HTML
--> --> -->To improve observation coverage, tailor-made satellites have been developed to provide atmospheric greenhouse gas (GHG) measurements at unprecedented precision. The Japanese GHG monitoring satellite mission, Greenhouse Gases Observing Satellite (GOSAT), was launched in 2009 (Kuze et al., 2009), and the U.S. satellite mission, Orbiting Carbon Observatory 2 (OCO-2), was launched in 2014 (Crisp et al., 2017).
Satellite measurements are advantageous for constraining top-down flux inversions because they provide continuous global observation coverage. Although satellite measurements are not as accurate as ground-based measurements, the increased spatial coverage can provide additional information not available from sparse surface networks (Buchwitz et al., 2007), and such data have been used in surface carbon flux inversion studies (Peylin et al., 2013; Saeki et al., 2013; Jiang et al., 2016; Chevallier et al., 2019; Wang et al., 2020). The CO2 column-averaged dry-air mole fractions (XCO2) measured by GOSAT and OCO-2 have been applied in regional carbon flux optimization studies (Basu et al., 2013; Maksyutov et al., 2013; Feng et al., 2017; Chevallier et al., 2019; Wang et al., 2020).
Recent studies have shown that the accuracy of inversion results inferred from the GOSAT and OCO-2 retrievals is comparable to traditional inversions using accurate but sparse surface networks, indicating that satellite GHG observations provide valuable complementary data for global carbon budget studies (Chevallier et al., 2019; Wang et al., 2020). Assimilation of satellite GHG observations has significantly changed or raised questions about our understanding of spatiotemporal patterns of surface CO2 fluxes. For example, GOSAT inversion data suggest high European biospheric uptake, nearly twice that suggested by in situ-only studies (Houweling et al., 2015; Feng et al., 2016; Reuter et al., 2017), which would modify the global carbon flux distribution (Houweling et al., 2015). Assimilated GOSAT and OCO-2 XCO2 measurements have also revealed unexpectedly high net emissions from tropical Africa, which are thought to be mainly caused by substantial land-use change, resulting in the release of carbon from large soil organic carbon stores (Palmer et al., 2019).
The Chinese Global Carbon Dioxide Monitoring Scientific Experimental Satellite (TanSat), funded by the Ministry of Science and Technology of China, the Chinese Academy of Sciences, and the China Meteorological Administration, was launched in December 2016 (Liu and Yang, 2016; Ran et al., 2019). The first global XCO2 map measured by TanSat was reported in a previous study (Yang et al., 2018), and Total Column Carbon Observing Network validation shows 2.2 ppm accuracy for version 1 of the TanSat L2 data product (Liu et al., 2018). The accuracy and precision of TanSat XCO2 retrievals were further improved using a wavelength dependence gain factor to correct the spectrum continuum feature (Yang et al., 2020). A new version of TanSat XCO2 was recently released to the public (Yang et al., 2021), which provides an opportunity to improve our knowledge of global carbon flux from flux inversions based on TanSat measurements.
In this study, we introduce the first estimate of global carbon flux based on TanSat global measurements. The following section shows the TanSat measurements and methods used in the flux inversion. Section 3 introduces the main results of the optimized carbon flux. We then close with our conclusions.
