HTML
--> --> -->Satellite-based hyperspectral infrared (IR) sounders (Menzel et al., 2018) onboard low earth orbiting (LEO) satellites, such as the Atmospheric Infrared Sounder (AIRS), Infrared Atmospheric Sounding Interferometer (IASI), Cross-track Infrared Sounder (CrIS), and the Hyperspectral Infra-Red Atmospheric Sounder (HIRAS), provide high spectral (or hyperspectral) IR radiance observations. These observations allow for a very high vertical resolution of the atmospheric state; assimilating them can reduce forecast errors in both global and regional NWP models (Pavelin et al., 2008; Hilton et al., 2009; Pangaud et al., 2009; Wang et al., 2014, 2017; Zheng et al., 2015). However, each sounder only provides observations twice a day over most of the globe, and frequent observations are needed to reflect the atmospheric changes for NWP and to increase the chances of obtaining cloud-free observations.
The spatial resolution of advanced imagers onboard GEO satellites, such as the Advanced Baseline Imager (ABI) (Schmit et al., 2005) onboard GOES-16/17, the Advanced Himawari Imager (AHI) (Bessho et al., 2016) onboard Himawari-8/9, and the Advanced Geosynchronous Radiation Imager (AGRI) onboard Fengyun-4A (Yang et al., 2017), have very high temporal (1–15 min) and high spatial (0.5 to 4 km at nadir) resolutions. The observations from these imagers can monitor rapidly changing weather systems, especially local severe storms (LSS), which have relatively short lifetimes but can cause severe damage with large hail, heavy precipitation, and strong winds. The development and movement of cloud and moisture fields within weather systems can be better understood through the use of advanced imager data (Ma et al., 2017; Kazumori, 2018; Lee et al., 2018; Schmit et al. 2019; Wang et al., 2019). However, there are only a few absorption bands for NWP applications and those bands that are included are spectrally wide, containing limited vertical information.
Hyperspectral sounders similar to those currently onboard LEO satellites are also needed in GEO orbits. Schmit et al. (2009) discussed that advanced sounding missions from GEO orbits can provide the high temporal and high spatial resolution 4-D moisture and dynamic motion information needed for the improvement of nowcasting weather predictions. The WMO 2040 Vision recommended at least six geostationary satellites with advanced imagers and hyperspectral IR sounders for weather forecasting (Balogh and Kurino, 2020). EUMETSAT plans to launch its first geostationary advanced IR sounder called the InfraRed Sounder (IRS) in the 2023 time frame. Okamoto et al. (2020) simulated a hyperspectral infrared sounder on a Himawari follow-on geostationary satellite and assessed the impacts in both regional and global NWP models, and they found added impacts from the GEO hyperspectral IR sounder on both large scale and mesoscale weather forecasts. The Geostationary Interferometric Infrared Sounder (GIIRS) onboard the Fengyun-4A (FY-4A) geostationary satellite was launched in 2016 and became operational in 2018 (Yin et al., 2020). Li et al. (2018) studied the added value from a GEO hyperspectral infrared sounder through a quick regional Observing System Simulation Experiment (OSSE). The quick OSSEs have been used by investigators and are considered to be a well-established type of impact experiment for understanding the value-added impact of future observations (Jones et al., 2017).
An OSSE is designed to use data assimilation to investigate the potential impact of future observing systems (Atlas, 1997; Atlas et al., 2015; Hoffman and Atlas, 2016). An OSSE starts with a nature run (NR) generated by a very high temporal and spatial resolution model output, which serves as the true state of the atmosphere, and comes from a free run NWP forecast. The NR is intended to be the best representation possible of the true environmental conditions; the temporal and spatial resolutions should be sufficient so that the data may be used as experimental samples and validation data for planning future observing systems. Ideally, the NR should be of finer resolution than the instrument being proposed. In an OSSE, synthetic observations are simulated from the NR with a state-of-art forward operator and then assimilated into an NWP model. The analysis and forecast can be assessed through comparison with the NR to quantify the impact of the sensor or the assimilation technique. There are four main challenges in an OSSE: (1) the simulated observations are difficult to characterize with realistic observation errors included, (2) the high impact weather events such as local severe storms and tropical cyclones (TCs) are difficult to be simulated realistically in a NR, (3) the forecast model used to evaluate the impacts should be neither too far apart nor too close to the NR, if it is too far apart, the impact is difficult to justify, while if it is too close, the impact might be meaningless, and (4) an OSSE is very difficult to calibrate. Here, calibration means the OSSE system needs to be adjusted so that when it is applied to the real observations the impacts from the real and the simulated data, respectively, are close and comparable.
To further understand and evaluate the impacts of hyperspectral sounders onboard GEO satellites over the continental US (CONUS), a hybrid Observing System Simulation Experiment (OSSE) method is used. Compared to the traditional OSSE, in a hybrid OSSE, most of the data are real observations, but new sensors are simulated from high temporal and spatial resolution global or regional re-analysis and forward models (Okamoto et al., 2020). Different from the traditional OSSE, which uses a free run NWP forecast as the NR, the hybrid OSSE in this study uses the the ECMWF Reanalysis v5 (ERA5) reanalysis to take the place of the NR in the traditional OSSE. In a hybrid OSSE, all observations are real except those from a future observation system, which are instead simulated (with realistic noise added) from high temporal and spatial resolution reanalysis using radiative transfer models. This approach allows for the evaluation of the future sensors using what we already have from real observations and simulating what we plan to have, such as a hyperspectral GEO IR sounder with high temporal resolution. A hybrid OSSE system is comprised of future system simulation and validation, hybrid OSSE verification, and impact assessment (Okamoto et al., 2020).
There are two distinct differences of the hybrid OSSE when compared with traditional OSSE that allow future observations to be evaluated in a more realistic environment. First, only future observations are simulated from ERA5 while the existing observations are real. For the traditional OSSE, all observations are simulated from the NR, which requires a careful calibration (verification) of the system to ensure that future observations have comparable impacts on the forecast that are as realistic as possible. This calibration is extremely computational expensive since there are many types of observations to be considered. The hybrid OSSE, on the other hand, has only future observations to be evaluated and verified. The verification can be conducted by comparing the impacts from real and simulated observations from a LEO hyperspectral IR sounder (e.g., CrIS). In this study, the CrIS full spectral resolution (FSR) is assumed to be onboard the GEO for impact studies, and the GEO CrIS radiances are simulated from ERA5 with CRTM. The simulated GEO CrIS radiances are verified through a two-step process. First, the validation against Suomi-NPP CrIS measurements ensures that the GEO CrIS radiances are properly simulated in terms of radiometric accuracy. To help ensure the GEO CrIS radiances will result in having the expected impacts on the forecast, Suomi-NPP CrIS radiances are also simulated using CRTM and assimilated, the impact is then verified against that which is observed from assimilating the real Suomi-NPP radiance measurements. The impacts from GEO CrIS-FSR radiances are then evaluated on LSS forecasts in a regional NWP model. The second distinctive difference is that the hybrid OSSE allows for the evaluation of the forecast using real observations, including the ERA5 reanalysis and additional observations. Since every component of the hybrid OSSE is real except for future observations, the evaluation of the forecasts will be relatively realistic. Especially regarding the use of additional observations such as stage IV precipitation to evaluate the precipitation forecast and provide for an independent means to quantify the added value, which is realistic and meaningful. It should be noted that in a hybrid OSSE, the reanalysis taking the place of the NR should be better than the forecast model as pointed out by Okamoto et al. (2020), which is usually true for most situations if ERA5 data are used for simulation, validation, and impact verification. In the next section, we describe the simulation work. The assimilation experiments are discussed in detail in Section 3. Section 4 explains the validation of the experiments. The added-value impact studies from GEO CrIS-FSR data are presented in Section 5. The discussion and summary are given in Sections 6 and 7, respectively.
