







Review of Genomic Microsatellite Status Detection Based on Machine Learning
ZHANG Shuying







通讯作者: *牛北方(E-mail:niubf@cnic.cn)
收稿日期:2021-01-21网络出版日期:2021-06-20
基金资助: |
Received:2021-01-21Online:2021-06-20
作者简介 About authors
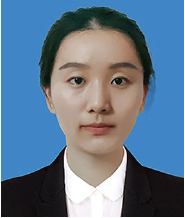
张舒莹,中国科学院计算机网络信息中心,在读硕士研究生,主要研究方向为癌症基因组学。
本文中承担的任务是论文构思以及撰写。
ZHANG Shuying is a master student at CNIC. She mainly focuses on cancer genome research.
In this paper, she is mainly responsible for paper design and writing.
E-mail:
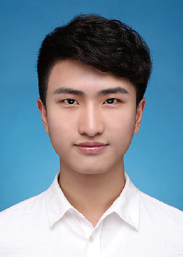
韩鑫胤,中国科学院计算机网络信息中心,在读博士研究生,主要研究方向为癌症基因组学。
本文中承担的任务是论文修稿。
HAN Xinyin is a Ph.D. student at CNIC. He is mainly engaged in cancer genome research.
In this paper, he is mainly responsible for revising the paper.
E-mail:
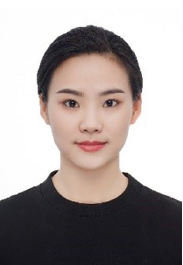
何小雨,中国科学院计算机网络信息中心,在读博士研究生,主要研究方向为高性能计算和癌症基因组学。
本文中承担的任务是论文修稿。
HE Xiaoyu is a Ph.D. student at CNIC. She is mainly engaged in high perfor-mance computing and cancer genomics.
In this paper, she is mainly responsible for revising the paper.
E-mail:
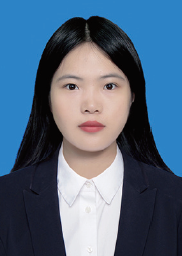
袁丹阳,中国科学院计算机网络信息中心,在读硕士研究生,主要致力于白血病相关生物信息学的研究。
本文中承担的任务是论文修稿。
YUAN Danyang is a master student at CNIC. She mainly focuses on leukemia related bioinformatics research.
In this paper, she is mainly responsible for revising the paper.
E-mail:
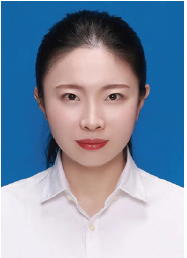
栾海晶,中国科学院计算机网络信息中心,在读硕士研究生,主要研究方向为高性能计算和癌症基因组学。
本文中承担的任务是论文修稿。
LUAN Haijing is a master student at CNIC. She is mainly engaged in high perform-ance computing and cancer genomics.
In this paper, she is mainly responsible for revising the paper.
E-mail:
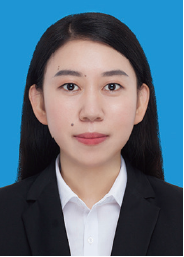
李瑞琳,中国科学院计算机网络信息中心,博士,助理研究员,主要研究方向为高性能计算和癌症基因组学。
本文中承担的任务是论文修稿。
LI Ruilin, Ph.D., is an assistant research fellow at CNIC. She is mainly engaged in high performance computing and cancer genomics
In this paper, she is mainly responsible for revising the paper.
E-mail:
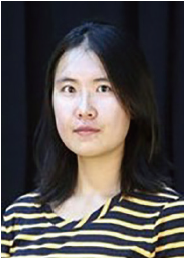
何佳茵,中国科学院计算机网络信息中心,硕士,助理工程师,主要研究方向为高性能计算和癌症基因组学。
本文中承担的任务是论文修稿。
HE Jiayin, M.D., is an assistant engineer at CNIC. She is mainly engaged in high performance computing and cancer genomics.
In this paper, she is mainly responsible for revising the paper.
E-mail:
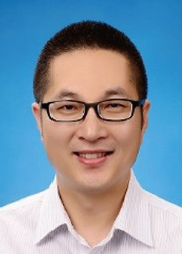
牛北方,中国科学院计算机网络信息中心,博士,研究员,主要研究方向为高性能计算和癌症基因组学。
本文中承担的任务是研究指导,论文结构统筹。
NIU Beifang, Ph.D., is a research fellow at CNIC. His activities mainly focus on high performance computing and cancer genomics.
