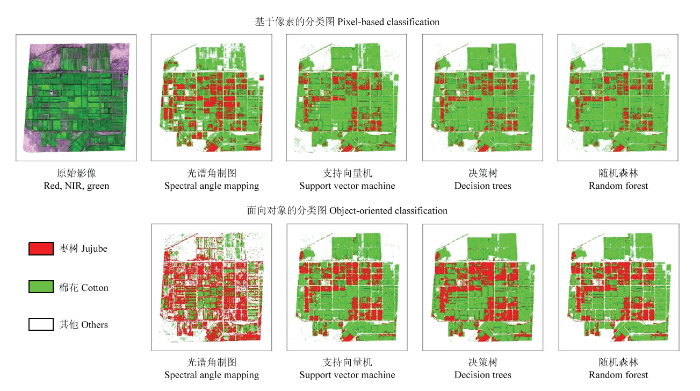
图5基于像素与面向对象的单时期(2016-09-07)作物分布图
Fig. 5Maps of crop classification from the image of September 7, 2016 using four classification methods at pixel and object levels
Table 3
表3
表3像素与对象水平的枣树及棉花单时期(2016-09-07)分类精度比较
Table 3
空间尺度 Spatial scale | 光谱角制图SAM | 支持向量机SVM | 决策树DTs | 随机森林RF | ||||||||||||
---|---|---|---|---|---|---|---|---|---|---|---|---|---|---|---|---|
枣树Jujube | 棉花Cotton | 枣树Jujube | 棉花Cotton | 枣树Jujube | 棉花Cotton | 枣树Jujube | 棉花Cotton | |||||||||
UA | PA | UA | PA | UA | PA | UA | PA | UA | PA | UA | PA | UA | PA | UA | PA | |
面向对象 Object-oriented | 69.17 | 50.44 | 63.81 | 54.40 | 96.17 | 60.61 | 64.47 | 96.09 | 97.65 | 67.75 | 67.12 | 92.49 | 98.29 | 62.19 | 65.76 | 92.29 |
基于像素 Pixel-based | 70.23 | 52.45 | 44.21 | 49.27 | 93.24 | 43.90 | 52.15 | 87.86 | 96.48 | 47.48 | 54.55 | 91.91 | 96.29 | 52.54 | 57.95 | 90.87 |
新窗口打开|下载CSV
如表2所示,本文所使用的4种分类方法中SVM、DTs、RF这3种分类算法在各个时期均可实现较高的作物分类总体精度,分类结果均明显优于SAM算法。但这3类算法的分类精度并未表现出明显差异。
3 讨论
3.1 多时相卫星影像的应用潜力
棉花和枣树为研究区内的两大作物,受当地气候及耕作管理措施的影响,随着时间的推移这两类作物往往呈现出明显的时空变化特征。大量的研究已经表明生育期是进行作物识别的关键信息,这种信息会对作物分类结果产生不可忽视的影响[34,35,36],然而大多数作物识别的研究都集中于对时间序列的分析,单个时期或关键生育期相互比较的研究往往少有涉及[37,38],因高空间分辨率卫星重返周期长、影像获取成本高,采用关键生育期的影像进行作物分类通常更具实用价值。图6展示了研究区不同时期(2016-05-10、2016- 09-07、2016-10-08)的地物分布情况,从图中可以看出5月份影像呈现出特有的地物分布特征,即棉花田呈现出白色,经实地调研后发现该时期(棉花苗期)的棉田被地膜所覆盖,覆膜为新疆棉花苗期(生长早期)管理的一项重要的措施,且持续时间较长,该时期的棉花田与其他地物差异最大,所以棉花的分类效果最好;除此之外该时期的枣树处于展叶期,呈现出一定的植被光谱特征,且研究区作物/地物类型相对较少,所以作物分类效果相对较好。因此,利用新疆棉花和枣树生长早期的差异性光谱特征,可以对这两类农作物进行有效识别。
图6
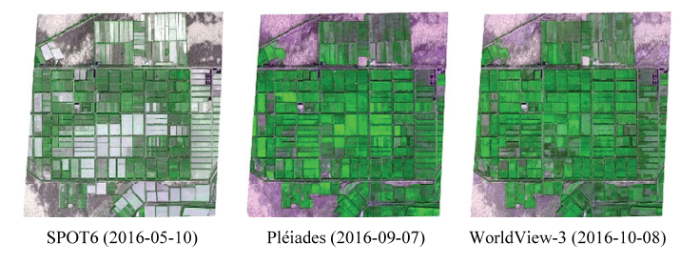
图6研究区不同时期的假彩色合成图
Fig. 6False color composites of the study area generated with satellite imagery acquired at different stages
目前在轨的高分商业卫星通常获取成本高,很难从同一卫星获取作物生长季内的时序影像,甚至是多幅影像,这也是目前高分影像在农业应用中所面临的一大现实问题。受高分影像获取难度大的限制,本研究开展当年仅有3景来自于不同传感器的高分卫星影像能覆盖研究区。虽然未能完整捕捉到棉花和枣树所有关键生育期的光谱特征,而且3幅影像的空间分辨率和波段数量不同,但他们都拥有红、绿、蓝、近红4个波段,而且可以重采样到最低的6 m分辨率,目前仍能从现有的3景影像分析影像获取时期对作物分类精度的影响,最大限度发挥历史影像在作物识别中的应用价值。因作物类型、种植面积及种植区域可能存在较大的年际变化,加入其他年度的影像会带入额外的不确定性,所以本研究没有加入相邻年度的遥感影像。在未来的研究中,可以对完整时间序列影像进行深入分析,进一步改善作物分类效果。
3.2 面向对象方法识别作物的优势
通过面向对象的图像处理我们可以对光谱、纹理及空间信息进行综合利用,虽然面向对象的策略在作物分类中表现出一定的潜力[39],但在我国尤其是新疆的作物分类应用中还不多见,面向对象分类过程中光谱及纹理等特征所起的作用依然不够明确[40,41,42]。明确光谱、纹理及空间特征在作物分类中的重要性,对丰富作物分类体系、进一步提高作物分类效率大有裨益。相较于9月份影像,10月份影像的作物分类精度出现了一定程度的提高(表2)。受当地气候的影响,枣树和棉花的反射率值在10月份都会经历一个下降阶段,此时部分棉花已经开始收获,受棉花分批成熟、多次收获的影响,棉花田内部表现出光谱差异增大的特征,但10月份影像分类精度却出现增高趋势,这种精度的提高很可能是由纹理信息的加入所致。
在对象水平,随机森林分类算法对9月份影像的作物分类精度提升效果最为明显(表2),所以我们对该时期分类过程中所有变量的重要性进行了探究。图7展示了参与分类的46个特征的重要性,从图中可以看出重要性排名前3的特征分别是Texture Red Mean、Spectral Red Mean、Texture Blue Mean;紧接着的2个特征分别是Texture Green Mean、Spectral Green Max。以上表明纹理特征的重要性高于光谱特征,纹理特征所占比例在前3和前5中均达到一半以上。在参与分类的46个特征中,Texture Red Mean重要性最高,Spectral Red Mean次之,红波段位于叶绿素的主要吸收带,另外,由于枣树冠层有明显的行状特征,纹理特征明显,所以Texture Red Mean在研究区作物的分类中具有很高的重要性。除此之外,Texture Green Mean、Spectral Green Max也表现出较高的重要性,该时期的棉花处于成熟吐絮前期,而枣树处于果实膨大期,此时棉花的棉絮和棉铃大范围出现,棉絮和棉铃叶绿素含量较低,而枣树冠层不存在这种变化,枣树和棉花绿波段的反射率存在差异,所以该时期的绿波段对枣树和棉花有较好的区分效果。但是以往作物分类中常常使用的近红外波段在本研究中却排名靠后,这可能与枣树和棉花在该时期近红外波段的差异较小有关。
图7
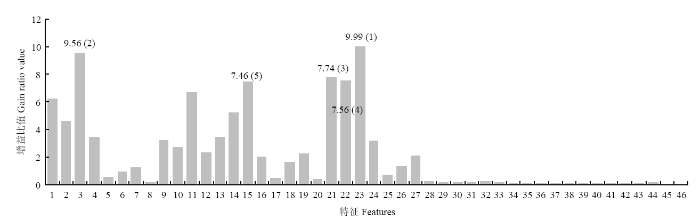
图7基于增益比值的特征重要性
1—4:蓝、绿、红、近红外波段光谱均值 Spectral Blue, Green, Red, NIR Mean;5—8:蓝、绿、红、近红外波段光谱标准差 Spectral Blue, Green, Red, NIR Std;9—12:蓝、绿、红、近红外波段光谱最小值 Spectral Blue, Green, Red, NIR Min;13—16:蓝、绿、红、近红外波段光谱最大值 Spectral Blue, Green, Red, NIR Max;17—20:蓝、绿、红、近红外波段纹理范围 Texture Blue, Green, Red, NIR Range;21—24:蓝、绿、红、近红外波段纹理均值Texture Blue, Green, Red, NIR Mean;25—28:蓝、绿、红、近红外波段纹理方差 Texture Blue, Green, Red, NIR Variance;29—32:蓝、绿、红、近红外波段纹理信息熵 Texture Blue, Green, Red, NIR Entropy;33:对象面积 Area;34:对象外边框周长 Length;35:紧凑型 Compactness;36:凸出的状态 Convexity;37:坚固性 Solidity;38:圆特性 Roundness;39:形状要素 Form Factor;40:延伸性 Elongation;41:矩形形状的度量 Rectangular Fit;42:主方向 Main Direction;43—44:长、短轴长度 Major, Minor Length;45:洞的个数 Number of Holes;46:对象和外轮廓的面积比 Hole Area/Solid Area
Fig. 7Importance of features based on the gain ratio
4 结论
本研究以多时相的高空间分辨率卫星影像为基本数据源,采用多种分类法(SAM、SVM、DTs、RF)对研究区主要作物(棉花、枣树)进行分类,全面比较了基于像素和面向对象的分类效果,结果表明:(1)5月份棉花覆膜期的作物分类精度最高,新疆棉花和枣树在生长早期具有独特的空间特征,新疆枣树和棉花的遥感识别应在作物生长早期进行;将作物光谱特征与栽培特性相结合,具有提高作物分类精度的潜力。
(2)在4种监督分类方法中,决策树和随机森林方法比其他分类方法的作物分类效果更好,但随机森林输入参数更少,优势相对明显。
(3)与基于像素的方法相比,面向对象的分类算法可以综合使用纹理与光谱特征,取得更高的分类精度,最高可达93.52%;3种机器学习方法在像素水平和对象水平间的分类精度差异不同;分类图中的田块完整性好,分类结果中的田块边界可以为当地农田信息化管理提供重要支撑。
(4)在棉花和枣树识别过程中,纹理特征的重要性高于光谱和空间特征;相较于其他波段,红波段和绿波段的反射率对棉花和枣树识别的贡献更大。
参考文献 原文顺序
文献年度倒序
文中引用次数倒序
被引期刊影响因子
[本文引用: 1]
[本文引用: 1]
DOI:10.6046/gtzyyg.2015.04.09URLMagsci [本文引用: 1]

