
Source apportionment and spatial prediction of heavy metals in soils of Yantai coastal zone
LYU Jianshu
收稿日期:2020-03-14修回日期:2021-02-28网络出版日期:2021-03-25
基金资助: |
Received:2020-03-14Revised:2021-02-28Online:2021-03-25
Fund supported: |
作者简介 About authors
吕建树(1986-), 男, 山东莱芜人, 博士, 副教授, 主要从事重金属环境地球化学和地质统计学等研究。E-mail:

摘要
关键词:
Abstract
Keywords:
PDF (3743KB)元数据多维度评价相关文章导出EndNote|Ris|Bibtex收藏本文
本文引用格式
吕建树. 烟台海岸带土壤重金属定量源解析及空间预测. 地理学报[J], 2021, 76(3): 713-725 doi:10.11821/dlxb202103015
LYU Jianshu.
1 引言
随着经济社会快速发展,土壤重金属污染日趋严重[1,2,3];2014年发布的《全国土壤污染状况调查公报》显示中国土壤环境状况总体不容乐观,主要以重金属无机污染为主。土壤重金属的影响因素多样且机制复杂,既有成土因素的制约,又有工业、农业等人类活动的深刻影响[4,5],进而展示出复杂的来源机制和空间异质性。定量解析污染来源并准确地绘制土壤重金属的空间分布图是土壤重金属调查评价的核心,可为区域土壤重金属管控提供参考。长期以来,土壤重金属的污染来源以定性的源识别为主,主要应用主成分、相关分析和聚类分析等多元统计方法将土壤重金属数据进行降维归类[6,7,8,9,10]。近年来,受体模型如正定矩阵因子分解(PMF)和绝对主成分/多元线性回归(APCS/MLR)越来越多的应用于土壤重金属的定量源解析。Lv等[11]应用PMF和APCS/MLR定量解析了山东广饶土壤重金属的来源;Guan等[12]使用PMF对河西走廊典型区域的土壤重金属开展了源解析研究;Luo等[13]利用APCS/MLR解析了厦门城市土壤重金属来源贡献率。PMF在非负约束并考虑数据的不确定性的前提下进行因子分解,得到源成分谱矩阵和因子贡献矩阵[14],比APCS/MLR有更高的精度和更广泛的适用性,是美国环保署(US-EPA)推荐的源解析方法。
受体模型是单纯的数理统计模型,缺乏空间异质性的表达,难以展示土壤重金属含量的空间分布规律。地统计学是刻画土壤重金属的空间结构和变异的范式性工具[15,16],包括克里格和随机模拟两大类。克里格插值估计应用最广。方斌等[3]运用普通克里格对江浙地区两个典型茶园土壤中Cu的空间分异规律进行了对比分析;吕建树等[5]基于指示克里格绘制了土壤重金属污染风险概率图;Lv等[17]应用因子克里格分析了土壤重金属的多尺度空间变异特征。然而,克里格的平滑效应限制了其空间预测的精度。序贯高斯模拟 (SGS)克服了克里格平滑效应的缺点,可以产生多个等概率的实现,并可进行不确定性分析[18,19]。由于土壤中各个重金属不是孤立的元素,多个重金属元素可能受同一环境因子的控制而具有空间相关性;多变量序贯高斯协同模拟(SGCS)越来越受到重视,主要应用协同化区域线性模型模拟变量之间的空间相关关系,进而实现多变量协同模拟[20,21]。然而,SGCS数据计算量巨大,运行时间缓慢,对计算机硬件配置要求非常高。独立成分分析(ICA)可将多个变量分解为不相关的独立成分(ICs),进而表达数据的相关关系[22,23],结合SGS可实现复杂的多元地统计模拟。
烟台是中国首批设立的14个沿海开放城市之一,工业发达且门类齐全,是中国最大的黄金产地,农业以水果生产为特色;海岸带地区是烟台市经济和社会发展的精华地带,密集的人类活动必然对土壤环境产生强烈影响。本文以烟台海岸带为研究区,系统采集了1067个表层土壤样品(0~20 cm),测定了土壤中As、Cd、Co、Cr、Cu、Hg、Mn、Ni、Pb和Zn的含量,采用PMF受体模型定量解析土壤重金属的来源,构建了基于ICA和SGS的多元地统计模拟方法,对土壤重金属含量进行空间预测,并应用不确定性分析划定潜在污染区域。
2 材料与方法
2.1 研究区概况
本文将烟台市牟平到龙口约271 km的海岸线向陆一侧做大致30 km的缓冲区,以此作为研究区(图1a),地理坐标介于120°12′E~121°56′E、37°12′N~37°51′N之间,濒临黄海和渤海;包括芝罘区、莱山区、牟平区、福山区、蓬莱市和龙口市4区2市的海岸带,总面积约3957 km2。研究区为暖温带大陆性季风气候,年平均温度13.1℃,年平均降雨量525 mm;主要河流有大沽夹河、黄水河及界河,均北流入海。海拔介于0~782 m之间,属于山东半岛低山丘陵区,起伏和缓;以基岩海岸为主,海蚀地貌发育。成土母质为花岗岩、变质岩、玄武岩及第四系(图1b),土壤类型主要为棕壤和潮土。研究区工业发达、门类齐全,主导产业为机械制造、电子信息、食品加工、黄金和现代化工;黄金储量丰富,分布有玲珑、大柳行、黑岚沟、邓格庄等金矿,并形成了众多金矿尾矿库。同时,烟台海岸带是中国北方著名的名优农产品生产基地,以苹果、樱桃、葡萄种植而闻名(图1c)。图1
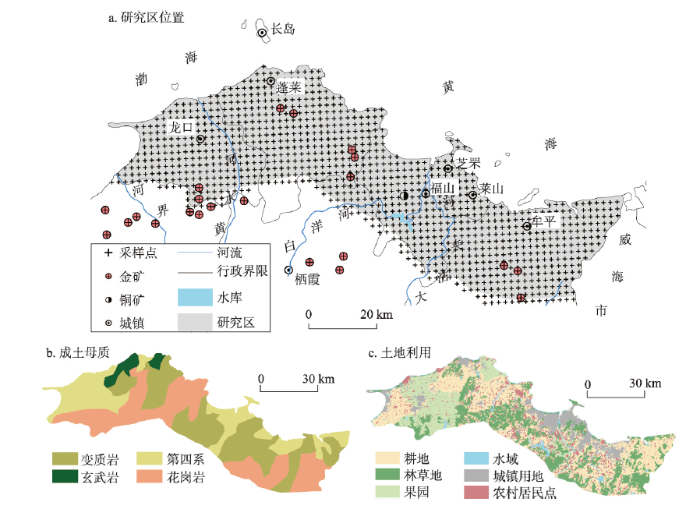
图1研究区位置、成土母质和土地利用类型
Fig. 1Location of the study area with sampling sites, parent materials, and land use types
2.2 土壤采样和分析测试
基于GIS技术将烟台海岸带分为2 km×2 km的网格,每个网格的中心为预设采样点,全区共包括1067个采样点(图1a)。本文土壤样品采集利用多点采样法进行,具体在预设采样点位周围设置5个子采样点,在每个子采样点垂直采集0~20 cm的表层土壤,等量混合后,装入干净自封袋,样品原始重量大于1 kg;利用GPS确定采样点的实际坐标位置,并记录相关的信息,如土地利用类型、植被状况、地貌及土壤类型等。土壤样品经过室温自然风干、去除杂物等初步处理后,取约10 g原始样品,用玛瑙无污染样品制备机将样品研磨至粒径小于0.149 mm备用。研磨后土壤样品经HClO4-HNO3-HF消解后,用电感耦合等离子体发射质谱仪测试Cd、Co、Cr、Cu、Mn、Ni、Pb和Zn的含量;经过H2SO4-HNO3-KMnO4消解后,用原子荧光光度计测定As和Hg的含量。分析过程以国家土壤一级标准物质(GSS-1)为质控标样,所有元素的回收率均在(100±10)%。2.3 PMF受体模型
本文使用US-EPA开发的PMF 5.0程序[24]实现土壤重金属的源解析。由m个样品、n个重金属元素组成的原始数据矩阵式中:
在矩阵E中,每个元素eij定义为:
式中:i表示从1到m个样品;j表示从1到n个元素;k表示从1到p个来源。
PMF的目标是在非负限制下最小化目标函数Q获取最优的矩阵G和F,用多线性引擎求解(Multilinear engine-2, ME-2)。
式中:uij代表第i个样品中第j种元素的不确定性。不确定性基于元素检出限和测量误差百分比计算[24]。
2.4 基于ICA和SGS的多元地统计模拟
本文提出的多元地统计模拟可分为7个基本步骤。(1)高斯变换(Gaussian Anamorphosis)。由于SGS需要正态分布的数据[15,16],因此首先将由n个重金属元素、m个样品组成始数据矩阵X(n×m)逐行进行高斯变换,形成每行数据符合高斯分布的矩阵Y(n×m)。原理如下[15, 17]:
原始变量
并且这个函数可以展开为埃尔米特多项式:
利用
(2)独立成分分析。Y(n×m)可以表达为[23]:
式中:A(n×n)为混合矩阵;S(n×m)为来源矩阵,每行代表一个独立成分。
来源矩阵S(n×m)可以表示为:
式中:W(n×n)为解混矩阵(Unmixing Matrix),可表示为A的逆矩阵A-1。
本文使用R软件包FastICA[23]来实现ICA分解,将包含高斯变量数据转换成同样数量的独立成分,并可以实现二者的逆转换。
(3)对各个独立成分进行经验变异函数计算,并拟合到理论变异函数。
(4)采用SGS对各个独立成分进行1000次模拟[16, 18]。首先创建通过所有网格节点的随机路径,每个节点在每个模拟序列中只被访问一次;在每个网格节点处:① 利用简单克里格估计条件累计分布函数(Conditional Cumulative Distribution Function, CCDF)的参数,包括均值和方差;② 从CCDF中随机抽取一个模拟的正态得分值,获得该点的模拟值,然后将其添加到网格节点;③ 沿着随机路径访问下一个网格节点,重复步骤①和②,直到每个节点均被赋予模拟值。
(5)利用公式(6)将独立成分模拟值逆转换到高斯变量模拟值。
(6)利用公式(5)将高斯变量的模拟值逆转换到原始变量模拟值。
(7)局部和空间不确定性分析。局部不确定性(Local Uncertainty)为土壤重金属在位置um处高于污染阈值zc的概率[25,26],可表示为:
式中:1000为模拟的次数,
如果设定一个污染临界概率(Critical Probability, P),应用
式中:
3 结果与讨论
3.1 烟台海岸带土壤重金属描述性统计及富集系数
