
2Department of Physics,
3Department of Mathematics,
Received:2020-04-22Revised:2020-08-04Accepted:2020-08-04Online:2020-11-12

Abstract
Keywords:
PDF (5434KB)MetadataMetricsRelated articlesExportEndNote|Ris|BibtexFavorite
Cite this article
Najeeb Alam Khan, Muhammad Ali Qureshi, Tooba Hameed, Saeed Akbar, Saif Ullah. Behavioral effects of a four-wing attractor with circuit realization: a cryptographic perspective on immersion. Communications in Theoretical Physics, 2020, 72(12): 125004- doi:10.1088/1572-9494/abb7d1
1. Introduction
The modern era has seen many great contributions, both theoretical and experimental, on the subject of fractional calculus, with its long history of non-integer order [1]. Some well-known types of fractional derivatives (FDs) include those proposed by Liouville, Riezs, Riemann, Caputo, Letnikov, Hadamard, Marchaud, Weyl, and Coimbra, among others. The role of FDs in science is to store information about a system's temporal and spatial non-locality, where non-locality is useful for describing an object's instant state variation spatially; with respect to time, this is known as memory [2]. The key representative property claimed for the FD is that it violates the standard Leibniz rule [3]; this property, while necessary, is not sufficient. The standard Leibniz rule is violated by various integro-differential operators, but it is important to note that these are not derivatives of a non-integer order. As mentioned above, spatial and temporal non-locality are new tools for defining systems, and the process is considered to be one of the most significant properties of FDs. The property of non-locality, in mathematical terms, states that a FD cannot be embodied as a finite set of derivatives of integer orders. Thus, based on the non-locality principle, we cannot describe non-local systems and processes containing differential equations only in terms of the derivatives of integer order [4].The chaotic system is an initial condition-sensitive and nonlinear dynamical system which is affected even by small variations, and was first introduced by Lorenz in 1963 in the context of a study of weather models, which constitute a two-scroll chaotic attractor [5]. After Lorenz's discovery of the chaotic system, the theory has been utilized in many different fields of science, including physics [6, 7], reactors [8], memristors [9], and encryption [10]. Chaotic attractors are classified into two categories: (i) self-excited attractors, and (ii) hidden attractors. Self-excited attractors possess a basin of attraction, created by to the intersection of unstable equilibrium points, and hidden attractors do not intersect with the neighborhood of equilibrium points.
In engineering applications, more complicated topological nonlinear periodic [11, 12] and chaotic [13] systems are widely employed in systems such as multi-wing attractors or multi-scrolls [14, 15]. For scientists, they therefore represent an attractive field of research. Multi-scroll systems can basically be categorized into into two types: the generalization of Chua's circuit [16], and the generalization of the Lorenz circuit [17]. The autonomous four-wing butterfly was proposed by Elwakil [18] having both symmetrical and non-symmetrical versions. Zidan et al then proposed a new V-shaped multi-scroll attractor [19]. Zhang [20] proposed a four-dimensional hyperchaotic system by modifying Lu's double-wing hyperchaotic system. Based on the saw tooth wave function, the Lorentz system was modified by Simin et al for generating multi-wing butterfly chaotic attractors [21]. Utilizing Lu's system, a new piece-wise linear function was considered, in order to generate a multi-wing butterfly chaotic system. The four-wing system achieved by Wang [22] was derived from previous 3D four-wing smooth continuous chaotic systems [23], and enhanced by extending these chaotic systems by adding linear or quadratic terms to the system. Research is ongoing in relation to methodology, theory, and application in multi-wing chaotic system, and great progress is being made [24]. Recently, Wang and Volos [25] initiated a simple three-dimensional system, and discussed the special features required for system validation. In this work, we extend Wang's model by taking the memory term via the time fractional derivative in the following form:
The public network of communication systems has rapidly become digitized over the past few years, as cross-country communication issues, such as transportation problems, speed of communication, confidentiality, etc, have arisen. The intelligence community is familiar with these issues, and protects our information using different mathematical methods, such as stenography and cryptography. Cryptography is an art among sciences, in that it creates meaningless redundancy around sensitive data as random entropy increases, so that data compression becomes more difficult for the would-be infiltrator. The multi-wing attractor, along with the fractional order mathematical system scheme, represents the process of evolution in the field of cryptography, in terms of the generation of random numbers, tested with NIST-800-22, and of complicated encryption processes, driven by a need for the secure communication of sensitive data.
The aim of this work is to present a multi-wing attractor using sine-hyperbolic nonlinearity combined with fractional order. The mathematical model, and other dynamic parameters, are calculated, and discussed in detail in the following sections, along with the electronic realization simulated in MultiSIM for different values of fractional order, using passive components such as Resistors, Capacitors, and Diodes, and active components, i.e. operational amplifiers. We conclude by investigating the cryptographic capability of our multi-wing chaotic system, using the Python programming language, to perform encryption for voice, image, and animation image data for cellular and telecommunication applications.
2. Preliminary definitions
In this section, the preliminary definitions used in this paper as tools for guidance, together with their properties, are elucidated.Assume $\theta \left(t\right)$ to be a continuous function ($\theta \left(t\right)\,\in {C}^{m}\left[0,1\right]$); the FD, in the Caputo sense of $\theta \left(t\right)$, with respect to $t$, is then defined as [1]:
The Laplace transform of the FD gives the following expression, as defined in [2]:
Finally, Ren et al [26] have derived an equivalent expression for ${D}_{t}^{\alpha }\theta \left(t\right)$, using the properties of the LT such that
3. Model of the fractional order four-wing attractor, and its properties
The system (1)-(2) is studied in this section, in order to explore the dynamical fluctuations around the real distinct values of $\alpha .$ Behavioral effects are also observed for distinct values of $a$ in system (1), to as to observe chaotic behavior with limit cycles and a multi-wing attractor. Firstly, we use the property of definition 2.2; equation (Next, we study the dynamical properties of physical system (8), which leads us to system (1).
3.1. Existence and uniqueness
The system of equation (Let (${u}_{xi}^{* },{u}_{yi}^{* },{u}_{zi}^{* }$) in ${\Re }^{3}$ be the equilibrium points of system (8), which can readily be obtained by substituting ${\dot{u}}_{1}\left(t\right)={\dot{u}}_{2}\left(t\right)={\dot{u}}_{3}\left(t\right)=0.$ ${P}_{i}={\left[\begin{array}{ccc}{u}_{1i}^{* } & {u}_{2i}^{* } & {u}_{3i}^{* }\end{array}\right]}^{{\rm{T}}}$, i.e. the set of five real equilibrium points at different values of $\alpha .$
The characteristic equation of system (8) at ${P}_{i}$ will be as follows:
$l={\eta }_{1}{u}_{1i}^{* }+\left(1-\alpha +\cosh \,{u}_{2i}^{* }\right){\left({u}_{2i}^{* }\right)}^{2}$ $+\,\alpha {\eta }_{1}\,\cosh \,{u}_{2i}^{* }\,+\alpha {\left({u}_{3i}^{* }\right)}^{2}+2{u}_{1i}^{* }{u}_{2i}^{* }{u}_{3i}^{* }+{\eta }_{2}$ with ${\eta }_{1}=-2-a+3\alpha ,$ ${\eta }_{2}\,=1+a-\alpha ,$ ${\eta }_{3}=-1-a+2\alpha +a\alpha -{\alpha }^{2},$ and ${\eta }_{4}\,=2\alpha +a\alpha -2{\alpha }^{2}.$
With the help of Lemma in [28], the corresponding eigenvalues, ${\lambda }_{1}$ and ${\lambda }_{2,3}$, of system (10) can then be calculated.
The process of two dimensional mapping using the deformed structure of system (8) along with the parameters, from minimum to maximum range, is known as the bifurcation scenario. The mechanism of bifurcation identifies that the structure of systems having different limit cycles or which are multi periodic give rise to either periodic or chaotic attractors, respectively.