In this paper, he is mainly responsible for research guidance and overall planning of the paper structure.
E-mail:

摘要
【目的】 探讨机器学习在基因组微卫星状态检测方法中的应用及未来研究方向。【文献范围】 本文收集了微卫星状态检测方法相关文献。【方法】 首先简要介绍微卫星状态检测的意义和常用的检测手段,其次对目前主流的基于机器学习的检测方法进行详细介绍,最后展望未来机器学习在微卫星状态检测领域中的研究方向。【结果】 基于机器学习的检测方法从大量测序数据中迭代学习,获取影响微卫星不稳定性的关键特征,该类检测方法可以取得较好的预测效果。【局限】 检测方法使用的数据类型各异,本文中无法使用同一数据集对各个检测方法进行实验比较。【结论】 机器学习已广泛应用于微卫星状态检测领域,提高检测方法的适用性以及从外周血样本中检测微卫星状态,是机器学习在此领域的未来研究方向。
关键词:
Abstract
[Objective] This paper discusses the application and future research direction of machine learning in microsatellite status detection. [Scope of the literature] We collected the related literature of microsatellite status detection methods.[Methods] Firstly, the significance of microsatellite status detection and common detection methods are briefly introduced. Secondly, the current mainstream detection methods based on machine learning are introduced in detail. Finally, perspective future research direction of machine learning in the field of microsatellite status detection is presented.[Results] The detection methods based on machine learning can iteratively learn from massive sequencing data and discern key features that affect microsatellite instability. They can achieve accurate prediction results. [Limitations] The data types used by the detection methods are different, so we cannot compare the detection methods within the same dataset. [Conclusions] Machine learning has been widely used in microsatellite status detection. Improving the applicability of detection methods and detecting microsatellite status from peripheral blood samples are the future research directions of machine learning in this field.
Keywords:
PDF (7874KB)元数据多维度评价相关文章导出EndNote|Ris|Bibtex收藏本文
本文引用格式
张舒莹, 韩鑫胤, 何小雨, 袁丹阳, 栾海晶, 李瑞琳, 何佳茵, 牛北方. 基于机器学习的基因组微卫星状态探测方法综述[J]. 数据与计算发展前沿, 2021, 3(3): 126-135 doi:10.11871/jfdc.issn.2096-742X.2021.03.011
ZHANG Shuying, HAN Xinyin, HE Xiaoyu, YUAN Danyang, LUAN Haijing, LI Ruilin, HE Jiayin, NIU Beifang.