2.1. Synthetic observation simulation
To simulate hyperspectral GEO IR sounders, ERA5 global dataset is used (Hersbach and Dee, 2017) to take the place of the NR for GEO IR sounder radiance simulation. The hourly outputs of the ERA5 have a 31 km horizontal resolution. It is resampled to 14 km at nadir for the GEO grid points. The channels of the simulated GEO IR are based on the CrIS-FSR resolution (GEO CrIS-FSR for short). There are a total of 2211 channels of CrIS-FSR in three bands covering longwave (LWIR, 650?1095 cm?1), mid-wave (MWIR 1210 ?1750 cm?1), and shortwave (SWIR 2155?2550 cm?1) bands (Zhou et al., 2019). The orbit of the GEO IR follows the current operational GOES-16 at 75.2°W. The viewing geometry of the GEO satellite is included in the simulation. To avoid using the same RTM for both simulation and assimilation, the hyperspectral IR all-sky radiative transfer model (HIRTM) (Li et al., 2017) is used for simulating a GEO hyperspectral sounder, while the CRTM Version 2.1.3 (Chen et al., 2012) is used for assimilation. Since the ERA5 analysis data serve as a replacement to the NR, the GSI and WRF-ARW models are used for the experiments with GFS reanalysis as the initial and boundary conditions, to avoid the identical twin problem. Both the clear sky and the cloudy sky are simulated for GEO CrIS-FSR data. The GEO CrIS-FSR is assumed to have a nadir spatial resolution of 14 km. The navigation system (latitude, longitude, satellite zenith angle, and satellite azimuth angle of each pixel) is obtained by degrading the ABI/GOES-16, 2 km navigation data, i.e. choosing one pixel out of seven pixels in both directions to represent a field of view (FOV) of the GEO CrIS-FSR. In the radiance simulation, the hourly ERA5 field (e.g. temperature, moisture, clouds, surface data, etc.) is linearly interpolated to the GEO CrIS-FSR grids to generate the inputs for the synthetic observation simulation. The GEO CrIS-FSR is assumed to have a temporal refresh rate of one hour, so there is no need for temporal interpolation. Considering the differences between ERA5 (taking the place of the NR) and NCEP FNL (used as initial and boundary conditions for regional NWP), and the differences from the radiative transfer model between HIRTM and CTRM, no explicit errors (random and systematic) are added to the synthetic observations. This is consistent with the study by Okamoto et al. (2020), and may cause an overestimate of the impact from the synthetic observations. Figure 1 shows the synthetic brightness temperature (BT) observations of GEO CrIS-FSR for channel 96 (Fig. 1, upper left) and channel 1183 (Fig. 1, lower left). Channel 96 (709.37 cm?1) of the GEO CrIS-FSR is in the CO2 absorption band. The effects of the satellite zenith angle on BT can be found from channel 96. Channel 1183 (1503.1 cm?1) of the GEO CrIS-FSR is in the water vapor absorption band. The temperature and mixing ratio from ERA5 at (43.3°N, 92.5°W) are shown in Fig. 1 (upper right), and the simulated BT spectra are shown in Fig. 1 (lower right).2
2.2. Synthetic Observation Evaluation
There are two main purposes for validating the synthetic GEO CrIS-FSR BT observations. First, it verifies that the ERA5 can be used to take the place of the NR to provide synthetic information, which means it can simulate the important weather systems in the CONUS domain and the thermodynamic and hydrometric information are reasonably close to the real atmosphere. Second, it ensures the simulated GEO CrIS-FSR BT observations are accurate. If the simulated BTs are reasonable, it would indicate that both ERA5 and the RTM have to be reasonable.For validation, the simulated GEO CrIS-FSR data are compared with real CrIS-FSR data from Suomi-National Polar-orbiting Partnership (S-NPP) with similar satellite zenith angles. The real CrIS-FSR observations are located at 24.45°N, 78.48°W with a satellite zenith angle at 32.07°. The related simulated radiance data is located at 24.65°N, 80.38°W with a satellite zenith angle at 29.44°. Since there were no major events at that time, the geolocation and angle differences between the two locations have no major impact on the comparison. Figure 2 shows the BT spectra of the simulated radiances and the observations from CrIS-FSR data. As expected, there are slight differences between the simulated CrIS-FSR and the observations except in the shortwave region, which is likely due to the limitation of the bidirectional reflectance distribution function (BRDF) effects in HIRTM. Where the simulated GEO CrIS-FSR data and the real observations are close to each other, especially for the longwave band and the mid-wave band, it confirms that the simulated radiances are reasonably accurate, which also indicates that both the ERA5 and the RTM are reasonably accurate to generate the simulated GEO CrIS-FSR data. The synthetic GEO CrIS-FSR observations will be used in the numerical experiments to evaluate the impacts of assimilating the GEO IR data with the CRTM.
In addition, synthetic S-NPP radiances are also simulated from ERA5 using the HIRTM. The main purpose is to verify the hybrid OSSE system, i.e. the impact of synthetic S-NPP radiances should be comparable to that from the real measurements. They also offer an opportunity to evaluate the synthetic S-NPP radiances with real measurements, as an indirect way to evaluate the synthetic GEO CrIS-FSR radiances. Figure 3 shows the data coverage of the S-NPP CrIS-FSR radiances of channel 1183 from the observations (Fig. 3a) and the simulations (Fig. 3b). The CrIS-FSR channel 1183 (1503.1 cm?1) is in the water vapor absorption band. It reflects the moisture pattern of the atmospheric state. Larger BT values represent less water vapor in the upper atmosphere. From the observations, there is a dry band in the southern part of the East Coast of the United States. This dry band also can be found in the simulated CrIS-FSR data (Fig. 3b), although less profound. Both the pattern and the intensity of the BT from the simulated CrIS-FSR are similar to those of the BT from the observations. The largest value of observed BT from channel 1183 is over 250 K, which is consistent with the simulated BT. Given the agreement between the simulation and the observations, ERA5 can be used to take the place of the NR and the synthetic observations simulated from it can be used to reasonably represent future GEO CrIS-FSR observations. However, there are two differences between the observations and the simulations. While the resolution of the simulated CrIS-FSR at 14 km at the nadir is the same as the observations, the simulated data is linearly interpolated from the ERA5 data with 31 km horizontal resolution, which means it lacks the detailed information achievable from high-resolution observations. Since in the data assimilation system, only the clear sky or clear channels of the hyperspectral IR will be used, the effects of less accurate clouds would be relatively small.
