
2.
3.
4.
Activity space-based segregation among neighbors and its influencing factors: An analysis based on shared activity spaces in suburban Shanghai
TA Na1,2, SHEN Yue
2.
3.
4.
通讯作者:
收稿日期:2018-11-27修回日期:2019-12-22网络出版日期:2020-04-25
基金资助: |
Received:2018-11-27Revised:2019-12-22Online:2020-04-25
Fund supported: |
作者简介 About authors
塔娜(1986-),女,内蒙古包头人,博士,副研究员,研究方向为城市社会地理学与行为地理学E-mail:nta@geo.ecnu.edu.cn。

摘要
关键词:
Abstract
Keywords:
PDF (1536KB)元数据多维度评价相关文章导出EndNote|Ris|Bibtex收藏本文
本文引用格式
塔娜, 申悦. 基于共享度的上海郊区社区居民活动空间隔离及其影响因素. 地理学报[J], 2020, 75(4): 849-859 doi:10.11821/dlxb202004013
TA Na.
1 引言
在全球化与社会经济转型的影响下,城市社会空间正在不断重构、破碎,展示出分异的格局。极端的社会空间分异可能形成隔离,并导致不同群体间的歧视性后果。在西方国家,社会空间分异与隔离已经成为一个重要的社会问题,不仅传统的种族隔离依然存在,因收入和教育水平差异而导致的居住隔离也在日益增加[1,2,3]。而在中国,城市社会空间分异伴随着阶层分化的加剧而凸显,门禁社区成为中高收入群体的“俱乐部”,经济适用房建设到城市边缘区,城中村成为外来人口的聚居地。在此背景下,以居住空间为对象的研究成为中西方社会空间分异与隔离研究的主流,****提出地理隔离加剧了弱势群体与其他群体的社会隔离[4,5],导致了相关的可达性、公共健康、空间错位等问题[6,7,8,9,10]。然而,社会空间分异与隔离不仅存在于居住空间,也存在于居民的日常活动与出行中[11,12,13,14]。在流动性日益增强与居民活动模式日益多样化的背景下[15,16,17],即使居住在同一社区的居民也会由于工作和非工作活动地点选择的差异而存在不同程度的隔离[11, 18],导致社会交往的可能性降低。因此,静态的基于居住空间的社会空间分异度量已经不能完全满足城市社会和社区研究的需要,有必要从活动空间角度分析居民在日常生活中面临的分异与隔离问题,从而更动态和全面地理解社会空间[14, 18-21]。作为城市时空行为模式的重要指标,活动空间是居民整日活动与出行的空间范围,反映了居民对城市空间的利用情况[21,22,23]。但由于尚处在方法的探索阶段,只有少数前沿研究对社区居民活动空间的隔离程度进行了定量测量[11,12,13,14]。
应用活动空间进行社会空间分异的研究,主要有两个维度。一是通过比较不同群体活动空间内部社会环境的差异,分析居民个体在其日常生活中与其他群体“相遇”的可能性[18, 21, 24]。例如,李菲等构建了活动空间分异指数和接触指数,分析个体与其活动空间内居住群体在社会经济属性方面的相似性与差异性,解释了个体层次的社会空间隔离及其影响机制[24]。这类研究利用普查数据将活动空间内的人群组成结构作为活动空间隔离的计算依据,具有数据相对易得、操作性强、与传统社会空间分异相联系的特点,但是并没有真实描述不同群体日常接触的状态。另一个维度是从个体行为数据出发,探讨不同个体活动空间的交互特征[25,26,27]。这些研究认为,由于就业、收入、政策、个体偏好等原因,即使居住在同一地点的两个人,其生活方式和活动空间也有巨大的差异[28],个体在活动空间中可以接触到的人群可能完全不同,导致邻里交往减少、社区社会融合降低。Park等使用时空临近性指数的概念,计算了一天不同时间内每个个体与其他群体的接触度,探讨了不同族裔群体社会交往的潜在可能性[12]。Browning等对同一社区居民的活动空间共享程度进行了研究,提出“共享活动空间”的概念,发现不同收入群体的活动空间存在较大差异[11]。这些研究更加强调以人为中心的度量,分析群体间活动空间的分异与交互程度,代表了社会空间隔离研究的新方向。但是由于数据获取难度较大,目前的研究还处在起步阶段。
对中国城市而言,在制度与市场的双重力量作用下,居民自由选择增加,社会分异正在拉大,有必要对不同群体的活动空间分异进行研究(表1)。居住空间研究以基于空间的度量为基本范式,更加关注居住问题,侧重于不同群体在住房上的分选过程,以社区为空间单元,认为社区和住房代表了个体在中长期尺度上的地位与资源可获得性,居住在不同社区的居民之间存在隔离。而活动空间研究则侧重基于人的度量,以个体活动空间为研究对象,认为即使居住在同一社区的居民也可能存在日常生活中的隔离,活动空间中的接触能减少隔离。随着以人为本理念的发展,基于人的空间研究范式将成为城市研究发展的重要方向,需要对这一研究领域进行更为深入的探索。
Tab. 1
表1
表1基于空间与基于人的社会空间分异度量
Tab. 1
基于空间的度量 | 基于人的度量 | |
---|---|---|
背景 | 城市化背景下中国城市社会分异出现,并通过住房选择在空间中表现出来 | 社会转型背景下中国城市生活方式差异出现,并通过日常活动的空间选择表现出来 |
假设 | 居住在同一社区的居民不存在隔离,社区间的差异带来了社会空间隔离 | 即使居住在同一社区的居民也可能存在日常生活中的隔离,活动空间中的接触能减少隔离 |
关注点 | 住房的空间分选过程 | 行为动态过程 |
时空间尺度 | 中长期、宏观 | 短期、微观 |
研究单元 | 社区或居住空间 | 活动空间 |
空间单元测量方法 | 居住社区、以家为中心的缓冲区 | 标准椭圆、最小凸多边形、最短路径面积、轨迹缓冲区等 |
影响因素 | 社区类型、个体社会经济属性、社区建成环境等 | 社区建成环境、个体社会经济属性、个体偏好等 |
新窗口打开|下载CSV
而面向实践应用,如何定量测量社区环境对活动空间隔离的调控作用也是规划与政策迫切需要解决的问题。在居住空间的视角下,****提出,混合居住政策能够增加不同群体的接触与交流[29,30]。然而,从空间行为视角出发,共同居住本身并不一定直接带来日常接触,而是需要居民在日常生活中通过共同利用社区空间和周边设施以形成交互。社区能否提供日常活动交互的潜在场所便成为了重要的考量,需要定量分析社区环境对不同群体活动空间共享的影响。
本文希望解答同一社区内不同居民在日常生活中是否存在活动空间隔离这一问题。本文利用活动空间共享度,分析上海市郊区同一社区内不同收入居民的活动空间隔离特征,建立个体尺度的活动空间分异指数和孤立指数,并通过回归模型分析活动空间共享度的影响因素。
2 数据来源与研究方法
2.1 数据来源