<p>针对不同的农作物种植结构区,研究影响遥感影像分类各因素与农作物种植面积估算精度的定性和定量关系是十分必要的。以RapidEye影像提取的早稻种植信息为研究对象,从农作物的种植成数、种植破碎度和地块形状指数3个角度进行了不同空间分辨率下各因素对农作物面积监测的影响研究。结果表明: 随着农作物种植成数的降低,种植结构越来越破碎,种植地块趋于狭长分布,各分辨率下农作物面积估算精度均呈递减趋势; 要达到85%以上的面积估算精度,当作物种植成数在50%以上时,可选取高于150 m分辨率的遥感数据; 当作物种植较为破碎时,需要在提高影像空间分辨率的同时融入其他技术手段; 当作物种植地块为狭长分布时,提高影像的空间分辨率并不能保证面积估算精度,必须通过其他技术手段达到精度要求; 并最终得到了4种影响因素对面积估算精度的定量评估模型。研究结果为解决不同农作物种植结构区遥感数据的选择、面积估算精度的提高,以及在特定研究区和数据源条件下可达到的面积估算水平等问题提供了理论基础。</p>
DOI:10.6046/gtzyyg.2015.04.09URLMagsci [本文引用: 1]

<p>针对不同的农作物种植结构区,研究影响遥感影像分类各因素与农作物种植面积估算精度的定性和定量关系是十分必要的。以RapidEye影像提取的早稻种植信息为研究对象,从农作物的种植成数、种植破碎度和地块形状指数3个角度进行了不同空间分辨率下各因素对农作物面积监测的影响研究。结果表明: 随着农作物种植成数的降低,种植结构越来越破碎,种植地块趋于狭长分布,各分辨率下农作物面积估算精度均呈递减趋势; 要达到85%以上的面积估算精度,当作物种植成数在50%以上时,可选取高于150 m分辨率的遥感数据; 当作物种植较为破碎时,需要在提高影像空间分辨率的同时融入其他技术手段; 当作物种植地块为狭长分布时,提高影像的空间分辨率并不能保证面积估算精度,必须通过其他技术手段达到精度要求; 并最终得到了4种影响因素对面积估算精度的定量评估模型。研究结果为解决不同农作物种植结构区遥感数据的选择、面积估算精度的提高,以及在特定研究区和数据源条件下可达到的面积估算水平等问题提供了理论基础。</p>
DOI:10.1016/j.isprsjprs.2015.09.013URL [本文引用: 1]

Global, timely, accurate and cost-effective cropland mapping is a prerequisite for reliable crop condition monitoring. This article presented a simple and comprehensive methodology capable to meet the requirements of operational cropland mapping by proposing (1) five knowledge-based temporal features that remain stable over time, (2) a cleaning method that discards misleading pixels from a baseline land cover map and (3) a classifier that delivers high accuracy cropland maps (>80%). This was demonstrated over four contrasted agrosystems in Argentina, Belgium, China and Ukraine. It was found that the quality and accuracy of the baseline impact more the certainty of the classification rather than the classification output itself. In addition, it was shown that interpolation of the knowledge-based features increases the stability of the classifier allowing for its re-use from year to year without recalibration. Hence, the method shows potential for application at larger scale as well as for delivering cropland map in near real time.
DOI:10.3390/s17061373URLPMID:5492153 [本文引用: 1]

