
2.
3.
Key factors affecting the spatial variation of summer maize yield in Henan Province, China
WU Zhenfu1, ZHAO Yanfeng2, CHENG Daoquan3, CHEN Jie
2.
3.
通讯作者:
收稿日期:2019-03-22修回日期:2019-06-11网络出版日期:2019-10-29
基金资助: |
Received:2019-03-22Revised:2019-06-11Online:2019-10-29
作者简介 About authors
巫振富,男,广西八步人,博士生,从事土地资源可持续利用研究E-mail:

摘要
关键词:
Abstract
Keywords:
PDF (18283KB)元数据多维度评价相关文章导出EndNote|Ris|Bibtex收藏本文
本文引用格式
巫振富, 赵彦锋, 程道全, 陈杰. 河南省夏玉米产量空间分布特征及其影响因素. 资源科学[J], 2019, 41(10): 1935-1948 doi:10.18402/resci.2019.10.15
WU Zhenfu.
1 引言
作物产量及其空间分异特征是区域农田自然资源禀赋、物质技术投入、作物品种质量以及农艺管理措施等多种因素共同作用的结果,系统分析和定量表达这些因素的影响,对于开展粮食生产功能区规划与基本农田划定、中低产田改良与高标准粮田建设、因地制宜选择种植制度与作物品种、全面实施“藏粮于地,藏粮于技”战略具有重要参考意义。作物产量影响因素研究长期以来一直是农学等相关学科门类的一个热点研究领域。总结和分析以往的研究案例发现,绝大多数研究可以归入两种类型:一类是集中研究案例地区施肥[1,2,3,4]、土壤[5,6]、气候[7,8,9,10,11,12,13]、品种[14,15,16]、灌溉[17,18]等某一种因素对作物产量的影响,如鲁艳红等[1]通过长期定位肥料试验和盆栽试验结果,发现氮磷钾肥或氮磷钾肥配施稻草均能提高双季稻产量;檀满枝等[5]应用模糊C均值算法,在河南省封丘案例区证明了土壤剖面质地构型对小麦产量的显著影响;戴彤等[13]分析了气候因素对中国西南地区1961—2010年春玉米产量的影响,指出生长季辐射降低、温度升高以及降水减少等气候因子的变化造成作物减产;Qian等[14]基于中国1980—2013年间玉米产量数据,利用一系列方法量化了品种对玉米增产的贡献,证实了玉米品种更新在以往20多年玉米产量提升过程中的重要作用;Corcoles等[18]在发现案例区甜玉米的产量与品质受灌溉方案显著影响的基础上,进一步确定了甜玉米产量最高、品质最优的当季度最佳灌溉水量。另一类则集中分析和研究不同因素在影响作物产量时的交互作用,但涉及的因素通常不超过2个[19,20,21,22,23,24]。例如Sun等[19]的研究表明,气候变化和管理措施这两类因素对华北平原夏玉米产量产生影响,通过优化播种日期和改善种植密度等适应措施,能有效降低由气候变化导致的夏玉米产量波动;Iizumi等[20]系统分析和评估了1980—2006年气候变化对美国玉米种植区作物产量的影响,结果发现气候变化引起玉米减产,但将玉米播种期适当提前可有效消除该减产现象;康利允等[24]对比分析了不同施磷和灌溉处理下的冬小麦产量,发现在土壤水分供应不足时,磷肥深施有助于作物根系的生长发育,从而提高作物对土壤水分、养分的吸收利用能力,有助于形成高产。
此外,也有****对作物产量的多种影响因素[25,26,27,28,29]进行了有益探讨,如Tamene等[25]在马拉维共和国代扎地区(Dedza)的研究结果表明,土壤养分状况、施肥等农艺措施、家庭财富等社会经济因素均可能对该区农户种植的玉米产量产生重要影响;Farmaha等[26]在美国内布拉斯加州(Nebraska)的研究发现,玉米高产通常与大豆-玉米轮作方式、适宜耕种的土壤条件、较高的氮肥投入以及可靠的灌溉保证率等因素密切相关。在作物产量多因素影响研究方面,大多数案例通常是基于不同产量水平下相关各因素的取值进行简单的分析与评价。
总体而言,目前尚无法完全厘清和定量描述多种因素对作物产量及其空间分异的相对贡献。玉米是全球重要的经、饲、粮作物,是产量最大的谷物类型,全球约23%的玉米产自中国[30]。河南省作为中国13个粮食主产区之一,生产了全国超过8%的玉米[31]。本文以河南省2008—2010年夏玉米产量数据为基础,定量分析与评价气候、土壤、品种、施肥、灌排等自然条件与社会经济因素对作物产量及其空间分异的影响程度,为提高作物种植水平、优化水肥资源管理、提升耕地基础地力、建设高产稳产的高标准粮田提供理论与技术支持。
2 数据来源与研究方法
2.1 数据来源及处理