本文选取上海市郊区作为案例地,探究其社区居民活动空间分异与隔离状况。在社会经济转型与空间重构背景下,上海城市社会空间异质性突出,社区居民组成的多样性在增强[6],特别是在大型居住区建设背景下,不同来源、不同阶层的居民迁入郊区并形成复杂的邻里结构[31];郊区居民面临的长距离通勤、生活空间疏离、社区归属感不强等问题也日益明显[31,32,33],具有大城市快速城市化与郊区发展下新空间的典型特征。为此,上海市积极推动15 min生活圈建设,希望重构社区生活空间、建立居民情感纽带、提升社区归属感与活力。在此背景下,研究郊区社区居民活动空间的共享程度、探讨社区活动空间隔离,就具有理论与实践意义。本文以2017年4—7月进行的上海市居民日常活动与出行调查为基础开展研究。本次调查采用多阶段抽样方法,结合空间位置、区域发展及社区类型特征,在上海郊区(除崇明外)8个行政区选取10个镇,每个镇中选取不同类型社区,共选取58个社区作为调查的基本单元,在选取的社区中开展入户调查(图1为样本社区分布)。调查采用调查员半访谈式询问与手持移动设备调查相结合的方式,记录居民个人与家庭社会经济属性、两天活动日志、主观感受与情感体验、惯常活动等信息。经过两轮调查,共发放问卷1593份,其中有效问卷1140份,有效率71.56%。
图1
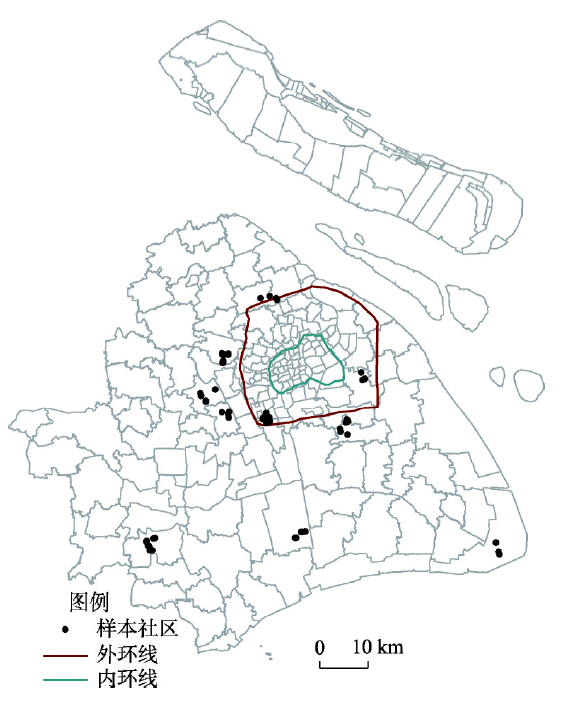
图1问卷抽样社区分布
Fig. 1Distribution of survey communities
本文关注社区内不同收入群体的活动空间隔离,故选取社会经济属性和惯常活动地点填写完整、居住在正规城市社区的居民,并去掉调查样本量过少的社区和学生群体。最终有效样本为748人,样本社会经济属性如表2所示。结合上海市2016年人均可支配收入、上海市廉租住房申请标准和困难家庭申请专项救助的收入标准等指标,按照人均月收入划分为三档,0~2500元为低收入,2501~7500元为中收入、7501元以上为高收入,不同收入群体比例为17∶59∶24,中等收入群体为主。本地居民为主体,男性略多于女性,年龄以30~49为主,教育程度以大专大学为主,研究生及以上人群偏少。
Tab. 2
表2
表2样本社会经济属性
Tab. 2
类别 | 样本量(人) | 比例(%) | |
---|---|---|---|
总样本 | 748 | 100 | |
性别 | 男 | 388 | 51.87 |
女 | 360 | 48.13 | |
教育程度 | 初中及以下 | 138 | 18.45 |
高中中专职高 | 154 | 20.59 | |
大专大学 | 419 | 56.01 | |
研究生及以上 | 37 | 4.95 | |
年龄(岁) | 18~29 | 178 | 23.80 |
30~39 | 283 | 37.83 | |
40~49 | 199 | 26.60 | |
50~60 | 88 | 11.77 | |
户口类型 | 上海 | 382 | 51.07 |
外地城市 | 212 | 28.34 | |
外地农村 | 154 | 20.59 | |
人均月收入 (元) | ≤ 2500 | 128 | 17.11 |
2501~7500 | 444 | 59.36 | |
≥ 7501 | 176 | 23.53 |
新窗口打开|下载CSV
2.2 研究方法
本文参考Browning等提出的共享活动空间概念[11],采用惯常活动地点分布信息,计算郊区居民的活动空间共享度,并在此基础上构建个体层级的活动空间分异指数和孤立指数。使用问卷中“每月至少去一次的活动地点”作为活动空间的基础点,将这些点在ArcGIS中数字化。由于本文的目的是研究社区居民在日常生活中是否存在活动空间隔离问题,而工作活动地点选择受到诸多因素的影响,且日常行为分布具有距离衰减性,经过比较,以社区中心点3 km范围内的非工作活动作为研究对象,分析社区尺度的活动空间共享度。样本平均报告非工作活动5.93个。首先,本文利用非工作活动地点的空间位置构建共享活动空间。共享活动空间用来衡量同一社区中的个体是否与其他群体到同一空间范围进行非工作活动。根据已有研究,居民共处于同一步行空间范围内交往和互动可能性会提升,****一般采用地块、普查区、200~400 m边长区域等空间单元[11, 24, 34-35]进行研究。综合考虑上海市郊区地块空间尺度和居民活动集中程度,本文选择200 m×200 m的空间网格作为基础分析单元,将社区中心点3公里范围内的空间分为若干个网格,所有的地点和网格相关联。每个居民的活动空间包括一个或多个网格,而每个网格与一个或多个收入群体相关联。每个网格按照活动空间内群体类型的组成被赋予一个共享活动空间指标值,分为单一群体独享、两群体共享和多群体共享3个类型,并分别取0、0.3和0.7作为权重计算活动空间共享度。共享度表达了不同群体活动空间分享的程度,用于分析是否存在活动空间分异与隔离。
其次,本文根据网格内不同群体活动的比例计算个体层次的活动空间分异指数Djm,衡量活动空间分异的均匀性维度。Djm代表了个体j活动空间内某一群体m与其他群体的分异程度,取值0~1,指数越大,活动空间内不同网格与总体平均值的偏离程度越高,活动分异程度越高。Djm的计算公式如下:
式中:i为样本j活动空间中网格编号;ti和pi分别为i网格中的总活动数和m群体活动数占该网格总活动数的比例;T和P为样本j活动空间内的活动总数和m群体活动数在活动总数中的比例。
最后,本文进一步计算个体层次的活动空间孤立指数Ijm,衡量活动空间分异的接触性维度。孤立指数越高,说明个体j活动空间内m群体比例越高、越孤立、与其他人群的联系越少。Ijm的计算公式如下:
式中:i为样本j活动空间中网格编号;xi和ti分别为i网格中的m群体活动数和总活动数;X为m群体在样本j活动空间内的总活动数。
3 活动空间共享度的群体分异
社区居民活动空间共享度相对较低,且群体之间存在显著差异(表3)。低收入群体的活动空间共享度为40.62%,高于其他收入群体,说明低收入群体更可能和其他群体共同出现在同一空间中。而中高收入群体的共享度偏低,特别是中等收入群体,他们与其他群体的活动空间共享度最低。Tab. 3
表3
表3不同收入群体的活动空间共享度及其差异检验
Tab. 3
群体差异 | 群体1占比(%) | 群体2占比(%) | 活动空间共享度差异(%) | P值 |
---|---|---|---|---|
中收入—低收入 | 31.24 | 40.62 | -9.38 | 0.000 |
高收入—低收入 | 34.75 | 40.62 | -5.87 | 0.007 |
高收入—中收入 | 34.75 | 31.24 | 3.51 | 0.047 |
新窗口打开|下载CSV
分析活动空间共享类型可以发现,居民更愿意与自己所属群体共享活动空间,并且这一模式具有群体差异特征(图2)。与低收入居民共享活动空间的有60%为低收入群体,显著高于其人口比例,而高收入群体仅有不到10%,说明低收入居民虽然活动空间共享度总体值较高,但依旧受到一定程度的隔离,特别是极少出现在高收入居民活动空间中。而与高收入居民共享活动空间的情况与之类似,出现了高收入群体自身的集聚,说明高收入居民由于其消费偏好、生活方式等原因出现了自我隔离[36,37]。中等收入居民的活动空间共享度则相对更加开放,他们出现在低收入和高收入群体活动空间中的比例远高于人口比例。多个群体共享的活动空间中,低收入出现的比例更高,这是由于他们更有可能到达社区居民共同出现的地点,比如菜市场、公园等公共领域。
图2
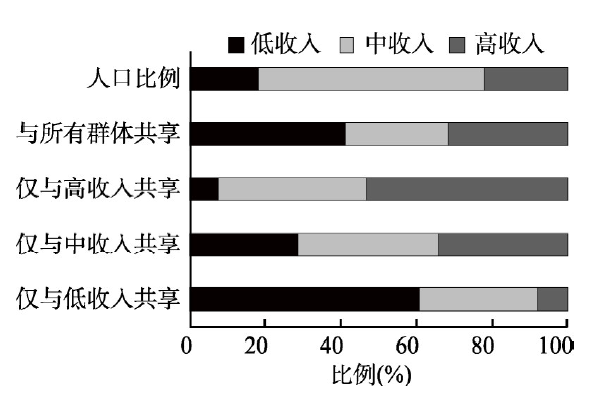
图2不同收入群体活动空间共享类型差异
Fig. 2Types of shared activity spaces among income groups