Classification methods based on Gaussian Markov Measure Field Models and other probabilistic approaches have to face the problem of construction of the likelihood. Typically, in these methods, the likelihood is computed from 1D or 3D histograms. However, when the number of information sources grows, as in the case of satellite images, the histogram construction becomes more difficult due to the high dimensionality of the feature space. In this work, we propose a generalization of Gaussian Markov Measure Field Models and provide a probabilistic segmentation scheme, which fuses multiple information sources for image segmentation. In particular, we apply the general model to classify types of crops in satellite images. The proposed method allows us to combine several feature spaces. For this purpose, the method requires prior information for building a 3D histogram for each considered feature space. Based on previous histograms, we can compute the likelihood of each site of the image to belong to a class. The computed likelihoods are the main input of the proposed algorithm and are combined in the proposed model using a contrast criteria. Different feature spaces are analyzed, among them are 6 spectral bands from LANDSAT 5 TM, 3 principal components from PCA on 6 spectral bands and 3 principal components from PCA applied on 10 vegetation indices. The proposed algorithm was applied to a real image and obtained excellent results in comparison to different classification algorithms used in crop classification.
DOI:10.1080/0143116031000139791URL [本文引用: 1]
DOI:10.14358/PERS.79.11.1053URL [本文引用: 1]

This research investigates the accuracy of pixel- and object-based classification techniques across varying spatial resolutions to identify crop types at parcel level and estimate the area at six test sites to find the optimum data source for the identification of crop parcels. Multi-sensor data with spatial resolutions of 2.5 m, 5 m and 10 m from SPOT5 and 30 m from Landsat-5 TM were used. Maximum Likelihood (ML), Spectral Angle Mapper (SAM), and Support Vector Machines (SVM) were used as pixel-based methods in addition to object-based image classification (OBC). Post-classification methods were applied to the output of pixel-based classification to minimize the noise effects and heterogeneity within the agricultural parcels. In addition, processing-time performance of the algorithms was evaluated for the test sites and district scale classification. OBC results provided comparatively the best performance for both parcel identification and area estimation at 10 m and finer spatial resolution levels. SVM followed OBC at 2.5 m and 5 m resolutions but accuracies decreased dramatically with coarser resolutions. ML and SAM results were worse up to 30 m resolution for both crop type identification and area estimation. In general, parcel identification efficiency was strongly correlated with spatial resolution while the classification algorithm was a more effective factor than spatial resolution for area estimation accuracy. Results also provided an opportunity to discuss the effects of image resolution and the classification algorithm independent factors such as parcel size, spatial distribution of crop types and crop patterns.
DOI:10.3390/rs1040875URL [本文引用: 1]

Nearest neighbor techniques are commonly used in remote sensing, pattern recognition and statistics to classify objects into a predefined number of categories based on a given set of predictors. These techniques are especially useful for highly nonlinear relationship between the variables. In most studies the distance measure is adopted a priori. In contrast we propose a general procedure to find an adaptive metric that combines a local variance reducing technique and a linear embedding of the observation space into an appropriate Euclidean space. To illustrate the application of this technique, two agricultural land cover classifications using mono-temporal and multi-temporal Landsat scenes are presented. The results of the study, compared with standard approaches used in remote sensing such as maximum likelihood (ML) or k-Nearest Neighbor (k-NN) indicate substantial improvement with regard to the overall accuracy and the cardinality of the calibration data set. Also, using MNN in a soft/fuzzy classification framework demonstrated to be a very useful tool in order to derive critical areas that need some further attention and investment concerning additional calibration data.
[本文引用: 1]
DOI:10.1016/j.rse.2006.11.021URL [本文引用: 1]

The global environmental change research community requires improved and up-to-date land use/land cover (LULC) datasets at regional to global scales to support a variety of science and policy applications. Considerable strides have been made to improve large-area LULC datasets, but little emphasis has been placed on thematically detailed crop mapping, despite the considerable influence of management activities in the cropland sector on various environmental processes and the economy. Time-series MODIS 250 m Vegetation Index (VI) datasets hold considerable promise for large-area crop mapping in an agriculturally intensive region such as the U.S. Central Great Plains, given their global coverage, intermediate spatial resolution, high temporal resolution (16-day composite period), and cost-free status. However, the specific spectral emporal information contained in these data has yet to be thoroughly explored and their applicability for large-area crop-related LULC classification is relatively unknown. The objective of this research was to investigate the general applicability of the time-series MODIS 250 m Enhanced Vegetation Index (EVI) and Normalized Difference Vegetation Index (NDVI) datasets for crop-related LULC classification in this region. A combination of graphical and statistical analyses were performed on a 12-month time-series of MODIS EVI and NDVI data from more than 2000 cropped field sites across the U.S. state of Kansas. Both MODIS VI datasets were found to have sufficient spatial, spectral, and temporal resolutions to detect unique multi-temporal signatures for each of the region's major crop types (alfalfa, corn, sorghum, soybeans, and winter wheat) and management practices (double crop, fallow, and irrigation). Each crop's multi-temporal VI signature was consistent with its general phenological characteristics and most crop classes were spectrally separable at some point during the growing season. Regional intra-class VI signature variations were found for some crops across Kansas that reflected the state's climate and planting time differences. The multi-temporal EVI and NDVI data tracked similar seasonal responses for all crops and were highly correlated across the growing season. However, differences between EVI and NDVI responses were most pronounced during the senescence phase of the growing season.
DOI:10.1016/j.rse.2011.11.020URL [本文引用: 1]

78 Pixel-based (PB) and object-based (OB) classifications are compared. 78 Three machine learning algorithms (MLAs) are examined. 78 No statistical difference between PB and OB classifications was found. 78 For OB classifications, significant differences between MLAs were found.
DOI:10.14358/PERS.79.11.1053URL [本文引用: 2]

This research investigates the accuracy of pixel- and object-based classification techniques across varying spatial resolutions to identify crop types at parcel level and estimate the area at six test sites to find the optimum data source for the identification of crop parcels. Multi-sensor data with spatial resolutions of 2.5 m, 5 m and 10 m from SPOT5 and 30 m from Landsat-5 TM were used. Maximum Likelihood (ML), Spectral Angle Mapper (SAM), and Support Vector Machines (SVM) were used as pixel-based methods in addition to object-based image classification (OBC). Post-classification methods were applied to the output of pixel-based classification to minimize the noise effects and heterogeneity within the agricultural parcels. In addition, processing-time performance of the algorithms was evaluated for the test sites and district scale classification. OBC results provided comparatively the best performance for both parcel identification and area estimation at 10 m and finer spatial resolution levels. SVM followed OBC at 2.5 m and 5 m resolutions but accuracies decreased dramatically with coarser resolutions. ML and SAM results were worse up to 30 m resolution for both crop type identification and area estimation. In general, parcel identification efficiency was strongly correlated with spatial resolution while the classification algorithm was a more effective factor than spatial resolution for area estimation accuracy. Results also provided an opportunity to discuss the effects of image resolution and the classification algorithm independent factors such as parcel size, spatial distribution of crop types and crop patterns.
DOI:10.5589/m05-027URL [本文引用: 1]