3.1. Data assimilation system
The data assimilation system used in this study is the Developmental Testbed Center (DTC) delivered the Community Gridpoint Statistical Interpolation (GSI) system (DTC-GSI) v3.7. GSI was designed by NOAA/NASA for assimilating observations in the operational NWP models (Hu et al., 2018). DTC began to collaborate with major GSI development groups to transform the operational GSI system into a community system in 2007 (Shao et al., 2016). The GSI version was updated by the DTC based on the development of the operational GSI model. In 2017, the DTC and the Environmental Modeling Center (EMC) worked together to build a unified GSI code repository for both operational and community developers. The latest version, GSI v3.7, was used. The GSI can be run as data assimilation of 2DVar (for surface data analysis), 3DVar, 3D ensemble-variational (3DEnVar), 4DEnVar, and 3D/4D hybrid EnVar. Due to the limitation of the computer resources for the regional NWP model, the 3Dvar method is used to evaluate the GEO CrIS-FSR in this study.For all observations, the background and observation errors are based on the North American Mesoscale Forecast System (NAM) regional model. For satellite observations, the enhanced variational bias correction (VarBC) method is used for bias correction, which is updated every cycle from the initial satellite bias coefficient (Zhu et al., 2014). In applying the VarBC to GEO CrIS-FSR with no explicit errors, the VarBC will identify the differences, in terms of radiance space, between the climatology of ERA5 and the climatology of the forecast model, as well as between the CRTM and the HIRTM. For the GEO CrIS-FSR, the bias coefficients are initiated from the current SNPP CrIS-FSR data. With the initial BC coefficient, the simulated GEO CrIS-FSR data were cycling assimilated several times in the current domain to fit the bias coefficients to the GEO CrIS-FSR data. The updated bias coefficients for the GEO CrIS-FSR data are then used as the coefficients in the assimilation experiments for impact assessment. To assimilate the GEO CrIS-FSR data, the module that reads the CrIS-FSR data is modified. The satellite zenith angles of GEO and LEO data are different. To use the current CrIS-FSR assimilating module without introducing extra uncertainties, any GEO CrIS-FSR data with a zenith angle larger than 60° are discarded. With some modifications of the CrIS-FSR assimilation module, the GEO CrIS-FSR data are converted to BUFR format for the GSI to assimilate.
The CRTM is used as the forward model to simulate the radiances based on the background from the analysis fields in the GSI system. It is a unified interface for all sensors and conditions. It includes six hydrometeor types, including water, ice, rain, snow, hail, and graupel. The CRTM also provides the Jacobians of the input variables. The details of the CRTM can be found in Chen et al. (2010, 2012) and Han et al. (2006). The CRTM coefficients version 2.3.0 is used in GSI v 3.7.
2
3.2. WRF-ARW forecast model
The Advanced Research Weather Research and Forecasting Model (WRF-ARW) model version 3.9.1 is used as the regional NWP model. It is a community mesoscale model developed by NCAR. It includes four main parts: the WRF model, the WRF pre-processing system (WPS), WRF Data Assimilation (WRF-DA), and WRF-Chemistry. In this work, the WRF model and WPS are used for the LSS case simulation. The WRF-ARW model is widely used at operational centers and in the research community, especially for regional NWP studies. It has a variety of physics schemes available to simulate different weather systems.The general settings of this experiment mainly follow the Rapid Refresh (RAP) and the High-Resolution Rapid Refresh (HRRR) (Benjamin et al., 2016; Lin et al., 2017) forecast models. A two-way nested domain with a horizontal resolution of 9 km for the outer domain (domain 1) and 3 km for the inner domain (domain 2) is adopted. The outer domain has 600 × 350 grid points (around 22°?50°N, 65°?130°W), the inner domain has 901 × 601 grid points (around 30°?46°N, 85°?110°W). There are 51 vertical levels extending from the surface to 10 hPa. The physical schemes are consistent with the RAP/HRRR model in that the microphysics scheme is Thompson aerosol-aware, the longwave and shortwave radiation scheme is RRTMG (Iacono et al., 2008), the land surface scheme uses the Noah land surface model, and the planetary boundary layer uses the Yonesei University scheme.
2
3.3. Data and experimental design
To evaluate the added value of GEO IR data, observations assimilated in the current systems are used, including conventional data (GTS), AMSU-A onboard NOAA-15, NOAA-18, NOAA-19, Metop-A, and Metop-B, ATMS and CrIS onboard Suomi-NPP and NOAA-20, and IASI onboard Metop-A/B. In the control (CNTRL) run, all the existing observations mentioned are assimilated. In the experiment (EXP), the CrIS-FSR data from S-NPP and NOAA-20 are replaced by the synthetic GEO CrIS-FSR observations.CNTRL: GTS + AMSU-A + IASI + ATMS + real SNPP/NOAA20 CrIS-FSR
EXP: GTS + AMSU-A + IASI + ATMS + simulated GEO CrIS-FSR
VER: GTS + AMSU-A + IASI + ATMS + simulated SNPP/NOAA20 CrIS-FSR
The thinning box of the AMSU-A, ATMS, ATMS, and CrIS-FSR is 60 km. The CNTRL represents the existing capability with the current observing system, while the EXP represents the added value from the future GEO hyperspectral IR sounder in replace of the LEO CrIS-FSR. The two experiments (CNTRL and EXP) can be viewed as the different impacts between using LEO sounders and using a GEO sounder for regional NWP.
Two LSS cases are selected for impact assessment. The precipitation of the first LSS case (Case I) occurred in Colorado and Oklahoma from 0000 UTC 24 June to 1800 UTC 24 June 2018. While the storm was relatively short-lived, the 6-h accumulated precipitation was more than 100 mm. For this case, the assimilation time is at 1800 UTC 23 June, followed by a 24-h forecast to 1800 UTC 24 June 2018. The evaluation will focus on precipitation forecasts for Case I. Figure 4 shows the BT evolution of GOES-16 channel 9 from 2200 UTC 23 June to 1800 UTC 24 June 2018. The temporal changes in BT of channel 9 reflect the development of the LSS case.