The trajectory formed by the $u\left(t\right)\in {\Re }^{3}$ solution of system (8), satisfying the fixed point condition with the phase variable ${u}^{\left(m\right)}$ ($m=1,2,3$) for different indexed variables mapping the folding co-dimension, is known as a Poincaré map.
The well-known Wolf's algorithm [29] is effective in terms of calculating the spectrum of LEs to confirm the existence of strange attractors, i.e. at least one positive LE. The values of the LEs are also relevant in terms of the fractional dimensions of strange attractors named after Kaplan and Yorke [30], using the following equation:
4. Simulation and results
4.1. Mathematical simulation
In this section, we present the numerical results of system (8) for distinct fractional values of $\alpha $ by means of tables and graphs, with the help of Python. Beginning from table 1, this consists of equilibrium points for different values of $\alpha $, obtained by running the numerical simulation using equation (Table 1.
Table 1.Equilibrium point of system (8) at $a=1.5.$
Points | $\alpha =1.00$ | $\alpha =0.99$ | $a=0.92$ | $a=0.90$ |
---|---|---|---|---|
${P}_{0}$ | (0.,0.,0.) | (0.000 66,0.000 98,−0.001 00) | (0.005 02,0.007 36,−0.008 65) | (0.006 18,0.009 02,−0.011 04) |
${P}_{1}$ | (1.126 69,1.224 74,1.379 91) | (1.125 08,1.222 75,1.388 58) | (1.111 26,1.206 51,1.448 63) | (1.106 47,1.201 13,1.465 58) |
${P}_{2}$ | (1.126 69,−1.224 74,−1.379 91) | (1.1248,−1.221 86,−1.389 24) | (1.108 74,−1.199 29,−1.454 02) | (1.103 23,−1.192 07,−1.472 37) |
${P}_{3}$ | (−1.126 69,1.224 74,−1.379 91) | (−1.124 23,1.222 58,−1.389 35) | (−1.104 29,1.2048,−1.454 83) | (−1.097 71,1.198 87,−1.473 34) |
${P}_{4}$ | (−1.126 69,−1.224 74,1.379 91) | (−1.125 95,−1.223 46,1.390 47) | (−1.117 77,−1.211 96,1.4638) | (−1.114 47,−1.207 85,1.484 58) |
New window|CSV
To verify the chaotic dynamics in system (8), topological structures, depicted by means of bifurcation maps, are calculated along the parameters $a,$ and $\alpha .$ In figures 1(a) and (b), these bifurcation maps, along with $a$ versus ${u}_{2}\left(t\right)$, are shown to be $\alpha =1.00$ and 0.99 respectively. It is evident in figures 1(a) and 1(b), that a periodic doubling trace appears when $a$ varies from 1 to 2, showing two distinct routes from $1\leqslant a\leqslant 1.5$ and $a\lt 2.$ The first route gives a clear picture of periodic doubling, but in the second route, the system loses chaotic oscillation. The parameter $a$ can therefore be used to control the degree of chaos. Interestingly, figure 1(c), analyzed in relation to system (8), shows a new bifurcation parameter $\alpha $ via a different route, with tangential and periodic doubling where $0\leqslant \alpha \leqslant 0.85$ and $0.85\lt \alpha \lt 1$, respectively.
Figure 1.
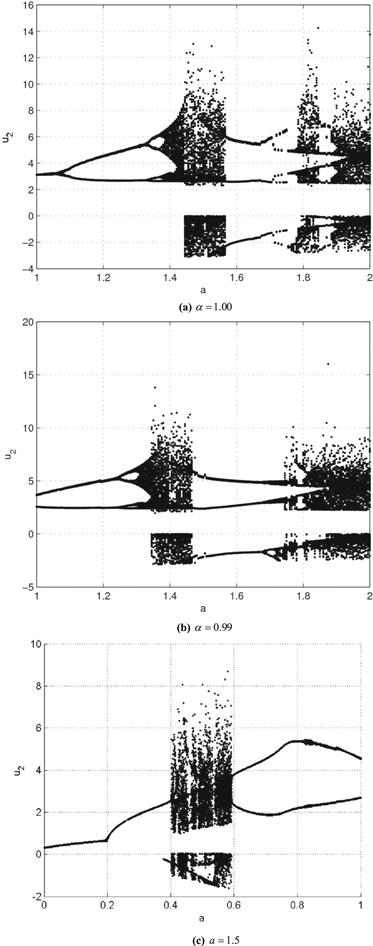
Figure 1.Bifurcation maps of system (8) for different parameters.
The trajectory of system (8) is calculated by means of the Poincaré section for co-dimensions in one phase space, as displayed in figures 2(a)-(c). Using equation (
Figure 2.
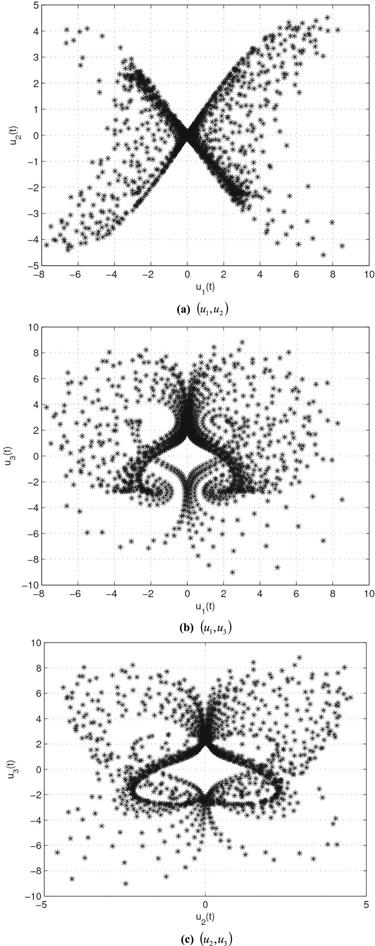
Figure 2.Poincaré section of system (8) at $\alpha =0.99.$
Figure 3.
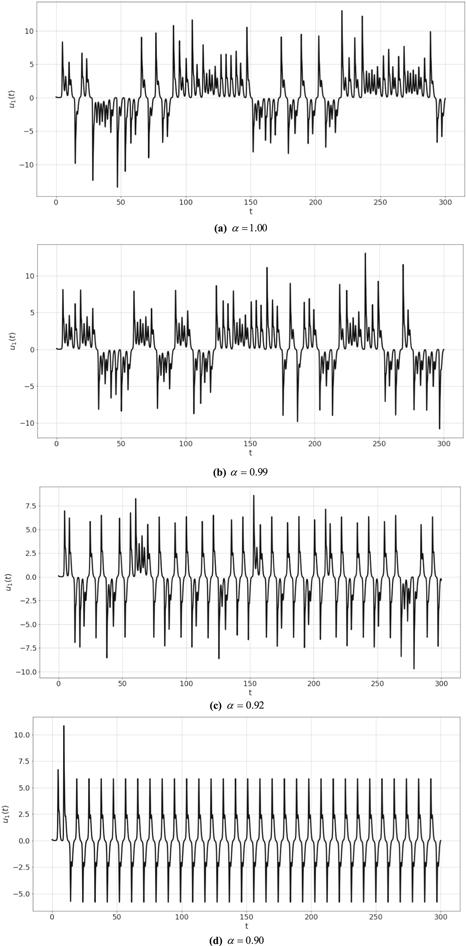
Figure 3.Plot ${u}_{1}\left(t\right)$ of system (8) for different values of $\alpha .$
Figure 4.