本文基础数据主要包括河南省2008—2010年夏玉米产量、作物品种类型、肥料结构和施用量、夏玉米种植区域的土壤性质和田间灌排设施条件、夏玉米生长季气候条件,数据来源和处理过程如下:(1)作物品种、施肥量(kg/hm2)和对应的夏玉米产量(kg/hm2)。为验证河南省县域测土配方施肥效果,2008—2010年各县农业局进行了三区示范田间试验,即不施肥处理的空白对照区(Control Check, CK)、按照当地农民习惯进行施肥处理的传统施肥区(Conventional Fertilization Treatment, CF)、根据土壤化验结果优化施肥处理的配方施肥区(formulated Fertilization Treatment, FF)。CK、CF和FF处理均详细记录了每年作物品种、氮肥(N)、磷肥(P2O5)和钾肥(K2O)施用量以及与之对应的作物产量。收集整理633个(2008年)、716(2009年)和694个(2010年)河南省夏玉米三区示范田间试验样点的上述数据(图1),并按80%与20%的比例分年度将试验样点随机划分为训练样点和验证样点。
图1
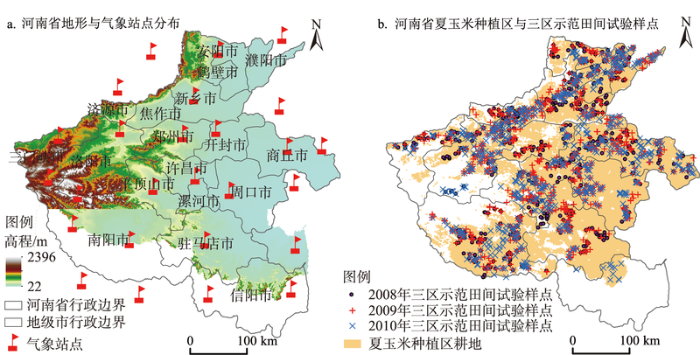
图1河南省夏玉米种植区以及气象站点与三区示范田间试验样点空间分布
Figure 1Spatial distribution of summer maize planting areas, meteorological stations, and field fertilization experiment sites in Henan Province
(2)土壤及农田灌排状况。土壤类型与土壤剖面性状、耕层土壤有机质、全氮、有效磷、速效钾含量、灌溉保证率和排涝能力等数据与信息均来自河南省农业厅2014年建立的全省耕地地力评价基础数据库。其中,土壤剖面性状系指在省域耕地地力评价中综合反映质地构型、障碍性状、土体厚度等影响农业生产性能的土壤剖面信息[32]。
(3)气象数据。通过整理中国地面气候资料日值数据集(V3.0)中的逐日气象资料(http://data.cma.cn/site/index.html)获得。为保证研究区边界区域气象数据的精度,将河南省境内及周边26个基准、基本气象站点共同作为本文的气象数据源(图1)。选取河南省2008—2010年夏玉米生育期内(6—9月)与作物产量形成密切相关的日照时数、降水量、蒸发量、≥10℃积温以及10~30℃积温作为本文气候因素[33]。按照空间距离最小、地形地貌相似的原则,将研究区各站点气候因素计算后赋值给不同空间位置。
为实施夏玉米产量空间预测,将河南省夏玉米种植区耕地进行2 km×2 km格网化处理,并将影响夏玉米产量的因素(不包括作物品种)按照空间位置一致性原则赋值给相应位置的格网。
2.2 研究方法
本文采用Boruta特征选择算法定量评价影响因素对夏玉米产量及其空间分异的重要性;采用随机森林(Random Forest,RF)模型计算不同因素组别对夏玉米产量及其空间分异的解释百分比,并对研究区夏玉米产量进行空间预测。2.2.1 Boruta特征选择
Boruta是围绕随机森林(Random Forest,RF)构建的用于特征选择的一种包装算法。本文应用Boruta特征选择算法定量评价研究区田间试验样点土壤类型、日照时数、作物品种、N施用量等17个影响因素对CK、CF和FF三种不同处理下夏玉米产量及其空间分异的重要性程度。
基于“RF中单棵树之间不相关”的假设,RF自身具有特征重要性评价功能[34],但是相关研究[35,36,37]表明这种假设并不总是成立。Boruta算法通过向原始特征(Original Features)增加随机性有效解决了这个问题,其核心思想如下:首先,通过随机复制原始特征创建阴影特征(Shadow Features),并将两者合并构建扩展特征(Extended Features);其次,基于扩展特征训练RF模型,以重要性指数(Z-score)为指标评价原始特征与阴影特征的重要性,Z-score越高代表越重要;第三,比较每个原始特征与阴影特征的相对重要性,只有重要性高于所有阴影特征,即高于最好阴影特征(shadowmax),的原始特征才被判定为重要特征[38]。
2.2.2 随机森林模型
RF是一种多功能机器学习算法,既是一种能够执行回归和分类任务的弱无偏分类器集合,同时它也是一种有效的数据降维手段。RF通过组合一定数量的分类器,能有效解决单一决策树分类规则复杂、局部最优解、过度拟合等一系列问题[34]。决策树的数量ntree和每棵决策树节点分裂时选用的随机特征数量mtry是RF建模的两个关键参数,本文参考以往相关研究,设置ntree=1000、mtry=log2 M+ 1(M为RF模型选定的特征总数量)[39]。
将本文选定的17个可能的产量影响因素划分为土壤、气候、灌排、品种、施肥等5个组别,以夏玉米产量为目标变量,依次以各组别因素和全部因素作为特征变量构建RF模型。基于RF模型袋外样本数据(Out-of-bag,OOB),根据公式(1)、(2)求算不同组别因素和全部因素对夏玉米产量及其空间分异的解释比PEVar:
式中:MSROOB表示对残差平方的无偏估计;k为训练样点数量(本文2008年、2009年、2010年k值分别为506、573、555);
