
1 南京大学心理系, 南京 210023
2 安徽工业大学工商管理系, 马鞍山 243032
3 中国科学院数学与系统科学研究院, 北京 100190
收稿日期:
2018-10-29出版日期:
2019-11-25发布日期:
2019-08-19通讯作者:
周仁来E-mail:rlzhou@nju.edu.cn基金资助:
* 中央高校基本科研业务费专项资金()(14370303);江苏省普通高校学术学位研究生科研创新计划项目(KYZZ16_0010);安徽省高校人文科学研究项目资助(SK2017A0084)Classification of test-anxious individuals using Event-Related Potentials (ERPs): The effectiveness of machine learning algorithms
ZHANG Wenpei1,2, SHEN Qunlun3, SONG Jintao1, ZHOU Renlai1(
1 Department of Psychology, Nanjing University, Nanjing, 210023, China
2 Department of Business Administration, School of Business, Anhui University of Technology, Maanshan, 243032, China
3 Academy of Mathematics and Systems Science, Chinese Academy of Sciences, 100190, China
Received:
2018-10-29Online:
2019-11-25Published:
2019-08-19Contact:
ZHOU Renlai E-mail:rlzhou@nju.edu.cn摘要/Abstract
摘要: 考试焦虑对个体的身心具有严重危害。传统诊断考试焦虑的方法容易受到个体主观态度的影响, 从而影响对个体考试焦虑的发现与及早干预。为了克服传统主观问卷对考试焦虑群体诊断的不足, 本研究提出脑电神经数据结合机器学习的客观综合诊断方法评估个体的考试焦虑水平。研究采用情绪Stroop范式, 结合脑电技术测量个体对考试焦虑者的注意抑制功能, 机器学习基于此前提, 提取P1, P2, N2, P3和LPP五种事件相关电位(ERP)成分, 以卷积神经网络(CNN)为主采用7种常见的机器学习算法对个体考试焦虑程度进行进一步的诊断。结果表明CNN对考试焦虑诊断的准确率达86.5%, F1-score为0.911, 显著高于其他6种常见算法。因此采用CNN对脑电信号进行深度学习得出的诊断模型能够有效地对个体的考试焦虑程度进行诊断。
图/表 7
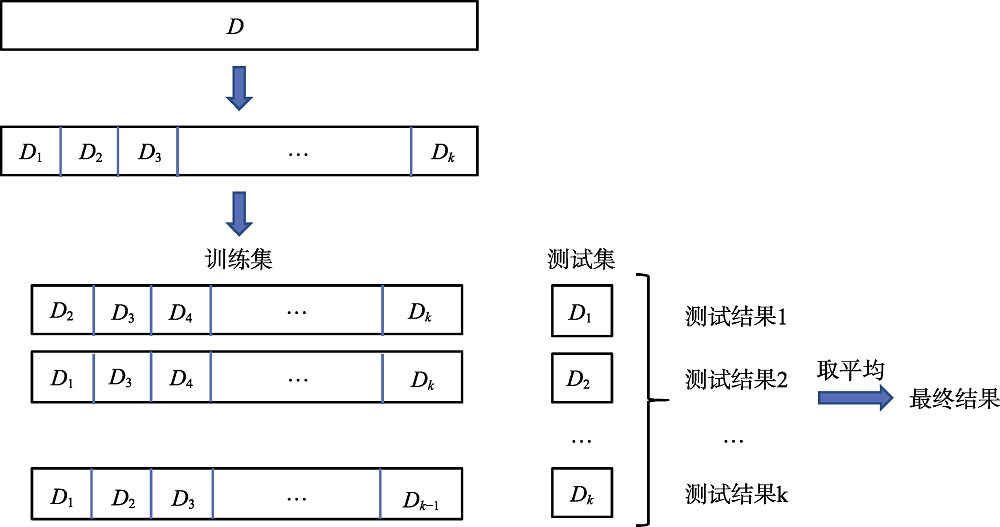
图1图中的D表示原始数据集, D1,D2,…Dk表示将D分成的k个相同大小的子集
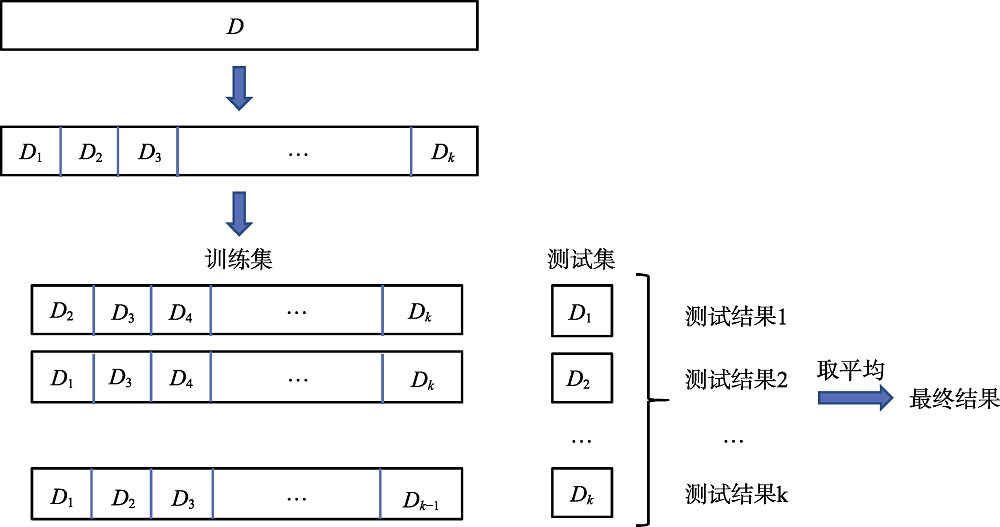

图2卷积操作的计算展示 注:这里的卷积是不进行补全的卷积, 即卷积运算之后数据矩阵会相应变小, 同时也有一种补全的卷积操作, 即在原数据矩阵周围添0, 使得卷积之后得到的数据矩阵大小不变。


图3最大池化的计算展示 注:图中表示的是一个4×4的矩阵上使用一个2×2的窗口以步长为2进行最大池化, 其原理就是取出每个2×2窗口中的的最大元素作为输出矩阵中对应元素的值。

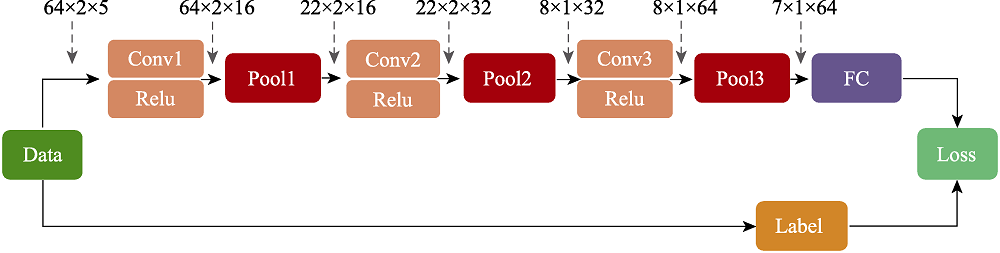
图4本研究使用的卷积神经网络架构图 注:每一层的具体参数见表1。横线上的数据表示这一层的输入数据的维度, 也即上一层输出数据的维度。Conv代表卷积操作, Pool代表池化操作, relu代表在卷积操作之后的非线性激活方法。
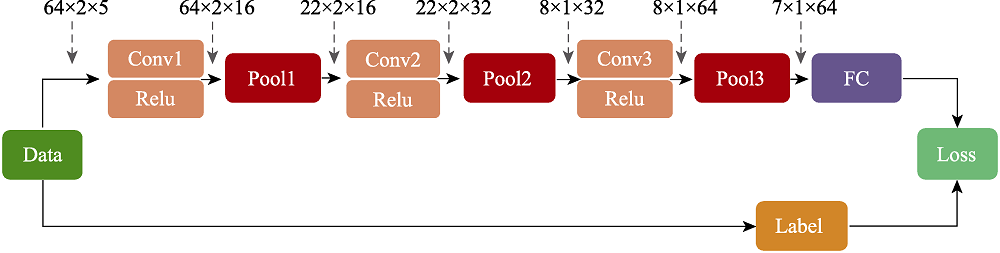
表1卷积神经网络架构
层数 | 层类型 | 卷积核(神经元)个数 | 卷积核大小 | 步长 | 滑动窗口大小 |
---|---|---|---|---|---|
1 | 卷积 | 16 | 5×1 | [1, 1] | / |
2 | 最大池化 | / | / | [3, 1] | [4, 1] |
3 | 卷积 | 32 | 3×1 | [1, 1] | / |
4 | 最大池化 | / | / | [4, 2] | [3, 2] |
5 | 卷积 | 64 | 3×1 | [1, 1] | / |
6 | 平均池化 | / | / | [1, 1] | [2, 1] |
7 | 全连接 | 2 | / | / | / |
表1卷积神经网络架构
层数 | 层类型 | 卷积核(神经元)个数 | 卷积核大小 | 步长 | 滑动窗口大小 |
---|---|---|---|---|---|
1 | 卷积 | 16 | 5×1 | [1, 1] | / |
2 | 最大池化 | / | / | [3, 1] | [4, 1] |
3 | 卷积 | 32 | 3×1 | [1, 1] | / |
4 | 最大池化 | / | / | [4, 2] | [3, 2] |
5 | 卷积 | 64 | 3×1 | [1, 1] | / |
6 | 平均池化 | / | / | [1, 1] | [2, 1] |
7 | 全连接 | 2 | / | / | / |
表2不同机器学习模型的结果对比
机器学习模型 | 准确率 | 查准率 | 查全率 | F1-score |
---|---|---|---|---|
卷积神经网络(CNN) | 86.5% | 84.0% | 100% | 0.911 |
逻辑回归(Logistic Regression) | 80.3% | 83.6% | 91.4% | 0.868 |