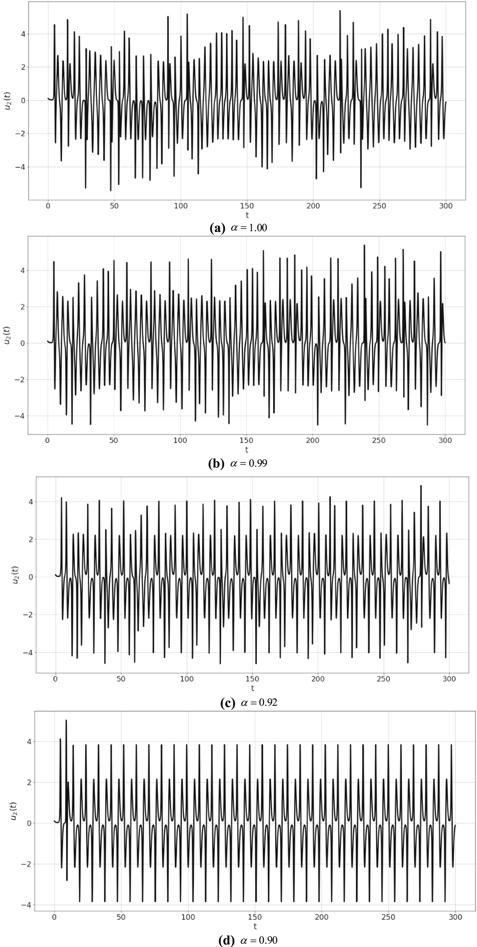
Figure 4.Plot ${u}_{2}\left(t\right)$ of system (8) at different values of $\alpha .$
Figure 5.
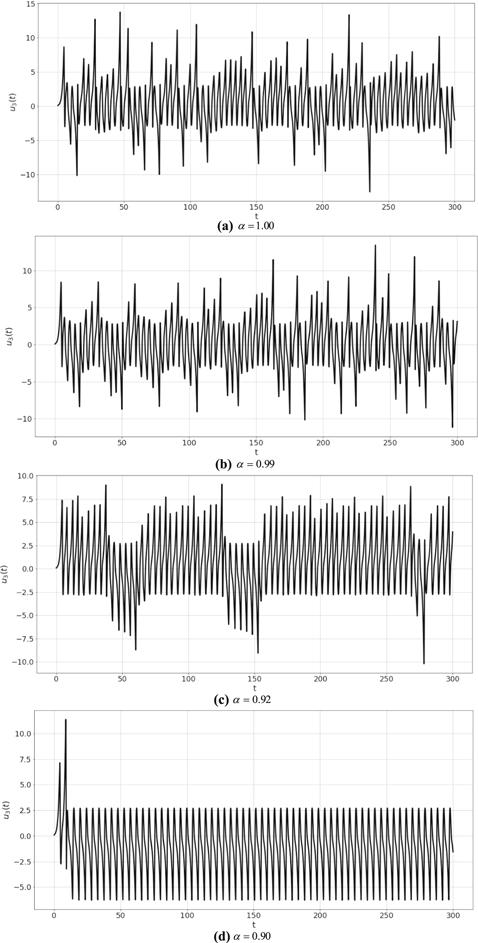
Figure 5.Plot ${u}_{3}\left(t\right)$ of system (8) for different values of $\alpha .$
Table 2.
Table 2.LE and KYD vakues for system (8).
$\alpha $ | ${L}_{1}$ | ${L}_{2}$ | ${L}_{3}$ | ${D}_{KY}$ |
---|---|---|---|---|
1.00 | 0.266 697 | −0.017 213 | −4.695 504 | 2.053 13 |
0.99 | 0.273 420 | −0.017 020 | −4.804 732 | 2.053 36 |
0.92 | 0.003 342 | −0.267 246 | −3.945 491 | 1.933 11 |
0.90 | 0.000 167 | −0.303 911 | −3.942 772 | 1.922 96 |
New window|CSV
Figure 6.
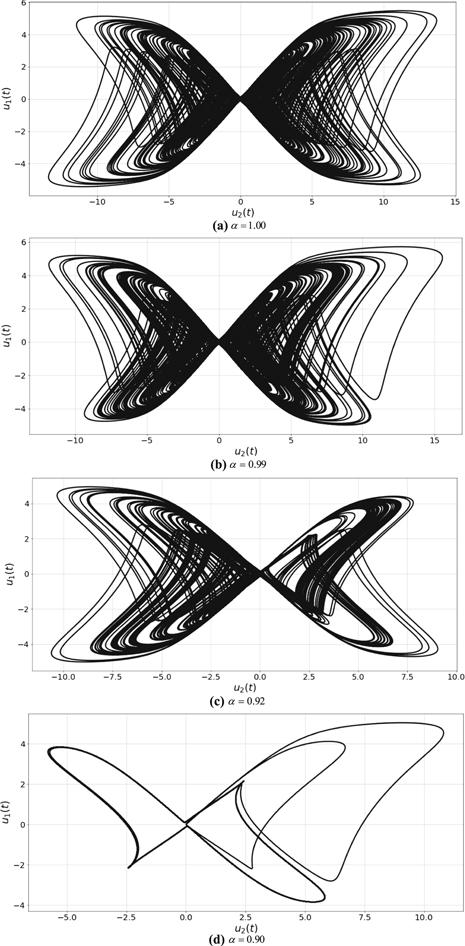
Figure 6.2D phase plot (${u}_{1},{u}_{2}$) of system (8) for different values of $\alpha .$
Figure 7.
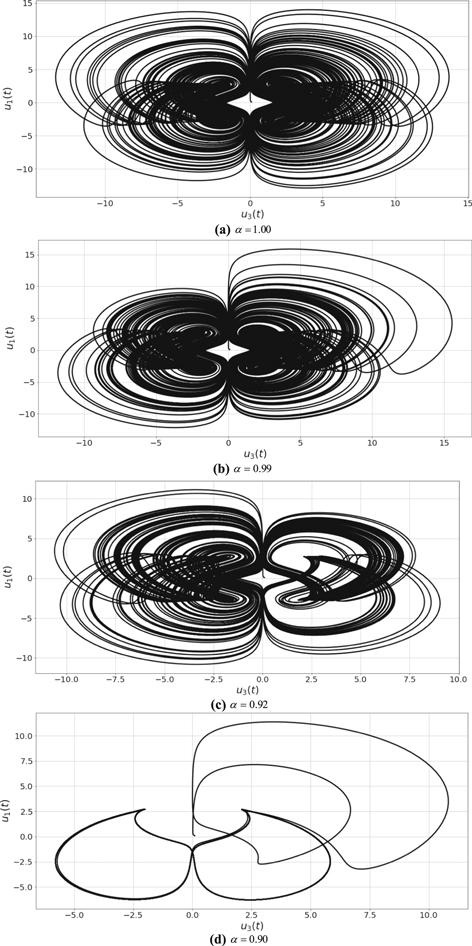
Figure 7.2D phase plot (${u}_{1},{u}_{3}$) of system (8) for different values of $\alpha .$
Figure 8.
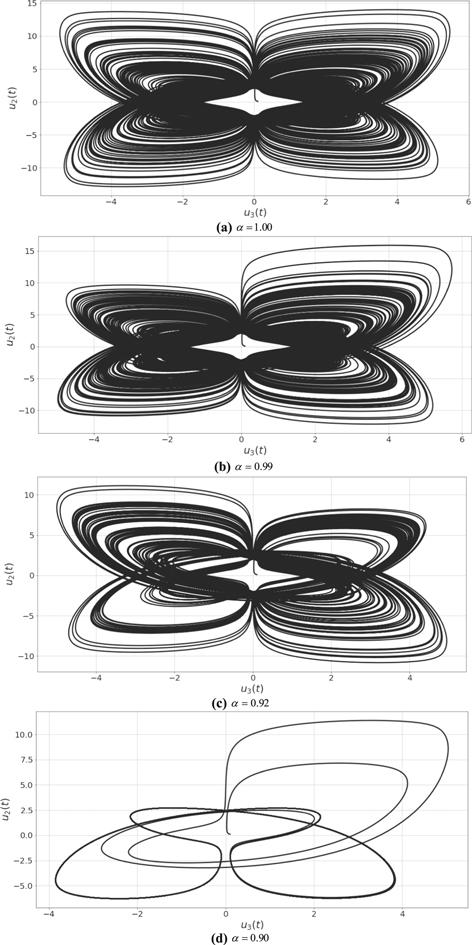
Figure 8.2D phase plot (${u}_{2},{u}_{3}$) of system (8) for different values of $\alpha .$
Figure 9.