式中:PEVar为特征变量对夏玉米产量空间分异的解释百分比;
最后,基于Boruta特征选择结果,分别构建CK、CF和FF三种处理下夏玉米产量关于重要特征变量(不含作物品种)的RF模型,对河南省夏玉米种植区进行产量空间预测。
河南省三区示范田间试验样点施肥量和耕地地力调查评价农化样点土壤养分空间插值(相关方法参考文献[40]和[41],插值结果为2 km×2 km栅格图)、夏玉米产量空间分布图绘制均利用ArcGIS 10.1完成。本文涉及的Boruta和Random Forest相关分析计算在软件R 3.5.1环境中完成,分析结果整理和图表绘制使用Microsoft Excel完成。
3 结果与分析
3.1 施肥量和夏玉米产量统计特征
研究区2008年、2009年、2010年三区示范田间试验样点CF和FF处理的施肥量统计结果显示(表1),CF偏重施N,轻施P2O5、K2O特征明显,FF则更注重在肥料类型与施用量两个方面优化N、P2O5、K2O结构。以2008年抽选的506个训练样点为例,FF采用N、P2O5、K2O配施的样点数为499个,配施样点比例远大于CF,并且FF处理在减小N施用量的同时,增加了P2O5、K2O施用量。本文在三区示范田间试验实施期间,CF处理总施肥量从2008年的287 kg/hm2逐年增加至2010年的307 kg/hm2,而FF处理则通过不断优化调整施肥结构的方式,将施肥总量从2008年的329 kg/hm2减至2010年的299 kg/hm2。Table 1
表1
表1研究区三区示范田间试验样点施肥量统计特征
Table 1
肥料类型 | 样点数 | N | P2O5 | K2O | N、P2O5、K2O总用量平均值 /(kg/hm2) | |||||||
---|---|---|---|---|---|---|---|---|---|---|---|---|
平均值 /(kg/hm2) | 变异系数/% | 平均值 /(kg/hm2) | 变异系数/% | 平均值 /(kg/hm2) | 变异系数/% | |||||||
2008年 | CF | NPK | 230 | 218 | 25.41 | 56 | 49.18 | 50 | 62.26 | — | ||
NP | 74 | 213 | 17.15 | 70 | 58.99 | — | — | — | ||||
NK | 9 | 198 | 25.30 | — | — | 35 | 44.26 | — | ||||
N | 193 | 248 | 26.11 | — | — | — | — | — | ||||
小计 | 506 | 228 | 25.78 | 36 | 107.16 | 23 | 139.30 | 287 | ||||
FF | NPK | 499 | 203 | 23.06 | 64 | 34.78 | 63 | 39.59 | — | |||
NP | 7 | 166 | 20.98 | 43 | 31.49 | — | — | — | ||||
小计 | 506 | 203 | 23.13 | 64 | 34.99 | 62 | 41.59 | 329 | ||||
2009年 | CF | NPK | 274 | 219 | 26.62 | 50 | 47.42 | 45 | 45.65 | — | ||
NP | 58 | 230 | 16.25 | 58 | 52.13 | — | — | — | ||||
NK | 9 | 233 | 11.83 | — | — | 41 | 16.91 | — | ||||
N | 232 | 265 | 22.28 | — | — | — | — | — | ||||
小计 | 573 | 239 | 25.29 | 30 | 106.71 | 22 | 119.92 | 291 | ||||
FF | NPK | 510 | 211 | 21.72 | 58 | 42.79 | 55 | 36.99 | — | |||
NP | 30 | 168 | 15.62 | 41 | 39.59 | — | — | — | ||||
NK | 22 | 187 | 13.23 | — | — | 37 | 24.93 | — | ||||
N | 11 | 252 | 17.20 | — | — | — | — | — | ||||
小计 | 573 | 209 | 22.01 | 54 | 51.02 | 51 | 47.77 | 313 | ||||
2010年 | CF | NPK | 255 | 232 | 32.61 | 62 | 54.03 | 52 | 49.85 | — | ||
NP | 65 | 231 | 17.05 | 66 | 46.91 | — | — | — | ||||
NK | 15 | 249 | 8.25 | — | — | 43 | 24.63 | — | ||||
N | 217 | 266 | 29.19 | — | — | — | — | — | ||||