烟台海岸带土壤中As、Cd、Co、Cr、Cu、Hg、Mn、Ni、Pb和Zn的平均值分别为7.8 mg kg-1、0.17 mg kg-1、11.2 mg kg-1、58.8 mg kg-1、32.3 mg kg-1、0.078 mg kg-1、491 mg kg-1、25.3 mg kg-1、34.7 mg kg-1和68.0 mg kg-1(表1)。除Mn以外的9种元素的平均值均不同程度的超过山东东部土壤背景值[27]。所有元素(除Co和Mn没有可比值)的平均值均低于农用地土壤污染风险筛选值(GB 15618-2018),但最大值均超过农用地土壤污染风险筛选值,其中Cd和Pb的最大值分别是农用地风险筛选值的27.6和7.7倍。除Cr、Mn和Zn没有可比值外,所有元素的平均值均低于第一类建设用地土壤污染风险筛选值(GB 36600-2018),As、Co和Pb的最大值超过第一类建设用地风险筛选值。As、Cd、Cu、Hg和Pb的变异系数分别为0.82、1.66、0.74、2.23和1.12,处于高变异水平,且正偏度均较大,说明异常值的存在。K-S检验表明烟台海岸带土壤中10种重金属元素均不符合正态分布,在地统计分析之前应该进行数据变换,使之符合正态分布。Tab. 1
表1
表1烟台海岸带土壤重金属描述性统计(mg kg-1)
Tab. 1
As | Cd | Co | Cr | Cu | Hg | Mn | Ni | Pb | Zn | pH | |
---|---|---|---|---|---|---|---|---|---|---|---|
最小值 | 2.2 | 0.01 | 0.6 | 7.2 | 1.1 | 0.006 | 63 | 3.6 | 16.1 | 6.9 | 4.66 |
最大值 | 99.0 | 8.27 | 45.2 | 206.6 | 302.1 | 3.210 | 1634 | 144.9 | 917.6 | 493.9 | 9.35 |
平均值 | 7.8 | 0.17 | 11.2 | 58.8 | 32.3 | 0.078 | 491 | 25.3 | 34.7 | 68.0 | 6.76 |
标准差 | 6.33 | 0.28 | 4.67 | 23.41 | 23.91 | 0.17 | 153.90 | 11.87 | 38.83 | 26.60 | 0.95 |
变异系数 | 0.82 | 1.66 | 0.42 | 0.40 | 0.74 | 2.23 | 0.31 | 0.47 | 1.12 | 0.39 | 0.14 |
偏度 | 7.69 | 23.13 | 1.17 | 1.40 | 3.56 | 9.50 | 1.61 | 2.43 | 19.05 | 4.85 | -0.05 |
峰度 | 90.04 | 647.80 | 3.94 | 5.74 | 25.28 | 126.58 | 8.22 | 16.28 | 408.44 | 64.44 | -1.07 |
K-S检验(P) | 0.000 | 0.000 | 0.002 | 0.001 | 0.000 | 0.000 | 0.000 | 0.000 | 0.000 | 0.000 | 0.000 |
山东东部土壤 重金属背景值[27] | 6.3 | 0.108 | 11.0 | 56.2 | 19.6 | 0.029 | 552 | 23.5 | 25.4 | 56.1 | - |
农用地土壤污染 风险筛选值* | 30 | 0.3 | - | 200 | 100 | 2.4 | - | 100 | 120 | 250 | - |
第一类建设用地土壤污染风险筛选值 | 20 | 20 | 20 | -** | 2000 | 8 | - | 150 | 400 | - | - |
新窗口打开|下载CSV
富集系数可以辨别土壤重金属相对于背景值的累积状况,一般采用土壤中性质稳定、难以迁移且极少受人类活动影响的大量元素作为参考元素[28,29]。参照Wu等[30]和Mao等[31]的研究,本文采用Ti作为参考元素,计算研究区土壤重金属的富集系数(图2)。As、Co、Cr、Mn和Ni的富集系数平均值分别为1.14、1.02、1.06、0.92和1.08,表明这5种重金属基本能保持自然本底水平;Cd、Cu、Hg、Pb和Zn的富集系数介于1.27~2.84之间,这些元素在表层土壤中产生明显富集。特别的,Cd、Hg和Pb的富集系数最大值达到62.08、110.97和46.42,说明这3种元素在局部强烈富集。
图2
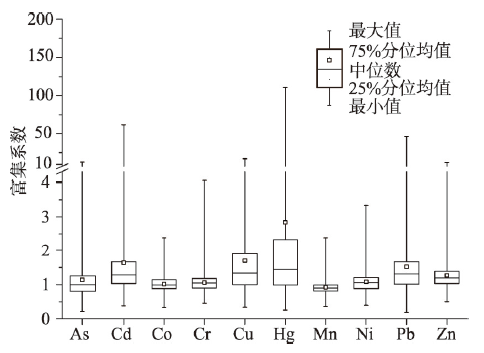
图2烟台海岸带土壤重金属富集系数箱线
Fig. 2Box plot of enrichment factors of heavy metals in soils of Yantai coastal zone
3.2 烟台海岸带土壤重金属来源定量解析
为了获得最佳的收敛性,本文测试了不同数量的因子输入PMF模型,最终选择4个因子作为PMF模型的最佳结果(表2)。所有重金属的预测值/实际值比值均接近1,预测值和实际值之间的决定系数R2均大于0.63,表明PMF模型的拟合精度较高。Tab. 2
表2
表2烟台海岸带土壤重金属PMF源解析结果
Tab. 2
模型验证 | 源成分谱(mg kg-1) | 源贡献率(%) | ||||||||||
---|---|---|---|---|---|---|---|---|---|---|---|---|
R2 | 预测值/实际值 | 因子1 | 因子2 | 因子3 | 因子4 | 因子1 | 因子2 | 因子3 | 因子4 | |||
As | 1.00 | 1.00 | 6.1 | 1.0 | 0.5 | 0.1 | 79.2 | 12.6 | 6.6 | 1.5 | ||
Cd | 0.79 | 0.96 | 0.06 | 0.01 | 0.10 | 0.00 | 33.8 | 58.3 | 5.7 | 2.1 | ||
Co | 0.93 | 0.98 | 9.3 | 0.7 | 0.8 | 0.2 | 85.0 | 6.2 | 6.9 | 1.9 | ||
Cr | 0.83 | 0.98 | 47.9 | 4.6 | 3.6 | 1.3 | 83.4 | 8.1 | 6.4 | 2.2 | ||
Cu | 1.00 | 1.00 | 9.9 | 5.7 | 16.3 | 0.4 | 30.7 | 17.6 | 50.5 | 1.2 | ||
Hg | 1.00 | 0.98 | 0.010 | 0.006 | 0.003 | 0.059 | 12.7 | 8.1 | 3.5 | 75.7 | ||
Mn | 0.88 | 0.96 | 369 | 66 | 29 | 8 | 78.2 | 14.0 | 6.2 | 1.6 | ||
Ni | 0.85 | 0.96 | 20.8 | 1.3 | 1.7 | 0.5 | 85.3 | 5.5 | 6.9 | 2.3 | ||
Pb | 0.83 | 0.93 | 15.5 | 13.1 | 2.8 | 1.1 | 47.7 | 40.3 | 8.5 | 3.5 | ||
Zn | 0.63 | 0.94 | 32.6 | 23.0 | 6.8 | 1.8 | 50.8 | 35.8 | 10.6 | 2.8 |
新窗口打开|下载CSV
因子1对As、Co、Cr、Mn、Ni和Zn的贡献率最大,达到了79.2%、85.0%、83.4%、78.2%、85.3%和50.8%,同时这些元素的富集系数小于或接近1。Co、Cr、Mn和Ni均为铁族元素,具有相似的原子半径和化学价态[32],在矿物形成过程中能够相互替代,易于在铁镁质矿物中共同产生富集,导致基性岩和超基性中铁族矿物的含量远高于其他岩类。而且,化肥和有机肥的施用对Co、Cr、Mn和Ni含量的影响均小于其土壤背景值[1, 33]。因而,因子1代表自然来源。本文与前人的结果相一致,Nanos等在西班牙Duero河流域[34]、Lv在山东广饶[11]以及Cai等在广东顺德[35]的研究均表明,Co、Cr、Mn、Ni与其他元素的组合指示该组合受到成土母质的控制。
因子2主要与Cd、Pb和Zn有关,贡献率分别为58.3%、40.3%和35.8%。研究区有发达的黄金和铝冶炼、金属加工、电镀和制造业,可通过“三废”排放,造成Cd、Pb和Zn在土壤中的富集[36,37,38]。同时,金矿尾矿库众多,尾矿渣中Cd、Pb和Zn的含量可达1500、1.0和100 mg kg-1 [39];在降水的冲刷下,极易造成周边土壤的污染。交通来源也是土壤Cd、Pb和Zn的重要来源。四乙基铅曾作为汽油防爆剂使用,尽管含铅汽油已被禁止,但土壤中的铅积累仍然存在;此外,刹车片磨损、润滑油损耗、轮胎磨损、汽车零部件的腐蚀都会造成Cd、Pb和Zn在土壤中的富集[40,41]。因此,F2代表工业和交通排放来源。Lv等[17]发现山东日照土壤中Cd、Pb和Zn受到人类活动的显著影响,且主要展示在小尺度上。Hu等[42]对河南邯郸的研究表明Cd、Pb和Zn主要来源于工业和交通排放,尤其是采矿和冶炼。Yang等[43]在浙江温岭的研究发现Cd主要来自工业排放,Pb和Zn主要来自于交通排放。
因子3对Cu的贡献率最高(50.5%)。烟台海岸带丘陵面积广阔,是中国重要的苹果、樱桃和葡萄产地。当地果农常年施用富含CuSO4的波尔多液作为果树杀菌剂,大量的Cu进入到果园土壤。山东省耕地地力调查与评价发现烟台市土壤中的有效铜含量达到7.27 mg kg-1,是全省平均值的3.74倍[44]。同时果园中普遍施用有机肥达20625 kg hm-2,也导致土壤Cu的富集[44]。Cu是家畜饲料的微量元素添加剂,帮助家畜防病抗菌。然而,饲料中添加的Cu并不能被家畜完全吸收,绝大部分的Cu随着粪便被排出体外,导致有机肥中Cu含量较高[45,46];根据王飞等[47]的研究,华北地区禽畜粪便有机肥中的Cu平均值达到69.22 mg kg-1。综上,因子3指示农业活动因素。Lu等对北京顺义区[48]、Sun等对东北黑土区[49]的研究均发现土壤中Cu污染来自于农药和化肥施用,与本文相一致。