引言
《2020全球癌症报告》显示,全球癌症病例数呈增长趋势,癌症已对人类健康产生了重大威胁。探究癌症的产生原因,可以对癌症进行预防并且有助于癌症患者的诊断和治疗。研究证实,癌症源于基因突变的不断积累,基因突变表现为基因序列上发生改变,包括碱基的点突变、碱基序列的插入和删除变异等[1]。人类基因组中有一些特殊的短串联重复序列,被称为微卫星(microsatellites,MS)。当MS序列发生插入或删除突变且无法被修复时,则会产生微卫星不稳定性(microsatellite instability,MSI)现象。1993年,MSI现象在遗传性结直肠癌中被发现[2]。后续的研究表明,除了结直肠癌外,子宫内膜癌、胃癌、肺癌和食管癌等多种癌症中均有不同比例的MSI现象发生[3,4,5,6]。MSI检测可以对癌症患者进行遗传筛查、预后判断以及免疫治疗等。
目前,已经有多种MSI检测的方法,包括传统的生物学实验方法以及基于高通量测序的方法[7]。随着人工智能的发展,机器学习逐渐渗入生物信息学领域并发挥巨大作用[8,9,10]。基于机器学习的MSI检测方法,借助机器学习的强大学习能力,可以对数据进行多维度的分析,找出影响MSI的主要因素。
1 研究背景
1.1 MSI及其检测意义
MS是一种以1-6个碱基为单位,重复次数为10-60次的短核苷酸序列[11]。MSI是指在DNA复制过程中由于滑移引起的MS序列长度改变的现象[12]。在正常情况下,细胞中的错配修复(mismatch repair,MMR)系统可以修复由于滑移导致的碱基错配,当MMR通路基因发生突变或甲基化则会导致MMR系统出现错配修复缺陷(deficient mismatch repair,dMMR),此时碱基错配无法被修复,从而产生MSI[13]。根据不稳定程度,MSI可以划分为:微卫星稳定性(microsatellite stability,MSS),低频微卫星不稳定性(MSI-low,MSI-L)和高频微卫星不稳定性(MSI-high,MSI-H)。在研究中通常将MSI-L作为MSS处理[14,15]。MSI现象在多种癌症中均有出现,其状态检测在临床上有重要意义。MSI的检测在林奇综合征遗传筛查中发挥重要作用。林奇综合征又称为遗传性非息肉病性结直肠癌,源于MMR基因发生胚系突变[16]。林奇综合征具有家族遗传倾向,该群体患有结直肠癌的概率可达80%[17,18]。除此之外,该群体也易患其它癌症[19,20]。因此,建议对所有癌症患者进行MSI检测,以便筛查林奇综合征[21],如果确诊林奇综合征可及早采取治疗,并对其直系亲属进行筛查和早期干预。
MSI状态的检测还有助于Ⅱ期结直肠癌患者的预后判断。相对于MSS结直肠癌群体,MSI-H群体的总生存期及无进展生存期有较为显著的延长[14,22-23]。另有研究表明,对Ⅱ/Ⅲ期结直肠癌患者使用5-氟尿嘧啶药物会影响其预后,缩短其总生存期[24]。因此,鉴于MSI-H的Ⅱ期结直肠癌患者具有较好预后,不建议对其使用氟尿嘧啶类的药物进行辅助化疗[25]。
MSI是重要的免疫治疗生物标志物。MSI-H/dMMR癌症患者体内携带大量的可被免疫系统识别的新生抗原,这使得患者对免疫检查点阻断疗法敏感[26,27]。大量研究证实,对于MSI-H癌症患者,使用免疫检查点抑制剂(PD-1/PD-L1抗体)治疗可取得较好的疗效[28,29,30]。MSI已成为重要的免疫治疗生物标志物,对患者进行MSI检测有助于指导患者后续治疗。
1.2 常用的MSI检测方法
常见的MSI检测方法主要分为两大类,第一类是传统的生物学实验的方法,第二类是基于高通量测序的方法。传统的生物学实验方法包括多重荧光PCR法(MSI-PCR)和蛋白免疫组织化学法(MMR-IHC)[31,32]。MSI-PCR使用多重荧光PCR结合毛细管电泳的方法,对肿瘤组织和正常组织中分离出的DNA序列进行扩增,比较扩增后的MS位点突变情况,进而判定样本的MSI状态。通常检测的位点是Bethesda panel中的5个MS位点,以及Promega分析系统提出的7个MS位点。MMR-IHC通常检测肿瘤组织中的4个MMR蛋白表达情况来查看MMR系统是否发生故障,从而判断样本MSI状态。相比于MSI-PCR,MMR-IHC操作较简单,成本较低,可广泛应用于临床检测中,但其需要人眼阅片计数,受个人主观因素影响较大。随着高通量测序技术的快速发展,以全基因组测序(WGS)、全外显子组测序(WES)以及靶向测序(TS)为主的高通量数据已纳入常规的生物信息学研究中。基于高通量测序的检测方法比生物学实验方法具有明显的优势:(1)不需要额外的临床测试和样本处理,对于不具备生物学实验条件的团队也可进行MSI检测;(2)可同时捕获多段基因序列,有助于从多个维度评估样本MSI状态,极大提高诊断效率和检测的灵敏性;(3)不同于MSI-PCR只检测个位数的MS位点,基于高通量测序的检测方法覆盖的MS位点数以千计,可以进行更加深入和全面的评估,并且可提供单个MS位点的定量信息。