K近邻(KNN) | 71.8% | 71.3% | 100.0% | 0.817 |
支持向量机(SVM) | 79.0% | 78.6% | 96.4% | 0.865 |
随机森林(Random Forest) | 73.1% | 78.7% | 84.2% | 0.814 |
人工神经网络(ANN) | 82.7% | 84.6% | 92.9% | 0.882 |
循环神经网络(RNN) | 79.2% | 77.0% | 100% | 0.870 |
表2不同机器学习模型的结果对比
机器学习模型 | 准确率 | 查准率 | 查全率 | F1-score |
---|---|---|---|---|
卷积神经网络(CNN) | 86.5% | 84.0% | 100% | 0.911 |
逻辑回归(Logistic Regression) | 80.3% | 83.6% | 91.4% | 0.868 |
K近邻(KNN) | 71.8% | 71.3% | 100.0% | 0.817 |
支持向量机(SVM) | 79.0% | 78.6% | 96.4% | 0.865 |
随机森林(Random Forest) | 73.1% | 78.7% | 84.2% | 0.814 |
人工神经网络(ANN) | 82.7% | 84.6% | 92.9% | 0.882 |
循环神经网络(RNN) | 79.2% | 77.0% | 100% | 0.870 |

图5情绪Stroop任务的ERP波形图 注:情绪Stroop任务中高、低考试焦虑在两种条件(中性词和考试相关威胁词)下的ERP总平均波形图(以Fz, FCz, Cz, CPz和Pz电极点为例)。

参考文献 63
[1] | Albert J., López-Martín S., & Carretié L. ( 2010). Emotional context modulates response inhibition: Neural and behavioral data. NeuroImage, 49( 1), 914-921. |
[2] | Berggren, N., & Derakshan, N. ( 2013). Attentional control deficits in trait anxiety: why you see them and why you don’t. Biological Psychology, 92( 3), 440-446. |
[3] | Boshra R., Ruiter K., Reilly J., & Connolly J. ( 2016). Machine learning based framework for EEG/ERP analysis. International Journal of Psychophysiology, 108, 105. |
[4] | Boureau Y-L., Bach F., LeCun Y., & Ponce J. ( 2010, June). Learning mid-level features for recognition. In 2010 IEEE Computer Society Conference on Computer Vision and Pattern Recognition( pp. 2559-2566). IEEE. |
[5] | Cecotti H., Rivet B., Congedo M., Jutten C., Bertrand O., Maby E., & Mattout J. ( 2011). A robust sensor-selection method for P300 brain-computer interfaces. Journal of Neural Engineering, 8( 1), 016001. |
[6] | Chen R., Liu X. N., & Zhou R. L . ( 2011). The attentional bias to threat stimuli in test-anxious students. Journal of Psychological Science, 34( 1), 151-154. |
[ 陈睿, 刘潇楠, 周仁来 . ( 2011). 不同程度考试焦虑个体对威胁性刺激注意机制的差异. 心理科学, 34( 1), 151-154.] | |
[7] | Chen, R., & Zhou, R. ( 2010). Attentional disengage from test-related pictures in test-anxious students: Evidence from event-related potentials. International Conference on Brain Informatics, 6334, 232-239. |
[8] | Chen, Z. Y . ( 2002). Fear of negative evaluation and test anxiety in middle school students. Chinese Mental Health Journal, 16( 12), 855-857. |
[ 陈祉妍 . ( 2002). 中学生负面评价恐惧与考试焦虑的相关性. 中国心理卫生杂志, 16( 12), 855-857.] | |
[9] | Cosme, D., & Wiens, S. ( 2015). Self-reported trait mindfulness and affective reactivity: A motivational approach using multiple psychophysiological measurese. PLoS ONE, 10( 3), e0119466. |