不同群体活动空间的分异指数和孤立指数也存在明显差异(图3)。分异指数表现了居民活动空间中不同群体的均匀性特征,高收入居民活动空间内各个群体的分异指数都比较高,而低收入居民活动空间内分异指数较低,说明在低收入居民的活动空间内不同群体的分布较为均衡而高收入居民活动空间则相反。孤立指数表现了居民活动空间中不同群体的比例和接触性。不同居民活动空间内其自身所在群体孤立指数都比较高,进一步说明个体都更有可能在其自身所属群体的活动空间内出现。在中高收入居民活动空间中,低收入群体指数较低,说明低收入者在其他群体活动空间内出现时,更有可能带来群体间接触,隔离性降低;中收入群体在所有居民活动空间内的孤立指数都很高,说明与其他群体接触少;高收入居民则存在自我隔离倾向,他们在自身群体的活动空间中表现出与其他群体接触较少的特征,但出现在其他居民活动空间中时,隔离倾向降低。
图3
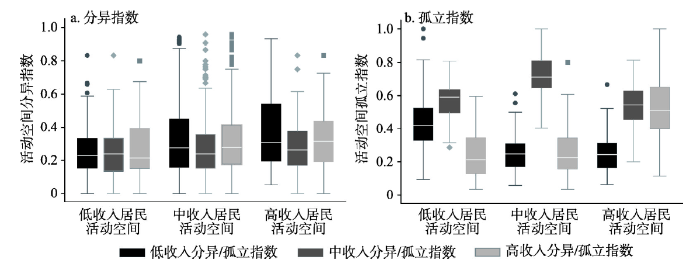
图3不同收入群体活动空间分异指数和孤立指数
Fig. 3Activity space differentiation index and isolation index
4 活动空间共享度的影响因素
为进一步分析活动空间共享度的影响因素,构建多层次序logit模型分析样本社会经济属性、社区内设施状况和社区周边设施状况对活动空间共享度的影响。因变量为活动空间共享度分级指标,参考不同收入群体活动空间共享度平均值及其分布特征,按照20%为一档,将活动空间共享度分为20%、21%~40%、41%~60%、61%以上共4档。上文发现不同群体在活动空间共享度上存在显著差异,故对全体样本、低收入、中收入和高收入分别建模,探究其影响因素。本文选取的自变量如表4所示。需要说明的是,社会环境包括了人口密度和社会混合度,混合度采用社区居民教育结构的熵值进行度量;建成环境包括了社区内部设施供给和社区周边设施密度状况,但是由于部分周边设施密度之间存在共线性,在最终模型中仅保留了购物中心密度和公园广场密度两个变量。Tab. 4
表4
表4居民活动空间共享度影响因素指标及描述性统计
Tab. 4
变量 | 意义 | 均值 | 标准差 |
---|---|---|---|
收入 | 居民平均月收入:1:低收入;2:中收入;3:高收入 | 2.06 | 0.02 |
户口 | 1:本地户口;2:外地城市户口;3:外地农村户口 | 1.69 | 0.03 |
女性 | 1:女性;0:男性 | 0.48 | 0.02 |
年龄 | 单位:岁 | 37.09 | 0.36 |
有正式就业 | 1:有正式就业;0:无正式就业 | 0.86 | 0.01 |
汽车所有权 | 1:有;0:无 | 0.62 | 0.02 |
居住两年以下 | 1:居住两年以下;0:居住两年以上 | 0.31 | 0.02 |
住房所有权 | 1:有住房所有权;0:无住房所有权 | 1.36 | 0.02 |
社区有围墙 | 1:有;0:无 | 0.93 | 0.01 |
社区有公共空间 | 如运动场、小广场等,1:有;0:无 | 0.93 | 0.01 |
社区有室内活动设施 | 如健身房、棋牌室等,1:有;0:无 | 0.70 | 0.02 |
社区有零售商业 | 如菜市场、小卖部等,1:有;0:无 | 0.62 | 0.02 |
社区社会混合度 | 根据六普数据计算社区不同教育水平人群的熵值来衡量社区混合程度,数值越高、社区居民组成混合程度越高 | 0.44 | 0.01 |
社区人口密度 | 社区人口数量除以社区面积计算,单位:万人/km2 | 1.91 | 0.08 |
周边便利店密度 | 社区周边3 km范围内便利店密度,单位:个/km2 | 5.25 | 0.13 |
周边餐饮设施密度 | 社区周边3 km范围内餐饮设施密度,单位:个/km2 | 48.48 | 0.95 |
周边超市密度 | 社区周边3 km范围内超市密度,单位:个/km2 | 4.40 | 0.11 |
周边公园广场密度 | 社区周边3 km范围内公园广场密度,单位:个/km2 | 0.28 | 0.01 |
周边购物中心密度 | 社区周边3 km范围内购物中心密度,单位:个/km2 | 0.30 | 0.01 |
周边室内体育休闲设施密度 | 社区周边3 km范围内室内体育休闲设施密度,单位:个/km2 | 8.01 | 0.20 |
到城市中心的距离 | 到人民广场的距离,对数形式 | 3.11 | 0.02 |
新窗口打开|下载CSV
从表5模型1可以看出个体收入对活动空间共享度确实有影响。与统计描述类似,相比于低收入居民,中高收入居民的共享度更低,说明较高收入的群体存在一定程度的自我隔离倾向。由于本文分析的是社区周边3 km的共享活动空间状况,这一结果一方面可能来源于中高收入居民倾向于到远离社区的中高级城市中心进行非工作活动;另一方面,低收入居民由于其非正规就业或者倾向于非机动出行等原因,更有可能在社区周边进行活动,所以与其他居民共享活动空间的可能性更高。
Tab. 5
表5
表5活动空间共享度影响因素多层次序logit模型
Tab. 5
模型1:总体活动空间共享度 | 模型2:低收入居民活动空间共享度 | 模型3:中收入居民活动空间共享度 | 模型4:高收入居民活动空间共享度 | ||||||||
---|---|---|---|---|---|---|---|---|---|---|---|
系数 | P值 | 系数 | P值 | 系数 | P值 | 系数 | P值 | ||||
收入(低收入为参照) | |||||||||||
中收入 | -1.34 | 0.000 | |||||||||
高收入 | -1.04 | 0.000 | |||||||||
户口(本地户口为参照) | |||||||||||
外地城市户口 | 0.59 | 0.004 | 0.97 | 0.158 | 0.75 | 0.006 | -0.06 | 0.887 | |||
外地农村户口 | 0.22 | 0.357 | -0.06 | 0.915 | 0.64 | 0.049 | -0.26 | 0.651 | |||
女性 | 0.19 | 0.213 | 0.16 | 0.717 | 0.16 | 0.433 | 0.09 | 0.784 | |||
年龄 | 0.00 | 0.884 | 0.00 | 0.855 | 0.00 | 0.884 | -0.01 | 0.560 | |||
有正式就业 | 0.44 | 0.065 | 0.51 | 0.264 | 0.60 | 0.082 | -0.45 | 0.583 | |||
汽车所有权 | -0.34 | 0.045 | -0.13 | 0.750 | -0.27 | 0.242 | -0.49 | 0.263 | |||
居住两年以下 | -0.29 | 0.125 | 0.07 | 0.882 | -0.36 | 0.156 | -0.73 | 0.071 | |||
住房所有权 | -0.38 | 0.061 | -0.71 | 0.189 | -0.54 | 0.049 | 0.19 | 0.670 | |||
社区有围墙 | -2.29 | 0.023 | -1.49 | 0.339 | -1.93 | 0.078 | -4.89 | 0.024 | |||
社区有公共空间 | 2.63 | 0.005 | 4.16 | 0.005 | 2.07 | 0.043 | 3.82 | 0.100 | |||