A back-propagation artificial neural network (ANN) was applied to classify multispectral remote sensing imagery data. The classification procedure included four steps: (i) noisy training that adds minor random variations to the sampling data to make the data more representative and to reduce the training sample size; (ii) iterative or multi-tier classification that reclassifies the unclassified pixels by making a subset of training samples from the original training set, which means the neural model can focus on fewer classes; (iii) spectral channel selection based on neural network weights that can distinguish the relative importance of each channel in the classification process to simplify the ANN model; and (iv) voting rules that adjust the accuracy of classification and produce outputs of different confidence levels. The Purdue Forest, located west of Purdue University, West Lafayette, Indiana, was chosen as the test site. The 1992 Landsat thematic mapper imagery was used as the input data. High-quality airborne photographs of the same time period were used for the ground truth. A total of 11 land use and land cover classes were defined, including water, broadleaved forest, coniferous forest, young forest, urban and road, and six types of cropland090009grassland. The experiment indicated that the back-propagation neural network application was satisfactory in distinguishing different land cover types at US Geological Survey levels II090009III. The single-tier classification reached an overall accuracy of 85%, and the multi-tier classification an overall accuracy of 95%. For the whole test region, the final output of this study reached an overall accuracy of 87%.
DOI:10.1007/s12524-008-0018-yURL [本文引用: 1]

Remote sensing provides a lucid and effective means for crop coverage identification. Crop coverage identification is a very important technique, as it provides vital information on the type and extent of crop cultivated in a particular area. This information has immense potential in the planning for further cultivation activities and for optimal usage of the available fertile land. As the frontiers of space technology advance, the knowledge derived from the satellite data has also grown in sophistication. Further, image classification forms the core of the solution to the crop coverage identification problem. No single classifier can prove to satisfactorily classify all the basic crop cover mapping problems of a cultivated region. We present in this paper the experimental results of multiple classification techniques for the problem of crop cover mapping of a cultivated region. A detailed comparison of the algorithms inspired by social behaviour of insects and conventional statistical method for crop classification is presented in this paper. These include the Maximum Likelihood Classifier (MLC), Particle Swarm Optimisation (PSO) and Ant Colony Optimisation (ACO) techniques. The high resolution satellite image has been used for the experiments.
[D].
[本文引用: 1]
.
DOI:10.1109/TGRS.2011.2179050URL [本文引用: 1]

Earth observation satellites are now providing images with short revisit cycle and high spatial resolution. The amount of produced data requires new methods that will give a sound temporal analysis while being computationally efficient. Dynamic time warping has proved to be a very sound measure to capture similarities in radiometric evolutions. In this letter, we show that its nonlinear distortion behavior is compatible with the use of a spatiotemporal segmentation of the data cube that is formed by a satellite image time series (SITS). While dealing with spatial and temporal dimensions of SITS at the same time had already proven to be very challenging, this letter proves that, by taking advantage of the spatial and temporal connectivities, both the performance and the quality of the analysis can be improved. Our method is assessed on a SITS of 46 Formosat -2 images sensed in 2006, with an average cloud cover of one third. We show that our approach induces the following: 1) sharply reduced memory usage; 2) improved classification results; and 3) shorter running time.
DOI:10.1016/j.compag.2015.02.015URL [本文引用: 1]

Detailed and timely information on crop area, production and yield is important for the assessment of environmental impacts of agriculture, for the monitoring of the land use and management practices, and for food security early warning systems. A machine learning approach is proposed to model crop rotations which can predict with good accuracy, at the beginning of the agricultural season, the crops most likely to be present in a given field using the crop sequence of the previous 3–5years. The approach is able to learn from data and to integrate expert knowledge represented as first-order logic rules. Its accuracy is assessed using the French Land Parcel Information System implemented in the frame of the EU’s Common Agricultural Policy. This assessment is done using different settings in terms of temporal depth and spatial generalization coverage. The obtained results show that the proposed approach is able to predict the crop type of each field, before the beginning of the crop season, with an accuracy as high as 60%, which is better than the results obtained with current approaches based on remote sensing imagery.
DOI:10.1016/j.rse.2017.10.005URL [本文引用: 1]

Efficient methodologies for mapping croplands are an essential condition for the implementation of sustainable agricultural practices and for monitoring crops periodically. The increasing spatial and temporal resolution of globally available satellite images, such as those provided by Sentinel-2, creates new possibilities for generating accurate datasets on available crop types, in ready-to-use vector data format. Existing solutions dedicated to cropland mapping, based on high resolution remote sensing data, are mainly focused on pixel-based analysis of time series data. This paper evaluates how a time-weighted dynamic time warping (TWDTW) method that uses Sentinel-2 time series performs when applied to pixel-based and object-based classifications of various crop types in three different study areas (in Romania, Italy and the USA). The classification outputs were compared to those produced by Random Forest (RF) for both pixel- and object-based image analysis units. The sensitivity of these two methods to the training samples was also evaluated. Object-based TWDTW outperformed pixel-based TWDTW in all three study areas, with overall accuracies ranging between 78.05% and 96.19%; it also proved to be more efficient in terms of computational time. TWDTW achieved comparable classification results to RF in Romania and Italy, but RF achieved better results in the USA, where the classified crops present high intra-class spectral variability. Additionally, TWDTW proved to be less sensitive in relation to the training samples. This is an important asset in areas where inputs for training samples are limited.
DOI:10.11873/j.issn.1004-0323.2015.4.0775URLMagsci [本文引用: 1]

<p>针对基于多时相遥感影像、多种特征量提取多种作物种植结构在我国研究较少的现状,利用多时相Landsat8 OLI影像数据,根据温宿县不同作物的农事历,通过分析主要地物的光谱特征和归一化植被指数的时间变化信息,构建不同作物种植结构提取的决策树模型,实现了对温宿县多种作物种植结构信息的提取。结果表明:①水稻的最佳识别依据是5月20日影像的近红外波段和7月23日影像的NDVI值;棉花和春玉米的最佳识别依据是5月20日~9月9日影像的NDVI变化值;冬小麦—夏玉米和林果的最佳识别依据是5月20日~7月23日影像的NDVI变化值;②与单时相监督分类相比,多时相决策树法对多种作物种植结构的提取效果更理想,总体精度提高了7.90%,Kappa系数提高了0.10;③Landsat8 OLI影像数据分辨率高,成本低,获取方便,是农作物遥感的良好数据源。</p>
DOI:10.11873/j.issn.1004-0323.2015.4.0775URLMagsci [本文引用: 1]

<p>针对基于多时相遥感影像、多种特征量提取多种作物种植结构在我国研究较少的现状,利用多时相Landsat8 OLI影像数据,根据温宿县不同作物的农事历,通过分析主要地物的光谱特征和归一化植被指数的时间变化信息,构建不同作物种植结构提取的决策树模型,实现了对温宿县多种作物种植结构信息的提取。结果表明:①水稻的最佳识别依据是5月20日影像的近红外波段和7月23日影像的NDVI值;棉花和春玉米的最佳识别依据是5月20日~9月9日影像的NDVI变化值;冬小麦—夏玉米和林果的最佳识别依据是5月20日~7月23日影像的NDVI变化值;②与单时相监督分类相比,多时相决策树法对多种作物种植结构的提取效果更理想,总体精度提高了7.90%,Kappa系数提高了0.10;③Landsat8 OLI影像数据分辨率高,成本低,获取方便,是农作物遥感的良好数据源。</p>
DOI:10.6041/j.issn.1000-1298.2017.10.017URL [本文引用: 1]