因子4对Hg的贡献为75.7%,对其他元素的贡献均小于5%。烟台海岸带有众多燃煤工厂和发电厂,Hg是煤炭中易挥发的组分,接近100%的Hg在燃烧过程中进入烟道气[50,51]。除尘器对氧化态汞(Hg2+)和颗粒态Hg的除尘效率较高;但单质汞(Hg)极难溶于水,很难被除尘器吸收,进而会逸入大气[52,53],进一步被氧化后通过干湿沉降进入土壤。值得注意的是,研究区金矿较多,历史上曾采用混汞法工艺来提纯黄金,在制备汞齐和煅烧汞齐两个阶段不可避免的会造成Hg蒸气的散逸[54],且主要是Hg;尽管混汞法早已于1996年被禁止,但对土壤Hg的影响可遗留至今。因此,因子4代表煤炭燃烧和混汞法提金所排放汞的大气沉降。Cai等[55]、Lv等[19]、Wang等[56]均发现Hg在多元受体模型分析中为孤立的元素,来自于人为排放的大气沉降。
3.3 烟台海岸带土壤重金属空间分布
将烟台海岸带土壤重金属数据进行高斯变换,得到符合正态分布的变量,利用ICA对高斯变量进行分析,得到10个ICs。在理论变异函数拟合过程中,多个基本变异函数套合可以精细化的表征变量的空间结构[38, 57]。本文尝试用块金效应(Nugget)和2个球状模型(Spherical)对ICs进行拟合(表3)。IC1、IC2、IC3、IC4、IC6、IC9和IC10均可用块金效应和2个球状模型拟合;IC5、IC7和IC8只能用块金效应和1个球状模型拟合,另外1个球状模型被舍弃。IC4、IC7和IC8的块金值/总方差值均低于0.25,表现出强烈的空间相关性;其余7个ICs的块金值/总方差值介于0.25和0.75之间,显示出中度空间相关性。Tab. 3
表3
表3独立成分的理论变异函数拟合参数
Tab. 3
变量 | 模型结构 | 块金值 | 结构1 | 结构2 | 块金值/总方差 | |||
---|---|---|---|---|---|---|---|---|
基台值 | 变程(m) | 基台值 | 变程(m) | |||||
IC1 | Nugget+Spherical+Spherical | 0.31 | 0.26 | 7195 | 0.46 | 29307 | 0.30 | |
IC2 | Nugget+Spherical+Spherical | 0.38 | 0.10 | 8299 | 0.47 | 29457 | 0.40 | |
IC3 | Nugget+Spherical+Spherical | 0.46 | 0.32 | 13513 | 0.35 | 37496 | 0.41 | |
IC4 | Nugget+Spherical+Spherical | 0.25 | 0.59 | 8674 | 10.08 | 1661216 | 0.02 | |
IC5 | Nugget+Spherical | 0.68 | 0.25 | 18673 | 0.73 | |||
IC6 | Nugget+Spherical+Spherical | 0.38 | 0.30 | 7733 | 0.28 | 25534 | 0.40 | |
IC7 | Nugget+Spherical | 0.65 | 2.73 | 548386 | 0.19 | |||
IC8 | Nugget+Spherical | 0.26 | 0.88 | 19044 | 0.23 | |||
IC9 | Nugget+Spherical+Spherical | 0.50 | 0.22 | 4317 | 0.30 | 20506 | 0.49 | |
IC10 | Nugget+Spherical+Spherical | 0.55 | 0.27 | 7141 | 0.30 | 61258 | 0.49 |
新窗口打开|下载CSV
图3为烟台海岸带土壤中10种重金属的空间分布图。可以看出,归属于同一PMF因子的重金属具有相似的空间分布格局。Co、Cr、Mn和Ni的空间分布与成土母质相一致,其高值区主要分布在玄武岩和变质岩发育的土壤,低值区主要分布在第四系和花岗岩发育的土壤(图3c、3d、3g、3h和图1b)。玄武岩富含辉石等铁镁质矿物,形成的土壤自然具有较高的Co、Cr、Mn和Ni含量。研究区的变质岩原岩主要为超镁铁质—镁铁质火山岩、长英质火山岩和碎屑沉积岩[58,59],在变质过程中仍然保持着超基性岩—基性岩的特征,因而铁族元素含量较高。As在研究区中部和北部呈现出两个高值区,含量超过18.9 mg kg-1(图3a),明显高于背景值;两个高值区的母岩主要为元古代粉子山群的片岩、石英岩[60];片岩中的As含量是玄武岩、花岗岩的10倍以上[61],因此As的高值区主要归因于自然背景。
图3
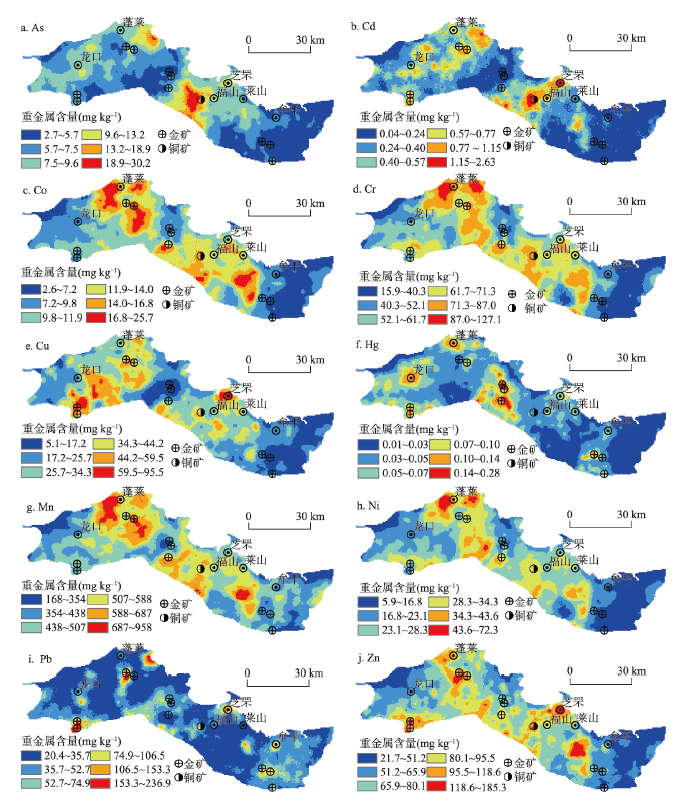
图3烟台海岸带土壤重金属含量空间分布
Fig. 3Spatial distribution of heavy metals concentrations in soils of Yantai coastal zone
Cd、Pb和Zn的空间分布较为零散,与成土母质分布没有明显的相关性;这3种重金属的高值区主要分布在金矿、铜矿的尾矿区以及烟台老城区(图3b、3i、3j),其空间分布受到矿山开采和交通排放的显著影响。Cu的高值区主要分布在西部的果园土壤中,主要受含铜杀菌剂和有机肥施用的影响(图3e、图1c);同时在烟台老城区有显著的高值区,说明土壤中Cu也受到工业活动和交通排放的影响。Hg的高值区主要分布于中部区域及西部的蓬莱、龙口市区周边(图3f)。研究区中部有多个金矿,历史上混汞法提金导致的Hg散逸形成了土壤Hg的高值区;同时,蓬莱和龙口市区燃煤电厂和工厂众多,煤炭燃烧也会导致土壤Hg的富集。
3.4 烟台海岸带土壤重金属潜在污染区域划定
根据地累积指数[62],将1.5倍的山东东部土壤背景值作为污染阈值;As、Cd、Co、Cr、Cu、Hg、Mn、Ni、Pb和Zn的污染阈值分别为9.5 mg kg-1、0.16 mg kg-1、16.5 mg kg-1、84.3 mg kg-1、29.4 mg kg-1、0.044 mg kg-1、828 mg kg-1、35.3 mg kg-1、38.1 mg kg-1和84.2 mg kg-1。分别设置0.8、0.85、0.9和0.95为临界概率,同时对应用4种污染临界概率所划定的潜在污染区域开展空间不确定性分析;研究发现,联合概率值随着临界概率值的增大而增大;当将临界概率设置为0.95时,所有重金属元素的联合概率值均达到0.8以上,可以产生可靠的潜在污染范围,这跟Zhao[25]、Lv等[38]的研究相一致。图4显示了烟台海岸带土壤10种重金属的潜在污染区域。Cu的潜在污染区域面积最大,达到1484 km2,占研究区总面积的37.5%;主要是面积广阔的果园施用波尔多液所导致(图4e)。Hg的潜在污染区域主要分布在金矿和城市周边(图4f),面积为565 km2,占总面积的14.3%。Cd的潜在污染区域面积为342 km2,占总面积的8.6%,主要分布在工业区、铜矿尾矿和城市周边(图4b)。尽管Pb和Zn的来源受到人类活动的影响,但潜在污染区域范围较小(图4i~4j),主要受点源式工矿企业污染的影响。As、Co、Cr和Ni的潜在污染区域主要与成土母质的高背景值有关(图4a、4c、4d、4h和图1b),Mn没有展示出潜在污染区域(图4g)。总的来看,土壤中Cu、Hg和Cd需要引起足够警惕,这3种元素的潜在污染区域已经连接成片;同时,要防止Pb和Zn污染区域的扩展。图4
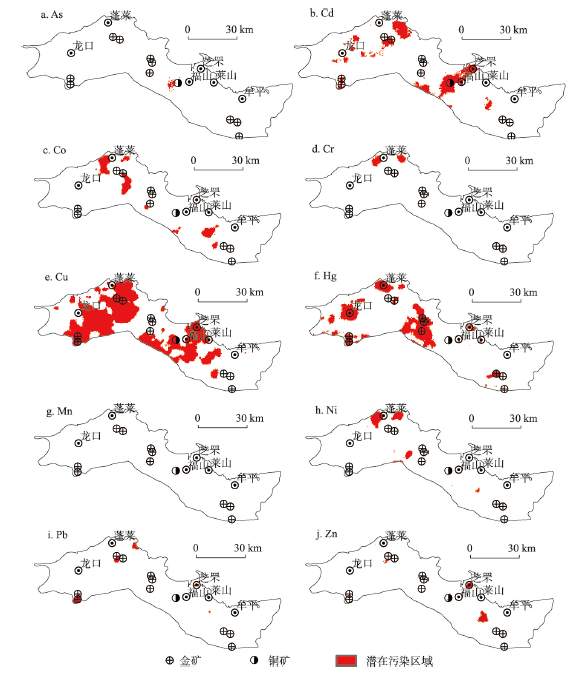
图4烟台海岸带土壤重金属潜在污染区域
Fig. 4Potential polluted areas of heavy metals in soils of Yantai coastal zone
4 结论
(1)烟台海岸带土壤中As、Cd、Co、Cr、Cu、Hg、Ni、Pb和Zn的平均含量超过山东东部土壤背景值;所有元素(除不具可比值的元素)的平均值均低于农用地和第一类建设用地土壤污染风险筛选值;富集系数表明,Cd、Cu、Hg、Pb和Zn呈现出明显的富集,尤其是Cd、Hg和Pb。(2)PMF获取了4个来源。As、Co、Cr、Mn和Ni主要受到成土母质的控制,为自然来源;Cd、Pb和Zn受到工业和交通排放以及成土母质的共同影响;Cu主要来自于波尔多液和有机肥施用等农业活动;Hg则主要来源于混汞法炼金和煤炭燃烧所排放Hg的大气沉降。
(3)本文提出了基于ICA和SGS的多元地统计模拟技术,并成功应用到烟台海岸带土壤重金属空间预测研究。As、Co、Cr、Mn和Ni的空间分布受到成土母质的控制,果园土壤呈现出Cu的高值区,Cd、Pb和Zn的污染热点主要分布在在金、铜矿的尾矿区以及烟台市区,Hg的高值区分布在金矿以及龙口、蓬莱市区周边。
(4)Cu、Hg和Cd的潜在污染区域面积分别达到了1484 km2、565 km2和342 km2,为污染分布最广的3种元素;这些元素的潜在污染区域已连续成片分布,需引起警惕。
参考文献 原文顺序
文献年度倒序
文中引用次数倒序
被引期刊影响因子
[本文引用: 2]
[本文引用: 1]
[本文引用: 1]
[本文引用: 2]
[本文引用: 2]
[本文引用: 1]
[本文引用: 2]
[本文引用: 2]
[本文引用: 1]
[本文引用: 1]
[本文引用: 1]
[本文引用: 1]
DOI:10.1016/j.envpol.2006.01.045URLPMID:16580763 [本文引用: 1]