社区有室内活动设施 | 0.49 | 0.284 | -0.72 | 0.359 | 0.57 | 0.263 | 1.35 | 0.047 | |||
社区有零售商业 | 0.92 | 0.022 | 0.06 | 0.934 | 0.78 | 0.075 | 1.85 | 0.002 | |||
社区社会混合度 | 3.86 | 0.029 | 1.71 | 0.533 | 4.01 | 0.039 | 5.06 | 0.058 | |||
社区人口密度 | 0.30 | 0.002 | 0.29 | 0.058 | 0.22 | 0.046 | 0.60 | 0.001 | |||
周边公园广场密度 | 0.20 | 0.797 | -1.40 | 0.326 | 0.83 | 0.338 | -0.70 | 0.501 | |||
周边购物中心密度 | -4.10 | 0.000 | -2.83 | 0.122 | -5.32 | 0.000 | -3.49 | 0.009 | |||
到城市中心的距离 | -1.38 | 0.002 | -2.72 | 0.000 | -1.53 | 0.002 | -1.17 | 0.091 | |||
门槛值1 | -5.06 | 0.011 | -10.65 | 0.003 | -4.80 | 0.034 | -4.11 | 0.232 | |||
门槛值2 | -2.18 | 0.271 | -7.43 | 0.030 | -1.72 | 0.443 | -1.11 | 0.746 | |||
门槛值3 | 0.37 | 0.852 | -4.85 | 0.149 | 0.99 | 0.661 | 1.87 | 0.583 | |||
var(_cons) | 1.00 | 1.41 | 1.06 | 1.05 | |||||||
Waldchi2 | 91.63 | 27.03 | 53.72 | 34.38 | |||||||
Loglikelihood | -758.31 | -134.01 | -432.54 | -175.72 |
新窗口打开|下载CSV
户口、正式就业、汽车所有权、住房所有权对活动空间共享度有显著影响,但性别、年龄、居住时间统计没有显著的影响(表5)。对于社区总体样本,拥有外地城市户口和正式就业会提高居民活动空间共享程度,特别是对于中收入居民,有就业、外地户口的人比无就业、本地户口的人更愿意共享其活动空间,但是户口和就业状况对于低收入和高收入居民没有显著影响。拥有汽车和住房所有权会降低居民的活动空间共享度,说明当居民资产增多时,其有可能进行自我隔离,但是在分模型中住房所有权仅对中收入群体有显著影响。
社区设施配置会影响活动空间共享状况。门禁社区会减少不同收入群体之间接触的可能性,降低其活动空间的共享程度,特别是中高收入居民居住在门禁社区中隔离程度显著上升。小区内配置有公共空间、零售商业等,有可能提高不同收入居民的社会接触,但是商业设施配置仅对中高收入居民有显著影响。其原因在于小区层面的设施承接了最基础的生活需求,如日用品购买、遛弯、基础体育锻炼等,开敞公共空间和零售商业能够服务不同收入的人群,因而提高了他们社区内部活动的可能性,提高活动空间共享度,降低社会隔离。对于高收入居民来说,小区内有室内活动场所,可以提高其活动空间共享度。社会混合度和人口密度都对活动空间共享度有正向影响,人口密度越高、人群越混合,不同群体社会接触的潜在可能性上升。这说明建立社会群体混合的社区,更有可能促进不同群体之间的社会交流;但是,从不同收入居民的模型来看,社会混合度对提升低收入居民的活动空间共享度影响不显著。社区周边购物中心数量增加,不同收入居民接触的可能性降低,特别是会显著降低中高收入居民与其他群体接触的可能性。这可能是受到经济支付能力的影响,购物中心有可能形成经济分选,低收入居民对这类设施的利用率可能相对较低。而社区离城市中心越远,居民活动空间共享度更低。
5 结论与讨论
本文以上海市郊区为例实证研究了社区居民的活动空间隔离状况。研究表明,社区中不同收入居民的活动空间共享度总体特征、分组共享状况、分异指数与孤立指数都存在差异,且其共享程度受到社会经济属性、社区设施配置和周边设施密度的影响,居住在人口密度高、社会混合度高、社区商业配置良好、公共空间充足的开放社区确实能提高居民的活动空间共享度,增加不同群体社会接触的可能性,而社区周边配置购物中心和远离城市中心会导致活动空间隔离。本文采用空间网格构建了不同收入群体的共享活动空间指标用来度量活动空间隔离,刻画了个体活动空间的交互特征。该方法在已有研究[11-12, 25-27]基础上考虑了上海市郊区空间的特征,并基于此指标尝试建立了个体尺度的活动空间分异指数和孤立指数,评价个体活动空间隔离的均匀性和接触性特征,是个体活动空间隔离交互指标的一次新尝试。通过比较不同收入群体的活动空间共享度及其结构,本文发现不同收入居民活动空间共享程度存在差异,居民更愿意与其社会经济属性相似的人共享活动空间,这一发现与已有研究一致[11]。但是相比于以往研究关注社会经济属性对活动空间分异的影响[24],本文分析了社区社会与建成环境的影响,能够为社区生活圈规划提供建议。在社区生活圈规划中应加强基础设施和公共空间的配置,以提升不同群体生活空间的交互。
对于中国城市社会空间隔离研究,本文提供了一个基于活动分析社区居民社会空间隔离的新视角,有利于综合理解城市社会空间。中国城市转型不仅导致了居住分异与隔离,也形成了活动空间的地理分选。即使居住在同一社区的居民,由于就业选择和生活方式的差异而形成了完全不同的活动空间。基于活动空间的社会空间隔离的形成受到多方面因素的影响。① 社区环境会影响不同居民接触的可能性,居住在高人口密度、社会混合社区中的居民接触到不同群体的可能性增加,他们在日常生活中共享活动空间的可能性会上升;社区良好的设施配套也会促进不同群体的日常接触,配置公共活动空间和基础商业设施、建设开放社区更有可能塑造共享度更好的社区生活空间。② 户口等制度因素依旧是影响居民日常生活的重要因素,外地居民与本地居民在活动空间共享度上存在差异。③ 市场化增加了不同收入阶层在生活方式上的差异,高收入阶层开始出现符号消费、自我隔离的倾向,导致不同群体之间的分异与隔离进一步加剧。
当然,本文也存在以下不足。首先,本文只从活动点重合的角度分析了活动空间的共享程度,没有考虑活动类型特征,无法考察不同收入居民在共享活动空间中活动的分异。其次,本文只分析了空间分异,没有考虑活动的时间分异,不同收入居民对于同一地点的利用可能存在时间差。再次,小样本分析虽然有利于探索活动空间隔离的人群分异属性,但是不免存在样本结构和行为多样性方面的偏差,引入大样本分析可以对共享活动空间实现更全局和综合的研究,也是未来需要探索的方向。最后,活动空间分异还受到居民主观感受和评价的影响,需要更多访谈与案例研究对其机制进行深入剖析。未来对于基于活动空间的社会空间分异与隔离研究依旧需要加强,特别是对时空结构和活动属性的深化研究亟待开展。
参考文献 原文顺序
文献年度倒序
文中引用次数倒序
被引期刊影响因子
[本文引用: 1]
[本文引用: 1]
DOI:10.1086/657114URLPMID:21648248 [本文引用: 1]