为快速获取大范围种植结构复杂区域的作物种植面积,以MODIS数据为数据源,选择归一化植被指数(Normalized difference vegetation index,NDVI)、增强植被指数(Enhanced vegetation index,EVI)、宽动态植被指数(Wide dynamic range vegetation index,WDRVI)、地表水分指数(Land surface water index,LSWI)、归一化雪被指数(Normalized difference snow index,NDSI)5种特征,结合同步的实地调查样本点,采用支持向量机算法(Support vector machines,SVM)提取黑龙江省主要农作物的种植面积.研究表明,在待选特征中NDVI、EVI与LSWI指数组合取得了最高的分类精度,总体分类精度为74.18%,Kappa系数为0.60;支持向量机算法与最大似然算法、随机森林算法相比,分类精度更优.该方法为在大区域中提取农作物种植面积提供了参考价值.
DOI:10.6041/j.issn.1000-1298.2017.10.017URL [本文引用: 1]

为快速获取大范围种植结构复杂区域的作物种植面积,以MODIS数据为数据源,选择归一化植被指数(Normalized difference vegetation index,NDVI)、增强植被指数(Enhanced vegetation index,EVI)、宽动态植被指数(Wide dynamic range vegetation index,WDRVI)、地表水分指数(Land surface water index,LSWI)、归一化雪被指数(Normalized difference snow index,NDSI)5种特征,结合同步的实地调查样本点,采用支持向量机算法(Support vector machines,SVM)提取黑龙江省主要农作物的种植面积.研究表明,在待选特征中NDVI、EVI与LSWI指数组合取得了最高的分类精度,总体分类精度为74.18%,Kappa系数为0.60;支持向量机算法与最大似然算法、随机森林算法相比,分类精度更优.该方法为在大区域中提取农作物种植面积提供了参考价值.
DOI:10.1016/j.rse.2017.06.040URL [本文引用: 1]

Very high resolution (VHR) satellite data is experiencing rapid annual growth, producing petabytes of remotely sensed data per year. The WorldView constellation, operated by DigitalGlobe, images over 1.202billion02km 2 annually at <02202m spatial resolution. Due to computation, data cost, and methodological concerns, VHR satellite data has mainly been used to produce needed geospatial information for site-specific phenomena. This project produced a VHR spatiotemporally explicit wall-to-wall cropland area map for the rainfed residential cropland mosaic of the Tigray Region, Ethiopia, which is comprised mostly of smallholder farms. Moderate resolution satellite data do not have adequate spatial resolution to capture the total area occupied by smallholder farms, i.e., farms with agricultural fields of ≤024502×024502m in dimension. In order to accurately map smallholder cropped area over a large region, hundreds of VHR images spanning two or more years are needed. Sub-meter WorldView-1 and WorldView-2 segmentation results were combined with median phenology amplitude from Landsat 8 data to map cropped area. Over 2700 VHR WorldView-1, -2 data were obtained from the U.S. National Geospatial-Intelligence Agency (NGA) via the NextView license agreement and were processed from raw imagery to produce a smallholder crop map in ~02102week using a semi-automated method with the large computing capacity of the Advanced Data Analytics Platform. We estimated cropped area in Tigray to be 46% with a commission error of 5%02±0210% and omission error of 15%02±0212%. This methodology is extensible to other regions with similar vegetation texture and can easily be expanded to run on much larger regions.
DOI:10.3390/rs9050488URL [本文引用: 1]

Leaf area index (LAI) is a key input in models describing biosphere processes and has widely been used in monitoring crop growth and in yield estimation. In this study, a hybrid inversion method is developed to estimate LAI values of winter oilseed rape during growth using high spatial resolution optical satellite data covering a test site located in southeast China. Based on PROSAIL (coupling of PROSPECT and SAIL) simulation datasets, nine vegetation indices (VIs) were analyzed to identify the optimal independent variables for estimating LAI values. The optimal VIs were selected using curve fitting methods and the random forest algorithm. Hybrid inversion models were then built to determine the relationships between optimal simulated VIs and LAI values (generated by the PROSAIL model) using modeling methods, including curve fitting, k-nearest neighbor (kNN), and random forest regression (RFR). Finally, the mapping and estimation of winter oilseed rape LAI using reflectance obtained from Pleiades-1A, WorldView-3, SPOT-6, and WorldView-2 were implemented using the inversion method and the LAI estimation accuracy was validated using ground-measured datasets acquired during the 2014 2015 growing season. Our study indicates that based on the estimation results derived from different datasets, RFR is the optimal modeling algorithm amidst curve fitting and kNN with R2 > 0.954 and RMSE <0.218. Using the optimal VIs, the remote sensing-based mapping of winter oilseed rape LAI yielded an accuracy of R2 = 0.520 and RMSE = 0.923 (RRMSE = 93.7%). These results have demonstrated the potential operational applicability of the hybrid method proposed in this study for the mapping and retrieval of winter oilseed rape LAI values at field scales using multi-source and high spatial resolution optical remote sensing datasets. Details provided by this high resolution mapping cannot be easily discerned at coarser mapping scales and over larger spatial extents that usually employ lower resolution satellite images. Our study therefore has significant implications for field crop monitoring at local scales, providing relevant data for agronomic practices and precision agriculture.
DOI:10.7621/cjarrp.1005-9121.20130601URL [本文引用: 1]

近10多年来,国内外农田生态系统碳足迹研究蓬勃开展并取得了某些成果。同时也存在着问题与误区,主要是碳流路径的短路和指标逻辑起点不一,导致研究结果的失真与扭曲。该文论述了全环式与半环式碳流路径的利弊,提出了改进3原则,对当前世界流行的5种指标体系进行比较、综合、筛选、补充与改进,初步形成了适合中国实际情况的包括碳流路径、指标体系及相应参数的碳足迹方法论,并通过1950年以来的全国性农田系统碳流分析和现代高效农田的案例加以验证和剖析,与当前流行的一些主流观点相悖,运用改进的碳足迹法进行的案例研究发现:(1)与多年生林木相似,农田上连年种植的一年生作物同样具有净固碳作用;(2)1952~2012年期间,随着农业集约化程度的不断提高,全国农田生态系统碳效率仍保持在正平衡状态;(3)尽管农田化合物大量投入,但在农田生态系统内增加的固碳量仍超过耗碳量,化学合成物在农田生态系统的合理应用也有减少温室气体的可能性。
DOI:10.7621/cjarrp.1005-9121.20130601URL [本文引用: 1]

近10多年来,国内外农田生态系统碳足迹研究蓬勃开展并取得了某些成果。同时也存在着问题与误区,主要是碳流路径的短路和指标逻辑起点不一,导致研究结果的失真与扭曲。该文论述了全环式与半环式碳流路径的利弊,提出了改进3原则,对当前世界流行的5种指标体系进行比较、综合、筛选、补充与改进,初步形成了适合中国实际情况的包括碳流路径、指标体系及相应参数的碳足迹方法论,并通过1950年以来的全国性农田系统碳流分析和现代高效农田的案例加以验证和剖析,与当前流行的一些主流观点相悖,运用改进的碳足迹法进行的案例研究发现:(1)与多年生林木相似,农田上连年种植的一年生作物同样具有净固碳作用;(2)1952~2012年期间,随着农业集约化程度的不断提高,全国农田生态系统碳效率仍保持在正平衡状态;(3)尽管农田化合物大量投入,但在农田生态系统内增加的固碳量仍超过耗碳量,化学合成物在农田生态系统的合理应用也有减少温室气体的可能性。
DOI:10.1080/01431160600784192URL [本文引用: 1]