The second LSS case (Case II) began in Oklahoma and moved northeastward to Wisconsin from 0000 UTC 26 May to 1200 UTC 27 May 2019. From genesis to dissipation, this storm lasted much longer than the storm in the first case. The assimilation is conducted every six hours from 0000 UTC 26 May to 1200 UTC 27 May, with each assimilation followed by a 72-h forecast (Fig. 5c). There are seven forecast groups for Case II. Figure 5 shows the 6-h accumulated precipitation from 0000 to 0600 UTC, and from 0600 to 1200 UTC on 26 May 2019. This longer-lived storm allows for a more comprehensive statistical analysis. For both cases, a final normalized score and the percentile of the CNTRL and EXP will be calculated to evaluate the impacts of the added value from GEO hyperspectral IR data.
In addition, a verification experiment (VER) is conducted where the synthetic CrIS-FSR radiances are assimilated in replace of real measurements. The purpose of VER is to ensure that assimilating the synthetic radiances has comparable impacts to those from the real observations. Any large deviations would indicate possible underestimates or overestimates of the impacts which may arise from the synthetic radiances. It is an important step to verify that the experimental results are reasonably calibrated and that the hybrid OSSE can be used to evaluate the impacts from a future satellite observing system with confidence.
2
4.1. Temperature validation
ERA5 is the fifth generation reanalysis dataset released by the ECMWF with hourly high-resolution grid points. The ERA5 data are used to take the place of the NR to represent the real atmosphere. It is widely used as the benchmark for validating satellite products and models (Hooker et al., 2018; Eicker et al., 2020; Ma et al., 2020). The atmospheric field of ERA5 is also used to validate the forecast results of the CNTRL and VER experiments in this study. As described in Section 3.3, the assimilation time is at 1800 UTC 23 June 2018 which is then followed by a 24-h forecast for Case I. Figure 6 shows the 850 hPa temperature fields of ERA5, CNTRL, and VER at 1800 UTC 24 June 2018. The temperature fields are based on the outer domain of the model (domain 1) with 9 km resolution, and then the data are linearly interpolated to the ERA5 grids. The missing data in New Mexico, Colorado, Wyoming, Utah, and Idaho are due to the high terrain in those regions. The standard deviation (STD) of the ERA5 – CNTRL is 1.69 K, and the root-mean-squared-error (RMSE) is 3.42 K. The differences between the ERA5 and VER (ERA5 – VER) are shown in Fig. 6e. The STD of the ERA5 – VER is 1.70 K, and the RMSE of the ERA5 – VER is 3.40 K. Comparing the ERA5 – CNTRL and ERA5 – VER at the 24-h forecast, the STD and RMSE are very close to each other.The RMSE and the errors of 95% confidence interval of the temperature fields of ERA5 – CNTRL and ERA5 – VER at 850 hPa are calculated at the analysis time and for the 6-h forecast, 12-h forecast, 18-h forecast, and 24-h forecast (Table 1). The smaller RMSE of the two groups of experiments is shown in red. From Table 1, it is hard to conclusively determine which experiment performs better overall. Similar results also can be found for the temperature at other levels and the other atmospheric variables, such as moisture and winds (not shown). Thus, these comparisons show that the forecast results of VER are comparable to the forecast results of CNTRL. In other words, the simulated synthetic S-NPP CrIS-FSR radiances have a similar impact on the analysis and forecast of thermodynamic fields as the real observations. These results provide confidence in using this hybrid OSSE to assess the impact of a GEO hyperspectral IR sounder.
2
4.2. Precipitation validation
For LSS cases, precipitation is one of the most important features for weather forecasting. The hybrid OSSE allows for the validation of the precipitation using real observations. The Stage IV dataset is the precipitation observation based on the multi-sensor hourly/6-hourly Stage III analysis produced by 12 River Forecast Centers (RFC) for the CONUS domain. The Stage IV data are provided as 1-h, 6-h, and 24-h accumulated precipitation at a resolution of 4 km. The data also has covered Alaska and Puerto Rico stations since 2017 (Seo et al., 2002). The Stage IV dataset has been used to depict the precipitation observations in research studies (Lopez and Bauer, 2007; Kalinga and Gan, 2010; Wang et al., 2020). The 24-h accumulated precipitation results from both the CNTRL and VER are compared with the Stage IV dataset. The rainfall belt of 24-h accumulated precipitation for Case I, an LSS case with strong precipitation, is oriented from the northwest toward the southeast in the domain area.To evaluate the precipitation forecasts, the equitable threat scores (ETS), probability of detection (POD), and false alarm ratio (FAR) are calculated for the 6-h, 12-h, 18-h, and 24-h accumulated precipitation in the domain from 32.0°N to 42.5°N, and from 90.0°W to 107.0°W. The ETS, POD, and FAR scores reflect the precipitation location and intensity forecasts compared to the Stage IV observations. ETS values range from ?1/3 to 1, with higher ETS scores reflecting better precipitation forecasts (or closer to the observations). POD and FAR scores both range from 0 to 1, with higher POD scores reflecting better precipitation detection, and smaller FAR scores indicating fewer false alarms. The ETS, POD, and FAR scores for CNTRL and VER over 0.1 mm accumulated precipitation are compared to each other. Table 2 lists the scores for CNTRL and VER for 6-h, 12-h, 18-h, and 24-h accumulated precipitation. The ETS scores for CNTRL and VER are comparable to each other. The POD for VER is slightly better (or larger) than that for CNTRL. The FAR for VER is slightly worse (or larger) than that for CNTRL. However, the differences between CNTRL and VER at 6-h, 12-h, 18-h, and 24-h forecasts are relatively small. The mean differences are 0.83% for ETS, 1.4% for POD, and 0.78% for FAR. The errors of the 95% confidence interval for ETS, POD, and FAR are listed in Table 2. The errors are very similar between CNTRL and VER. These small differences indicate that the precipitation forecasts of the two experiments are comparable to each other.
Based on the validation of the atmospheric fields using ERA5 and the precipitation using the Stage IV observations, the CNTRL and VER are similar or comparable to each other in the atmospheric fields and the accumulated precipitation forecast. It is therefore reasonable to believe that the simulated LEO CrIS-FSR can provide impacts similar to the real CrIS-FSR in the NWP model for LSS forecasts. Since simulating the GEO CrIS-FSR follows the same steps as the simulated LEO CrIS-FSR, the assimilation of the simulated GEO CrIS-FSR should be able to reflect the expected added value for LSS forecasts.