In this work the content of seven heavy metals (Cd, Cr, Cu, Hg, Ni, Pb and Zn) and other parameters (the pH, organic matter, carbonates and granulometric fraction) in agricultural topsoil in the Ebro basin are quantified, based on 624 samples collected according to an 8 by 8 km square mesh. The average concentrations (mg/kg) obtained were: Cd 0.415+/-0.163, Cr 20.27+/-13.21, Cu 17.33+/-14.97, Ni 20.50+/-22.71, Pb 17.54+/-10.41, Zn 17.53+/-24.19 and Hg 35.6+/-42.05 microg/kg. The concentration levels are relatively low in areas of high pH and low organic matter content concentration. The results of factor analysis group Cd, Cu, Hg, Pb and Zn in F1 and Cr y Ni in F2. The spatial heavy metals component maps based on geostatistical analysis, show definite association of these factors with the soil parent material. The local anomalies (found in Cu, Zn and Pb) are attributed to anthropogenic influence.
[本文引用: 1]
[本文引用: 1]
[本文引用: 1]
DOI:10.1016/j.envpol.2018.09.147URLPMID:30321714 [本文引用: 2]

Absolute principal component score/multiple linear regression (APCS/MLR) and positive matrix factorization (PMF) were applied to a dataset consisting of 10 heavy metals in 300 surface soils samples. Robust geostatistics were used to delineate and compare the factors derived from these two receptor models. Both APCS/MLR and PMF afforded three similar source factors with comparable contributions, but APCS/MLR had some negative and unidentified contributions; thus, PMF, with its optimal non-negativity results, was adopted for source apportionment. Experimental variograms for each factor from two receptor models were built using classical Matheron's and three robust estimators. The best association of experimental variograms fitted to theoretical models differed between the corresponding APCS and PMF-factors. However, kriged interpolation indicated that the corresponding APCS and PMF-factor showed similar spatial variability. Based on PMF and robust geostatistics, three sources of 10 heavy metals in Guangrao were determined. As, Co, Cr, Cu, Mn, Ni, Zn, and partially Hg, Pb, Cd originated from natural source. The factor grouping these heavy metals showed consistent distribution with parent material map. 43.1% of Hg and 13.2% of Pb were related to atmosphere deposition of human inputs, with high values of their association patterns being located around urban areas. 29.6% concentration of Cd was associated with agricultural practice, and the hotspot coincided with the spatial distribution of vegetable-producing soils. Overall, natural source, atmosphere deposition of human emissions, and agricultural practices, explained 81.1%, 7.3%, and 11.6% of the total of 10 heavy metals concentrations, respectively. Receptor models coupled with robust geostatistics could successfully estimate the source apportionment of heavy metals in soils.
DOI:10.1016/j.chemosphere.2017.10.151URLPMID:29131977 [本文引用: 1]