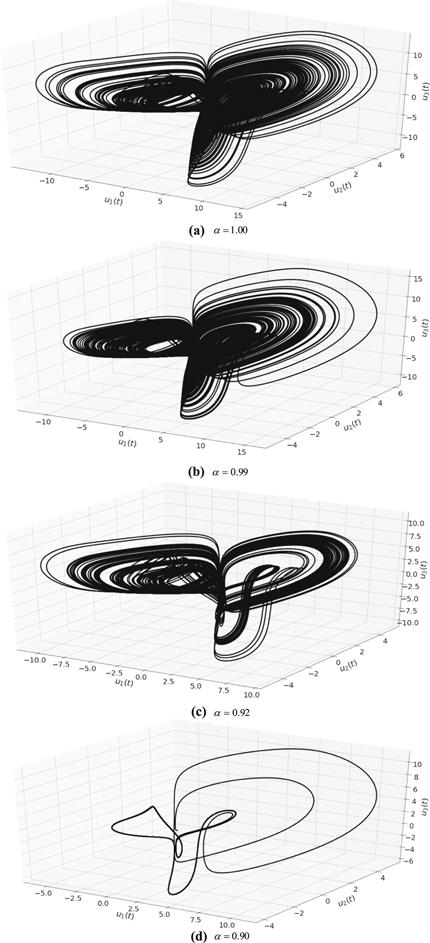
Figure 9.3D flows of system (8) for different values of $\alpha .$
Figure 10.
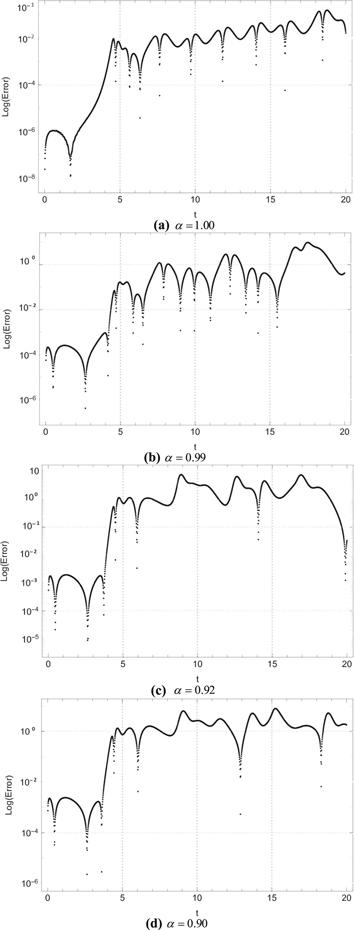
Figure 10.Log errors for ${u}_{1}\left(t\right)$ between system (1) and system (8).
Figure 11.
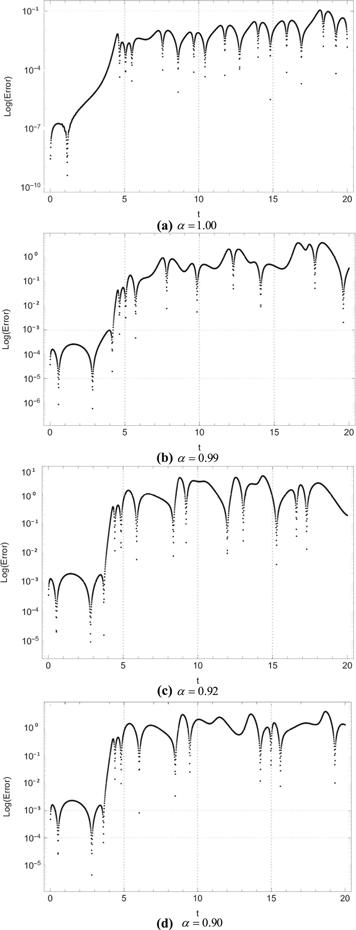
Figure 11.Log errors for ${u}_{2}\left(t\right)$ between system (1) and system (8).
Figure 12.
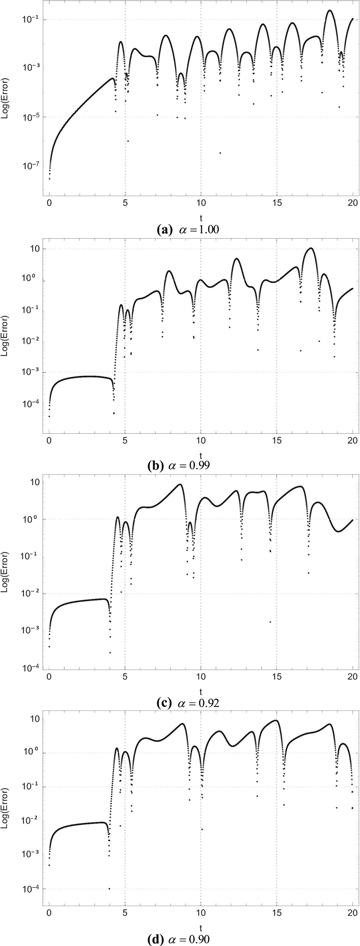
Figure 12.Log errors for ${u}_{3}\left(t\right)$ between system (1) and system (8).
Figure 13.
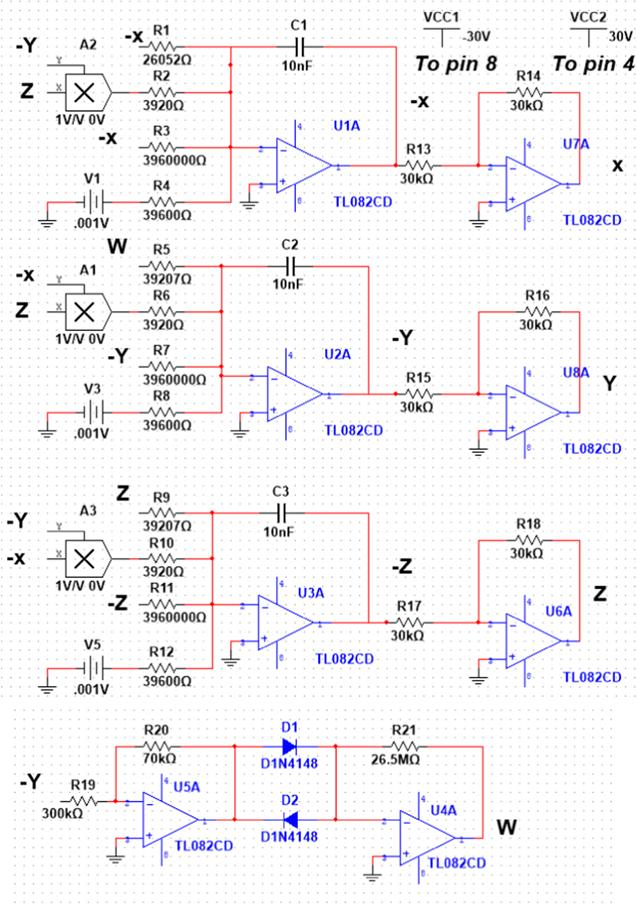
Figure 13.Schematic view of system (8), using MultiSIM 14.1.
4.2. Analog simulation
A circuit simulation for system (8) is presented in this section, with the help of the circuit equations shown below:Table 3.