5.1. Data impacts on analysis fields
The data assimilation method uses information from the observations to improve the analysis fields which go on to further improve forecast results. The analysis fields directly reflect the data impact after assimilation, so analyzing the changes in the analysis fields is an important step to study the GEO CrIS-FSR data impact. Figure 7 provides the data coverage of CrIS-FSR channel 97 from both S-NPP and NOAA20 after assimilation in the GSI system at 0600 UTC on 27 May 2019. The thinning box of the real CrIS-FSR (Fig. 7a) is 60 km following the operational settings. While the simulated GEO data can cover the whole model domain, only satellite zenith angles less than 60° are used in the GSI system. As an example, in Fig. 7b, when the satellite zenith angle is greater than 60°, there are gaps where the data have been removed, which occurs mainly in the northwestern part of the CONUS. The missing data in the Fig. 7 also includes observations affected by clouds as the clear detection method inside the GSI system is used to remove cloud contamination. Due to the differences in the satellite zenith angle, the BTs of the real CrIS-FSR and the simulated GEO CrIS-FSR are different. More data are available from the simulated GEO CrIS-FSR than from the real CrIS-FSR, especially in Texas and the southwestern coastal area.In this study, ERA5 is used to take the place of the NR, which is assumed to be the “true” atmosphere. The analysis fields are compared with the ERA5 atmospheric state. Figure 8 shows the moisture differences between the ERA5 and CNTRL (ERA5 – CNTRL) as well as ERA5 and EXP (ERA5 – EXP) at 850 hPa for both model domains. The analysis fields directly reflect the atmospheric state after assimilating the data. In general, the main patterns of the moisture differences are similar for the two experiments. CNTRL appears to have slightly larger differences compared with ERA5, especially in Texas. This can be seen more easily from the statistics. For domain 1, the RMSE of the ERA5 – CNTRL is 2.86 g kg?1, and the STD is 2.24 g kg?1. For ERA5 – EXP, the RMSE is 2.26 g kg?1, and the STD is 2.00 g kg?1. For domain 2, the RMSE of ERA5 – CNTRL is 2.75 g kg?1, and the STD is 2.10 g kg?1; while the RMSE of ERA5 – EXP is 2.58 g kg?1, and the STD is 2.11 g kg?1. These results show that the EXP moisture field is closer to ERA5 than the moisture field from the CNTRL for both model domain 1 and domain 2 at the analysis time. Similar results also can be found for other atmospheric state variables, such as temperature and winds.
2
5.2. Data impacts on forecast fields
The assimilation affects not only the analysis fields but also the forecasts. The ERA5 reanalysis dataset is also used to evaluate the forecast results from the CNTRL and EXP. For each experiment in Case II, the RMSE and STD of the averaged 00-h, 06-h, 12-h, 18-h, and 24-h forecast time at the standard atmospheric levels are calculated. The STD of the temperature and moisture at 850 hPa and the STD of the U/V wind at 500 hPa for all seven groups of the experiment from 0000 UTC 26 May to 1200 UTC 27 May 2019 are shown in Fig. 9. At 850 hPa, the temperature STD of ERA5 – EXP is slightly smaller than that of ERA5 – CNTRL for the first six groups of the experiment. The moisture fields at 850 hPa and U/V winds at 500 hPa from EXP are always closer to the ERA5 reanalysis fields than those from CNTRL. To further evaluate the impacts from the GEO hyperspectral IR data, T/Q/U/V at the four standard atmospheric levels, i.e., 850 hPa, 700 hPa, 500 hPa, and 200 hPa are compared with ERA5 for all seven groups of experiments for Case II. The RMSE and the STD of the ERA5 – CNTRL and ERA5 – EXP are listed in Table 3. Smaller RMSEs or STDs are shown in red. As seen from the table, all RMSEs and STDs of EXP are smaller than those of CNTRL, which indicates that the temperature, moisture, and U/V wind forecast fields from EXP are closer to ERA5 than those from CNTRL for all cycling runs. Thus, assimilating GEO CrIS-FSR data provides positive impacts on the analysis fields, which will likely lead to improved forecast fields by reducing the forecast error attributed to the atmospheric state variables.2
5.3. Data impacts on precipitation
Similar results were found for the forecasts of the T/Q/U/V where EXP is closer to ERA5 (not shown). For LLS, the evaluation of the precipitation forecast is important because of its relationship to potential loss. The 24-h accumulated precipitation from CNTRL and EXP are compared with the Stage IV observations for all experiments. Figure 10 shows one example of the 6-h accumulated precipitation from the experiment time starting at 0600 UTC 27 May 2019. From the Stage IV observations (Fig. 10a), the LSS precipitation covered Minnesota, North Dakota, South Dakota, Iowa, Nebraska, and Kansas. The main patterns of precipitation from CNTRL and EXP are similar. The forecast precipitation over Kansas is less than the Stage IV precipitation, which is due to the model initial conditions (FNL data). However, a spurious storm forms in CNTRL over northeastern Texas (in the red circle). The artificial storm produces heavy precipitation and propagates further northeastward in the following forecast. The assimilation of GEO CrIS-FSR data was able to remove the spurious storm. Similarly, the development of the spurious storm in CNTRL is also removed in EXP in the following forecast.The ETS, POD, and FAR of each experiment are calculated (Fig. 11) applying a threshold of over 0.1 mm. The scores reflect the accuracy of the precipitation location and intensity forecasts. The scores are calculated using the forecasts based on domain 2 inside a box from 34°N to 45°N and from 84°W to 104°W. The accumulated precipitation starts at 0000 UTC 26 May and continues to 1200 UTC 27 May 2019. ETS scores from EXP are higher than those from CNTRL for all seven groups of experiments, especially for the forecast starting at 0600 UTC 27 May, where the largest improvement of the ETS is seen. The POD scores for the experiments are shown in Fig. 11b. Overall, the EXP POD scores are better than those of CNTRL, but they are quite close to each other, except for the forecast starting at 0600 UTC 26 May. FAR scores measure the fraction of rain detections that were false alarms. The FAR of CNTRL and EXP (Fig. 11c) shows that for most of the experiments, the FAR of EXP is substantially smaller than that of CNTRL. These results indicate that the assimilation of the GEO CrIS-FSR data improves the 24-h accumulated precipitation forecast by increasing the EST and POD scores and by reducing the FAR scores for this case study.
2
5.4. Final scores
To further assess the impact of the GEO CrIS-FSR data on LSS forecasts, an overall evaluation strategy is carried out for CNTRL and EXP. A final score is calculated based on the evaluation of the atmospheric state at the four standard atmospheric levels in the troposphere (850 hPa, 700 hPa, 500 hPa, and 200 hPa), and the three precipitation scores, ETS, POD, and FAR. The purpose is to use this one final score to characterize the overall impact on the forecast results.For atmospheric state variables (T/Q/U/V), the averaged RMSEs of ERA5 – CNTRL and ERA5 – EXP are calculated for each variable for the whole model domain at the four standard levels for all seven groups of experiments listed in Table 3. Since higher ETS and POD scores are better, the ETS and POD scores are calculated for the average of (1 – ETS) and (1 – POD) to be consistent with the RMSEs of the other variables. The ETS, POD, and FAR are calculated using the threshold of 0.1 mm precipitation.