小计 | 552 | 246 | 30.13 | 36 | 109.62 | 25 | 124.14 | 307 | ||||
FF | NPK | 491 | 211 | 23.42 | 48 | 35.42 | 52 | 37.96 | — | |||
NP | 30 | 144 | 47.84 | 49 | 34.78 | — | — | — | ||||
NK | 14 | 197 | 12.96 | — | — | 32 | 26.73 | — | ||||
N | 17 | 222 | 27.94 | — | — | — | — | — | ||||
小计 | 552 | 207 | 25.48 | 45 | 43.96 | 47 | 50.50 | 299 |
新窗口打开|下载CSV
训练样点的夏玉米产量统计结果见表2,CK、CF和FF三种处理下夏玉米产量依次增大的同时,各样点之间产量变异系数依次减小。上述结果表明,施肥明显提高了夏玉米产量,同时能有效提高夏玉米产量在空间上的稳定性;比较而言,FF处理对夏玉米产量及其空间稳定性的提升效果明显好于CF处理。
Table 2
表2
表2研究区三区示范田间试验样点夏玉米产量统计特征
Table 2
年份 | 施肥处理 | 最低产量/(kg/hm2) | 平均产量/(kg/hm2) | 最高产量/(kg/hm2) | 标准差/(kg/hm2) | 变异系数/% |
---|---|---|---|---|---|---|
2008年 | CK | 2460 | 6020 | 10485 | 1429 | 23.74 |
CF | 3240 | 7630 | 11550 | 1355 | 17.76 | |
FF | 4509 | 8460 | 12495 | 1410 | 16.67 | |
2009年 | CK | 1889 | 5826 | 11505 | 1445 | 24.80 |
CF | 2154 | 7455 | 12150 | 1367 | 18.34 | |
FF | 2334 | 8137 | 13395 | 1430 | 17.57 | |
2010年 | CK | 2906 | 5787 | 11595 | 1282 | 22.15 |
CF | 4050 | 7332 | 11645 | 1345 | 18.34 | |
FF | 4200 | 7992 | 12338 | 1431 | 17.91 |
新窗口打开|下载CSV
3.2 不同因素对夏玉米产量空间分异的重要性分析
Boruta分析结果显示(图2),2008—2010年研究区CK处理下三区试验样点的蒸发量、降水量、土壤类型、10~30℃积温、日照时数、有机质、作物品种、全氮、≥10℃积温、速效钾、灌溉保证率、土壤剖面性状、排涝能力和有效磷等14个因素的重要性指数(Z-score)均大于最好阴影特征(shadowmax),表明这些因素对玉米产量及空间分异具有重要影响。CF和FF处理下,除上述14个因素外,三区试验样点的N、P2O5、K2O施用量同样对夏玉米产量及空间分异有重要影响。就管理有关因素而言,任何处理下,灌溉保证率的重要性均大于排涝能力;施肥处理下,无论是CF还是FF,N肥施用量的重要性均最大。图2
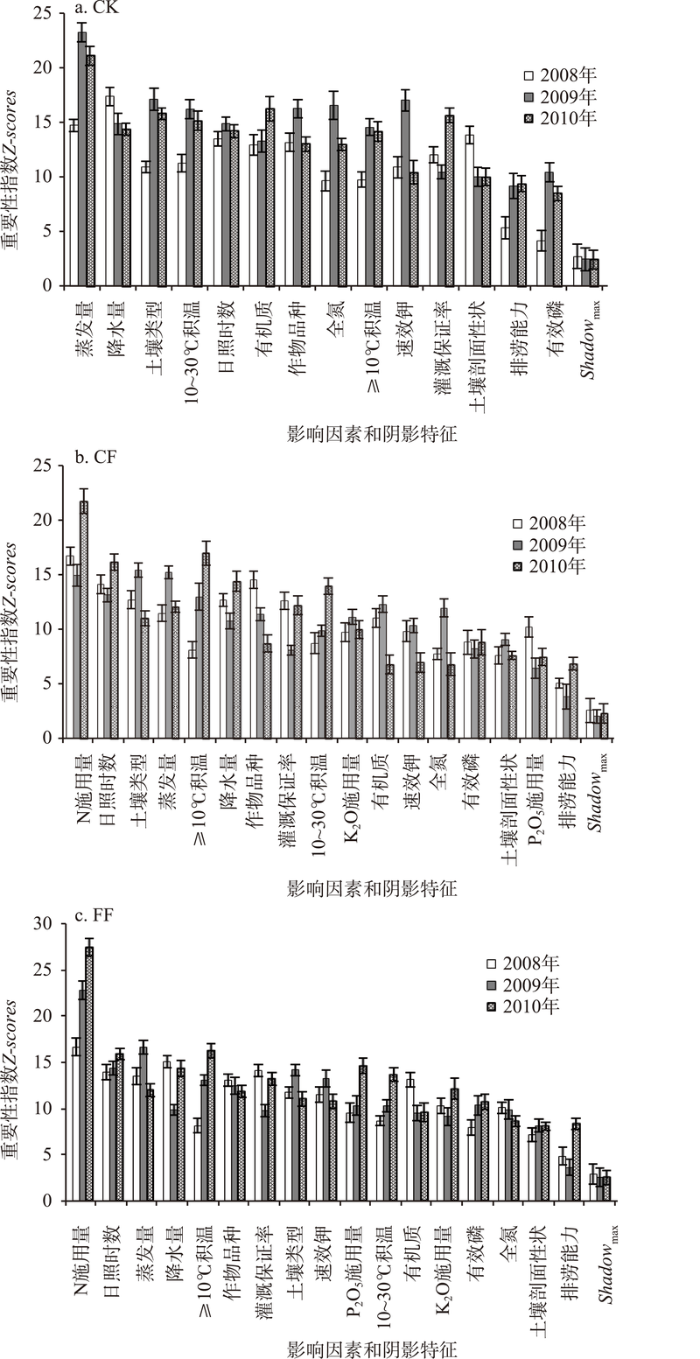
图2影响因素和阴影特征的重要性(误差线为±标准差)
Figure 2Z-score values of influencing factors and shadowmax (error bar represents ±1 standard deviation)
3.3 不同因素组别对夏玉米产量空间变异的解释 程度
如图3所示,2008—2010年研究区三区示范田间试验实施期间,CK、CF和FF任一处理下,土壤、气候、品种和灌排对样点夏玉米产量及空间分异的解释程度均依次减小;施肥因素对CF和FF处理下样点夏玉米产量及空间分异的解释程度最高,3年平均水平分别达到27.41%和29.42%,且施肥因素对FF处理样点夏玉米产量及空间分异的解释程度呈现出逐年增大的趋势。与CK处理相比,施肥处理条件下其他组别的因素对夏玉米产量及空间分异的解释程度均有下降,其中以品种、气候和土壤因素下降最为明显,表明在研究区内施肥是夏玉米产量最为重要的影响因素,其他因素的作用受施肥措施的影响。图3
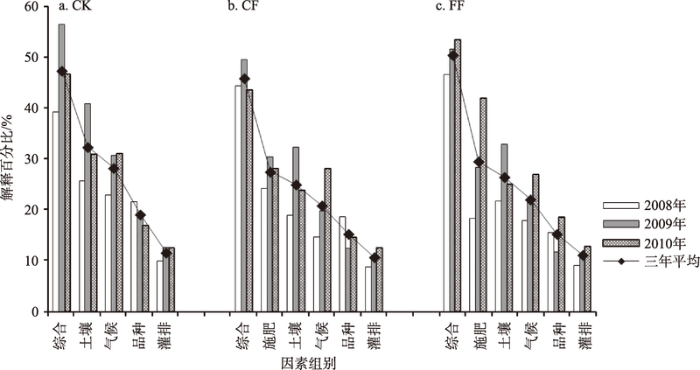
图3不同因素组对夏玉米产量空间分异的解释百分比
Figure 3Percent of spatial variation of summer maize yield explained by factor categories