2.1. TanSat measurement
TanSat flies in a sun-synchronous low Earth orbit (LEO) with an equator crossing time around 1330 local time and operates in three observation modes including nadir (ND), glint (GL), and target modes. ND mode is used when TanSat flies over land surfaces, and GL mode is activated over the ocean to increase the incident signal and ensure the signal-to-noise ratios meet the requirements. The atmospheric carbon dioxide grating spectrometer on board TanSat provides hyperspectral measurements of the O2 A band (0.76 μm) and CO2 weak (1.61 μm) and strong (2.04 μm) bands. The ND footprint size of TanSat measurement is ~2 × 2 km with nine footprints across the track in a frame, while the total field-of-view width is ~18 km. The TanSat repeat measurement cycle is 16 days, and the ND and GL measurements are staggered. In ND mode, TanSat has the same ground track interval as OCO-2.XCO2 retrieval is performed using the Institute of Atmospheric Physics carbon dioxide retrieval algorithm for satellite remote sensing (Liu et al., 2013; Yang et al., 2015). The version 1 TanSat XCO2 retrievals only used the CO2 weak band due to calibration issues on the O2 A band (which can cause critically biased estimates of surface pressure). Therefore, the flux inversion precision and accuracy were not adequate for studying global surface carbon flux. Further study on solar calibration measurements revealed a remaining feature on the solar spectrum fitting residual. A Fourier series model was applied as a gain factor to the continuum in the retrieval, significantly improving the fitting residual, especially on the O2 A band. The O2 A band and CO2 weak band measurements have been used together in new retrievals that correct the parameters of water vapor, surface pressure, temperature, aerosol, cirrus, and instrument model. A genetic algorithm was used in post screening, and then a multiple linear regression model was applied to correct bias (Yang et al., 2020). In this study, we used a 15-month (March 2017–May 2018) XCO2 retrieval from TanSat ND measurements.
Only “good” retrievals have been provided in the TanSat v2 product used in flux inversion. We used single sounding uncertainty (posterior error) to construct weighted time–space average data (Crowell et al., 2019). First, we calculated a 1-second span average:
where


where we assumed soundings in a 1-second span were highly correlated. Because the posterior error mainly considered the theoretical error from the measurement noise level, the









In this study, we used a 5-second span average for flux inversion. The


2
2.2. Carbon flux inversion system
We used an ensemble transform Kalman filter (ETKF) data assimilation system (Feng et al., 2009, 2011, 2016) to estimate global and regional carbon flux. The carbon flux and CO2 concentration were optimized by TanSat XCO2 measurements via:where



where R is the observation error covariance matrix, namely, a diagonal matrix representing measurement errors, including instrument error, retrieval error, model error, and representation error (Peylin et al., 2002).





We used sequentially assimilated TanSat 5-second averaged measurements day by day in each assimilation step (1 month) to optimize the a priori estimation of surface CO2 fluxes. The GEOS-5 meteorological analyses, provided by the NASA Goddard Global Modelling and Assimilation Office, were used to drive the GEOS-Chem run. The model was run at a horizontal resolution of 4° (latitude) × 5° (longitude) with 47 vertical levels, which spanned from the surface to the mesosphere, typically with 35 levels in the troposphere. Monthly inventories have been used as the a priori flux for GEOS-Chem runs, including ocean flux (Takahashi et al., 2009), biomass burning fluxes (van der Werf et al., 2010), 3-hourly terrestrial biosphere fluxes (Olsen, 2004), and fossil fuel emissions (Oda and Maksyutov, 2011). For the terrestrial biosphere, we used a 3-hour flux to better represent and optimize the uptakes and emissions from photosynthesis and respiration processes, respectively (Olsen, 2004). The inversion for surface flux spanned 792 regions globally, including 475 land regions and 317 ocean regions, based on a TransCom 3 study (Gurney et al., 2002).
3.1. Annually integrated global carbon net flux
In this study, only the ND model land observations were applied to constrain the flux optimization. Due to the low signal-to-noise ratio, the ND measurements over the ocean are not provided in the L2 XCO2 data product, and no further information is provided by TanSat ocean measurements. Therefore, a very strong prior constraint was applied to the ocean CO2 flux. The inversion result is shown as carbon flux based on CO2 flux using the mass ratio between CO2 and carbon.Error reduction for global carbon sinks indicates how well the measurements optimized the estimations. The error reduction in July was greater than in other months (Fig. 1); for example, it was > 50% in northern Asia, Europe, and America, but lower in Australia, southern Asia, and India. South America and Africa showed 20%–40% error reduction. TanSat measurements improved results in South Africa mostly in April and October, in North and South America mostly in July, and in northern Eurasia in all three months.