Remote sensing provides one way of obtaining more accurate information on total cropped area and crop types in irrigated areas. The technique is particularly well suited to arid and semi‐arid areas where almost all vegetative growth is associated with irrigation. In order to obtain more information with regard to crop patterns in the irrigated areas in the Zayandeh Rud basin, a classification analysis was made of the Landsat 7 image of 2 July 2000. The target of the classification was to primarily focus on the agricultural land use. The date of the image fell in the transition period where the first crops were harvested and many fields were being prepared for the second crop. The image has therefore captured an instantaneous picture of a system generally in transition from the first to the second crop, but with significant differences from system to system, both with respect to crop types and agricultural cycles. The overall accuracy of image registration was about 3002m (one pixel). Fieldwork was conducted on various occasions in August–October 2000 and May–October 2001. Farmers were interviewed to determine the situation on 2 July 2000. Fields were mapped in detail with the GPS instruments, and data compiled for 112 fields. Using a supervised classification system, training areas were selected and initial classifications were made to determine the validity of the classes. After merging several classes and testing several new classes a final classification system was made. All seven Landsat bands were used in the determination of the feature statistics. The final classification was made with the minimum distance algorithm. The statistics with respect to areas and crop type for the districts was obtained by crossing the raster map with the irrigation district raster map. The results with respect to crop type and total irrigated area per district were compared with those of previous studies. This included both NOAA/AVHRR and conventional agricultural district statistics.
DOI:10.1071/AR06279URL [本文引用: 1]

Cereal grain is one of the main export commodities of Australian agriculture. Over the past decade, crop yield forecasts for wheat and sorghum have shown appreciable utility for industry planning at shire, state, and national scales. There is now an increasing drive from industry for more accurate and cost-effective crop production forecasts. In order to generate production estimates, accurate crop area estimates are needed by the end of the cropping season. Multivariate methods for analysing remotely sensed Enhanced Vegetation Index (EVI) from 16-day Moderate Resolution Imaging Spectroradiometer (MODIS) satellite imagery within the cropping period (i.e. April?November) were investigated to estimate crop area for wheat, barley, chickpea, and total winter cropped area for a case study region in NE Australia. Each pixel classification method was trained on ground truth data collected from the study region. Three approaches to pixel classification were examined: (i) cluster analysis of trajectories of EVI values from consecutive multi-date imagery during the crop growth period; (ii) harmonic analysis of the time series (HANTS) of the EVI values; and (iii) principal component analysis (PCA) of the time series of EVI values. Images classified using these three approaches were compared with each other, and with a classification based on the single MODIS image taken at peak EVI. Imagery for the 2003 and 2004 seasons was used to assess the ability of the methods to determine wheat, barley, chickpea, and total cropped area estimates. The accuracy at pixel scale was determined by the percent correct classification metric by contrasting all pixel scale samples with independent pixel observations. At a shire level, aggregated total crop area estimates were compared with surveyed estimates. All multi-temporal methods showed significant overall capability to estimate total winter crop area. There was high accuracy at pixel scale (>98% correct classification) for identifying overall winter cropping. However, discrimination among crops was less accurate. Although the use of single-date EVI data produced high accuracy for estimates of wheat area at shire scale, the result contradicted the poor pixel-scale accuracy associated with this approach, due to fortuitous compensating errors. Further studies are needed to extrapolate the multi-temporal approaches to other geographical areas and to improve the lead time for deriving cropped-area estimates before harvest.
.
DOI:10.1080/01431161.2010.531783 [本文引用: 1]

Agriculture in Brazilian Amazonia is going through a period of intensification. Crop mapping is important in understanding the way this intensification is occurring and the impact it is having. Two successive classifications based on MODIS (MODerate Resolution Imaging Spectroradiometer)-TERRA/EVI (Enhanced Vegetation Index) time series are applied (1) to map agricultural areas and (2) to identify five crop classes. These classes represent agricultural practices involving three commercial crops (soybean, maize and cotton) planted in single or double cropping systems. Both classifications are based on five steps: (1) analysis of the MODIS/EVI time series, (2) application of a smoothing algorithm, (3) application of a feature selection/extraction process to reduce the data set dimensionality, (4) application of a classifier and (5) application of a post-classification treatment. The first classification detected 95% of the agricultural areas (50261702250 ha during the 2006–2007 harvest) and correlation coefficients with agricultural statistics exceeded 0.98 for the three crop classes at municipality level. The second classification (overall accuracy65=6574% and kappa index65=650.675) allowed us to obtain the spatial variability mapping of agricultural practices in the state of Mato Grosso. A total of 30% of the total planted area was cultivated through double cropping systems, especially along the BR163 highway and in the Parecis plateau region.
DOI:10.1016/j.rse.2010.12.017URL [本文引用: 1]

78 We demonstrate if spectral information alone is practical in urban classification. 78 We develop multiscale object-based classification procedures for urban mapping. 78 We discuss the application of various parameters and features for segmentation. 78 We report uncertainties and limitations associated with object-based classifier. 78 We find that the object-based approach is more accurate than per-pixel classifiers.
DOI:10.1016/j.isprsjprs.2013.09.014URLPMID:3945831 [本文引用: 1]

The amount of scientific literature on (Geographic) Object-based Image Analysis – GEOBIA has been and still is sharply increasing. These approaches to analysing imagery have antecedents in earlier research on image segmentation and use GIS-like spatial analysis within classification and feature extraction approaches. This article investigates these development and its implications and asks whether or not this is a new paradigm in remote sensing and Geographic Information Science (GIScience). We first discuss several limitations of prevailing per-pixel methods when applied to high resolution images. Then we explore the paradigm concept developed by Kuhn (1962) and discuss whether GEOBIA can be regarded as a paradigm according to this definition. We crystallize core concepts of GEOBIA, including the role of objects, of ontologies and the multiplicity of scales and we discuss how these conceptual developments support important methods in remote sensing such as change detection and accuracy assessment. The ramifications of the different theoretical foundations between the ‘per-pixel paradigm’ and GEOBIA are analysed, as are some of the challenges along this path from pixels, to objects, to geo-intelligence. Based on several paradigm indications as defined by Kuhn and based on an analysis of peer-reviewed scientific literature we conclude that GEOBIA is a new and evolving paradigm.
DOI:10.1016/j.isprsjprs.2017.06.001URL [本文引用: 1]