This article investigates how the growth in income inequality from 1970 to 2000 affected patterns of income segregation along three dimensions: the spatial segregation of poverty and affluence, race-specific patterns of income segregation, and the geographic scale of income segregation. The evidence reveals a robust relationship between income inequality and income segregation, an effect that is larger for black families than for white families. In addition, income inequality affects income segregation primarily through its effect on the large-scale spatial segregation of affluence rather than by affecting the spatial segregation of poverty or by altering small-scale patterns of income segregation.
[本文引用: 1]
[本文引用: 1]
[本文引用: 1]
DOI:10.11821/dlxb201704003URL [本文引用: 1]

Spatial differentiation of urban housing prices is the marketization expression of the non-equilibrium allocation of residential space resources, which reflects the contrast between social groups belonging to different social classes in selection preference and demand for housing. There is a certain degree of correlational coupling between urban housing prices' spatial differentiation and residential spatial differentiation with respect to the mechanism and the pattern. The paper chooses 1204 commercial housing communities in Nanjing's urban center as its research object and constructs a characteristic variable index system of housing prices. The GWR model is used to analyze major factors that lead to spatial differentiation in housing prices, as well as their combination relationship and spatial-temporal dynamics. Results demonstrate that: (1) there are various factors affecting housing prices, their subsequent spatial differentiation, and they are likely to evolve over time. The dominant factors are the level of the community, school district resources, quality of the landscape, access to leisure facilities, and so on. These may mainly reflect housing consumers' earning capacity, values, residential environment demands, life style and cultural tastes. (2) The pattern of housing prices in Nanjing generally shows the spatial pattern of "circle + fan-shaped + enclave". High priced housing mainly includes new apartments and gated communities in the inner city, communities in elite primary and secondary school districts, high-grade gated communities close to the Ming City Wall, housing in the center of Hexi New Town, and landscaped villas in the urban periphery. (3) Differing types and strata of housing communities attract and gather consumer groups with specific economic and social attributes, which makes housing price differentiation notable correlational coupling with residential differentiation in acting mechanisms and spatial patterns. As the degree of urban housing marketization is continuously deepening and the cultural characteristics and residential preference of social classes become increasingly mature, the spatial differentiation of urban housing prices is more than just an expression. It is also an important driving mechanism to promote residential spatial differentiation and re-differentiation, and can also predict basic trends pertaining to urban residential spatial differentiation in the future. Therefore, the spatial differentiation of urban housing prices can provide a prospective observational and analytical tool for the study of urban social space. It can further make up the time-lag defect which arises in traditional social space research using census data, as well as helps to predict and identify future trends regarding urban social spatial differentiation.