Hexi Corridor is the most important base of commodity grain and producing area for cash crops. However, the rapid development of agriculture and industry has inevitably led to heavy metal contamination in the soils. Multivariate statistical analysis, GIS-based geostatistical methods and Positive Matrix Factorization (PMF) receptor modeling techniques were used to understand the levels of heavy metals and their source apportionment for agricultural soil in Hexi Corridor. The results showed that the average concentrations of Cr, Cu, Ni, Pb and Zn were lower than the secondary standard of soil environmental quality; however, the concentrations of eight metals (Cr, Cu, Mn, Ni, Pb, Ti, V and Zn) were higher than background values, and their corresponding enrichment factor values were significantly greater than 1. Different degrees of heavy metal pollution occurred in the agricultural soils; specifically, Ni had the most potential for impacting human health. The results from the multivariate statistical analysis and GIS-based geostatistical methods indicated both natural sources (Co and W) and anthropogenic sources (Cr, Cu, Mn, Ni, Pb, Ti, V and Zn). To better identify pollution sources of heavy metals in the agricultural soils, the PMF model was applied. Further source apportionment revealed that enrichments of Pb and Zn were attributed to traffic sources; Cr and Ni were closely related to industrial activities, including mining, smelting, coal combustion, iron and steel production and metal processing; Zn and Cu originated from agricultural activities; and V, Ti and Mn were derived from oil- and coal-related activities.
DOI:10.1016/j.chemosphere.2015.01.048URLPMID:25698100 [本文引用: 1]