Object-based image classification for land-cover mapping purposes using remote-sensing imagery has attracted significant attention in recent years. Numerous studies conducted over the past decade have investigated a broad array of sensors, feature selection, classifiers, and other factors of interest. However, these research results have not yet been synthesized to provide coherent guidance on the effect of different supervised object-based land-cover classification processes. In this study, we first construct a database with 28 fields using qualitative and quantitative information extracted from 254 experimental cases described in 173 scientific papers. Second, the results of the meta-analysis are reported, including general characteristics of the studies (e.g., the geographic range of relevant institutes, preferred journals) and the relationships between factors of interest (e.g., spatial resolution and study area or optimal segmentation scale, accuracy and number of targeted classes), especially with respect to the classification accuracy of different sensors, segmentation scale, training set size, supervised classifiers, and land-cover types. Third, useful data on supervised object-based image classification are determined from the meta-analysis. For example, we find that supervised object-based classification is currently experiencing rapid advances, while development of the fuzzy technique is limited in the object-based framework. Furthermore, spatial resolution correlates with the optimal segmentation scale and study area, and Random Forest (RF) shows the best performance in object-based classification. The area-based accuracy assessment method can obtain stable classification performance, and indicates a strong correlation between accuracy and training set size, while the accuracy of the point-based method is likely to be unstable due to mixed objects. In addition, the overall accuracy benefits from higher spatial resolution images (e.g., unmanned aerial vehicle) or agricultural sites where it also correlates with the number of targeted classes. More than 95.6% of studies involve an area less than 300 ha, and the spatial resolution of images is predominantly between 0 and 2 m. Furthermore, we identify some methods that may advance supervised object-based image classification. For example, deep learning and type-2 fuzzy techniques may further improve classification accuracy. Lastly, scientists are strongly encouraged to report results of uncertainty studies to further explore the effects of varied factors on supervised object-based image classification.
DOI:10.1080/01431161.2014.943325URL [本文引用: 1]

Crop type identification is the basis of crop acreage estimation and plays a key role in crop production prediction and food security analysis. However, the accuracy of crop type identification using remote-sensing data needs to be improved to support operational agriculture-monitoring tasks. In this paper, a new method integrating high-spatial resolution multispectral data with features extracted from coarse-resolution time-series vegetation index data is proposed to improve crop type identification accuracy in Hungary. Four crop growth features, including peak value, date of peak occurrence, average rate of green-up, and average rate for the senescence period were extracted from time-series Moderate Resolution Imaging Spectroradiometer (MODIS) normalized difference vegetation index (NDVI) profiles and spatially enhanced to 30 m resolution using resolution merge tools based on a multiplicative method to match the spatial resolution of Landsat Thematic Mapper (TM) data. A maximum likelihood classifier (MLC) was used to classify the TM and merged images. Independent validation results indicated that the average overall classification accuracy was improved from 92.38% using TM to 94.67% using the merged images. Based on the classification results using the proposed method, acreages of two major summer crops were estimated and compared to statistical data provided by the United States Department of Agriculture (USDA). The proposed method was able to achieve highly satisfactory crop type identification results.
DOI:10.1016/j.isprsjprs.2009.06.004URL [本文引用: 1]

Remote sensing imagery needs to be converted into tangible information which can be utilised in conjunction with other data sets, often within widely used Geographic Information Systems (GIS). As long as pixel sizes remained typically coarser than, or at the best, similar in size to the objects of interest, emphasis was placed on per-pixel analysis, or even sub-pixel analysis for this conversion, but with increasing spatial resolutions alternative paths have been followed, aimed at deriving objects that are made up of several pixels. This paper gives an overview of the development of object based methods, which aim to delineate readily usable objects from imagery while at the same time combining image processing and GIS functionalities in order to utilize spectral and contextual information in an integrative way. The most common approach used for building objects is image segmentation, which dates back to the 1970s. Around the year 2000 GIS and image processing started to grow together rapidly through object based image analysis (OBIA - or GEOBIA for geospatial object based image analysis). In contrast to typical Landsat resolutions, high resolution images support several scales within their images. Through a comprehensive literature review several thousand abstracts have been screened, and more than 820 OBIA-related articles comprising 145 journal papers, 84 book chapters and nearly 600 conference papers, are analysed in detail. It becomes evident that the first years of the OBIA/GEOBIA developments were characterised by the dominance of rey literature, but that the number of peer-reviewed journal articles has increased sharply over the last four to five years. The pixel paradigm is beginning to show cracks and the OBIA methods are making considerable progress towards a spatially explicit information extraction workflow, such as is required for spatial planning as well as for many monitoring programmes.
[本文引用: 1]
[本文引用: 1]
DOI:10.3233/FI-2000-411207URL [本文引用: 1]

The watershed transform is the method of choice for image segmentation in the field of mathematical morphology. We present a critical review of several definitions of the watershed transform and the associated sequential algorithms, and discuss various issues which often cause confusion in the literature. The need to distinguish between definition, algorithm specification and algorithm implementation is pointed out. Various examples are given which illustrate differences between watershed transforms based on different definitions and/or implementations. The second part of the paper surveys approaches for parallel implementation of sequential watershed algorithms.
DOI:10.1006/cviu.1999.0822URL [本文引用: 1]

In recent years, the watershed line has emerged as the primary tool of mathematical morphology for image segmentation. Several very efficient algorithms have been devised for the determination of watersheds. Nevertheless, the application of watershed algorithms to an image is often disappointing: the image is oversegmented into a large number of tiny, shallow watersheds, where one wanted to obtain only a few deep ones. This paper presents a novel approach to watershed merging. Mainly, it addresses the following question: given an image, what is the closest image that has a simpler watershed structure? The basic idea is to replicate the process of watershed merging that takes place when rain falls over a real landscape: smaller watersheds progressively fill until an overflow occurs. The water then flows to a nearby, larger or deeper watershed, in which the overflown watersheds are merged. The methods presented in this paper apply the minimum extensive modifications possible to a given image to obtain a new one that has many fewer watersheds but is still “close” to the original. Their usefulness is demonstrated for several biomedical applications.
DOI:10.1016/j.rse.2011.01.009URL [本文引用: 1]

78 Decision tree modeling is suitable to identify crops at different field conditions. 78 Consideration of intra-class variations is required to improve classifications. 78 Textural features improve discrimination among heterogeneous permanent crops. 78 Information from NIR and SWIR bands is needed for detailed crop identification. 78 Crop identification requires the study of field status in distinct growing seasons.
DOI:10.3390/rs70100512URL [本文引用: 1]

Since the 2000s, bioenergy land use has been rapidly expanded in U.S. agricultural lands. Monitoring this change with limited acquisition of remote sensing imagery is difficult because of the similar spectral properties of crops. While phenology-assisted crop mapping is promising, relying on frequently observed images, the accuracies are often low, with mixed pixels in coarse-resolution imagery. In this paper, we used the eight-day, 500 m MODIS products (MOD09A1) to test the feasibility of crop unmixing in the U.S. Midwest, an important bioenergy land use region. With all MODIS images acquired in 2007, the 46-point Normalized Difference Vegetation Index (NDVI) time series was extracted in the study region. Assuming the phenological pattern at a pixel is a linear mixture of all crops in this pixel, a spatially constrained phenological mixture analysis (SPMA) was performed to extract crop percent covers with endmembers selected in a dynamic local neighborhood. The SPMA results matched well with the USDA crop data layers (CDL) at pixel level and the Crop Census records at county level. This study revealed more spatial details of energy crops that could better assist bioenergy decision-making in the Midwest.
DOI:10.1080/01431161.2010.527397URL [本文引用: 1]