[10] | Dem?ar, J. ( 2006). Statistical comparisons of classifiers over multiple data sets. Journal of Machine Learning Research, 7, 1-30. |
[11] | Dennis, T. A., & Chen, B. ( 2009). Trait anxiety and conflict monitoring following threat: An ERP study. Psychophysiology, 46( 1), 122-131. |
[12] | Derakshan N., Smyth S., & Eysenck M. W. ( 2009). Effects of state anxiety on performance using a task-switching paradigm: An investigation of attentional control theory. Psychonomic Bulletin & Review, 16( 6), 1112-1117. |
[13] | Diegomantecón, J. ( 2015). Instrument adaptation in cross-cultural studies of students’ mathematics-related beliefs: Learning from healthcare research. Compare: A Journal of Comparative and International Education, 45( 4), 545-567. |
[14] | Donaldson K. R., Ait Oumeziane B., Hélie S., & Foti D. ( 2016). The temporal dynamics of reversal learning: P3 amplitude predicts valence-specific behavioral adjustment. Physiology and Behavior, 161, 24-32. |
[15] | Edwards M. S., Burt J. S., & Lipp O. V. ( 2010). Selective attention for masked and unmasked threatening words in anxiety: Effects of trait anxiety, state anxiety and awareness. Behaviour Research and Therapy, 48( 3), 210-218. |
[16] | Felmingham K. L., Stewart L. F., Kemp A. H., & Carr A. R. ( 2016). The impact of high trait social anxiety on neural processing of facial emotion expressions in females. Biological Psychology, 117, 179-186. |
[17] | Feng, X. ( 2003). Result representation and method application: Analysis of 141 investigations. Sociological Research, 18( 2), 28-38. |
[ 风笑天 . ( 2003). 结果呈现与方法运用——141项调查研究的解析. 社会学研究, 18( 2), 28-38.] | |
[18] | Fotin S. V., Yin Y., Haldankar H., Hoffmeister J. W., & Periaswamy S. ( 2016, March). Detection of soft tissue densities from digital breast tomosynthesis: comparison of conventional and deep learning approaches. In Medical Imaging 2016: Computer-Aided Diagnosis( Vol. 9785 , p. 97850X). International Society for Optics and Photonics. |
[19] | Gootjes L., Coppens L. C., Zwaan R. A., Franken I. H. A ., & van Strien, J. W. ( 2011). Effects of recent word exposure on emotion-word Stroop interference: An ERP study. International Journal of Psychophysiology, 79( 3), 356-363. |
[20] | Gu R., Lei Z., Broster L., Wu T., Jiang Y., & Luo Y-J. ( 2011). Beyond valence and magnitude: A flexible evaluative coding system in the brain. Neuropsychologia, 49( 14), 3891-3897. |