目前,已发布了多种使用测序数据进行MSI检测的方法,比如MSIsensor[33]、mSINGS[34]和MANTIS[35]等。其中,MSIsensor已经被成功应用于FDA批准的基于高通量测序的肿瘤检测方法MSK-IMPACT中[36]。这些方法分别采用卡方检验、Z-score和平均距离等传统的统计学方法评估MS位点稳定性,它们虽然可以判定MSI状态,但是缺乏多维度的考量。测序数据本身蕴含丰富的生物学信息[37],传统的统计学方法无法高效处理复杂的海量数据,可能会忽略某些影响MSI判定的关键要素。机器学习作为传统统计学的延伸,可以从大量的数据中抽取关键特征进行迭代学习,并且在此过程中屏蔽复杂的细节。机器学习在MSI的探索中发挥了巨大的作用,同时也为MSI检测提供了新角度和新思路。
2 基于机器学习的MSI检测方法
MSI检测在机器学习领域是一个二分类任务,使用决策树、支持向量机、逻辑回归等常用的机器学习算法可以高效的解决此类问题。本文对目前基于机器学习的MSI检测方法进行了充分的调研,涵盖了主流的检测方法,比较了各个方法使用数据集的测序方法和最终采用的机器学习算法,以及该数据集在对应机器学习模型中的检测效果(表1)。下面将分别介绍这些方法结合机器学习算法进行MSI状态检测的流程。Table 1
表1
表1基于机器学习的MSI检测方法
Table 1
方法 | 测序方法 | 机器学习算法 | 准确率(%) | 灵敏性(%) | 特异性(%) |
---|---|---|---|---|---|
MSIseq[38] | WES | 决策树 | 98.8 | - | - |
MSIpred[39] | WES | 支持向量机 | 98.3 | 93.6 | 99.6 |
MOSAIC[40] | WES | 决策树 | 96.6 | 95.8 | 97.6 |
MIRMMR[41] | - | 逻辑回归 | 93.8 | 91.9 | 94.2 |
MIAmS[42] | TS(amplicon panel) | 支持向量机(默认) | 100.0 | - | - |
新窗口打开|下载CSV
(1)MSIseq
MSIseq算法考虑到dMMR会影响单核苷酸替代(single nucleotide substitution,SNS)比率和小片段插入删除(indel)比率,因此从SNS和indel这两个突变信息入手,构建了9个待选特征,具体含义如表2中(1-9行)所示,其中括号内表示的是该特征在MSIpred中的标记。
Table 2
表2
表2MSIseq和MSIpred的特征
Table 2
序号 | 特征 | 含义 |
---|---|---|
1 | T.sns(SNP) | 样本中SNS比率 |
2 | S.sns(SNP_R) | MS序列中SNS比率 |
3 | T.ind(INDEL) | 样本中indel比率 |
4 | S.ind(INDEL_R) | MS序列中indel比率 |
5 | T(t_mutation) | 样本中突变比率 |
6 | S(t_mutation_R) | MS序列中突变比率 |
7 | S.sns/T.sns(SNP_R/SNP) | - |
8 | S.ind/T.ind(INDEL_R/ INDEL) | - |
9 | S/T(t_mutation_R/t_ mutation) | - |
10 | Frame_Shift_Del | 导致ORF偏移的删除比率 |
11 | Frame_Shift_Ins | 导致ORF偏移的插入比率 |
12 | In_Frame_Del | ORF没有偏移的删除比率 |
13 | In_Frame_Ins | ORF没有偏移的插入比率 |
14 | Missense_Mutation | 错义突变比率 |
15 | Nonsense_Mutation | 无义突变比率 |
16 | Silent | 沉默突变比率 |
17 | Splice_Site | 剪接位点的突变比率 |
18 | 3’UTR | 3’UTR区域突变比率 |
19 | 3’Flank | 3’Flank区域突变比率 |
20 | 5’UTR | 5’UTR区域突变比率 |
21 | 5’Flank | 5’Flank区域突变比率 |