The global annual carbon sinks (Fig. 2) constrained by TanSat measurements differed significantly from a priori fluxes. For example, the land sink increased in most of Northern Asia, Europe, and the Americas, but decreased in the middle of Africa and India. Similar results have been reported in GOSAT and OCO-2 CO2 flux inversion studies (Wang et al., 2019, 2020). The land sink also increased in southwest China, which is consistent with carbon flux estimations based on additional ground-based in-situ measurements from Asia (Wang et al., 2020). The optimization was not significant over the ocean because no direct measurements were used to constrain the oceanic carbon flux.
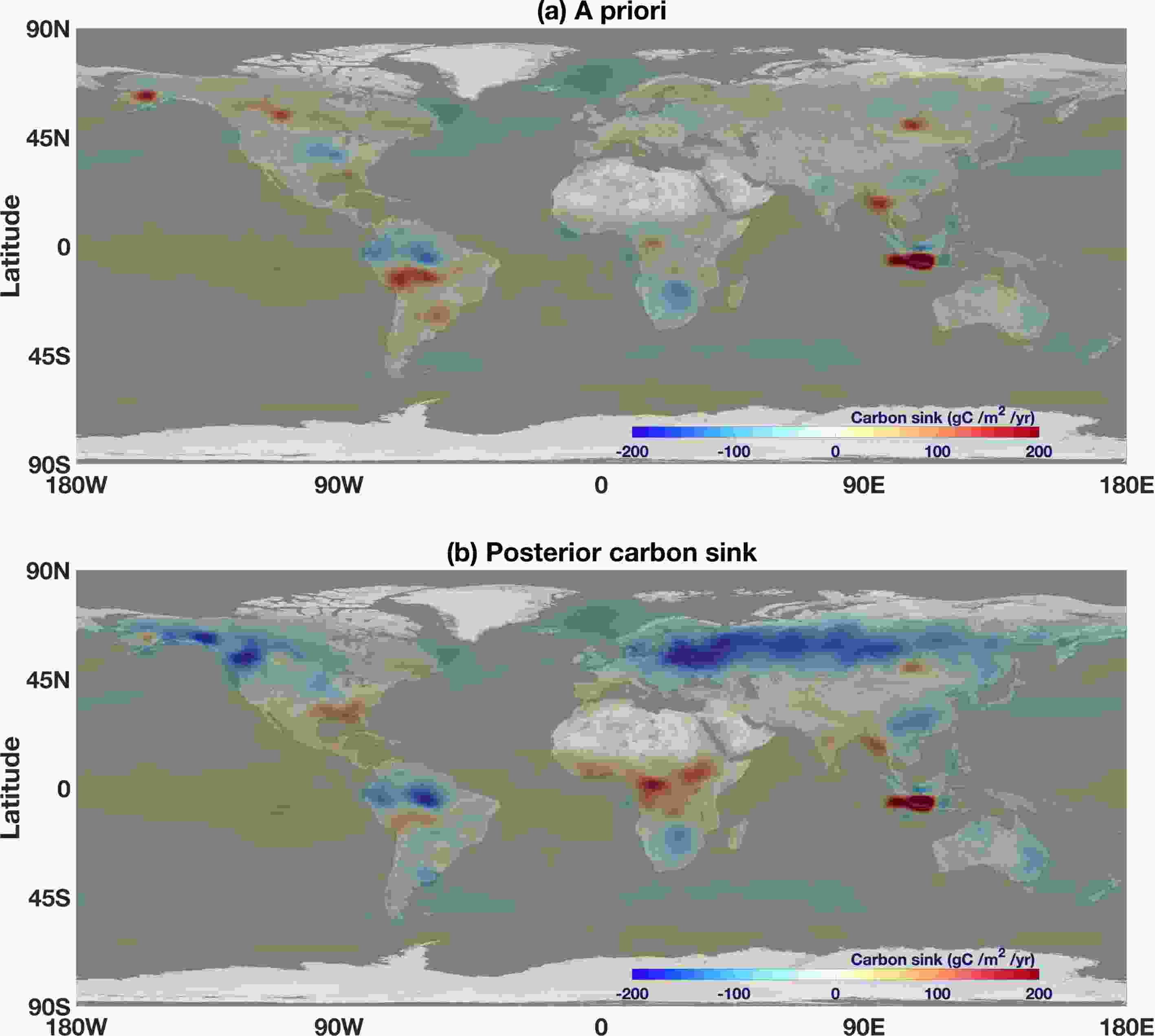
Our TanSat inversion indicated a land net flux integrated over 12 months (May 2017–April 2018) of 6.71 Gt C yr?1 with an uncertainty of 0.76 Gt C yr?1. Table 1 shows the land carbon net flux over the same period constrained by the new L2 OCO-2 XCO2 data product (v10) as a reference. The annul integrated OCO-2 net carbon flux was 5.13 ± 0.59 Gt C yr?1, which is 23.5% lower than the TanSat results. Several studies have reported different carbon flux estimations based on satellite measurements for 2009 onwards. The GOSAT measurement was the first spaced-based GHG measurement used in global CO2 flux estimation (Basu et al., 2013). GOSAT measurements indicated land net carbon fluxes estimated by the carbon tracker (Peters et al., 2007;