Table 4 lists the RMSEs from temperature, moisture, U-wind, V-wind, and ETS, POD, and FAR precipitation scores. The smaller of the RMSEs between CNTRL and EXP are listed in red. The percentage change is reported in the last column. The improvement in EXP can be found in most of the variables, especially for the temperature and moisture fields. The improvement in temperature fields is around 2%, and the improvement in moisture fields is around 16%. The improvement in U/V winds is around 3%. Thus, the assimilation of GEO CrIS-FSR has large impacts on the atmospheric thermodynamic information of the forecast fields. For precipitation, there is slight improvement using FAR scores, yet there is a slight decline of POD scores, which is consistent with Fig. 11. The ETS scores show around 1.5% improvement with the assimilation of the GEO CrIS-FSR data. By assimilating these data, the error between the forecast fields and the ERA5 is reduced, which further reduces the precipitation forecast error. The improvement in precipitation is less than the improvement in the thermodynamic fields. This is to be expected since the precipitation is a non-linear process and is also related to the physical schemes (i.e. microphysics schemes, etc.).
To get an overall score that may represent the overall performance of the forecast, a normalization process is needed to ensure each parameter has a controlled weight in the final score with a confidence interval of 95%. After the normalization of each variable is calculated, the final score, or the final normalized RMSE, is calculated by averaging each normalized variable with different weights (Li et al., 2018, 2020). The thermodynamic parameters are each weighted at 10%, including T/Q/U/V. The precipitation scores are each weighted at 20% to emphasize the importance of the precipitation forecast, including ETS/POD/FAR. For Case II, the final normalized RMSE of CNTRL is 0.7258, and the final normalized RMSE of EXP is 0.6872 (Fig. 12a). The final normalized RMSE is significantly reduced by 5.3%. The same method is applied to LSS Case I (Fig. 12b); the scores of Case I are based on one forecast result. The final normalized RMSE of CNTRL is 0.7148, and that of EXP is 0.6818, so the final normalized RMSE is significantly reduced by 4.6%. Based on the results of Case I and Case II, the normalized RMSE is significantly reduced by approximately 5% with the assimilation of GEO hyperspectral IR sounder radiances. The reduction is largely attributed to the improvement in the thermodynamic atmospheric fields.
Although the synthetic radiances are evaluated using real CrIS radiances in section 2.2, the hybrid OSSE is not fully calibrated in this study. To partially solve that problem, the final normalized RMSE from VER is also calculated for LSS Case I and compared with that from EXP. It is found that the normalized RMSE is reduced by 3.0% from VER to EXP. Although this number is slightly smaller than the reduction of 4.6%, it confirms that GEO CrIS data can more significantly reduce the overall forecast error compared to the LEO CrIS data. The less profound reduction is likely due to the lack of some elements in the synthetic radiance simulation, such as spectral and spatial correlation errors, the polarization bias (Taylor et al., 2018), the earth-rotation doppler shift impact (Chen et al., 2013), and explicit errors.
These results are consistent with previous quick regional OSSE studies on GEO hyperspectral IR sounders. In Li et al. (2018), they assimilated synthetic temperature and moisture sounding retrievals from a GEO hyperspectral IR sounder, and generated a forecast error reduction of 3.56%, on top of the IR radiances from AIRS, IASI, and CrIS. While the numbers in forecast error reduction are different, due to the use of different cases, observation types (radiances versus sounding retrievals), and a different OSSE framework (hybrid versus traditional), both studies show that the GEO hyperspectral IR sounders have added-value when compared with LEO ones..
However, there are still some limitations that may underestimate or overestimate the impact of GEO hyperspectral IR sounder data on forecasts. First, the spatial resolution of the simulated GEO hyperspectral IR data is coarser than that of real data. Although the ERA5 reanalysis dataset is of high enough quality to take the place of the NR, its resolution is 31 km. Despite interpolation, the simulated radiances have an actual resolution of 31 km instead of the intended 14 km or better, or possibly as fine as 4 km for the future GEO hyperspectral IR sounder. Consequently, detailed information within the 31 km grid points is missed, which can be found in Fig. 3. It may have less impact on the global model assimilation since the thinning box for the global model is usually 145 km for LEO satellites. It would degrade the forecast results for regional models with a 9 km and 3 km model nested domain and 60 km assimilation thinning box of satellite data.
Second, the detailed information from sub-hourly data is missing. The simulated GEO hyperspectral IR data from the ERA5 are hourly, so atmospheric changes on timescales less than an hour are missing due to the NR temporal resolution. Severe weather systems usually have large amounts of precipitation, large gradients in temperature and moisture fields, and strong wind shear. Those atmospheric conditions change very fast in LSS cases. Therefore, the sub-hourly data from GEO satellites are of special value for nowcasting and short-term NWP users, which are not accounted for in this study.
Third, the 3DVar data assimilation method is not able to fully explore the value of the GEO hyperspectral IR data. One of the advantages of GEO hyperspectral IR compared to LEO hyperspectral IR is the high temporal resolution. The temporal resolution of ABI data onboard GOES-16 and AHI data onboard Himawari-8 are 1–15 minutes. Compared to the 3DVar data assimilation method, the 4D-Var method can use the tangent linear and adjoint models to produce the propagation of the analysis increment over the assimilation window (Errico et al., 1993a, b; Errico, 1997). The high temporal GEO hyperspectral IR data can be better used in 4D-Var by the adjoint models to improve the analysis increment. However, that is beyond the scope of this study due to the limited computing resources.
In addition, a subset of the spectral bands was used, for a total of 431 channels from the CrIS-FSR. While the 431 channel subset is considered representative of the original 2211 channels, some channels are given lower weights, such as the window and water vapor channels, and some channels are not used at all, such as the shortwave band. These channels remain challenging in NWP radiance assimilation. Besides the added value of the GEO hyperspectral IR radiances, a major advantage of a GEO hyperspectral instrument would be the capability of deriving three dimensional (3D) atmospheric motion vectors (AMVs) from the retrieved water vapor channels, which has proven to be useful among the existing GEO imagers (Stettner et al., 2019; Li et al., 2020).
The hybrid OSSE, just like any other OSSE studies, may overestimate the impact due to the nature of the simulation study. For example, the synthetic GEO CrIS FSR radiances have no errors due to spectral and spatial correlations, the polarization bias (Taylor et al., 2018), the earth-rotation doppler shift impact (Chen et al., 2013), and explicit errors. While the impacts from some of these could be reduced with the VarBC technique, the lack of them in the synthetic radiances may result in an overestimation of the impact upon the forecast. Also, as pointed out before, using the ERA5 reanalysis in the place of the NR, although arguably one of the best NWP model output products, may not fully represent the real atmosphere. Any information that is not simulated or not well simulated by ERA5, such as the lack of fine-scale information, the use of parameterization of microphysics, the larger uncertainties of the surface and the boundary layer, and the wetter bias in the upper troposphere (Xue et al., 2020a, b), may cause difficulty for the hybrid OSSE to precisely quantify the impact.
With the above limitations in mind, the hybrid OSSE provides a method to evaluate future observing systems in the current data assimilation system. The hybrid OSSE is complementary to traditional OSSEs with several distinctive differences, including not needing to simulate existing observations and having the capability for validation using real measurements. These differences allow institutions with limited computing resources to carry out OSSE studies with real observations.