22 | Intron | 内含子区域突变比率 |
新窗口打开|下载CSV
该研究共收集了526例多癌种的WES突变数据,这些样本也使用MSI-PCR进行了状态测定。在实验中,分别使用决策树、逻辑回归、随机森林和贝叶斯算法,采用k折交叉验证法(k=5)进行训练,将验证结果与MSI-PCR测定的结果进行对照,其一致性分别为98.6%、96.5%、98.1%和96.7%。从结果上看,决策树模型的准确率最高。
进一步研究发现,在决策树模型中,特征S.ind对结果的判定取决定性作用,即只需这一个特征就可以将MSI-H和MSS样本区分开,当S.ind>0.395时,样本被标记为MSI-H,否则为MSS。出于准确率考虑,该研究最终选取只具有一个特征(S.ind)的决策树算法进行MSI状态的检测,该模型在测试集中的准确性高达98.8%。
该方法选取解释性较强的决策树算法构建检测流程,其输入的是MAF格式的突变数据,相较于mSINGS等需要BAM格式数据的方法节省了大量的计算资源。从测试结果上看,该方法判定样本MSI状态的准确率很高,但是其只使用一个特征参与模型训练和预测,会产生过拟合现象。
(2)MSIpred
与MSIseq类似,MSIpred也是基于突变信息构建特征。不同的是,为了防止过拟合,MSIpred在MSIseq的9个待选特征基础上,又新增了13个特征,如表2中所示。其中第1-9行特征与MSIseq的待选特征一致,描述的是SNS和indel信息,10-22行是新增的特征,描述了突变有害程度的关键信息。
该研究共收集了1 432例多癌种的WES突变数据,这批数据也具有MSI-PCR的验证结果作为参照。在实验中,采用支持向量机算法进行训练及测试,测试结果显示该方法的准确率达98.3%,灵敏性达93.6%,特异性达99.6%。该研究同时对MSIseq的9个待选特征单独进行了训练和测试,结果显示使用全部的22个特征训练的模型预测效果更好。
该方法的输入同样是MAF格式的突变数据,可以节省计算资源,提高检测效率。除此之外,在MSIseq研究的基础上,选取具有22个特征的支持向量机算法构建检测流程,弥补了MSIseq的不足之处,减少了过拟合风险。
(3)MOSAIC
MOSAIC从MS位点稳定性出发,根据MS位点的不稳定情况判定样本的MSI状态。该方法需要使用肿瘤样本(Tumor,T)配对的正常样本(Normal,N)作为参照。首先获得单个MS位点在T和N中的等位基因分布数据,由于MS位点不稳定会伴随着MS序列长度发生波动,因此对比T和N中的等位基因支持的reads数即可评估此MS位点的稳定性。
该研究共收集了617例多癌种T-N配对的WES测序数据,根据MSI-PCR的结果将其划分为两组,一组为MSI-H的T-N样本,一组为MSS的T-N样本,分别对这两组样本中的MS位点进行稳定性分析。该研究设定以N中的等位基因分布为基准,如果T中出现在N中没有的等位基因,则该MS位点为不稳定的位点。该研究使用Fisher精确检验评估了每个MS位点在MSI-H和MSS样本中的区分能力,对在MSI-H样本中最显著不稳定的MS位点进行了排名,其中位于DEFB105A/B基因上的chr. 8:7679723-7679741位点排在第一位,在该研究中被记作defbsite。
基于以上分析,该研究结合前100个在MSI-H样本中显著不稳定的MS位点(包括defbsite)和另外4个待选特征进行分析(表3)。采用决策树算法进行训练,并使用留一法进行验证,筛选可以预测MSI状态的最佳特征,结果显示peak_avg和defbsite是最显著的两个特征,当只使用这两个特征进行训练时,结果准确率达96.6%。
Table 3
表3
表3MOSAIC的待选特征
Table 3
序号 | 特征 | 含义 |
---|---|---|
1 | peak_avg | T相对于N出现的新等位基因个数的平均值 |
2 | peak_var | T相对于N出现的新等位基因个数的方差 |
3 | num_unstable | 不稳定的MS位点个数 |
4 | prop_unstable | 不稳定的MS位点占比 |
新窗口打开|下载CSV
该研究对单个MS位点进行稳定性分析,可以提供位点的定量信息,获得影响样本MSI状态的显著MS位点集合,有助于MSI检测的后续探索。该方法只适用于具有配对正常样本(T-N)的情况,如果没有可参照的正常样本,则无法使用该方法进行MSI检测。
(4)MIRMMR