Because heavy metals (HMs) occurring naturally in soils accumulate continuously due to human activities, identifying and apportioning their sources becomes a challenging task for pollution prevention in urban environments. Besides the enrichment factors (EFs) and principal component analysis (PCA) for source classification, the receptor model (Absolute Principal Component Scores-Multiple Linear Regression, APCS-MLR) and Pb isotopic mixing model were also developed to quantify the source contribution for typical HMs (Cd, Co, Cr, Cu, Mn, Ni, Pb, Zn) in urban park soils of Xiamen, a representative megacity in southeast China. Furthermore, distribution patterns of their concentrations and sources in 13 soil profiles (top 20 cm) were investigated by different depths (0-5, 5-10, 10-20 cm). Currently the principal anthropogenic source for HMs in urban soil of China is atmospheric deposition from coal combustion rather than vehicle exhaust. Specifically for Pb source by isotopic model ((206)Pb/(207)Pb and (208)Pb/(207)Pb), the average contributions were natural (49%)>coal combustion (45%)>>traffic emissions (6%). Although the urban surface soils are usually more contaminated owing to recent and current human sources, leaching effects and historic vehicle emissions can also make deep soil layer contaminated by HMs.
[本文引用: 1]
[本文引用: 3]
[本文引用: 3]
URLPMID:23973471 [本文引用: 3]
[本文引用: 2]
[本文引用: 3]
[本文引用: 1]
DOI:10.1016/j.envpol.2015.12.030URLPMID:26745395 [本文引用: 2]

This work develops a new approach for delineating sites that are contaminated by multiple soil heavy metals and applies it to a case study. First a number of contaminant sample data are transformed into multiple spatially un-correlated factors using Uniformly Weighted Exhaustive Diagonalization with Gauss iterations (U-WEDGE). Sequential Gaussian simulation (sGs) is then used to generate sets of realizations of each resultant factor. These are then transformed into sets of sGs contaminant distribution realizations, which are then used to analyze the local and spatial (global) uncertainties in the distribution and concentration of contaminants via joint simulation. Finally, Info-Gap Decision Theory (IGDT) is used to consider different monitoring and or remediation regimes based on the analysis of contaminant realization spatial uncertainty. In our case study each heavy metal contaminant was considered individually and together with all other heavy metals; as the number of heavy metals considered increased, higher critical proportion values of local probability were chosen to obtain a low global uncertainty (to provide high reliability). Info-Gap Decision Theory (IGDT) yielded the most appropriate critical proportion values which minimized information loss in terms of specific goals. When the false negative rate is set to zero, meaning that it is necessary to monitor all potentially polluted areas, the corresponding false positive rates are at least 63%, 65%, 66%, 68%, 70%, and 78% to yield robustness levels of 0.50, 0.60, 0.70, 0.80, 0.90, and 1.00 respectively. However, when the false negative rate tolerance threshold is raised to 50%, the false positive rate tolerance which yields robustness levels of 0.50, 0.60, 0.70, 0.80, 0.90 and 1.00 drop to 12%, 14%, 15%, 18%, 20%, and 39%. The case study demonstrates the effectiveness of the developed approach at making robust decisions concerning the delineation of sites contaminated by multiple heavy metals.
[本文引用: 1]
[本文引用: 3]
[本文引用: 2]
[本文引用: 2]
DOI:10.1016/j.envpol.2003.07.001URLPMID:14568722 [本文引用: 1]

Mapping the spatial distribution of soil pollutants is essential for delineating contaminated areas. Currently, geostatistical interpolation, kriging, is increasingly used to estimate pollutant concentrations in soils. The kriging-based approach, indicator kriging (IK), may be used to model the uncertainty of mapping. However, a smoothing effect is usually produced when using kriging in pollutant mapping. The detailed spatial patterns of pollutants could, therefore, be lost. The local uncertainty of mapping pollutants derived by the IK technique is referred to as the conditional cumulative distribution function (ccdf) for one specific location (i.e. single-location uncertainty). The local uncertainty information obtained by IK is not sufficient as the uncertainty of mapping at several locations simultaneously (i.e. multi-location uncertainty or spatial uncertainty) is required to assess the reliability of the delineation of contaminated areas. The simulation approach, sequential indicator simulation (SIS), which has the ability to model not only single, but also multi-location uncertainties, was used, in this study, to assess the uncertainty of the delineation of heavy metal contaminated soils. To illustrate this, a data set of Cu concentrations in soil from Taiwan was used. The results show that contour maps of Cu concentrations generated by the SIS realizations exhausted all the spatial patterns of Cu concentrations without the smoothing effect found when using the kriging method. Based on the SIS realizations, the local uncertainty of Cu concentrations at a specific location of x', refers to the probability of the Cu concentration z(x') being higher than the defined threshold level of contamination (z(c)). This can be written as Prob(SIS)[z(x')>z(c)], representing the probability of contamination. The probability map of Prob(SIS)[z(x')>z(c)] can then be used for delineating contaminated areas. In addition, the multi-location uncertainty of an area A,delineated as contaminated based on the probability map of Prob(SIS)[z(x')>z(c)], can be calculated to assess the reliability of delineation. Multi-location uncertainty refers to the probability of Cu concentrations in several locations, x'(1), x'(2), em leader, x'(m,) in the area A, being higher than the threshold (z(c)) as denoted by Prob(SIS)[z(x'(1))>z(c), z(x'(2))>z(c), em leader, andz(x'(m))>z(c)] or Prob(SIS)[z(A)>z(c)]. The multi-location uncertainty Prob(SIS)[z(A)>z(c)], obtained from the SIS, can be used to assess the reliability of delineation for regions suspected of contamination, (A), which has been delineated as contaminated. Reliance on this information facilitates the decision making process in determining which areas are contaminated and require cleanup action.
[本文引用: 2]
[本文引用: 2]
[本文引用: 1]
[本文引用: 1]
[本文引用: 1]
DOI:10.1016/j.chemosphere.2018.02.122URLPMID:29524824 [本文引用: 1]

The Qinghai-Tibet Plateau, especially the northeastern region, is not a pure land any more due to recently increasing anthropogenic activities. This study collected soil samples from 70 sites of the northeastern Qinghai-Tibet Plateau to evaluate pollution, ecological-health risks, and possible pollution sources of heavy metals. The concentrations of heavy metals in soil were relatively high. Values of geo-accumulation index exhibited that Hg pollution was the most serious meanwhile Hg possessed the strongest enrichment feature based on enrichment factor values. The modified degrees of contamination showed that about 54.3% and 17.1% of sampling sites were at moderate and high contamination degree while pollution load indexes illustrated that 72.9% and 27.1% of sampling sites possessed moderate and high contamination level, respectively. Ecological risk indexes of heavy metals in soil ranged from 234.6 to 3759.0, suggesting that most of sites were under considerable/very high risks. Cancer risks for adults and children were determined as high and high-very high levels while non-cancer risks for children were high although those for adults were low. Industrial source contributed to the main fraction of ecological and health risks. Summarily speaking, heavy metals in soil of the study area has caused significantly serious pollution and exerted high potential ecological and health risks, especially for children who are more susceptible to hurt from pollutants. Therefore, more efficient and strict pollution control and management in study area should be put out as soon as possible.
DOI:10.1007/s11356-018-3836-7URLPMID:30488244 [本文引用: 1]