[21] | Hornik, K. ( 1991). Approximation capabilities of multilayer feedforward networks. Neural Networks, 4( 2), 251-257. |
[22] | Jo H-G., Schmidt S., Inacker E., Markowiak M., & Hinterberger T. ( 2016). Meditation and attention: a controlled study on long-term meditators in behavioral performance and event-related potentials of attentional control. International Journal of Psychophysiology, 99, 33-39. |
[23] | Jung, Y. ( 2018). Multiple predicting K-fold cross-validation for model selection. Journal of Nonparametric Statistics, 30( 1), 197-215. |
[24] | Kalanthroff E., Henik A., Derakshan N., & Usher M. ( 2016). Anxiety, emotional distraction, and attentional control in the Stroop task. Emotion, 16( 3), 293-300. |
[25] | Kanske, P., & Kotz, S. A. ( 2012). Effortful control, depression, and anxiety correlate with the influence of emotion on executive attentional control. Biological psychology, 91( 1), 88-95. |
[26] | Krizhevsky A., Sutskever I., & Hinton G. E. ( 2012). Imagenet classification with deep convolutional neural networks. In Advances in neural information processing systems( pp. 1097-1105). |
[27] | Kumar A., Irsoy O., Ondruska P., Iyyer M., Bradbury J., Gulrajani I., … Com R. ( 2016). Ask me anything: Dynamic memory networks for natural language processing. In International conference on machine learning( pp. 1378-1387). |
[28] | LeCun, Y., & Bengio, Y.( 1995) . Convolutional networks for images, speech, and time-series. The Handbook of Brain Theory and Neural Networks (pp. 255-258). M. A. Arbib, Ed.Cambridge, MA: MIT Press. |
[29] | Lee, A. ( 2015). Comparing deep neural networks and traditional vision algorithms in mobile robotics. Swarthmore University. Retrieved from |
[30] | Lin M., Chen Q., & Yan S. ( 2013). Network in network. arXiv preprint arXiv:1312.4400. Retrieved from |
[31] | Lotz, C., & Sparfeldt, J. R. ( 2017). Does test anxiety increase as the exam draws near? - students’ state test anxiety recorded over the course of one semester. Personality and Individual Differences, 104, 397-400. |
[32] | Lowe P. A., Lee S. W., Witteborg K. M., Prichard K. W., Luhr M. E., Cullinan C. M., .. Janik M. ( 2008). The Test Anxiety Inventory for Children and Adolescents (TAICA) examination of the psychometric properties of a new multidimensional measure of test anxiety among elementary and secondary school students. Journal of Psychoeducational Assessment, 26( 3), 215-230. |