不同于以上三种方法,MIRMMR不再局限于根据MS序列的插入删除情况来评估样本MSI状态,而是从MSI发生的根本原因出发,分析35个MMR通路基因的甲基化水平和突变数据,构建逻辑回归模型预测样本状态。该方法提供5个模块,其中三个模块(univariate、stepwise和penalized)代表三种构建模型的策略,另有一个预测模块(predict)和一个比较模块(compare)。
Univariate模块将对每个单变量建立逻辑回归模型,最终汇集每个单变量的模型供后续使用。Stepwise模块对特征进行筛选,选择最佳的特征组合参与训练。Penalized模块采用了弹性网络回归模型,使用k折交叉验证的方法寻找最优的参数(k=10),该模块是MIRMMR默认使用的策略。Predict模块使用前期训练好的模型进行预测,给出MSI-H的概率值,由用户权衡灵敏性和特异性划分判定MSI状态的基准线。Compare模块用来比较不同策略下的结果,绘制出对应的ROC曲线以及计算AUC值。
MIRMMR提供了三种构建模型的策略,用户可使用多种策略构建检测模型,验证检测结果。MIRMMR的研究对象是35个MMR通路基因,提供了一个不依赖于MS位点检测MSI的新方法。
(5)MIAmS
MIAmS的检测流程主要分两步,第一步是MIAmS_learn,在这一步骤中会对MS位点进行筛选和标注标签,当MS位点的测序深度不能满足最小测序深度限制时,该位点会被过滤掉,默认的最小测序深度是300X。第二步是MIAmS_tag,对样本MSI状态进行检测,在这一步中,MIAmS工具提供了两种检测模式,第一种借助mSINGS进行评估,第二种使用机器学习的方式进行评估。
mSINGS模式是采用的传统统计方法,首先借助MSS样本计算MS位点的等位基因个数的平均数mean和方差SD,以[mean+3×SD]作为当前MS位点的基线,在测试过程中,如果MS位点的等位基因个数超过对应的基线,那么这个位点被判别为不稳定,最终根据样本中不稳定的MS位点个数在所有MS位点中的占比情况判断样本MSI状态。
机器学习模式默认使用支持向量机模型,可使用classifier参数更改为决策树、逻辑回归和随机森林等模型。该方法是结合MS位点的等位基因稳定和不稳定分布模型对该位点进行评估,每个MS位点会得到一个分数,以样本中所有MS位点得分的平均值判断样本MSI状态。
MIAmS包含基于传统统计学以及基于机器学习的两种检测方式,并提供友好的图形化界面对结果进行展示,有助于从多个角度评估样本MSI状态。
以上方法使用机器学习算法对MSI状态检测进行了多方面的探索。MSIseq和MSIpred使用突变数据构建训练特征,MSIseq最终只使用MS序列小片段插入删除情况判定样本状态。为了更全面的探究突变对MSI状态的影响,MSIpred对突变数据进行了更详细的分类,最终构建了22个特征进行检测。MOSAIC和MIAmS从单个MS位点出发,检测MS序列的波动情况评估该位点的稳定性,进而判定样本状态。MIRMMR从MSI产生的原因入手,根据MMR通路基因的甲基化水平和突变情况构建机器学习模型预测样本状态。总体而言,基于机器学习的MSI检测方法一般从MSI发生的原因或者MSI伴随的现象入手,根据MMR通路基因的突变信息或者MS序列区域的插入删除情况来预测样本的MSI状态。
3 结论与展望
本文首先介绍了MSI产生的原因以及其状态检测在临床上的重要性,并对目前常用的检测方法进行了介绍,归纳了基于高通量测序的MSI检测方法的优势。相对于高通量测序方法,传统的统计学方法无法聚焦MSI发生的分子机制,而人工智能领域的发展为此提供了新的思路。作为人工智能领域重要的分支之一,机器学习可以高效的从海量数据中学习知识,挖掘出影响MSI的要素并对数据进行多维度的分析。本文对目前主流的基于机器学习的检测方法进行了介绍,各项结果显示该类方法可以对样本的MSI状态进行较为准确的判别。目前机器学习算法已经广泛的应用到MSI检测中,并且取得了很好的检测效果,但是在临床应用中仍有探索空间及挑战:
(1)如何提高检测方法的适用性。目前多数检测方法基于WES数据展开,覆盖的MS位点数量庞大,但当检测数据是基于小panel的靶向测序数据时,使用该方法进行MSI状态检测,检测结果会产生较大偏差。
(2)如何从外周血中检测MSI状态。当前的检测方法多数采用肿瘤组织测序数据,但是组织活检具有侵入性,部分患者无法完成检测。科研人员继而开展从外周血中检测MSI状态,该项研究的主要难点在于外周血中的肿瘤DNA在癌症早期含量较低[43],无法精确捕获MSI信号。
应对以上挑战是MSI检测未来发展的方向,也是如何灵活应用机器学习算法助力的新方向。
利益冲突声明
所有作者声明不存在利益冲突关系。参考文献 原文顺序
文献年度倒序
文中引用次数倒序
被引期刊影响因子
[J].