土壤、气候、品种和灌排等4组因素对CK处理样点2008年、2009年、2010年夏玉米产量空间分异的综合解释比分别为39.05%、56.11%、46.45%,3年平均综合解释比为47.20%;施肥、土壤、气候、品种和灌排等5组因素对CF和FF处理样点2008年、2009年、2010年夏玉米产量空间分异的综合解释比分别为44.21%、49.34%、43.28%和46.30%、51.39%、53.15%,3年平均综合解释比分别为45.61%、50.28%。可以看出,各种影响因素对FF处理样点夏玉米产量空间分异的综合解释程度最高;虽然增加了施肥因素,但各种因素对CF处理样点夏玉米产量空间分异的综合解释程度低于CK处理样点。
3.4 夏玉米产量空间预测
基于Boruta特征变量甄选结果,研究区CK处理试验样点选择蒸发量、降水量、土壤类型、10~30℃积温、日照时数、有机质、全氮、≥10℃积温、速效钾、灌溉保证率、土壤剖面性状、排涝能力和有效磷等13个因素为特征变量,CF和FF处理试验样点在上述13个因素中另加N、P2O5、K2O施用量共16个因素为特征变量,分别构建CK、CF和FF处理下夏玉米产量与重要特征变量的RF模型,实施研究区夏玉米产量空间预测。输出结果显示,2008—2010年不同施肥处理下夏玉米产量实测值与预测值呈极显著相关,相关系数0.60~0.75,均方根误差952~1145 kg/hm2,基于验证样点的预测精度列入表3。根据空间预测结果可以发现(图4),首先,CK、CF和FF三种施肥处理下研究区3年平均单产分别为4035~8491 kg/hm2、5953~9808 kg/hm2和6302~10385 kg/hm2,虽然不同处理方式下夏玉米3年平均单产差距明显,但空间分布格局基本一致:高产区主要分布于濮阳、安阳、鹤壁、新乡、开封等河南省境内的黄淮海平原北部地区,中产区主要分布在商丘、周口、漯河、郑州、许昌等黄淮海平原南部地区以及南阳盆地,低产区则分布在豫西、豫南山区以及南阳盆地周边的丘陵山地。另外,相同施肥处理下夏玉米产量存在明显年际变化,其产量空间分布格局也存在局部差异:如FF处理下,2008年、2009年、2010年研究区夏玉米单产分别为5849~11102 kg/hm2、5570~11047 kg/hm2、5449~11219 kg/hm2;产量空间分布方面,2008年低产区集中分布在郑州、许昌、平顶山3市和洛阳交界的山地丘陵区,2009年低产区则零散分布于平顶山和许昌交界处、洛阳北部和西部、南阳盆地东侧等山地丘陵区,2010年低产区扩展至驻马店南部,而商丘东部则由原来的中低产区变为高产区。本文田间试验开展期间(2008—2010年)与2015—2017年期间的河南省各市夏玉米平均产量散点图表明(图5),虽然各市夏玉米产量水平在两个时期有所变化,但是全省夏玉米产量空间分布格局并未发生根本性改变。
Table 3
表3
表3夏玉米产量预测精度
Table 3
2008年 | 2009年 | 2010年 | |||||||||
---|---|---|---|---|---|---|---|---|---|---|---|
CK | CF | FF | CK | CF | FF | CK | CF | FF | |||
相关系数r | 0.60*** | 0.64*** | 0.66*** | 0.75*** | 0.69*** | 0.71*** | 0.67*** | 0.65*** | 0.73*** | ||
均方根误差RMSE/(kg/hm2) | 1145 | 1045 | 1060 | 965 | 997 | 1011 | 952 | 1018 | 982 |
新窗口打开|下载CSV
图4
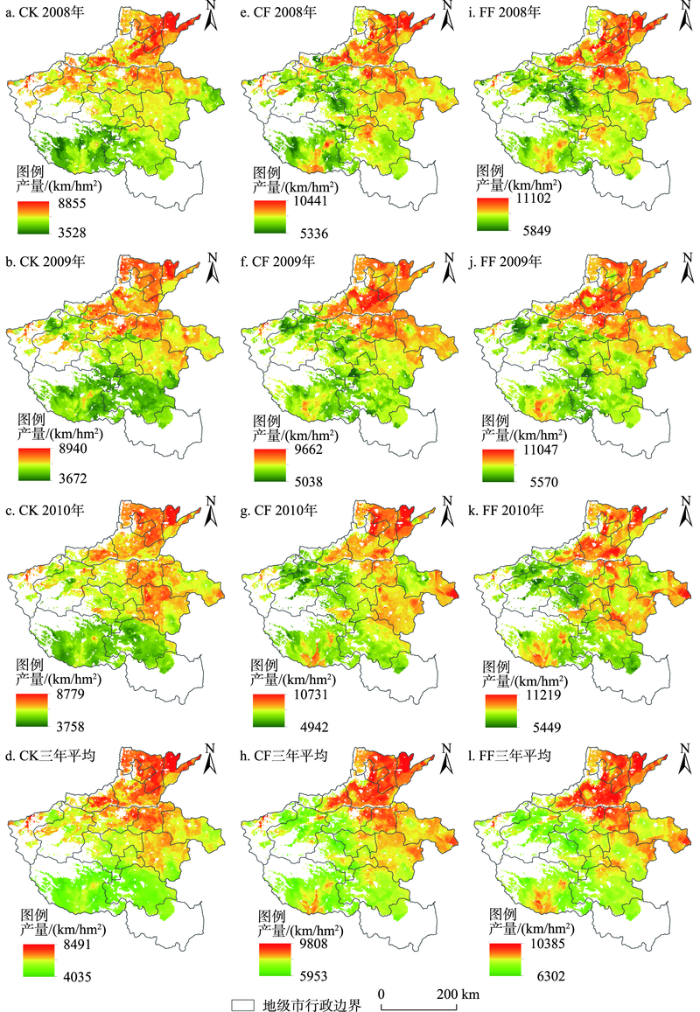
图4研究区夏玉米预测产量空间分布图
Figure 4Spatial distribution of predicted summer maize yields in Henan Province
图5
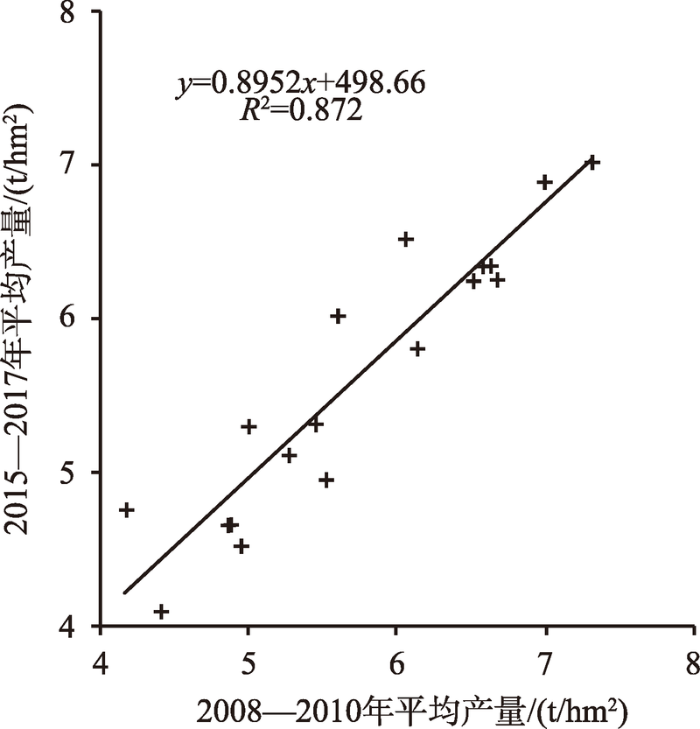
图5河南省各市2008—2010年和2015—2017年夏玉米3年平均产量散点图
注:依据2009—2011年、2016—2018年《河南统计年鉴》[42,43]中各市夏玉米单位面积产量数据整理。
Figure 5Scatter graph of average summer maize yields over three years of 2008-2010 and 2015-2017 of different cities in Henan Province
以同一空间位置多年作物产量的标准差表征该位置产量的年际波动情况,统计结果显示(图6),2008—2010年河南省夏玉米产量表现最稳定的区域主要是位于豫东商丘、周口、漯河、许昌等地的中产区,南阳盆地周边低产区和豫北高产区内的零星区域夏玉米产量也较稳定。
图6

图62008—2010年3年夏玉米产量标准差空间分布图
Figure 6Spatial distribution of standard deviation of summer maize yields, 2008-2010
4 讨论与结论
4.1 讨论