[33] | Lu Y., Jiang H., & Liu W. ( 2017, September). Classification of EEG signal by STFT-CNN framework: identification of right-/left-hand motor imagination in BCI systems. In The 7th International Conference on Computer Engineering and Networks( Vol. 299 , p. 001). |
[34] | Luck S. J., Woodman G. F., & Vogel E. K. ( 2000). Event-related potential studies of attention. Trends in Cognitive Sciences, 4( 11), 432-440. |
[35] | Mahendran, A., & Vedaldi, A. ( 2015). Understanding deep image representations by inverting them. In Proceedings of the IEEE conference on computer vision and pattern recognition( pp. 5188-5196). |
[36] | Mochcovitch M. D., da Rocha Freire, R. C., Garcia R. F., & Nardi A. E. ( 2014). A systematic review of fMRI studies in generalized anxiety disorder: evaluating its neural and cognitive basis. Journal of Affective Disorders, 167, 336-342. |
[37] | Mok, W. S. Y., & Chan, W. W. L. ( 2016). How do tests and summary writing tasks enhance long-term retention of students with different levels of test anxiety? Instructional Science, 44( 6), 567-581. |
[38] | Morel S., George N., Foucher A., Chammat M., & Dubal S. ( 2014). ERP evidence for an early emotional bias towards happy faces in trait anxiety. Biological Psychology, 99( 1), 183-192. |
[39] | Nair, V., & Hinton, G. E. ( 2010). Rectified linear units improve restricted boltzmann machines. In Proceedings of the 27th international conference on machine learning (ICML-10)( pp. 807-814). |
[40] | Newman, E. ( 1996). No more test anxiety: effective steps for taking tests and achieving better grades. Los Angeles: Learning Skills Publications. |
[41] | Peng M., Cai M., & Zhou R. ( 2015). Processing of task-irrelevant emotional faces impacted by implicit sequence learning. NeuroReport, 26( 17), 1056-1060. |
[42] | Putwain D. W., Langdale H. C., Woods K. A., & Nicholson L. J. ( 2011). Developing and piloting a dot-probe measure of attentional bias for test anxiety. Learning and Individual Differences, 21( 4), 478-482. |
[43] | Raz S., Dan O., Arad H., & Zysberg L. ( 2013). Behavioral and neural correlates of emotional intelligence: An event- related potentials (ERP) study. Brain Research, 1526, 44-53. |
[44] | Righi S., Mecacci L., & Viggiano M. P. ( 2009). Anxiety, cognitive self-evaluation and performance: ERP correlates. Journal of Anxiety Disorders, 23( 8), 1132-1138. |
[45] | Sarason, I. G. ( 1978). The test anxiety scale: Concept and research. In Stress and Anxiety (Vol. 5, pp. 193-216). Washington DC: Hemisphere. |