Before assessing the impact of the synthetic GEO CrIS-FSR data, the hybrid OSSE system is verified to ensure synthetic observations have similar impacts as real measurements. In the VER, a new set of CrIS-FSR data in the same LEO orbits as the CrIS-FSR are simulated. The VER experiment has the same data and settings as CNTRL but the observed CrIS-FSR data are replaced with the synthetic LEO CrIS-FSR data. Other measurements assimilated include conventional data (GTS), AMSU-A onboard NOAA-15, NOAA-18, NOAA-19, Metop-A and Metop-B, ATMS onboard Suomi-NPP and NOAA-20, and IASI onboard Metop-A/B. The CNTRL and VER are compared with the ERA5 atmospheric state. Results show that the forecast results of VER are comparable to those of CNTRL for both the atmospheric state fields (T/Q/U/V) and the 24-h accumulated precipitation. With the agreement among the CNTRL and VER, it is reasonable to believe that the simulated LEO CrIS-FSR can provide a similar impact as the real CrIS-FSR measurements for LSS forecasts from the NWP model. The hybrid OSSE system can be used to evaluate the simulated GEO CrIS-FSR data.
Two LSS cases from 2018 and 2019 are selected for case demonstrations of the value-added impacts from the GEO CrIS-FSR data. The impact of assimilating simulated GEO CrIS-FSR data at both the analysis time and the forecast times is studied. Atmospheric thermodynamic information (T/Q/U/V) is improved from the assimilation of the GEO hyperspectral IR data over the LEO CrIS-FSR measurements. The percentage-based precipitation improvement is smaller in comparison with the improvements of the thermodynamic field due to the complexity of the LSS precipitation processes and the physical schemes. To further evaluate the overall impacts of the synthetic GEO hyperspectral IR data, a final normalized RMSE is calculated for both CNTRL and EXP by combining the atmospheric thermodynamic variables and precipitation scores with different weights. The final normalized RMSE of CNTRL is 0.7148 for Case I and 0.7258 for Case II. The final normalized RMSE of EXP is 0.6818 for Case I and 0.6872 for Case II. An overall 5% significant reduction in RMSE was found from using the GEO hyperspectral IR sounder radiances for both cases.
It should be noted that these positive impacts should be interpreted with the understanding of some limitations of the hybrid OSSE study. Some limitations may underestimate the value-added impact, such as the coarse resolution of the ERA5 in the place of the NR, the use of 3DVar instead of 4DVar data assimilation system, and the use of a subset of the spectral bands assimilated. Furthermore, some limitations may lead to an overestimate of the value-added impacts, such as the lack of spectral/spatial correlations, the polarization bias, the earth-rotation doppler shift impact, and the explicit errors in the synthetic radiances. It is believed that by resolving the limitations, the GEO sounders may more realistically characterize the forecast error in NWP models. In addition to applications in NWP, GEO hyperspectral IR data provide valuable information in the pre-convection environment for nowcasting and situation awareness (Li et al., 2011, 2012) and in other application areas, such as monitoring clouds, dust, atmospheric composition, aviation hazards, surface emissivity, and temperatures.
Acknowledgements. This work is supported by the NOAA GeoXO program (NA15NES4320001). The view, opinions, and findings contained in this report are those of the authors and should not be construed as an official National Oceanic and Atmospheric Administration’s or U.S. government’s position, policy, or decision. Thanks to the JCSDA (Joint Center for Satellite Data Assimilation) for providing the “S4” supercomputer (Supercomputer for Satellite Simulations and Data Assimilation) physically located at SSEC at the University of Wisconsin-Madison as the main computational resource for this research study.
Open Access This article is distributed under the terms of the Creative Commons Attribution 4.0 International License (





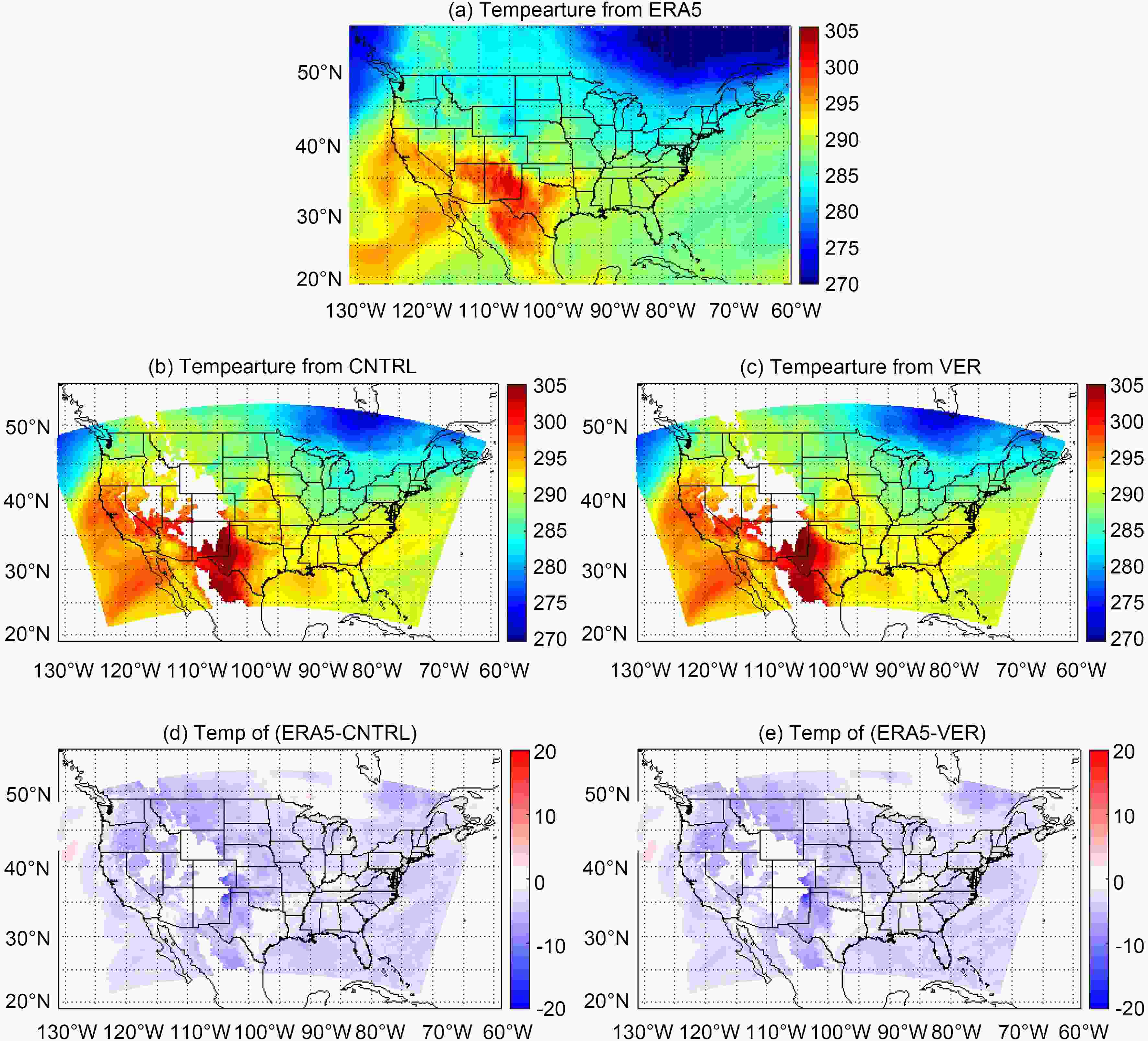
Forecast Hours | Analysis | 6-h | 12-h | 18-h | 24-h | |
RMSE | ERA5–CNTRL | 3.00 | 3.39 | 2.90 | 2.78 | 3.45 |
ERA5–Ver | 3.11 | 3.44 | 2.94 | 2.82 | 3.4 | |
Error at 95% confidence level | ERA5–CNTRL | 0.0083 | 0.0089 | 0.0067 | 0.0078 | 0.0098 |
ERA5–Ver | 0.0082 | 0.0088 | 0.007 | 0.0078 | 0.0095 |
Table1. The RMSE and the error at the 95% confidence level for the temperature fields (unit: K) of ERA5–CNTRL and ERA5–Ver at 850 hPa with different forecast hours. The red numbers show the smaller RMSE between the two groups of experiments.