Table 3.The values of resistors and voltages for capacitors ${C}_{1}={C}_{2}={C}_{3}=10\,{\rm{nF}}$ for different values of $\alpha .$
$\alpha $ | Voltage (${\rm{V}}$) | Resistor ($k{\rm{\Omega }}$) | $\alpha $ | Voltage (${\rm{V}}$) | Resistor ($k{\rm{\Omega }}$) | ||||
---|---|---|---|---|---|---|---|---|---|
1.00 | ${V}_{1}$ | — | ${R}_{1}$ | 20 | 0.92 | ${V}_{1}$ | 0.009 | ${R}_{1}$ | 24.29 |
${R}_{2}$ | 3 | ${R}_{2}$ | 3.63 | ||||||
${R}_{3}$ | 30 | ${R}_{3}$ | 440 | ||||||
${V}_{2}$ | — | ${R}_{4}$ | 3 | ${V}_{2}$ | 0.009 | ${R}_{4}$ | 39.60 | ||
${R}_{5}$ | 30 | ${R}_{5}$ | 36.33 | ||||||
${R}_{6}$ | 3 | ${R}_{6}$ | 3.63 | ||||||
${V}_{3}$ | — | ${R}_{7}$ | — | ${V}_{3}$ | 0.009 | ${R}_{7}$ | 440 | ||
${R}_{8}$ | — | ${R}_{8}$ | 39.60 | ||||||
${R}_{9}$ | — | ${R}_{9}$ | 36.33 | ||||||
${R}_{10}$ | — | ${R}_{10}$ | 3.63 | ||||||
${R}_{11}$ | — | ${R}_{11}$ | 440 | ||||||
${R}_{12}$ | — | ${R}_{12}$ | 39.60 | ||||||
0.99 | ${V}_{1}$ | 0.001 | ${R}_{1}$ | 26.05 | 0.90 | ${V}_{1}$ | 0.011 | ${R}_{1}$ | 23.76 |
${R}_{2}$ | 3.92 | ${R}_{2}$ | 35.64 | ||||||
${R}_{3}$ | 3960 | ${R}_{3}$ | 356.75 | ||||||
${V}_{2}$ | 0.001 | ${R}_{4}$ | 39.60 | ${V}_{2}$ | 0.011 | ${R}_{4}$ | 39.60 | ||
${R}_{5}$ | 39.20 | ${R}_{5}$ | 35.64 | ||||||
${R}_{6}$ | 3.920 | ${R}_{6}$ | 3.564 | ||||||
${V}_{3}$ | 0.001 | ${R}_{7}$ | 3960 | ${V}_{3}$ | 0.011 | ${R}_{7}$ | 356.75 | ||
${R}_{8}$ | 39.60 | ${R}_{8}$ | 39.60 | ||||||
${R}_{9}$ | 39.20 | ${R}_{9}$ | 35.64 | ||||||
${R}_{10}$ | 3.92 | ${R}_{10}$ | 3.564 | ||||||
${R}_{11}$ | 3960 | ${R}_{11}$ | 356.75 | ||||||
${R}_{12}$ | 39.60 | ${R}_{12}$ | 39.60 |
New window|CSV
Figure 14.
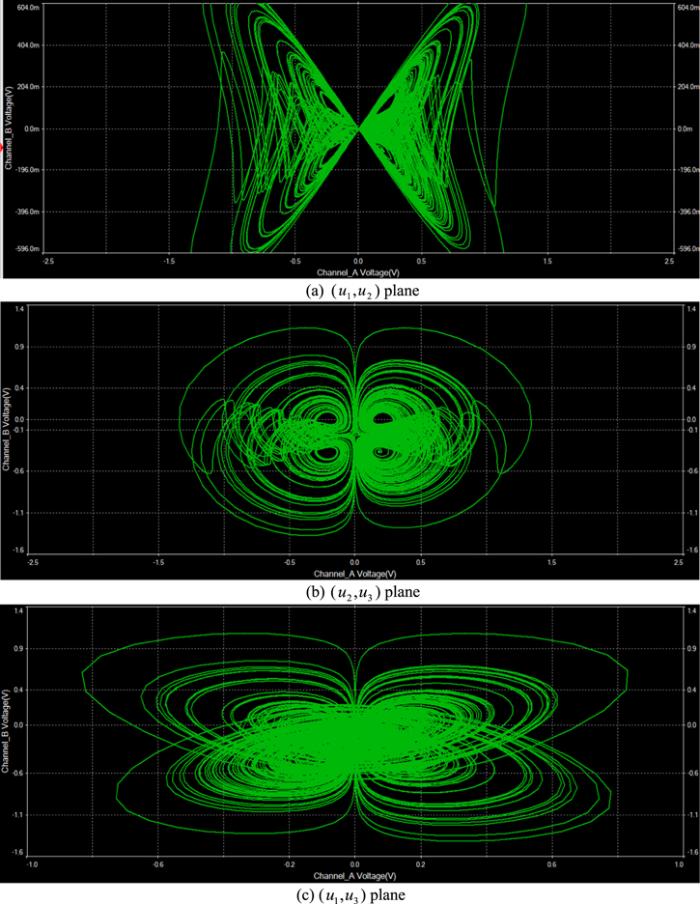
Figure 14.Analogous phase plots of system (8) where $\alpha =1.00.$
Figure 15.
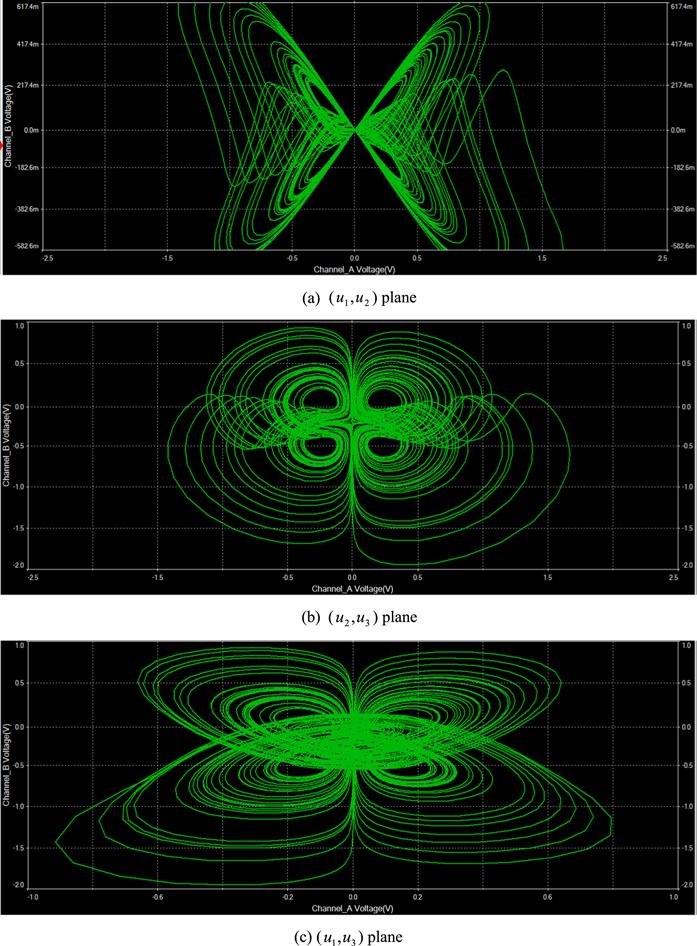
Figure 15.Analogous phase plots of system (8) where $\alpha =0.99.$
4.3. Cryptographic simulation
This section considers the cryptography of voice, image, and animation data, using random number generation (RNG) produced via the solution given by our system (10). The system solution results were tested via NIST-800-22 [31], a rigorous statistical test used in chaotic systems to investigate the consistency of RNG [31] via the calculation of P-values. Here, P-values are given in tables 4-6 for equation (Table 4.