[46] | Schutz P. A., Davis H. A., & Schwanenflugel P. J. ( 2002). Organization of concepts relevant to emotions and their regulation during test taking. The Journal of Experimental Education, 70( 4), 316-342. |
[47] | Seijdel N., Ramakrishnan K., Losch M., & Scholte S. ( 2016). Overlap in performance of CNN’s, human behavior and EEG classification. Journal of Vision, 16( 12), 501. |
[48] | Strier, R., & Shechter, D. ( 2016). Visualizing access: Knowledge development in university-community partnerships. Higher Education, 71( 3), 343-359. |
[49] | Su Y., Hu B., Xu L. X., Zhang X. W., & Chen J . ( 2015). EEG-data-oriented knowledge modeling and emotion recognition. Chinese Science Bulletin (Chinese Version), 60( 11), 1002-1009. |
[ 宿云, 胡斌, 徐立新, 张晓炜, 陈婧 . ( 2015). 面向脑电数据的知识建模和情感识别. 科学通报, 60( 11), 1002-1009.] | |
[50] | Thomas S. J., Johnstone S. J., & Gonsalvez C. J. ( 2007). Event-related potentials during an emotional Stroop task. International Journal of Psychophysiology, 63( 3), 221-231. |
[51] | Tillman, C. M., & Wiens, B. ( 2011). Behavioral and ERP indices of response conflict in Stroop and flanker tasks. Psychophysiology, 48( 10), 1405-1411. |
[52] | van Bockstaele B., Verschuere B., Tibboel H., de Houwer J., Crombez G., & Koster, E. H. W. ( 2014). A review of current evidence for the causal impact of attentional bias on fear and anxiety. Psychological Bulletin, 140( 3), 682-721. |
[53] | Verhaak C. M., Smeenk J. M., van Minnen A., & Kraaimaat F. W. ( 2004). Neuroticism, preattentive and attentional biases towards threat, and anxiety before and after a severe stressor: A prospective study. Personality and Individual Differences, 36( 4), 767-778. |
[54] | Wabnitz P., Martens U., & Neuner F. ( 2016). Written threat: electrophysiological evidence for an attention bias to affective words in social anxiety disorder. Cognition and Emotion, 30( 3), 516-538. |
[55] | Wang, C. K . ( 2001). Reliability and validity of test anxiety scale-Chinese version. Chinese Mental Health Journal, 15( 2), 96-97. |
[ 王才康 . ( 2001). 考试焦虑量表在大学生中的测试报告. 中国心理卫生杂志, 15( 2), 96-97.] | |
[56] | Wang W.-W., Xie X., & Shao F . ( 2008). Early-onset depression and its neural basis. Advances in Psychological Science, 16( 3), 411-417. |
[ 王玮文, 谢希, 邵枫 . ( 2008). 早发性抑郁及其神经基础. 心理科学进展, 16( 3), 411-417.] | |
[57] | Wan, Y-N., & Sun, B-Y. ( 2017). Cigarette craving EEG classification based on convolution neural networks. Computer Systems & Applications, 26( 6), 256-260. |
[ 王艳娜, 孙丙宇 . ( 2017). 基于卷积神经网络的烟瘾渴求脑电分类. 计算机系统应用, 26( 6), 256-260.] | |