DOI:10.11821/dlxb201704003URL [本文引用: 1]

Spatial differentiation of urban housing prices is the marketization expression of the non-equilibrium allocation of residential space resources, which reflects the contrast between social groups belonging to different social classes in selection preference and demand for housing. There is a certain degree of correlational coupling between urban housing prices' spatial differentiation and residential spatial differentiation with respect to the mechanism and the pattern. The paper chooses 1204 commercial housing communities in Nanjing's urban center as its research object and constructs a characteristic variable index system of housing prices. The GWR model is used to analyze major factors that lead to spatial differentiation in housing prices, as well as their combination relationship and spatial-temporal dynamics. Results demonstrate that: (1) there are various factors affecting housing prices, their subsequent spatial differentiation, and they are likely to evolve over time. The dominant factors are the level of the community, school district resources, quality of the landscape, access to leisure facilities, and so on. These may mainly reflect housing consumers' earning capacity, values, residential environment demands, life style and cultural tastes. (2) The pattern of housing prices in Nanjing generally shows the spatial pattern of "circle + fan-shaped + enclave". High priced housing mainly includes new apartments and gated communities in the inner city, communities in elite primary and secondary school districts, high-grade gated communities close to the Ming City Wall, housing in the center of Hexi New Town, and landscaped villas in the urban periphery. (3) Differing types and strata of housing communities attract and gather consumer groups with specific economic and social attributes, which makes housing price differentiation notable correlational coupling with residential differentiation in acting mechanisms and spatial patterns. As the degree of urban housing marketization is continuously deepening and the cultural characteristics and residential preference of social classes become increasingly mature, the spatial differentiation of urban housing prices is more than just an expression. It is also an important driving mechanism to promote residential spatial differentiation and re-differentiation, and can also predict basic trends pertaining to urban residential spatial differentiation in the future. Therefore, the spatial differentiation of urban housing prices can provide a prospective observational and analytical tool for the study of urban social space. It can further make up the time-lag defect which arises in traditional social space research using census data, as well as helps to predict and identify future trends regarding urban social spatial differentiation.