Table 4.${\rm P}$-value test results (NIST-800-22) for chaotic series ${u}_{1}\left(t\right),$ at different values of $\alpha .$
S. No | Test Name | $\alpha $=1 | $\alpha $=0.99 | $\alpha $=0.92 | Results |
---|---|---|---|---|---|
1 | Mono bitFrequency Test | 0.665 006 | 0.484 806 | 0.317 884 | Successful |
2 | Block Frequency Test | 0.678 612 | 0.985 867 | 0.518 35 | Successful |
3 | Runs Test | 0.707 856 | 0.193 446 | 0.360 131 | Successful |
4 | Longest Runs Ones 10 000 | 0.235 903 | 0.556 629 | 0.063 0856 | Successful |
5 | Binary Matrix Rank Test | 0.443 084 | 0.298 222 | 0.045 5016 | Successful |
6 | Spectral Test | 0.474 456 | 1. | 0.458 246 | Successful |
7 | Non-Overlapping Template Matching | 0.529 55 | 0.840 569 | 0.488 286 | Successful |
8 | Overlaping Template Matching | 0.544 82 | 0.981 736 | 0.375 187 | Successful |
9 | Maurers universal Statistic Test | 0.463 56 | 0.183 854 | 0.385 959 | Successful |
10 | Linear Complexity Test | 0.701 088 | 0.515 697 | 0.503 53 | Successful |
11 | Serial Test | {0.259 896,0.713 345} | {0.326 326,0.718 48} | {0.760 873,0.839 907} | Successful |
12 | Approximate Entropy Test | 0.344 719 | 0.223 394 | 0.141 817 | Successful |
13 | Cumulative Sums Test | 0.638 251 | 0.825 325 | 0.593 75 | Successful |
14 | Random Excursions Test | 0.296 283 | 0.194 65 | 0.123 997 | Successful |
15 | Random Excursions Variant Test | 1 | 0.245 779 | 0.863 832 | Successful |
16 | Cumulative Sums Test Reverse | 0.976 076 | 0.822 779 | 0.593 75 | Successful |
New window|CSV
Table 5.
Table 5. ${\rm P}$-value test results (NIST-800-22) for chaotic series ${u}_{2}\left(t\right),$ at different values of $\alpha .$
S. No | Test Name | $\alpha $=1 | $\alpha $=0.99 | $\alpha $=0.92 | Results |
---|---|---|---|---|---|
1 | Mono bitFrequency Test | 0.716 059 | 0.953 96 | 0.367 766 | Successful |
2 | Block Frequency Test | 0.638 647 | 0.474 88 | 0.617 961 | Successful |
3 | Runs Test | 0.004 748 35 | 0.567 602 | 0.784 317 | Successful |
4 | Longest Runs Ones 10 000 | 0.692 827 | 0.933 756 | 0.377 388 | Successful |
5 | Binary Matrix Rank Test | 0.332 02 | 0.180 156 | 0.903 766 | Successful |
6 | Spectral Test | 0.289 315 | 0.524 923 | 0.542 336 | Successful |
7 | Non-Overlapping Template Matching | 0.873 754 | 0.329 533 | 0.137 408 | Successful |
8 | Overlaping Template Matching | 0.366 99 | 0.422 48 | 0.279 795 | Successful |
9 | Maurers universal Statistic Test | 0.336 621 | 0.535 156 | 0.844 607 | Successful |
10 | Linear Complexity Test | 0.229 947 | 0.969 042 | 0.224 795 | Successful |
11 | Serial Test | {0.235 703,0.593 431} | {0.574 309,0.443 98} | {0.864 631,0.918 161} | Successful |
12 | Approximate Entropy Test | 0.023 8884 | 0.586 246 | 0.249 462 | Successful |
13 | Cumulative Sums Test | 0.983 936 | 0.840 404 | 0.627 666 | Successful |
14 | Random Excursions Test | 0.769 331 | 0.804 348 | 0.316 433 | Successful |
15 | Random Excursions Variant Test | 0.947 793 | 0.388 667 | 0.331 918 | Successful |
16 | Cumulative Sums Test Reverse | 0.727 184 | 0.788 972 | 0.263 668 | Successful |
New window|CSV
Table 6.
Table 6.${\rm P}$-value test results (NIST-800-22) for chaotic series ${u}_{3}\left(t\right),$ at different values of $\alpha .$
S. No | Test Name | $\alpha $=1 | $\alpha $=0.99 | $\alpha $=0.92 | Results |
---|---|---|---|---|---|
1 | Mono bitFrequency Test | 0.981 575 | 0.623 605 | 0.724 701 | Successful |
2 | Block Frequency Test | 0.702 138 | 0.786 538 | 0.330 245 | Successful |
3 | Runs Test | 0.145 69 | 0.463 838 | 0.724 432 | Successful |
4 | Longest Runs Ones 10 000 | 0.746 165 | 0.415 693 | 0.423 45 | Successful |
5 | Binary Matrix Rank Test | 0.685 773 | 0.574 195 | 0.570 329 | Successful |
6 | Spectral Test | 0.194 273 | 0.633 482 | 0.474 456 | Successful |
7 | Non-Overlapping Template Matching | 0.834 296 | 0.498 41 | 0.512 831 | Successful |
8 | Overlaping Template Matching | 0.219 927 | 0.664 717 | 0.230 988 | Successful |
9 | Maurers universal Statistic Test | 0.235 758 | 0.139 78 | 0.688 589 | Successful |
10 | Linear Complexity Test | 0.709 004 | 0.523 366 | 0.803 015 | Successful |
11 | Serial Test | {0.412 075,0.209 017} | {0.197 128,0.442 195} | {0.437 12,0.534 607} | Successful |
12 | Approximate Entropy Test | 0.734 978 | 0.135 837 | 0.845 66 | Successful |
13 | Cumulative Sums Test | 0.845 347 | 0.770 349 | 0.791 612 | Successful |
14 | Random Excursions Test | 0.654 05 | 0.281 157 | 0.428 759 | Successful |
15 | Random Excursions Variant Test | 0.262 747 | 0.326 878 | 0.391 404 | Successful |
16 | Cumulative Sums Test Reverse | 0.825 325 | 0.360 73 | 0.635 598 | Successful |
New window|CSV
Figure 16.
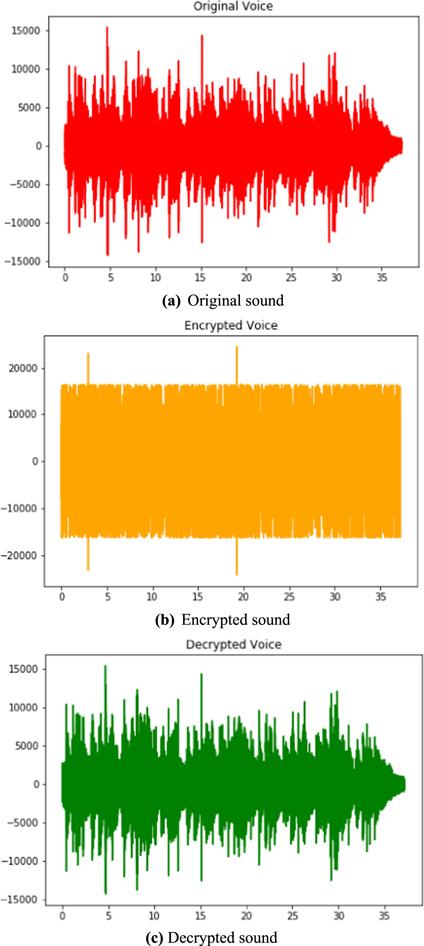
Figure 16.Sound data.
Figure 17.