[58] | Yan, H., & Lu, L. ( 2014). Effects of exam stress on psychosomatic response saliva immuneglobulin and cortisol among medical college student. Chinese Journal of School Health, 35( 6), 813-816. |
[ 闫慧, 卢莉 . ( 2014). 考试应激对医学生心身反应唾液免疫球蛋白及皮质醇的影响. 中国学校卫生, 35( 6), 813-816.] | |
[59] | Yang Z., Yang D., Dyer C., He X., Smola A., & Hovy E. ( 2016). Hierarchical attention networks for document classification. Proceedings of the 2016 Conference of the North American Chapter of the Association for Computational Linguistics: Human Language Technologies, 1480-1489. |
[60] | Yiend, J. ( 2010). The effects of emotion on attention: A review of attentional processing of emotional information. Cognition and Emotion, 24( 1), 3-47. |
[61] | Yu, F., & Koltun, V. ( 2015). Multi-scale context aggregation by dilated convolutions. arXiv preprint arXiv:1511.07122. Retrieved from |
[62] | Zeidner M. , & Matthews, G.( 2005) . Evaluation anxiety. In A. J. Elliot & C. S. Dweck (Eds.), Handbook ofcompetence and motivation (pp. 141-163). London: Guildford Press. |
[63] | Zeiler, M. D., & Fergus, B. ( 2014, September). Visualizing and understanding convolutional networks. In European conference on computer vision( pp. 818-833). Springer, Cham. |
相关文章 15
[1] | 曹敏, 谢和平, 孙丽君, 张冬静, 孔繁昌, 周宗奎. 网络游戏中化身参照的加工优势:来自行为与ERPs的证据[J]. 心理学报, 2021, 53(6): 639-650. |
[2] | 周文洁, 邓丽群, 丁锦红. 物体颜色对情景记忆的影响[J]. 心理学报, 2021, 53(3): 229-243. |
[3] | 李琎, 孙宇, 杨子鹿, 钟毅平. 社会价值取向对自我社会奖赏加工的影响——来自ERPs的证据[J]. 心理学报, 2020, 52(6): 786-800. |
[4] | 孙鑫, 黎坚, 符植煜. 利用游戏log-file预测学生推理能力和数学成绩——机器学习的应用[J]. 心理学报, 2018, 50(7): 761-770. |
[5] | 焦江丽, 刘毅, 闻素霞. 不同熟练类型双语者情绪Stroop中的自动化情绪通达[J]. 心理学报, 2017, 49(9): 1150-1157. |
[6] | 杨海波;赵欣;汪洋;张磊;王瑞萌; 张毅;王力. PTSD青少年执行功能缺陷的情绪特异性[J]. 心理学报, 2017, 49(5): 643-652. |
[7] | 赵思敏;吴岩;李天虹;郭庆童. 词汇识别中歧义词素语义加工:ERP研究[J]. 心理学报, 2017, 49(3): 296-306. |
[8] | 范伟;钟毅平;杨子鹿;李琎;欧阳益; 蔡荣华; 李慧云 ;傅小兰 . 外倾个体的自我参照加工程度效应[J]. 心理学报, 2016, 48(8): 1002-1012. |
[9] | 吴岩;莫德圆;王海英; 于溢洋;陈烜之;张明. 语义分类任务中部件位置在汉字识别中的作用[J]. 心理学报, 2016, 48(6): 599-606. |
[10] | 王协顺;吴岩;赵思敏;倪超;张明. 形旁和声旁在形声字识别中的作用[J]. 心理学报, 2016, 48(2): 130-140. |
[11] | 李婧; 陈安涛;陈杰;龙长权. 词语型类别属性归纳中分类与属性推理过程的时间特征[J]. 心理学报, 2016, 48(11): 1410-1422. |
[12] | 岳鹏飞;杜婉婉;白学军;许远理. 情绪标注对情绪的抑制发生在何时:一项ERPs研究[J]. 心理学报, 2015, 47(9): 1124-1132. |
[13] | 吴岩;王协顺;陈烜之. 汉字识别中部件结合率的作用:ERP研究[J]. 心理学报, 2015, 47(2): 157-166. |
[14] | 白学军;刘湍丽;沈德立. 部分线索效应的认知抑制过程:情绪Stroop任务证据[J]. 心理学报, 2014, 46(2): 143-155. |
[15] | 于丽霞;凌霄;江光荣. 自伤青少年的冲动性[J]. 心理学报, 2013, 45(3): 320-335. |
PDF全文下载地址:
http://journal.psych.ac.cn/xlxb/CN/article/downloadArticleFile.do?attachType=PDF&id=4530