本文研究得出,施肥处理可以显著提高田间试验样点夏玉米产量,并有效降低产量的空间变异性,这得到了在研究区实施的其他相关研究结果的支持[44,45]。另外,相关研究表明[46,47],在研究区当前光温水平、土壤养分状况等条件下,在确保作物产量不降低甚至有所提升的前提下,通过FF处理优化施肥结构、提高肥料利用率从而减少化肥施用量具有现实可行性。2015年中国提出“力争到2020年主要农作物化肥使用量实现零增长”的宏伟目标已经提前3年实现,测土配方施肥技术发挥了巨大作用。氮素是夏玉米生长所需的重要元素[48],直接影响夏玉米生长发育并决定籽粒产量,是夏玉米最终产量的重要限制因子[49,50,51]。本文分析结果表明,N施用量对夏玉米产量重要性最大,在研究区开展的其他相关研究也得出了相同或相似的结论[52,53]。河南省降水量年际波动较大,空间分布不均,总体呈纬向分布,自南向北逐渐减少,北部夏玉米高产区降水日数相对较少,西部、北部均为夏玉米生产期内干旱灾害的高发区[54,55],在这样的农业气候资源条件下,通过灌溉有效补充土壤水分、保障作物生长期需水、缓解和消除夏玉米生理干旱对产量形成的影响,是保证研究区夏玉米高产稳产的关键措施。因此,相对于农田排涝能力,灌溉保证率对研究区夏玉米产量的重要性更大。
灌排因素在本文涉及的5个因素组别中对夏玉米产量空间变异的解释比最小,但这并不意味着田间水分管理对夏玉米产量不重要,与之相反,灌排管理可以有效改善农田土壤水分状况、提高肥料利用率、保证土壤通气性与适耕性,且相对于气候、土壤、品种等因素,灌排水平的改善与提高相对容易。灌排因素对夏玉米产量空间变异解释比低,是因为研究区内超过总面积60%的耕地灌溉保证、超过总面积90%的耕地排涝水平无明显差异[42,43]。从较大时间尺度上看,作物产量提高首先得益于作物品种的不断改良与新品种的不断推广[56,57],其重要性超过土壤、气候等自然因素以及施肥、灌排等管理因素。而本文分析结果却显示,与施肥、土壤、气候和灌排4个因素组别相比,品种对于研究区夏玉米产量及空间分异的重要性只高于灌排水平,这显然与相关研究的结论不同。相关研究的时间尺度较大,旨在揭示过去几十年甚至更长周期内品种的推陈出新对玉米产量的重要影响;而本文则是在较小的时间尺度上、从空间视角出发,探讨包括品种在内的多种因素对夏玉米产量空间分异的影响,在这里,品种的差异主要体现为不同地区的品种本地化特征方面,同一试验样点3个连续不同年份的品种变化几乎不存在。
本文CK、CF、FF三种施肥处理条件下的夏玉米产量空间分布格局基本一致:高产区域主要分布在豫北黄淮海平原区,中产区主要分布在豫东平原地区和南阳盆地,低产区主要分布在豫西、豫南和南阳盆地外围的丘陵山地,这一结论与相关研究结果相似[58,59]。作物产量及其空间分异受多种因素综合影响[25],主要包括土壤类型、土体构型和有效养分含量等土壤因素[60,61,62],气温、降水、日照时数和有效积温等气候因素[63,64,65],抗病、抗逆等作物品种特性[66,67],灌溉排涝条件等田间基础设施因素[68,69,70],以及施肥结构和施用量等施肥管理因素[71,72,73,74,75]。毋庸置疑,施肥能够在很大程度上提高夏玉米产量,但在省域空间尺度上,夏玉米产量的空间分布格局仍然深受气候要素、土壤类型、立地条件、管理水平等区域性因素的综合制约[45],施肥措施并不能改变作物产量空间分布格局。
4.2 结论
基于河南省2008—2010年三区示范田间试验数据、省域耕地地力评价数据和夏玉米生长季逐日气象资料,采用Boruta算法和RF模型定量评价施肥、土壤、气候、品种和灌排等5组共计17个因素对河南省夏玉米产量及其空间分异的影响,主要结论如下:(1)作物品种、土壤类型、土壤剖面性状、有机质、全氮、有效磷、速效钾、日照时数、降水量、蒸发量、≥10℃积温、10~30℃积温、灌溉保证率、排涝能力和N、P2O5、K2O施用量等17个因素对河南省夏玉米产量空间分异均具有重要影响。
(2)河南省夏玉米产量高产区主要分布在豫北黄淮海平原区,中产区主要分布在豫东平原区和南阳盆地,低产区分布在豫西、豫南和南阳盆地外围的丘陵山地。产量年际波动较小的稳产区主要集中分布于豫东黄淮海平原中产区,零散分布于南阳盆地周边低产区以及豫北高产区。
(3)作物产量空间分布格局主要受气候、土壤和立地条件等区域性因素的综合制约,施肥(尤其是配方施肥)虽然可以有效提高作物产量,但是并不能改变作物产量的空间分布格局。在国家大力推进化肥减量提效的背景下,立足现有农业气候资源条件,有效保护高产稳产的优质耕地资源、通过消除土壤障碍因素和改善耕地立地条件提高耕地基础地力是河南省贯彻落实“藏粮于地”战略,实现粮食可持续高产稳产的重要途径。
参考文献 原文顺序
文献年度倒序
文中引用次数倒序
被引期刊影响因子
[J].
[本文引用: 2]
[J].
[本文引用: 2]
[J].
[本文引用: 1]
[J].
[本文引用: 1]
[J].
[本文引用: 1]
[J].
[本文引用: 2]
[J].
[本文引用: 2]
[J].
[本文引用: 1]
[J].