[本文引用: 2]
[本文引用: 1]
[本文引用: 1]
[本文引用: 1]
[本文引用: 1]
DOI:10.1016/j.cities.2014.12.008URL [本文引用: 1]
[本文引用: 1]
DOI:10.7758/RSF.2017.3.2.09URLPMID:29034322 [本文引用: 8]

Residential segregation by income and education is increasing alongside slowly declining black-white segregation. Segregation in urban neighborhood residents' non-home activity spaces has not been explored. How integrated are the daily routines of people who live in the same neighborhood? Are people with different socioeconomic backgrounds that live near one another less likely to share routine activity locations than those of similar education or income? Do these patterns vary across the socioeconomic continuum or by neighborhood structure? The analyses draw on unique data from the Los Angeles Family and Neighborhood Survey that identify the location where residents engage in routine activities. Using multilevel p2 (network) models, we analyze pairs of households located in the same neighborhood and examine whether the dyad combinations across three levels of SES conduct routine activities in the same location, and whether neighbor socioeconomic similarity in the co-location of routine activities is dependent on the level of neighborhood socioeconomic inequality and trust. Results indicate that, on average, increasing SES diminishes the likelihood of sharing activity locations with any SES group. This pattern is most pronounced in neighborhoods characterized by high levels of socioeconomic inequality. Neighborhood trust explains a nontrivial proportion of the inequality effect on the extent of routine activity sorting by SES. Thus stark, visible neighborhood-level inequality by SES may lead to enhanced effects of distrust on the willingness to share routines across class.
[本文引用: 4]
DOI:10.1007/s13524-014-0283-zURLPMID:24719273 [本文引用: 2]

Research on neighborhood effects has focused largely on residential neighborhoods, but people are exposed to many other places in the course of their daily lives-at school, at work, when shopping, and so on. Thus, studies of residential neighborhoods consider only a subset of the social-spatial environment affecting individuals. In this article, we examine the characteristics of adults' "activity spaces"-spaces defined by locations that individuals visit regularly-in Los Angeles County, California. Using geographic information system (GIS) methods, we define activity spaces in two ways and estimate their socioeconomic characteristics. Our research has two goals. First, we determine whether residential neighborhoods represent the social conditions to which adults are exposed in the course of their regular activities. Second, we evaluate whether particular groups are exposed to a broader or narrower range of social contexts in the course of their daily activities. We find that activity spaces are substantially more heterogeneous in terms of key social characteristics, compared to residential neighborhoods. However, the characteristics of both home neighborhoods and activity spaces are closely associated with individual characteristics. Our results suggest that most people experience substantial segregation across the range of spaces in their daily lives, not just at home.
[本文引用: 3]
[本文引用: 1]
[本文引用: 1]
[本文引用: 1]
[本文引用: 1]
[本文引用: 1]
[本文引用: 1]
DOI:10.1007/s10109-010-0112-xURLPMID:21643546 [本文引用: 3]

While the literature clearly acknowledges that individuals may experience different levels of segregation across their various socio-geographical spaces, most measures of segregation are intended to be used in the residential space. Using spatially aggregated data to evaluate segregation in the residential space has been the norm and thus individual's segregation experiences in other socio-geographical spaces are often de-emphasized or ignored. This paper attempts to provide a more comprehensive approach in evaluating segregation beyond the residential space. The entire activity spaces of individuals are taken into account with individuals serving as the building blocks of the analysis. The measurement principle is based upon the exposure dimension of segregation. The proposed measure reflects the exposure of individuals of a referenced group in a neighborhood to the populations of other groups that are found within the activity spaces of individuals in the referenced group. Using the travel diary data collected from the tri-county area in southeast Florida and the imputed racial-ethnic data, this paper demonstrates how the proposed segregation measurement approach goes beyond just measuring population distribution patterns in the residential space and can provide a more comprehensive evaluation of segregation by considering various socio-geographical spaces.
DOI:10.1016/j.jtrangeo.2015.10.009URL
[本文引用: 3]
[本文引用: 3]
[本文引用: 1]
DOI:10.1111/j.1435-5597.1970.tb01464.xURL [本文引用: 1]
DOI:10.1177/0308518X16673213URL [本文引用: 4]
[本文引用: 2]
DOI:10.1080/00045608.2012.689238URL [本文引用: 1]
DOI:10.2747/0272-3638.33.2.256URL [本文引用: 2]

The widespread development of gated communities has generated much concern over urban fragmentation and social segregation. The social division and segregation between residents inside and outside urban enclaves exist not only in their residential spaces, but also in their values, social relations, and daily lives. In this study, it is argued that sociospatial segregation research should pay more attention to individuals' actual usage of urban space in their daily lives. By examining the activity space of the residents from different types of neighborhoods, a spatiotemporal approach to studying sociospatial segregation in Beijing, China is described. Significant differences are found in the usage of time and space between residents inside and outside the so-called privileged enclaves. Their activity spaces are found to vary significantly in terms of extensity, intensity, and exclusivity. The study suggests that the fragmentation of urban space is the result not only of residential segregation, but also of how different social groups spend their time and use urban space.