PMID:10688858 [本文引用: 1]

There is increasing evidence that in eukaryotic cells, DNA undergoes continuous damage, repair and resynthesis. A homeostatic equilibrium exists in which extensive DNA damage is counterbalanced by multiple pathways for DNA repair. In normal cells, most DNA damage is repaired without error. However, in tumor cells this equilibrium may be skewed, resulting in the accumulation of multiple mutations. Among genes mutated are those that function in guaranteeing the stability of the genome. Loss of this stability results in a mutator phenotype. Evidence for a mutator phenotype in human cancers includes the frequent occurrence of gene amplification, microsatellite instability, chromosomal aberrations and aneuploidy. Current experiments have centered on two mechanisms for the generation of genomic instability, one focused on mutations in mismatch repair genes resulting in microsatellite instability, and one focused on mutations in genes that are required for chromosomal segregation resulting in chromosomal aberrations. This dichotomy may reflect only the ease by which these manifestations can be identified. Underlying both pathways may be a more general phenomenon involving the selection for mutator genes during tumor progression. During carcinogenesis there is selection for cells harboring mutations that can overcome adverse conditions that limit tumor growth. These mutations are produced by direct DNA damage as well as secondarily as a result of mutations in genes that cause a mutator phenotype. Thus, as tumor progression selects for cells with specific mutations, it also selects for cancer cells harboring mutations in genes that normally function in maintaining genetic instability.
[J].
DOI:10.1126/science.8484121URL [本文引用: 1]
[J].
DOI:10.1093/carcin/bgm228URL [本文引用: 1]
[J].
[本文引用: 1]
[J].
DOI:10.3748/wjg.v20.i14.3927URL [本文引用: 1]
[J].
DOI:10.1016/j.ctrv.2016.10.005URL [本文引用: 1]
[J].
[本文引用: 1]
[J].
DOI:10.1038/nrg3920URL [本文引用: 1]
[J].
[本文引用: 1]
[J].
[本文引用: 1]
[J].
DOI:10.1093/gbe/evq046URL [本文引用: 1]
[J].
DOI:10.1038/nrclinonc.2009.237URL [本文引用: 1]
[J].
[本文引用: 1]
[J].
DOI:10.1056/NEJMoa022289URL [本文引用: 2]
[J].
[本文引用: 1]
[J].
DOI:10.1002/(ISSN)1098-1004URL [本文引用: 1]
[J].
PMID:10348829 [本文引用: 1]
[J].
DOI:10.1111/ahg.1994.58.issue-3URL [本文引用: 1]
[J].
DOI:10.1056/NEJMra012242URL [本文引用: 1]
[J].
DOI:10.1200/JCO.2008.17.5950URL [本文引用: 1]
[J].
[本文引用: 1]
[J].
DOI:10.1200/JCO.2009.27.0652PMID:20516444 [本文引用: 1]

Microsatellite instability (MSI) is a clonal change in the number of repeated DNA nucleotide units in microsatellites. It arises in tumors with deficient mismatch repair due to the inactivation of one of the four mismatch repair genes: MSH2, MLH1, MSH6, and PMS2. In order to determine the MSI status of a tumor, microdissection and polymerase chain reaction-based detection strategies are required. For practical purposes, MSI is equivalent to the loss of staining by immunohistochemistry (IHC) of one of the mismatch repair genes since both signify an abnormality in mismatch repair. Of all colorectal cancers (CRCs), 15% to 20% display MSI or abnormal IHC (often referred to as microsatellite instability [MIN] pathway). The remaining 80% to 85% of CRCs are microsatellite stable but most are characterized by chromosomal instability (CIN pathway). Almost all Lynch syndrome tumors have MSI or abnormal IHC and they account for up to one third of all MIN CRCs (3% to 5% of all CRCs). The remaining MIN tumors are sporadic as a result of somatic inactivation of the MLH1 gene caused by methylation of its promoter. Thus, the presence of a MSI/IHC abnormality prompts further investigations to diagnose Lynch syndrome, whereas its absence excludes Lynch syndrome. We recommend screening all CRC tumors for IHC or MSI. MIN tumors have a more favorable outcome than CIN tumors, and fluorouracil-based adjuvant chemotherapy does not improve the outcome of stage II or stage III MIN tumors. More data are needed to determine how best to treat patients with stage II and stage III MIN CRCs.
[J].
DOI:10.1200/JCO.2006.06.9781URL [本文引用: 1]
[J].