DOI:10.1016/S2095-3119(14)60810-8Magsci [本文引用: 1]

With consecutive occurrences of drought disasters in China in recent years, it is important to estimate their potential impacts on regional crop production. In this study, we detect the impacts of drought on wheat and maize yield and their changes at a 0.5 degrees x0.5 degrees grid scale in the wheat-maize rotation planting area in the North China Plain under the A1B climate change scenario using the Decision Support System for Agrotechnology Transfer (DSSAT) model and the outputs of the regional climate modeling system - Providing Regional Climates for Impacts Studies (PRECIS). Self-calibrating palmer drought severity index was used as drought recognition indicator. Two time slices used for the study were the baseline (1961-1990) and 40 years of 2011-2050. The results indicate that the potential planting region for double crop system of wheat-maize would expend northward. The statistic conclusions of crop simulations varied considerably between wheat and maize. In disaster-affected seasons, wheat yield would increase in the future compared with baseline yields, whereas in opposite for maize yield. Potential crop yield reductions caused by drought would be lower for wheat and higher for maize, with a similar trend found for the ratio of potential crop yield reductions for both crops. It appears that the negative impact of drought on maize was larger than that on wheat under climate change A1B scenario.
[J].
[本文引用: 1]
[J].
[本文引用: 1]
[J].
[本文引用: 1]
[J].
[本文引用: 1]
[J].
[本文引用: 1]
[J].
[本文引用: 1]
[J].
[本文引用: 1]
[J].
[本文引用: 1]
[J].
[本文引用: 1]
[J].
[本文引用: 2]
[J].
[本文引用: 2]
[J].
[本文引用: 2]
[J].
[本文引用: 1]
[J].
[本文引用: 1]
[J].
[本文引用: 1]
[J].
[本文引用: 1]
[J].
[本文引用: 2]
[J].
[本文引用: 2]
[J].
DOI:10.2480/agrmet.D-13-00023Magsci [本文引用: 2]

The consequences of changes observed in climate and management to yield trends in major crop-producing regions have implications for future food availability. We present an assessment of the impacts of historical changes in sowing date and climate to the maize yield trend in the U.S. Corn Belt from 1980 to 2006 by using large-area crop modeling and a data assimilation technique (i.e., the model optimization based on the Markov chain Monte Carlo method). Calibrated at a regional scale, the model captured the major characteristics of the changes reported in yield as well as the timing and length of maize growth periods across the Corn Belt. The simulation results using the calibrated model indicate that while the climate change observed for the period likely contributed to a decreasing yield trend, the positive contribution from the reported shift to an earlier sowing date offset the negative impacts. With the given spread in the assessment results across previous studies and in this study, the conclusion that the negative impacts of climate change on U.S. maize yield trend more likely derive from a decreasing trend in growing-season precipitation than to an increasing trend in temperature.
[J].
DOI:10.1556/CRC.2014.0009Magsci [本文引用: 1]

Different long-term experiments were carried on chernozem (Debrecen) and loamy (Hajduboszormeny) soils. They included the most important agrotechnical, biological (hybrid) and agroecological (crop year, soil) factors in maize production. This paper evaluated the results of polyfactorial long-term experiments. For the maize the most favourable crop rotation was winter wheat (in a tri-culture) with an N 60-120, P2O5 60-70, K2O and 90-110 kg ha(-1), and a density of 75-90,000 plants ha(-1). The different input levels of maize crop management systems can modify an adaptive capacity to ecological conditions and the resilience of agro-ecosystems. The optimalization of agrotechnical elements reduces the harmful climatic effects. The yields of maize varied between 2-11 t ha(-1) in extensive and 10-15 t ha(-1) in intensive crop management systems, respectively.
[J].
[本文引用: 1]
[J].
[本文引用: 1]
[J].
[本文引用: 2]
[J].
[本文引用: 2]
[J].
[本文引用: 3]
[J].
[本文引用: 2]
[J].
[本文引用: 1]
[J].
[本文引用: 1]
[J].
DOI:10.1007/s00484-011-0460-3Magsci [本文引用: 1]

Clarification of influencing factors (cultivar planted, cultivation management, climatic conditions) affecting yields of summer maize (Zea mays L.) would provide valuable information for increasing yields further under variable climatic conditions. Here, we report actual maize yields in the Huanghuaihai region over the past 50 years (1957-2007), simulated yields of major varieties in different years (Baimaya in the 1950s, Zhengdan-2 in the 1970s, Yedan-13 in the 1990s, and Zhengdan-958 in the 2000s), and factors that influence yield. The results show that, although each variety change has played a critical role in increasing maize yields, the contribution of variety to yield increase has decreased steadily over the past 50 years (42.6%-44.3% from the 1950s to the 1970s, 34.4%-47.2% from the 1970s to the 1990s, and 21.0%-37.6% from the 1990s to the 2000s). The impact of climatic conditions on maize yield has exhibited an increasing trend (0.67%-22.5% from the 1950s to the 1970s, 2.6%-27.0% from the 1970s to the 1990s, and 9.1%-51.1% from the 1990s to the 2000s); however, interannual differences can be large, especially if there were large changes in temperature and rainfall. Among climatic factors, rainfall had a greater positive influence than light and temperature on yield increase. Cultivation measures could change the contribution rates of variety and climatic conditions. Overall, unless there is a major breakthrough in variety, improving cultivation measures will remain important for increasing future summer maize yields in the Huanghuaihai region.
[EB/OL]. (
URL [本文引用: 1]
[本文引用: 1]
[本文引用: 1]
[J].
[本文引用: 1]
[J].
[本文引用: 1]
[J].
[本文引用: 1]
[J].
[本文引用: 1]
[J].
DOI:10.1023/A:1010933404324URLMagsci [本文引用: 2]

<a name="Abs1"></a>Random forests are a combination of tree predictors such that each tree depends on the values of a random vector sampled independently and with the same distribution for all trees in the forest. The generalization error for forests converges a.s. to a limit as the number of trees in the forest becomes large. The generalization error of a forest of tree classifiers depends on the strength of the individual trees in the forest and the correlation between them. Using a random selection of features to split each node yields error rates that compare favorably to Adaboost (Y. Freund & R. Schapire, <i>Machine Learning</i>: <i>Proceedings of the Thirteenth International conference</i>, ***, 148–156), but are more robust with respect to noise. Internal estimates monitor error, strength, and correlation and these are used to show the response to increasing the number of features used in the splitting. Internal estimates are also used to measure variable importance. These ideas are also applicable to regression.
[J].
[本文引用: 1]
[J].
[本文引用: 1]
[本文引用: 1]
[J].
[本文引用: 1]
[D].
[本文引用: 1]
[D].
[本文引用: 1]
[J].
[本文引用: 1]
[J].
[本文引用: 1]
[J].
[J].
[本文引用: 2]
[本文引用: 2]
[本文引用: 2]
[本文引用: 2]
[J].