DOI:10.11821/dlxb201508007URL [本文引用: 1]

Rapid suburbanization in China is dramatically reshaping the daily life of suburban residents. Characterized by fragmentation, increasing car ownership and job-housing separation, suburbanization is changing the way residents use urban space. In China, long-distance commuting, traffic congestion, spatial mismatch and the low quality of life emerging in the progress of residential suburbanization have caused widespread concerns. Suburbanization of residents' daily life is believed to be a solution to the socio-spatial issues in suburbs. Therefore, scholars have argued that there is an urgent need to introduce behavioral perspectives to the study of suburbanization. This approach will enhance our understanding of the process and the mechanism of suburbanization from the perspective of an individual’s daily life. The important step in this study is to portray the nature of the daily lifestyle in the suburbs. Along with the trend toward behavioral perspectives in human geography, space-time behavior has become an important perspective for the study of urban space. Activity space and travel behavior often serve as important indicators of individual behavior in urban and geographic studies. This study focuses on daily activity-travel behavior, introduces the concept of lifestyle, and brings forward a concept of daily lifestyle based on a combined measure of activity space and trip frequency. The daily lifestyle of suburban residents can be classified into five categories: localization, spatial exclusion, suburbanism, polarization, and city dependence, according to the size of the activity space and the trip frequency. Based on a GPS-facilitated activity-travel survey dataset collected in the Shangdi-Qinghe area in Beijing in 2012, this paper compares the differences in activity-travel behavior among five daily lifestyle groups. The study finds that there are intergroup differences in the spatial distribution of activities, activity participation and modal split among these five lifestyle groups. Furthermore, this paper analyzes the impact of suburbanization on daily lifestyle by examining the relationship between daily lifestyle and built environment while controlling socio-demographic factors using a multinomial logistic model. Women and older people are more likely to be in the categories of localization and spatial exclusion. Low-income people have the higher possibility to be spatially excluded. These outcomes indicate that traditionally disadvantaged groups could be trapped in suburban areas, raising definite concerns for their daily life in suburban areas. Factors associated with the built environment primarily impact the localization lifestyle. Mixed land use in danwei and higher retail density contribute to the concentration of activity space in suburbs, as well as more active travel. The concept of daily lifestyle provides an effective and reasonable way to understand complicated activity-travel behavior of suburban residents. The paper offers a special perspective on understanding suburbanization and the interaction between urban space and individual behavior.
DOI:10.11821/dlxb201508007URL [本文引用: 1]

Rapid suburbanization in China is dramatically reshaping the daily life of suburban residents. Characterized by fragmentation, increasing car ownership and job-housing separation, suburbanization is changing the way residents use urban space. In China, long-distance commuting, traffic congestion, spatial mismatch and the low quality of life emerging in the progress of residential suburbanization have caused widespread concerns. Suburbanization of residents' daily life is believed to be a solution to the socio-spatial issues in suburbs. Therefore, scholars have argued that there is an urgent need to introduce behavioral perspectives to the study of suburbanization. This approach will enhance our understanding of the process and the mechanism of suburbanization from the perspective of an individual’s daily life. The important step in this study is to portray the nature of the daily lifestyle in the suburbs. Along with the trend toward behavioral perspectives in human geography, space-time behavior has become an important perspective for the study of urban space. Activity space and travel behavior often serve as important indicators of individual behavior in urban and geographic studies. This study focuses on daily activity-travel behavior, introduces the concept of lifestyle, and brings forward a concept of daily lifestyle based on a combined measure of activity space and trip frequency. The daily lifestyle of suburban residents can be classified into five categories: localization, spatial exclusion, suburbanism, polarization, and city dependence, according to the size of the activity space and the trip frequency. Based on a GPS-facilitated activity-travel survey dataset collected in the Shangdi-Qinghe area in Beijing in 2012, this paper compares the differences in activity-travel behavior among five daily lifestyle groups. The study finds that there are intergroup differences in the spatial distribution of activities, activity participation and modal split among these five lifestyle groups. Furthermore, this paper analyzes the impact of suburbanization on daily lifestyle by examining the relationship between daily lifestyle and built environment while controlling socio-demographic factors using a multinomial logistic model. Women and older people are more likely to be in the categories of localization and spatial exclusion. Low-income people have the higher possibility to be spatially excluded. These outcomes indicate that traditionally disadvantaged groups could be trapped in suburban areas, raising definite concerns for their daily life in suburban areas. Factors associated with the built environment primarily impact the localization lifestyle. Mixed land use in danwei and higher retail density contribute to the concentration of activity space in suburbs, as well as more active travel. The concept of daily lifestyle provides an effective and reasonable way to understand complicated activity-travel behavior of suburban residents. The paper offers a special perspective on understanding suburbanization and the interaction between urban space and individual behavior.
[本文引用: 1]
[本文引用: 1]
[本文引用: 1]
[本文引用: 2]
[本文引用: 2]
[本文引用: 1]
[本文引用: 1]
[本文引用: 1]
[本文引用: 1]
DOI:10.1016/j.healthplace.2017.02.004URLPMID:28237743 [本文引用: 1]

There is growing international evidence that supportive built environments encourage active travel such as walking. An unsettled question is the role of geographic regions for analyzing the relationship between the built environment and active travel. This paper examines the geographic region question by assessing walking trip models that use two different regions: walking activity spaces and self-defined neighborhoods. We also use two types of built environment metrics, perceived and audit data, and two types of study design, cross-sectional and longitudinal, to assess these regions. We find that the built environment associations with walking are dependent on the type of metric and the type of model. Audit measures summarized within walking activity spaces better explain walking trips compared to audit measures within self-defined neighborhoods. Perceived measures summarized within self-defined neighborhoods have mixed results. Finally, results differ based on study design. This suggests that results may not be comparable among different regions, metrics and designs; researchers need to consider carefully these choices when assessing active travel correlates.
[本文引用: 1]
[本文引用: 1]
[本文引用: 1]
[本文引用: 1]