Forecast Hours | 6-h | 12-h | 18-h | 24-h | |
ETS | CNTRL | 0.2215 (0.0025) | 0.2835 (0.0027) | 0.2964 (0.0027) | 0.2578 (0.0026) |
VER | 0.2195 (0.0025) | 0.281 (0.0027) | 0.2994 (0.0026) | 0.2513 (0.0026) | |
POD | CNTRL | 0.6668 (0.0028) | 0.7818 (0.0024) | 0.8254 (0.0023) | 0.8762 (0.002) |
VER | 0.6795 (0.0028) | 0.7883 (0.0024) | 0.8423 (0.0022) | 0.884 (0.0019) | |
FAR | CNTRL | 0.6741 (0.0028) | 0.5318 (0.003) | 0.4598 (0.003) | 0.4745 (0.003) |
VER | 0.6788 (0.0028) | 0.5358 (0.003) | 0.4614 (0.003) | 0.4809 (0.003) |
Table2. The ETS, POD, and FAR scores of CNTRL and VER for the 6-h, 12-h, 18-h, and 24-h accumulated precipitation over 0.1 mm. The 6-h represents the precipitation from 1800 UTC 23 June to 0000 UTC 24 June; the 12-h represents the precipitation from 1800 UTC 23 June to 0600 UTC 24 June; the 18-h represents the precipitation from 1800 UTC 23 June to 1200 UTC 24 June; and the 24-h represents the precipitation from 1800 UTC 23 June to 1800 UTC 24 June 2018. The error at the 95% confidence levels are italicized in the brackets.



RMSE | Errors at the 95% confidence level | ||||
CNTRL | EXP | CNTRL | EXP | ||
0000 UTC 26 May | T | 1.5478 | 1.5129 | 0.0036 | 0.0035 |
Q | 1.1186 | 0.8908 | 0.0025 | 0.0024 | |
U | 3.8785 | 3.7814 | 0.0102 | 0.0101 | |
V | 4.2881 | 4.1083 | 0.0114 | 0.0115 | |
0600 UTC 26 May | T | 1.6661 | 1.6032 | 0.0041 | 0.0036 |
Q | 0.9925 | 0.8947 | 0.0023 | 0.0023 | |
U | 3.8869 | 3.7143 | 0.0102 | 0.0096 | |
V | 4.3792 | 4.1691 | 0.0113 | 0.0108 | |
1200 UTC 26 May | T | 1.6013 | 1.5671 | 0.0038 | 0.0033 |
Q | 1.0255 | 0.8882 | 0.0025 | 0.0022 | |
U | 3.63 | 3.5673 | 0.0094 | 0.0092 | |
V | 4.2545 | 4.1192 | 0.0111 | 0.0107 | |
1800 UTC 26 May | T | 1.6376 | 1.5858 | 0.0039 | 0.0036 |
Q | 1.0286 | 0.8249 | 0.0025 | 0.0022 | |
U | 3.7635 | 3.499 | 0.01 | 0.092 | |
V | 4.162 | 4.0214 | 0.0112 | 0.0108 | |
0000 UTC 27 May | T | 1.5595 | 1.5534 | 0.0035 | 0.0033 |
Q | 1.046 | 0.9047 | 0.0024 | 0.0021 | |
U | 3.385 | 3.3084 | 0.0089 | 0.0085 | |
V | 2.5081 | 2.4035 | 0.0106 | 0.0102 | |
0600 UTC 27 May | T | 1.643 | 1.623 | 0.0039 | 0.0036 |
Q | 1.0488 | 0.9352 | 0.0025 | 0.0023 | |
U | 3.5483 | 3.4515 | 0.0092 | 0.0089 | |
V | 2.727 | 2.6447 | 0.0112 | 0.0109 | |
1200 UTC 27 May | T | 1.5663 | 1.5575 | 0.0036 | 0.0033 |
Q | 1.2015 | 0.9314 | 0.0024 | 0.0023 | |
U | 3.3338 | 3.28 | 0.0085 | 0.0083 | |
V | 3.9501 | 3.8747 | 0.0103 | 0.01 |
Table3. The RMSEs and errors at the 95% confidence level for temperature (T), moisture (Q), and winds (U/V) between ERA5 and CNTRL, and between ERA5 and EXP for seven groups of forecasts from 0000 UTC 26 May to 1200 UTC 27 May 2019.


Variables | CNTRL | EXP | Percentage change |
Temperature (K) | 1.6031 (0.0038) | 1.5718 (0.0035) | 2% |
Moisture (g kg?1) | 1.066 (0.0024) | 0.896 (0.0023) | 16% |
U-wind (m s?1) | 3.6433 (0.0095) | 3.5143 (0.0091) | 3.2% |
V-wind (m s?1) | 3.7527 (0.011) | 3.6201 (0.0107) | 3.5% |
(1– ETS) scores | 0.58 (0.0028) | 0.5522 (0.0028) | 1.5% |
(1–POD) scores | 0.2277 (0.0023) | 0.2425 (0.0024) | ?0.3% |
FAR scores | 0.2228 (0.0023) | 0.1933 (0.0022) | 0.7% |
Table4. The averaged RMSE of ERA5–CNTRL and ERA5–EXP for T/Q/U/V and ETS/POD/FAR scores for all the experiments of Case II. The percentage change between the EXP from CNTRL is listed in the last column. The 95% confidence levels are italicized in the brackets.