Region | A priori | TanSat | OCO-2 | |||||
Flux | 1-σ | Flux | 1-σ | Flux | 1-σ | |||
North American Boreal (NAB) | 0.17 | 0.33 | ?0.82 | 0.19 | ?0.23 | 0.19 | ||
North American Temperate (NAT) | 1.52 | 0.58 | 1.53 | 0.29 | 1.62 | 0.27 | ||
South American Tropical (SAT) | 0.094 | 0.40 | 0.78 | 0.26 | 0.09 | 0.22 | ||
South American Temperate (SAM) | 0.49 | 0.39 | 0.065 | 0.26 | ?0.76 | 0.20 | ||
North Africa (NAF) | 0.20 | 0.24 | 1.059 | 0.18 | 0.81 | 0.15 | ||
South Africa (SAF) | ?0.057 | 0.44 | 0.74 | 0.25 | ?0.19 | 0.18 | ||
Eurasia Boreal (EAB) | 0.10 | 0.60 | ?1.12 | 0.23 | ?0.12 | 0.25 | ||
Eurasia Temperate (EAT) | 3.83 | 0.42 | 3.68 | 0.24 | 2.89 | 0.22 | ||
Tropical Asia (TA) | 1.23 | 0.27 | 0.90 | 0.20 | 0.61 | 0.16 | ||
Australia (AUS) | 0.069 | 0.20 | ?0.21 | 0.17 | ?0.056 | 0.15 | ||
Europe (EU) | 1.15 | 0.66 | ?0.18 | 0.30 | 0.18 | 0.28 | ||
North land (NL) | 6.78 | 1.43 | 3.10 | 0.52 | 4.34 | 0.41 | ||
South land (SL) | 0.50 | 0.62 | 0.60 | 0.38 | ?1.00 | 0.30 | ||
Tropical land (TL) | 1.52 | 0.54 | 2.73 | 0.36 | 1.51 | 0.30 | ||
Global Land | 9.08 | 1.74 | 6.71 | 0.77 | 5.13 | 0.59 |
Table1. The a priori and a posteriori fluxes estimated by TanSat (v2) and OCO-2 (v10) for 11 TransCom regions and continents.