Variability of background concentration of toxic trace metal(loid)s in sediments can often lead to under/over-report of contamination level, even in detailed scale. In this study, both surface (5-10 cm) and subsurface (> 10 cm) sediments were collected at many sites in a small lake (0.528 km(2)) with multi-function (irrigation, aquaculture, and watercourse) in an industrial area. Total concentration of trace metal(loid)s (Cd, Cr, Co, Cu, Ni, Sb, Pb, and Zn) and potential reference elements (Ti, Zr, Rb, and Li) were analyzed. The results showed that although the trace metal(loid)s were mainly lithogenic in subsurface sediments, the variability of baseline concentration was significant. For Sb, this variability was a result of alteration in hydrological parameters as well as sediment properties including Fe/Mn oxide contents, particle size distribution, and organic matter contents. Comparison of the normalized Sb concentration in samples from two sediment cores indicated that Ti is the best reference element for normalizing Sb to reduce the impact from particle size and natural source. Enrichment assessment using modified EFs (Ti as reference element) and Igeo index (measured baseline concentration) suggested that about 70% of the surface sediments were at least moderately polluted by Sb in the lake, as a result of recent anthropogenic input, mainly from nearby industries, e.g., concrete factory and textile factory. Modified EFs should be used, instead of Igeo index, when Sb enrichment was relatively low in sediment. The anomalies of Sb background concentration may need regulator attention when assessing the level of sediment contamination.
[本文引用: 1]
[本文引用: 1]
[本文引用: 1]
[本文引用: 1]
[本文引用: 1]
DOI:10.1016/j.envpol.2019.02.045URLPMID:30818114 [本文引用: 1]

Contamination of agricultural soil by heavy metals has become a global issue concerning food security and human health risk. In this study, a soil investigation was conducted to evaluate metals accumulation, potential ecological and health risks as well as to identify sources of metals in paddy soils in Hanzhong City, which is located in a sedimentary basin. Ninety-two (92) surface soil samples (bulk soil) and their corresponding rice samples, 21 irrigation water samples, and 18 fertilizer samples were collected from two typical counties and quantified for the heavy metals (i.e., As, Cd, Cu, Hg, Pb, and Zn) concentrations. The results showed that As, Cd, and Zn were the main contaminants in soils in the studied area. Additionally, elevated Hg content in soils might also pose risks to the local ecosystem. Cadmium and As demonstrated high mobility, and their average contents in rice grains were slightly higher than the permissible threshold (0.20mgkg(-1)). Moreover, Pb, As, and Cd intake via rice consumption might result in potential risks to local residents. Metal distribution revealed that pollution in the studied area is non-homogeneous, and agricultural activities (As, Cu, and Cd), transportation emission (Cu and Pb), coal combustion (Hg and As), and smelting activities (Zn, Pb, and Cu) were ascertained as the potential sources based on the Positive matrix factorization (PMF) analysis results.
[本文引用: 3]
[本文引用: 1]
[本文引用: 1]
[本文引用: 1]
[本文引用: 1]
[本文引用: 1]
[本文引用: 1]
URLPMID:32058225 [本文引用: 1]
DOI:10.1016/j.envint.2019.105239URLPMID:31639603 [本文引用: 1]

Conducting integrated analysis of the source, exposure and health risk of heavy metals is critical for developing mitigation strategies of soil contamination. Taking the former electronic waste (e-waste) dismantling center in China as an example this study quantitatively apportioned source contribution of soil heavy metals in this area by statistical analysis and positive matrix factorization (PMF) model. Furthermore, the human health risk of identified sources were quantified by combining source profiles and exposure risk assessment. The seven heavy metals investigated were arsenic (As), cadmium (Cd), copper (Cu), chromium (Cr), nickel (Ni), lead (Pb) and Zinc (Zn). Results indicated that agricultural soils were mainly contaminated with Cd and Cu. Parent material and pesticide, fertilizer application, industrial discharge, and vehicle emission accounted for 46.6, 22.2, and 31.2%, respectively, of the accumulation of metals in the soil. Moreover, these sources contributed 52.9, 19.0, and 28.1%, respectively of the total non-cancer risk. For the total cancer risk, the contribution of these three sources was 39.2, 45.3, and 15.5%, respectively. Despite that industrial discharge contributed the least to the accumulation of metals (22.2%), it contributed the most to the total cancer risk (45.3%). Reducing industrial emission was crucial for minimizing the heavy metal input to agricultural soils and preventing potential health hazard. These findings could provide support for environmental protection authority to improve the management and risk prevention of contaminated farmland.
[本文引用: 2]
[本文引用: 2]
URL [本文引用: 1]

Brassica chinensis L.), soil microbial biomass carbon, and soil enzymes (dehydrogenase, urease, and acid phosphatase) activities. The results showed that pig manure application increased the aboveground biomass of pakchoi significantly, but had no significant effects on the aboveground biomass Cu concentration. Under the application of pig manure, soil dehydrogenase and urease activities increased in a definite period of time, but their activities as well as soil acid phosphatase activity were depressed gradually with the increase of soil Cu concentration. When the soil total Cu concentration reached 301.3 mg·kg-1, the aboveground biomass of pakchoi, soil microbial biomass carbon, and the activities of dehydrogenase, urease, and acid phosphatase were greatly reducd. The aboveground biomass Cu concentration of pakchoi had significant positive correlations with the concentration of soil total and water soluble Cu (P<0.01), while the aboveground biomass was negatively correlated with soil total Cu concentration (P<0.05). It was suggested that the threshold value of Cu in black soil under vegetable cultivation should be less than 301.3 mg·kg-1.]]>
[本文引用: 1]
[本文引用: 1]
[本文引用: 1]
URL [本文引用: 1]