An accurate and timely crop-type map is essential in water planning in California. So far, no effort has been made to effectively and efficiently identify specific crop types on an annual basis in this area. We have explored the potential of Moderate Resolution Imaging Spectroradiometer (MODIS) reflectance images to annually map major crop types in the San Joaquin Valley, California. A phenology-based classification approach has been employed, which has extracted phenological metrics from normalized difference vegetation index (NDVI) profiles and identified crop types based on these metrics using decision trees. According to a comparison with traditional maximum-likelihood classification, this phenology-based approach has shown great advantages when the size of the training set was limited by ground-truth availability and when the central tendency was absent in agricultural systems heavily influenced by human activities.
DOI:10.1016/j.isprsjprs.2014.09.008URL [本文引用: 1]

Many public and private decisions rely on geospatial information stored in a GIS database. For good decision making this information has to be complete, consistent, accurate and up-to-date. In this paper we introduce a new approach for the semi-automatic verification of a specific part of the, possibly outdated GIS database, namely cropland and grassland objects, using mono-temporal very high resolution (VHR) multispectral satellite images. The approach consists of two steps: first, a supervised pixel-based classification based on a Markov Random Field is employed to extract image regions which contain agricultural areas (without distinction between cropland and grassland), and these regions are intersected with boundaries of the agricultural objects from the GIS database. Subsequently, GIS objects labelled as cropland or grassland in the database and showing agricultural areas in the image are subdivided into different homogeneous regions by means of image segmentation, followed by a classification of these segments into either cropland or grassland using a Support Vector Machine. The classification result of all segments belonging to one GIS object are finally merged and compared with the GIS database label. The developed approach was tested on a number of images. The evaluation shows that errors in the GIS database can be significantly reduced while also speeding up the whole verification task when compared to a manual process.
DOI:10.1016/j.isprsjprs.2015.03.004URL [本文引用: 1]

Agricultural management increasingly uses crop maps based on classification of remotely sensed data. However, classification errors can translate to errors in model outputs, for instance agricultural production monitoring (yield, water demand) or crop acreage calculation. Hence, knowledge on the spatial variability of the classier performance is important information for the user. But this is not provided by traditional assessments of accuracy, which are based on the confusion matrix. In this study, classification uncertainty was analyzed, based on the support vector machines (SVM) algorithm. SVM was applied to multi-spectral time series data of RapidEye from different agricultural landscapes and years. Entropy was calculated as a measure of classification uncertainty, based on the per-object class membership estimations from the SVM algorithm. Permuting all possible combinations of available images allowed investigating the impact of the image acquisition frequency and timing, respectively, on the classification uncertainty. Results show that multi-temporal datasets decrease classification uncertainty for different crops compared to single data sets, but there was no ne-image-combination-fits-all solution. The number and acquisition timing of the images, for which a decrease in uncertainty could be realized, proved to be specific to a given landscape, and for each crop they differed across different landscapes. For some crops, an increase of uncertainty was observed when increasing the quantity of images, even if classification accuracy was improved. Random forest regression was employed to investigate the impact of different explanatory variables on the observed spatial pattern of classification uncertainty. It was strongly influenced by factors related with the agricultural management and training sample density. Lower uncertainties were revealed for fields close to rivers or irrigation canals. This study demonstrates that classification uncertainty estimates by the SVM algorithm provide a valuable addition to traditional accuracy assessments. This allows analyzing spatial variations of the classifier performance in maps and also differences in classification uncertainty within the growing season and between crop types, respectively.
DOI:10.3390/rs71215820URL [本文引用: 1]

Cropland mapping via remote sensing can provide crucial information for agri-ecological studies. Time series of remote sensing imagery is particularly useful for agricultural land classification. This study investigated the synergistic use of feature selection, Object-Based Image Analysis (OBIA) segmentation and decision tree classification for cropland mapping using a finer temporal-resolution Landsat-MODIS Enhanced time series in 2007. The enhanced time series extracted 26 layers of Normalized Difference Vegetation Index (NDVI) and five NDVI Time Series Indices (TSI) in a subset of agricultural land of Southwest Missouri. A feature selection procedure using the Stepwise Discriminant Analysis (SDA) was performed, and 10 optimal features were selected as input data for OBIA segmentation, with an optimal scale parameter obtained by quantification assessment of topological and geometric object differences. Using the segmented metrics in a decision tree classifier, an overall classification accuracy of 90.87% was achieved. Our study highlights the advantage of OBIA segmentation and classification in reducing noise from in-field heterogeneity and spectral variation. The crop classification map produced at 30 m resolution provides spatial distributions of annual and perennial crops, which are valuable for agricultural monitoring and environmental assessment studies.
DOI:10.1016/j.rse.2011.11.020URL [本文引用: 1]

78 Pixel-based (PB) and object-based (OB) classifications are compared. 78 Three machine learning algorithms (MLAs) are examined. 78 No statistical difference between PB and OB classifications was found. 78 For OB classifications, significant differences between MLAs were found.
DOI:10.1016/j.isprsjprs.2014.12.026URL [本文引用: 1]

Unmanned Aerial Vehicle (UAV) has been used increasingly for natural resource applications in recent years due to their greater availability and the miniaturization of sensors. In addition, Geographic Object-Based Image Analysis (GEOBIA) has received more attention as a novel paradigm for remote sensing earth observation data. However, GEOBIA generates some new problems compared with pixel-based methods. In this study, we developed a strategy for the semi-automatic optimization of object-based classification, which involves an area-based accuracy assessment that analyzes the relationship between scale and the training set size. We found that the Overall Accuracy (OA) increased as the training set ratio (proportion of the segmented objects used for training) increased when the Segmentation Scale Parameter (SSP) was fixed. The OA increased more slowly as the training set ratio became larger and a similar rule was obtained according to the pixel-based image analysis. The OA decreased as the SSP increased when the training set ratio was fixed. Consequently, the SSP should not be too large during classification using a small training set ratio. By contrast, a large training set ratio is required if classification is performed using a high SSP. In addition, we suggest that the optimal SSP for each class has a high positive correlation with the mean area obtained by manual interpretation, which can be summarized by a linear correlation equation. We expect that these results will be applicable to UAV imagery classification to determine the optimal SSP for each class.
DOI:10.1016/j.jag.2011.05.011URL [本文引用: 1]

The availability of numerous spectral, spatial, and contextual features with object-based image analysis (OBIA) renders the selection of optimal features a time consuming and subjective process. While several feature selection methods have been used in conjunction with OBIA, a robust comparison of the utility and efficiency of approaches would facilitate broader and more effective implementation. In this study, we evaluated three feature selection methods, (1) Jeffreys鈥揗atusita distance (JM), (2) classification tree analysis (CTA), and (3) feature space optimization (FSO) for object-based vegetation classifications with sub-decimeter digital aerial imagery in arid rangelands of the southwestern U.S. We assessed strengths, weaknesses, and best uses for each method using the criteria of ease of use, ability to rank and/or reduce input features, and classification accuracies. For the five sites tested, JM resulted in the highest overall classification accuracies for three sites, while CTA yielded highest accuracies for two sites. FSO resulted in the lowest accuracies. CTA offered ease of use and ability to rank and reduce features, while JM had the advantage of assessing class separation distances. FSO allowed for determining features relatively quickly, because it operates within the OBIA software used in this analysis (eCognition). However, the feature ranking in FSO is not transparent and accuracies were relatively low. While all methods offered an objective approach for determining suitable features for classifications of sub-decimeter resolution aerial imagery, we concluded that CTA was best suited for this particular application. We explore the limitations, assumptions, and appropriate uses for this and other datasets.