2
3.2. Seasonal and regional CO2 flux
Seasonal net carbon flux was grouped every three months and denoted following the Northern Hemisphere seasons, namely, summer (June, July, August), autumn (September, October, November), winter (December, January, February), and spring (March, April, May). The distribution of global carbon flux differed significantly from a priori flux after assimilation of TanSat measurements (Fig. 3). For example, the measurements (satellite data) significantly changed carbon fluxes for South America for the whole year (Fig. 4). The South America tropical region showed the largest impacts from TanSat measurements, with the seasonal phase shifted significantly from a priori fluxes. We found that the satellite did not provide dense measurements over tropical South America, but even a small amount of TanSat data can still significantly change the carbon flux feature. TanSat measurements increased carbon sinks in spring and summer around Eurasia, including in Europe and boreal and temperate Eurasia, compared with a priori fluxes. North Africa also showed significant increases in the carbon sink in winter. In addition, the seasonal variation of India decreased during spring and summer.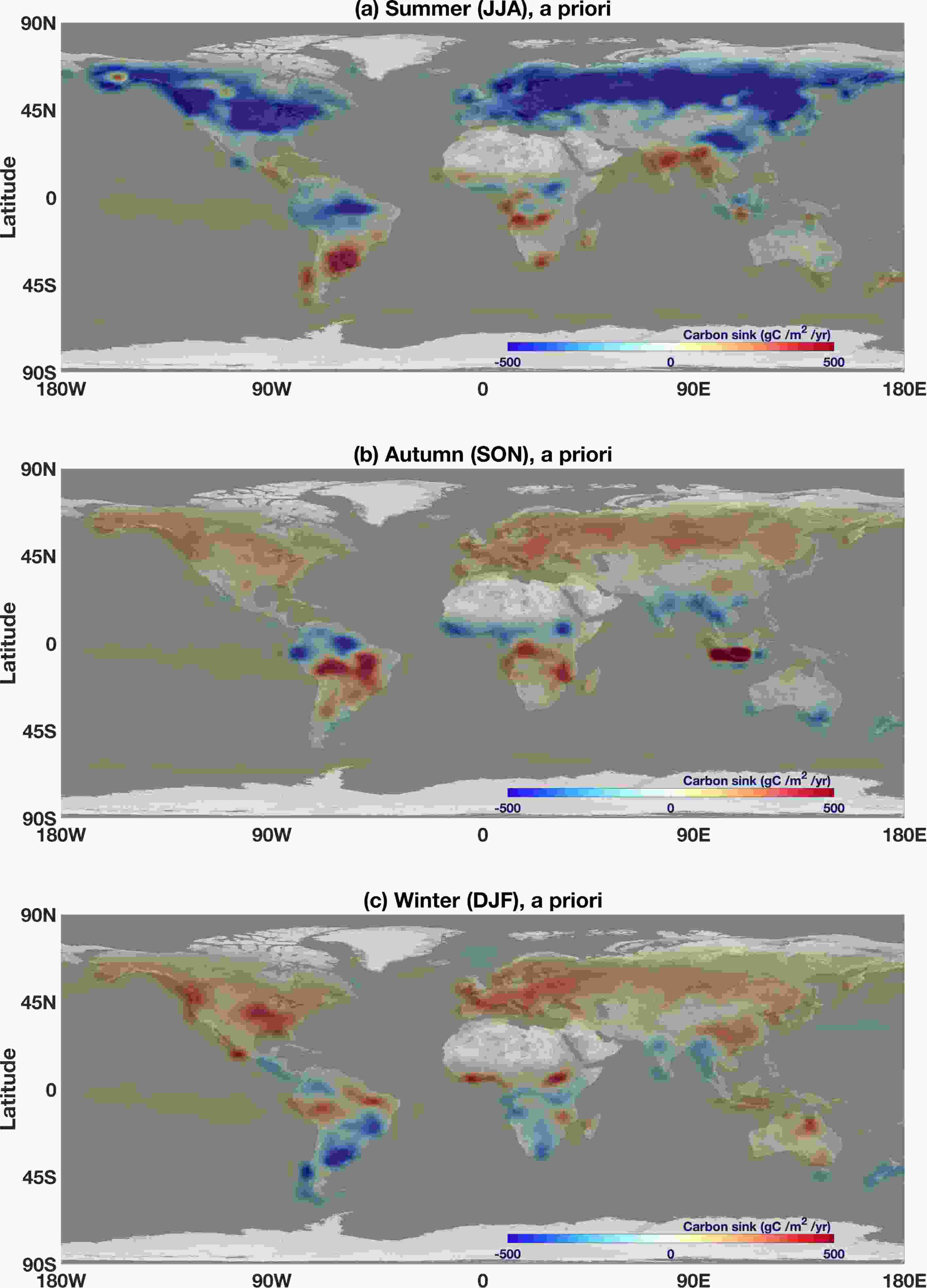
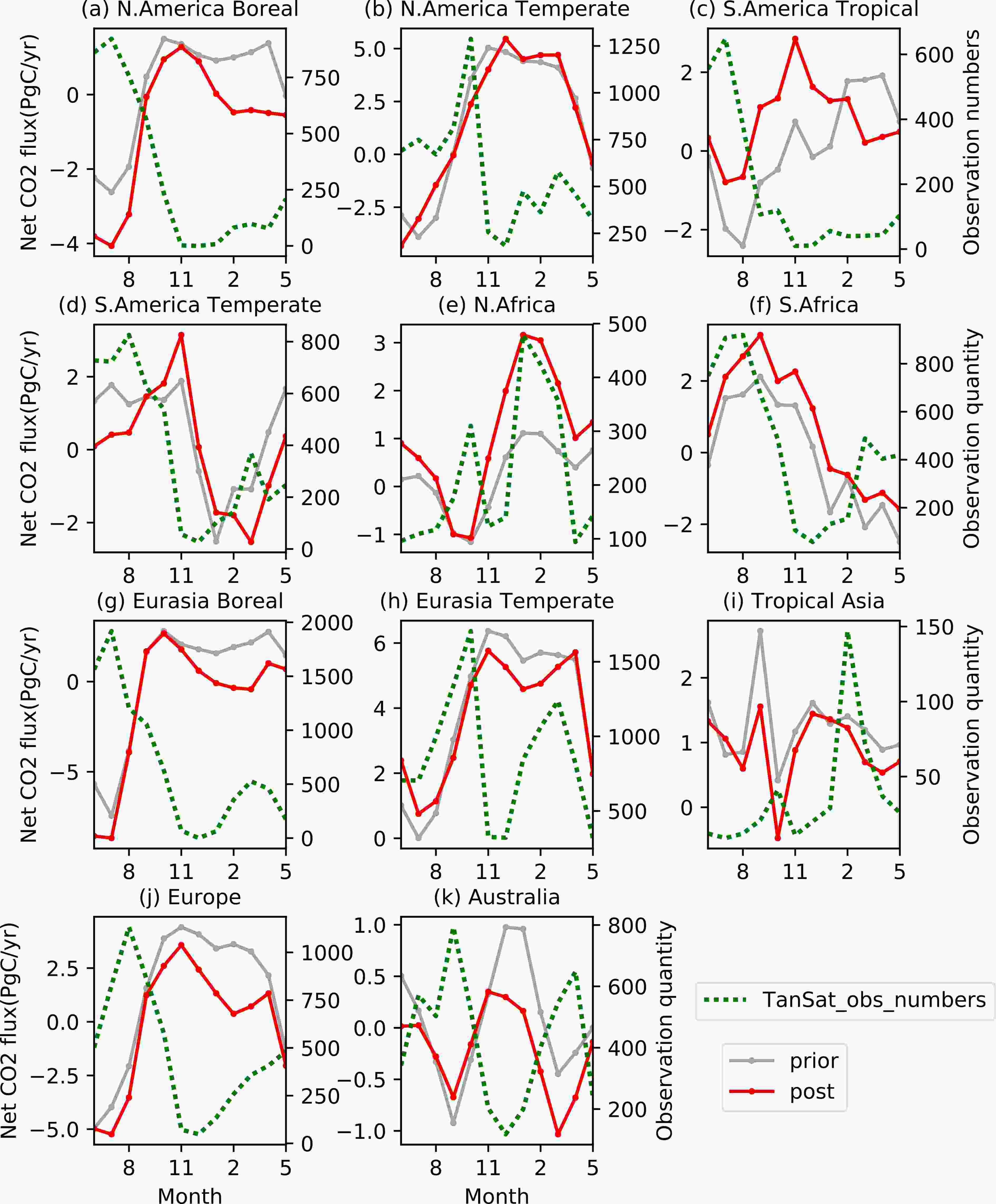
We also observed changes in China’s carbon flux in autumn, winter, and spring; in general, the carbon sink increased compared to a priori fluxes, especially in southwest China in the summer and autumn. Similar results have been reported by studies of vegetation trend measurements (Chen et al., 2019) and flux estimations (Wang et al., 2020).