At present, commercial organic fertilizer in China generally exists a problem that heavy metal content exceeds standard limitation. In this study, we took the main field for agricultural products in North China as the research scope. In order to get the situation of heavy metal pollution of commercial organic fertilizer in North China, we investigated and analyzed the commercial organic fertilizer samplings. By using method of principal component analysis, we present a preliminary discussion of the heavy metal sources. We are also clear about an important factor affecting the heavy metal contamination of organic fertilizer products in North China. The results showed that the average content of heavy metal Cd, Cr, Cu, Pb, Zn, Ni, As, Hg in commercial organic fertilizer used by agricultural products producing area in North China were 0.21、45.42、69.22、87.40、274.58、16.50、3.21、0.33 mg/kg. According to the China's organic fertilizer industry standard, the content of Pb exceeded the rate of up to 80.56%, the rest of the heavy metal content is not ovenproof. However, according to the German rotten compost standards, most of the heavy metal content exceeds standard limitation, the content of Cr, Cu, Pb, Zn, Ni, Hg exceeds the rate of 8.33%, 13.89%, 16.67%, 19.44%, 2.78% and 11.11% respectively, yet the content of Cd is not ovenproof. The results of principal component analysis showed that the contribution rates of the first three principal components were 34.7%, 16.6%, 13.0%, which can explain 64.3% of total variables and reflected most of the original data information. Combining with the characteristics of raw materials of commercial organic fertilizer and process analysis, we find that the major source of heavy metal pollution in commercial organic fertilizer in the agricultural regions of North China can be feed additives with high content of Pb, Hg, additives with phosphate fertilizer, and feed additives with high content of Cu, Zn. Meanwhile, the average content of heavy metal Cd, Cr, Cu, Pb, Zn, Ni, As, Hg in farm feed used by agricultural products producing area in North China were 0.02, 13.89, 87.027, 6.32, 297.90, 23.00, 0.65, 0.01mg kg-1. Furthermore, correlation analysis of heavy metal content in the feed and organic fertilizer results showed that, the contents of most of heavy metal in the fertilizer had significant positive correlation with their contents in the feed except Ni and As. This determination results confirmed the above view of the main sources of heavy metals. However, from the result of principal component analysis, other components can still explain 35.7% of the total variable, which indicated that there were other sources of heavy metals in organic fertilizer. Binder is a necessary add material in commercial organic fertilizer production which conclude attapulgite, bentonite, and phosphogypsum. Studies have shown that the common binder contained higher heavy metal. Therefore, binder may be one of the sources of heavy metal in organic fertilizer. In addition, the environmental factors such as atmospheric dust fall and traffic pollution related to the organic fertilizer production process and the background values of heavy metals in the soil may affect the heavy metal contents of organic fertilizer, which needs to be further studied.
[本文引用: 1]
DOI:10.1016/j.scitotenv.2012.03.003URL [本文引用: 1]
DOI:10.1016/j.chemosphere.2013.02.063URL [本文引用: 1]

The characterization of the content and source of heavy metals in soils are necessary to establish quality standards on a regional level and to assess the potential threat of metals to food safety and human health. The surface horizons of 114 agricultural soils in Dehui, a representative agricultural area in the black soil region, Northeast China, were collected and the concentrations of Cr, Ni, Cu, Zn, and Pb were analyzed. The mean values of the heavy metals were 49.7 +/- 7.04, 20.8 +/- 3.06, 18.9 +/- 8.51, 58.9 +/- 7.16, and 35.4 +/- 9.18 mg kg(-1) for Cr, Ni, Cu, Zn, and Pb, respectively. Anthropic activities caused an enrichment of Cu and Pb in soils. However, metal concentrations in all samples did not exceed the guideline values of Chinese Environmental Quality Standard for Soils. Multivariate and geostatistical analyses suggested that soil Cr, Ni, and Zn had a lithogenic origin. Whereas, the elevated Cu concentrations in the study area were associated with industrial and agronomic practices, and the main sources of Pb were industrial fume, coal burning exhausts, and domestic waste. Source identification of heavy metals in agricultural soil is a basis for undertaking appropriate action to reduce metal inputs. (C) 2013 Elsevier Ltd.
[本文引用: 1]
[本文引用: 1]
DOI:10.1021/es062639uURLPMID:16568746 [本文引用: 1]
[本文引用: 1]
[本文引用: 1]
[本文引用: 1]
DOI:10.1016/j.ecoenv.2018.10.092URLPMID:30388535 [本文引用: 1]

To investigate contamination level, origins and spatial distribution characteristics of heavy metals (Cu, Pb, Zn, Hg, Ni, Cd, As, and Cr) in agricultural soils of Gaogang Town, a typical industrial transfer-undertaking region of the Pearl River Delta (PRD), China, a total of 162 surface soil samples were collected in August 2016 and determined using inductively coupled plasma mass spectrometry, inductively coupled plasma optical emission spectrometry and atomic fluorescence spectrometry. Moreover, heavy metals contents were systematically analyzed by pollution index, enrichment factor, multivariate statistical approaches and geostatistical analysis. The results showed that the mean concentrations of Cd, Pb, Zn, Ni, Cu and Hg were higher than the soil background values of Guangdong Province, and the relatively high values of pollution index and enrichment factor indicated that these elements (Cd, Pb, Zn and Hg) had cumulative trends in soil. All results of multivariate statistical approaches and geostatistical analysis showed that pollution were heavily distributed in areas of industries, river and dense road network. The eight heavy metals in agricultural soils of Gaogang Town came from three different sources. Arsenic, Cr, Cu and Ni arose mainly from parent materials. Agricultural practices and traffic activities were the main sources of Cd, Pb and Zn. Mercury mainly came from industrial practices.
DOI:10.1016/j.chemosphere.2019.125266URLPMID:31896197 [本文引用: 1]

Determining the reliable source contribution and spatial distribution of potentially toxic elements (PTEs) is a focal issue for soil regulation and remediation. For this purpose, three receptor models, US-EPA positive matrix factorization (EPAPMF), weighted alternating least squares positive matrix factorization (WALSPMF), and non-negative constrained absolutely principle analysis (NCAPCA), were used to a dataset consisting of ten PTEs (As, Cd, Co, Cr, Cu, Hg, Mn, Ni, Pb, and Zn) for source apportionment. Hazardous areas of ten PTEs were delineated using sequential indicator simulation (SIS) and uncertainty analysis. Three factors for ten PTEs were derived by three receptor models with a one-to-one correspondence between the factors. To obtain more appropriate results, the three receptor models were combined to calculate the ensemble-average source contributions. As, Co, Cr, Cu, Mn, and Ni were derived from a natural source with ensemble-average contributions higher than 85.72%. Cd, Hg, Pb, and Zn were contributed by both parent material and anthropogenic influence. More than half of Hg concentrations were associated with atmospheric deposition caused by human emissions. The concentrations of 28.04% for Cd, 20.74% for Hg, 43.49% for Pb, and 23.71% for Zn were associated with human inputs including agriculture practice, industrial activities, and vehicle emissions. The maps of spatial distribution generated by the SIS indicated that parent materials controlled the spatial distributions of As, Cd, Co, Cr, Cu, Mn, Ni, Pb, and Zn. 27.1% and 32.1% of the total area for Cd and Hg were identified as hazardous areas exceeding 1.5 times background values of Shandong province.
DOI:10.1016/j.jhazmat.2016.01.026URLPMID:26808251 [本文引用: 1]

Mercury pollution is a global environmental problem that has serious implications for human health. One of the most important sources of anthropogenic mercury emissions are coal-burning power plants. Hg accumulations in soil are associated with their atmospheric deposition. Our study provides the first assessment of soil Hg on the entire Spanish surface obtained from one sampling protocol. Hg spatial distribution was analysed with topsoil samples taken from 4000 locations in a regular sampling grid. The other aim was to use geostatistical techniques to verify the extent of soil contamination by Hg and to evaluate presumed Hg enrichment near the seven Spanish power plants with installed capacity above 1000 MW. The Hg concentration in Spanish soil fell within the range of 1-7564 mug kg(-1) (mean 67.2) and 50% of the samples had a concentration below 37 mug kg(-1). Evidence for human activity was found near all the coal-fired power plants, which reflects that metals have accumulated in the basin over many years. Values over 1000 mug kg(-1) have been found in soils in the vicinity of the Abono, Soto de Ribera and Castellon power plants. However, soil Hg enrichment was detectable only close to the emission source, within an approximate range of only 15 km from the power plants. We associated this effect with airborne emissions and subsequent depositions as the potential distance through fly ash deposition. Hg associated with particles of ash tends to be deposited near coal combustion sources.
[本文引用: 1]
[本文引用: 1]
[本文引用: 1]
[本文引用: 1]
[本文引用: 1]
[本文引用: 1]
[本文引用: 1]
[本文引用: 1]
[本文引用: 1]