DOI:10.1200/JCO.2009.27.1825PMID:20498393 [本文引用: 1]

Prior reports have indicated that patients with colon cancer who demonstrate high-level microsatellite instability (MSI-H) or defective DNA mismatch repair (dMMR) have improved survival and receive no benefit from fluorouracil (FU) -based adjuvant therapy compared with patients who have microsatellite-stable or proficient mismatch repair (pMMR) tumors. We examined MMR status as a predictor of adjuvant therapy benefit in patients with stages II and III colon cancer. MSI assay or immunohistochemistry for MMR proteins were performed on 457 patients who were previously randomly assigned to FU-based therapy (either FU + levamisole or FU + leucovorin; n = 229) versus no postsurgical treatment (n = 228). Data were subsequently pooled with data from a previous analysis. The primary end point was disease-free survival (DFS). Overall, 70 (15%) of 457 patients exhibited dMMR. Adjuvant therapy significantly improved DFS (hazard ratio [HR], 0.67; 95% CI, 0.48 to 0.93; P =.02) in patients with pMMR tumors. Patients with dMMR tumors receiving FU had no improvement in DFS (HR, 1.10; 95% CI, 0.42 to 2.91; P =.85) compared with those randomly assigned to surgery alone. In the pooled data set of 1,027 patients (n = 165 with dMMR), these findings were maintained; in patients with stage II disease and with dMMR tumors, treatment was associated with reduced overall survival (HR, 2.95; 95% CI, 1.02 to 8.54; P =.04). Patient stratification by MMR status may provide a more tailored approach to colon cancer adjuvant therapy. These data support MMR status assessment for patients being considered for FU therapy alone and consideration of MMR status in treatment decision making.
[J].
[本文引用: 1]
[J].
DOI:10.1126/science.aaf1490URL [本文引用: 1]
[J].
DOI:10.1002/(ISSN)1097-0142URL [本文引用: 1]
[J].
DOI:10.1056/NEJMoa1500596URL [本文引用: 1]
[J].
DOI:10.1038/bjc.2016.211URL [本文引用: 1]
[J].
DOI:10.1158/1078-0432.CCR-15-1678URL [本文引用: 1]
[J].
DOI:10.1016/S1525-1578(10)60611-3URL [本文引用: 1]
[J].
DOI:10.1097/PAS.0b013e3181b15aa2URL [本文引用: 1]
[J].
DOI:10.1093/bioinformatics/btt755URL [本文引用: 1]
[J].
DOI:10.1373/clinchem.2014.223677URL [本文引用: 1]
[J].
DOI:10.18632/oncotarget.13918PMID:27980218 [本文引用: 1]

In current clinical practice, microsatellite instability (MSI) and mismatch repair deficiency detection is performed with MSI-PCR and immunohistochemistry. Recent research has produced several computational tools for MSI detection with next-generation sequencing (NGS) data; however a comprehensive analysis of computational methods has not yet been performed. In this study, we introduce a new MSI detection tool, MANTIS, and demonstrate its favorable performance compared to the previously published tools mSINGS and MSISensor. We evaluated 458 normal-tumor sample pairs across six cancer subtypes, testing classification performance on variable numbers of target loci ranging from 10 to 2539. All three computational methods were found to be accurate, with MANTIS exhibiting the highest accuracy with 98.91% of samples from all six diseases classified correctly. MANTIS displayed superior performance among the three tools, having the highest overall sensitivity (MANTIS 97.18%, MSISensor 96.48%, mSINGS 76.06%) and specificity (MANTIS 99.68%, mSINGS 99.68%, MSISensor 98.73%) across six cancer types, even with loci panels of varying size. Additionally, MANTIS also had the lowest resource consumption (<1% of the space and <7% of the memory required by mSINGS) and fastest running times (49.6% and 8.7% of the running times of MSISensor and mSINGS, respectively). This study highlights the potential utility of MANTIS in classifying samples by MSI-status, allowing its incorporation into existing NGS pipelines.
[J].
[本文引用: 1]
[J].
[本文引用: 1]
[J].
DOI:10.1038/srep13321URL [本文引用: 1]
[J].
DOI:10.1038/s41598-018-35682-zURL [本文引用: 1]
[J].
DOI:10.1038/nm.4191URL [本文引用: 1]
[J].
DOI:10.1093/bioinformatics/btx507URL [本文引用: 1]
[J].
[本文引用: 1]
[J].
DOI:10.1007/s13277-012-0634-6URL [本文引用: 1]