[本文引用: 1]
[J].
[本文引用: 1]
[J].
[本文引用: 2]
[J].
[本文引用: 2]
[J].
[本文引用: 1]
[J].
[本文引用: 1]
[J].
[本文引用: 1]
[J].
[本文引用: 1]
[J].
DOI:10.1023/A:1021197525875Magsci [本文引用: 1]

<a name="Abs1"></a>Are present nutrient management recommendations for the world's major cereal cropping systems adequate to sustain the productivity gains required to meet food demand while also assuring acceptable standards of environmental quality? To address this question, the current nutrient management approaches and their scientific basis in large-scale, mechanized maize (<i>Zea mays</i> L.)-based cropping systems of the USA and more labor-intensive, small-scale irrigated rice (<i>Oryza sativa</i> L.) production systems in Asia were evaluated. The principal challenges in both systems are similar: (1) there is no compelling evidence for significant increases in the genetic yield potential in both systems during the past 30 years, (2) farm yields are presently about 40–65% of the attainable yield potential, and (3) nutrient management mostly relies on approaches that do not account for the dynamic nature of crop response to the environment. Because average farm yield levels of 70–80% of the attainable yield potential are necessary to meet expected food demand in the next 30 years, research must seek to develop nutrient management approaches that optimize profit, preserve soil quality, and protect natural resources in systems that consistently produce at these high yield levels. Achieving these goals will require novel strategies for more precise plant nutrient management tailored to the technologies, dynamics and spatial scales relevant to each system. Significant advances in soil chemistry, crop physiology, plant nutrition, molecular biology, and information technology must be combined in this effort. Future field-oriented plant nutrition research must be of a more strategic, interdisciplinary, and quantitative nature. Systems approaches at micro- to meso-scales are required for gaining a more quantitative understanding of crop response to nutrients based on interactions among the essential crop nutrient requirements and on response to dynamic environmental conditions.
[J].
[本文引用: 1]
[J].
[本文引用: 1]
[J].
[本文引用: 1]
[J].
[本文引用: 1]
[J].
[本文引用: 1]
[J].
[本文引用: 1]
[J].
[本文引用: 1]
[J].
[本文引用: 1]
[J].
[本文引用: 1]
[J].
[本文引用: 1]
[J].
[本文引用: 1]
[J].
[本文引用: 1]
[J].
[本文引用: 1]
[J].
[本文引用: 1]
[J].
[本文引用: 1]
[J].
[本文引用: 1]
[J].
[本文引用: 1]
[J].
[本文引用: 1]
[J].
[本文引用: 1]
[J].
[本文引用: 1]
[J].
[本文引用: 1]
[J].
[本文引用: 1]
[J].
[本文引用: 1]
[J].
[本文引用: 1]
[J].
[本文引用: 1]
[J].
[本文引用: 1]
[J].
[本文引用: 1]
[J].
[本文引用: 1]
[J].
[本文引用: 1]
[J].
[本文引用: 1]
[J].
[本文引用: 1]
[J].
Magsci [本文引用: 1]

South-central Nebraska is one of the most extensively irrigated areas in the U. S., with over 65,000 active irrigation wells, and maize is the major agronomical crop produced. Maize production in this region requires supplementary irrigation for maximum productivity. Effective on-farm implementation of full and limited irrigation practices for potential improvements of crop productivity requires knowledge of locally developed crop yield response to water functions. In this study, the effects of full and limited irrigation practices on maize (Zea mays L.) plant height, leaf area index (LAI), grain yield and biomass production, actual crop evapotranspiration (ETa), yield production functions, yield response factors (K-y), and harvest index (HI) were investigated. Field experiments were conducted in 2009 and 2010 under center-pivot irrigation at the University of Nebraska-Lincoln, South Central Agricultural Laboratory near Clay Center, Nebraska. Four irrigation regimes [fully irrigated treatment (FIT), 75% FIT, 60% FIT, and 50% FIT] and a rainfed treatment were evaluated each year. Maize ETa, LAI, biomass production, grain yield, and HI were significantly affected by the irrigation regimes. Maize yields varied from 9.05 Mg ha(-1) for the rainfed treatment to 15.5 Mg ha(-1) for FIT in 2009 and from 11.7 to 15.5 Mg ha(-1) for the respective treatments in 2010. HI ranged between 0.49 for rainfed and 0.57 for FIT with an all-treatment average of 0.54. ETa ranged from 481 mm for rainfed treatment to 620 mm for FIT in 2009 and from 579 to 634 mm for the same treatments in 2010. Strong yield vs. irrigation relationships (R-2 >= 0.98 in both years) and yield vs. ETa relationships (R-2 = 0.94 in 2009 and R-2 = 0.97 in 2010) were measured. There was a strong linear increase in ETa with increasing irrigation amounts (R-2 = 0.97). The yield-irrigation and yield-ETa relationships showed variation between the two years due to the impact of weather variability on these relationships, indicating the importance of accounting for weather variability impact on the slopes of crop yield production functions. Based on the slopes of the ETa vs. grain yield relationships, 1.2 Mg ha(-1) (in 2009) and 1.7 Mg ha(-1) (in 2010) of grain yield was produced per 25.4 mm of ETa beyond 280 mm (in 2009) and 403 mm (in 2010) of ETa that was used by maize to start producing grain yield, which is also called the amount of ETa required for establishing grain yield. Yield response factors varied between treatments and with year for the same treatment and averaged 1.65 in 2009 and 2.85 in 2010, with a two-year average of 1.82. No statistically significant difference (p > 0.05) in grain yield was found between 75% FIT and 100% FIT. In terms of crop response to water performance, the 75% FIT and 60% FIT treatments were very comparable to the fully irrigated treatment and are viable practices in increasing crop water productivity of maize with supplementary irrigation under these experimental, soil and crop management, and climatic conditions.
[J].
[本文引用: 1]
[J].
[本文引用: 1]
[J].
[本文引用: 1]
[J].
[本文引用: 1]
[J].
[本文引用: 1]
[J].
[本文引用: 1]
[J].
[本文引用: 1]
[J].
[本文引用: 1]
[J].
[本文引用: 1]
[J].
[本文引用: 1]
[J].
[本文引用: 1]
[J].
[本文引用: 1]
[J].
[本文引用: 1]