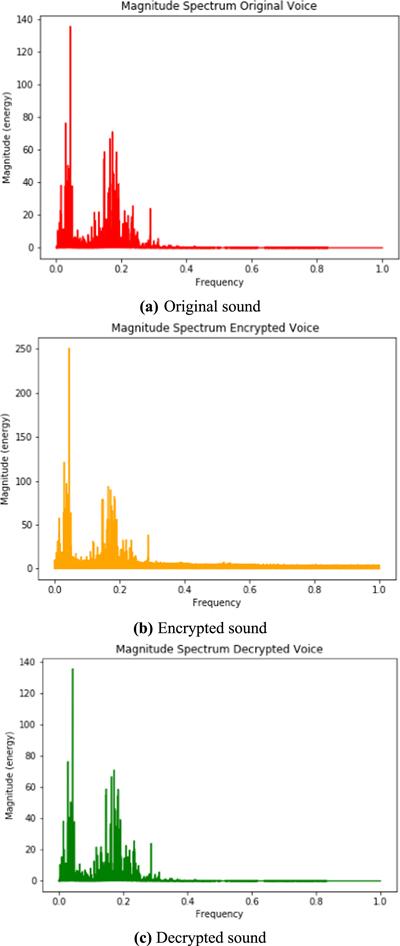
Figure 17.Magnitude spectrum of sound.
Figure 18.
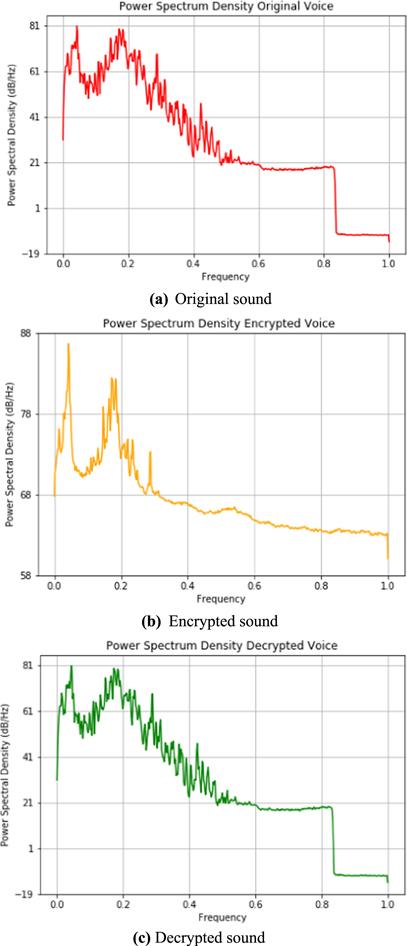
Figure 18.Power spectrum density of sound data.
Figure 19.
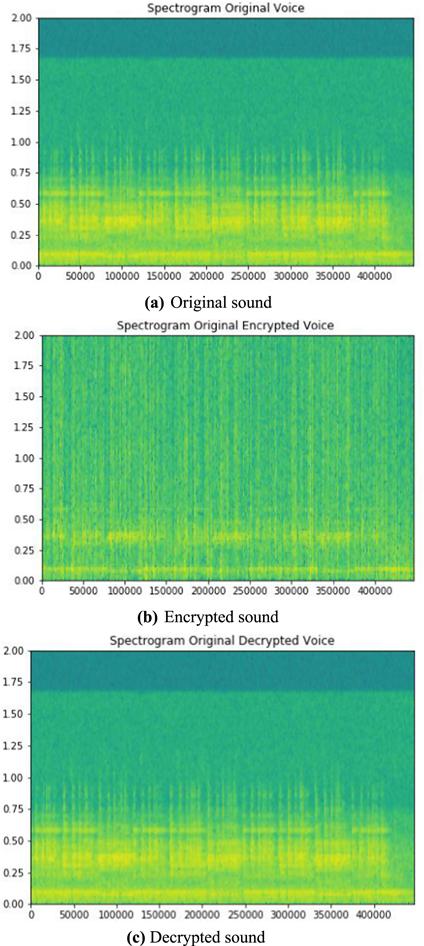
Figure 19.Spectrogram of sound data.
Figure 20.
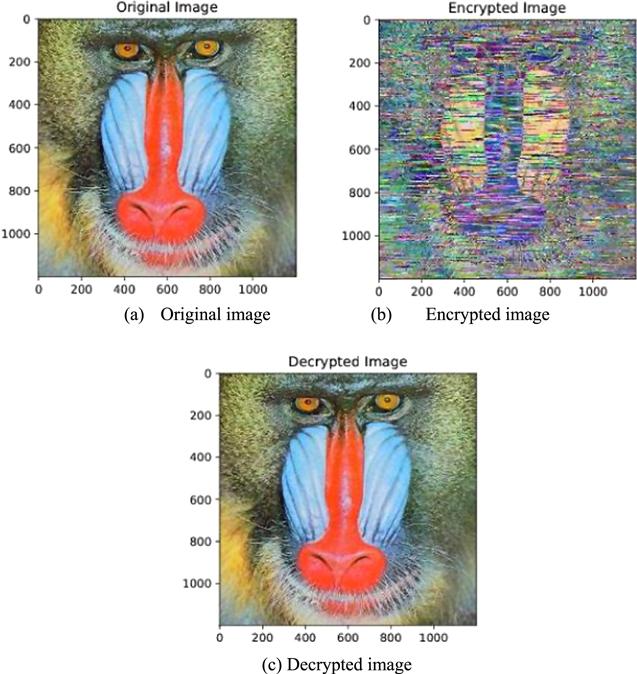
Figure 20.Cryptographic test on image 1 (Mandrill).
Figure 21.
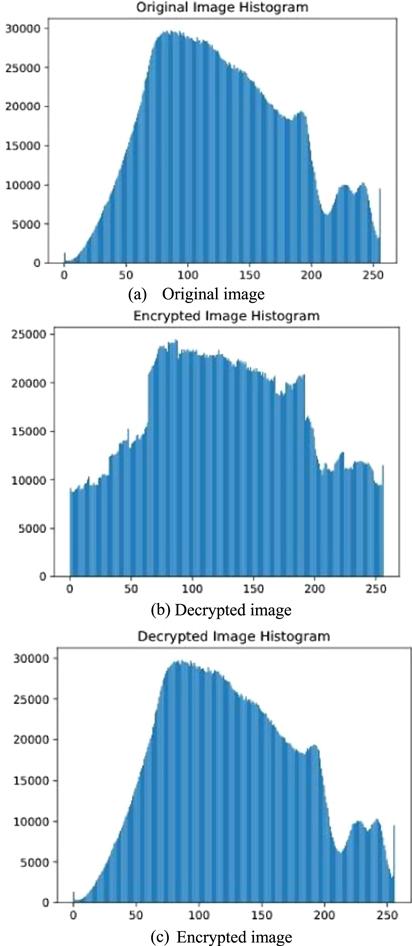
Figure 21.Histogram of cryptographic test on image 1.
Figure 22.
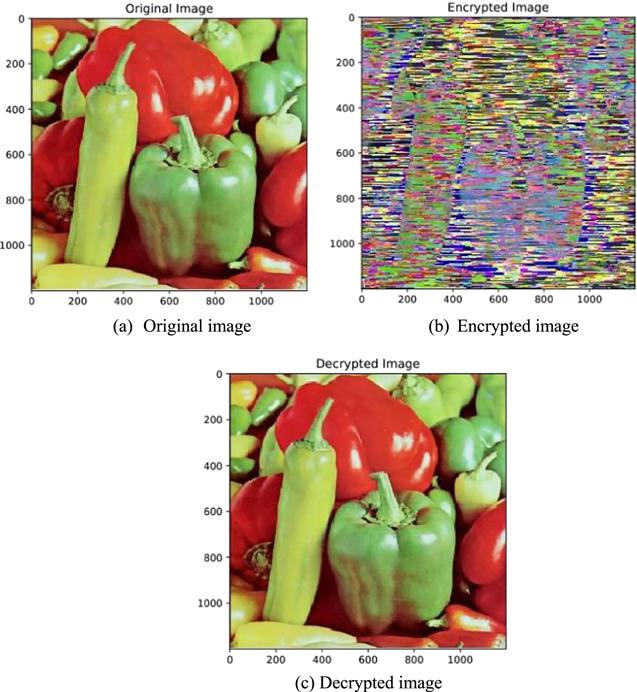
Figure 22.Cryptographic test on image 2 (Peppers).