Figure 5 shows regional carbon flux from OCO-2 and TanSat measurements, as well as a priori fluxes. OCO-2 and TanSat have similar optimization effects on a priori fluxes in most regions. The biggest discrepancy was in the Southern Hemisphere, where TanSat measurements and a priori fluxes indicated a source, whereas OCO-2 measurements indicated a sink; the difference in outcomes was driven in large part by results from tropical and temperate South America. This contradiction has also been reported when comparing results from GOSAT and OCO-2 measurements (Wang et al., 2019).

These carbon flux data are publicly accessible worldwide on the China GEO TanSat data service archive, the Cooperation on the Analysis of Carbon Satellites Data (CASA;
TanSat provides preliminary global measurements that extend the ground-based network from local measurements to global estimates. However, the coverage, repeat, pixel size, and accuracy of measurements are still inadequate to meet the Global Stocktake of the Paris Agreement and inventory validation requirements of IPCC standards. For example, GOSAT measurements have indicated that flux estimation is sensitive to measurement coverage (Deng et al., 2014). XCO2 measurements are also sensitive to regional flux inversion (Feng et al., 2009; Palmer et al., 2011). The future European Copernicus Carbon Dioxide Monitoring satellite mission (Kuhlmann et al., 2019) will improve measurements of global coverage and investigations of anthropogenic emissions. The plan for the next TanSat mission has been discussed and is in the design phase. One possibility is a multi-satellite LEO constellation. Several satellites would capture global background measurements with large pixel size and a wide swath, while others would focus on target measurements for city and point sources with a small pixel size. This would capture carbon sinks and sources at multiple scales to elucidate the influences of natural processes and anthropogenic activities on the carbon cycle.
Column CO2 measurements are still inadequate for separating local carbon exchanges from long-range transport processes because the signal is mixed into the column value (Keppel-Aleks et al., 2011). The signal of local flux is critical to city and point-source research. Ground-based measurements near the surface will be helpful for future investigations. Therefore, coordinating satellites and surface measurement networks will significantly improve the study of small-scale anthropogenic emission sources.
Acknowledgements. This work is supported by the National Key R&D Program of China (Grant No. 2016YFA0600203), the Key Research Program of the Chinese Academy of Sciences (ZDRW-ZS-2019-1), the National Key R&D Program of China (Grant No. 2017YFB0504000), and the Youth Program of the National Natural Science Foundation of China (Grant No. 41905029). Liang FENG is supported by the UK NERC National Centre for Earth Observation (NCEO). The TanSat L1B data service is provided by IRCSD and CASA (131211KYSB20180002). We also thank the FENGYUN Satellite Data Center of the National Satellite Meteorological Center, who provided the TanSat L1B data service. The authors thank the TanSat mission and highly appreciate the support from everyone involved.