Figure 23.
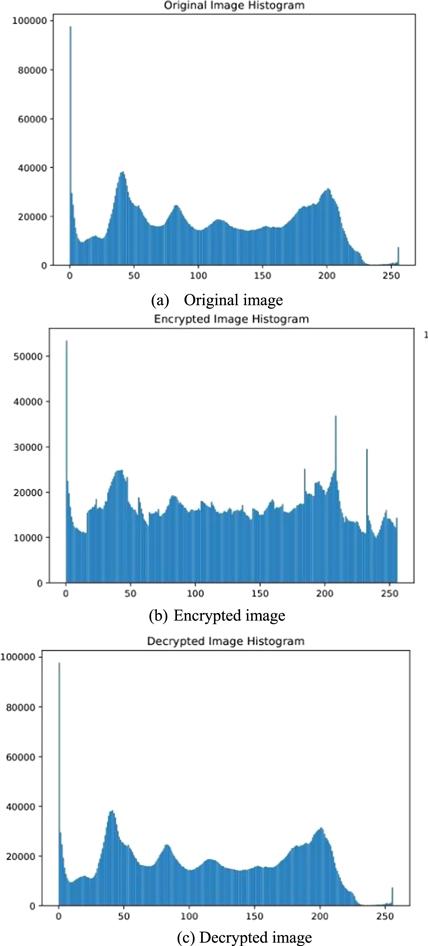
Figure 23.Histogram of cryptographic test on image 2.
Figure 24.
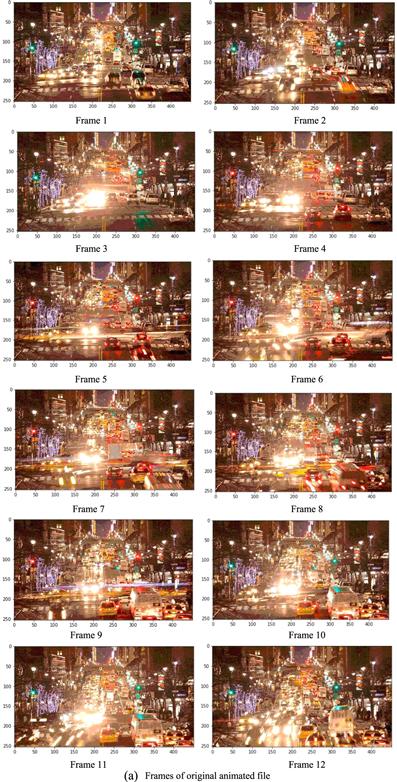
Figure 24.Frames from an animated (.gif) file original, encrypted, and decrypted, together with the corresponding histograms.
4.4. Security analysis
In this section, the cryptosystem's sensitivity, as well as its capacity to withstand attack, are analyzed in relation to the test images, on the basis of the parameters shown below.4.4.1. Histogram analysis
The statistical characteristics of the images are presented in the form of histograms. The original and encrypted images are analyzed in relation to the behavior of the graphs. The graph of encrypted image shows uniformity, which suggests us that the encryption is capable of resisting a statistical attack. Histograms for the Mandrill and Pepper images are provided in figures 21 and 23, respectively.4.4.2. Key sensitivity analysis
Small changes in the secret key, which alter the encryption image, are indicative of key sensitivity. The two parameters, NPCR and UAIC, are used to measure key sensitivity, and are discussed below:NPCR:The number of pixel change rate (NPCR) can be expressed as follows:UACI: The unified average changing intensity (UACI) can be written as follows:
4.4.2.1. Information entropy analysis
Entropy indicates the randomness of a system, and can be calculated as follows:The mean number of pixels in the Mandrill and Peppers images are assumed to be 98.958 079 and 99.104 309, respectively, indicating that these images differ by only one pixel. Meanwhile, the UACI values indicate the absence of significant change between the test images used in the study. Analyses conducted by means of these parameters are performed on the two test images, and the results are tabulated in table 7. The correlations of original and encrypted images of the Mandrill image are 0.924 877 and 0.861 089, respectively, and those for the Peppers image are 0.956 570 and 0.934 504, respectively, indicating that the images are neither identical nor opposite. Furthermore, table 7 clearly shows that the entropy of the original image is less than the entropy of the encrypted image; hence, rare uncertainty transmits.
Table 7.
Table 7.Numerical values of Parameters for security analysis.
Entropy | ||||
---|---|---|---|---|
Test images | NPCR (%) | UAIC (%) | Plain Image | Encrypted Image |
Mandrill | 98.958 079 | 21.426 310 | 5.333 076 | 5.777 796 |
Peppers | 99.104 309 | 24.509 208 | 4.342 038 | 5.742 104 |
New window|CSV
5. Conclusion
This paper presents a four-wing chaotic attractor system, having sine-hyperbolic nonlinearity, and incorporating FDs. This fusion of FDEs has vast implications in terms of the secure encryption of different types of data, such as voice, image, and animated images, which are ubiquitous in digital communication. The equations are tested numerically and via electronic simulation, using Mathematica and MultiSIM, respectively. Numerical results are simulated for different values of $\alpha $ in order to confirm the validity of this multi-wing chaotic system. The cryptographical element is coded in Python, and is tested on audio, image, and animated gif files. The study offers the following conclusions: The multi-wing system possesses multi-stability equilibrium points at distinct values of $\alpha .$The physical parameters of the model can be simulated by means of circuit design.
Many keys can be generated to form sheltered systems for data communication.
The proposed mechanism has the capacity to track files in different formats.
This system is also suitable for other chaos-based applications.
Reference By original order
By published year
By cited within times
By Impact factor
[Cited within: 3]
[Cited within: 2]
DOI:10.1016/j.cnsns.2013.04.001 [Cited within: 1]
DOI:10.1016/j.cnsns.2018.02.019 [Cited within: 1]
DOI:10.1175/1520-0469(1963)020<0130:DNF>2.0.CO;2 [Cited within: 1]
DOI:10.1007/978-3-319-13132-0_4 [Cited within: 1]
DOI:10.1088/1402-4896/ab8581 [Cited within: 1]
DOI:10.1016/j.cjph.2017.11.009 [Cited within: 1]
DOI:10.1016/j.aeue.2017.03.003 [Cited within: 1]
DOI:10.1177/1077546315574649 [Cited within: 1]
DOI:10.1016/j.camwa.2011.05.029 [Cited within: 1]
DOI:10.1163/156939312798954964 [Cited within: 1]
DOI:10.1063/1.4958717 [Cited within: 1]
DOI:10.1016/j.chaos.2016.01.016 [Cited within: 1]
DOI:10.1016/j.chaos.2017.10.032 [Cited within: 1]
DOI:10.1177/1461348418813015 [Cited within: 1]
DOI:10.1109/81.995671 [Cited within: 1]
DOI:10.1142/S0218127403008405 [Cited within: 1]
DOI:10.1142/S021812741250143X [Cited within: 1]
DOI:10.1002/cta.736 [Cited within: 1]
DOI:10.1142/S0218127410025387 [Cited within: 1]
DOI:10.1016/j.chaos.2007.01.029 [Cited within: 1]
DOI:10.7498/aps.63.080505 [Cited within: 1]
DOI:10.1142/S0218127406015179 [Cited within: 1]
DOI:10.1016/j.ijleo.2016.12.016 [Cited within: 1]
DOI:10.1016/j.apm.2015.10.011 [Cited within: 1]
DOI:10.1109/PACET48583.2019.8956261 [Cited within: 1]
[Cited within: 1]
DOI:10.1016/0167-2789(85)90011-9 [Cited within: 1]
[Cited within: 1]
[Cited within: 2]
DOI:10.5281/zenodo.3693098 [Cited within: 2]