


Using Neural Network Coupled Genetic Algorithm to Optimize the SPME Conditions of Volatile Compounds in Korla Pear
ZHANG Fang


通讯作者:
第一联系人:
责任编辑: 赵伶俐
收稿日期:2018-05-25接受日期:2018-09-17网络出版日期:2018-12-01
基金资助: |
Received:2018-05-25Accepted:2018-09-17Online:2018-12-01

摘要
关键词:
Abstract
Keywords:
PDF (515KB)元数据多维度评价相关文章导出EndNote|Ris|Bibtex收藏本文
本文引用格式
张芳, 未志胜, 王鹏, 李凯旋, 詹萍, 田洪磊. 基于BP神经网络和遗传算法的库尔勒香梨挥发性物质萃取条件的优化[J]. 中国农业科学, 2018, 51(23): 4535-4547 doi:10.3864/j.issn.0578-1752.2018.23.012
ZHANG Fang, WEI ZhiSheng, WANG Peng, LI KaiXuan, ZHAN Ping, TIAN HongLei.
0 引言
【研究意义】库尔勒香梨(Pyrus bretschneideri Rehd)属白梨系,具有细嫩多汁、香味浓郁、皮薄质脆等特点[1,2,3],除鲜食外,香梨汁加工已成为其产业链延伸的主导途径。然而,香梨汁在深加工过程中,天然果香的严重失真并伴随其风味的劣变严重限制了新疆库尔勒香梨产业的发展。因此,明晰库尔勒香梨中香气物质的组成并建立其相应的萃取分析方法,对于后期库尔勒香梨相关产品天然风味的拟合及调控极为重要。【前人研究进展】固相微萃取技术(SPME)是目前应用最广且有效的萃取挥发性物质的方法[4,5,6],其萃取效果多受萃取头种类、萃取温度、萃取时间、盐离子浓度和平衡时间等因素的影响。目前,多采用单因素逐级分析[7,8]、正交实验[9,10]和响应面等方法优化SPME萃取条件[11]。然而传统的单因素法费力、费时,无法准确确定最优萃取条件[12],正交实验存在同样的问题;响应面法虽然能够克服上述两种缺点,但其适用范围却存在一定的局限性,仅适用于二次模型[13]。BP神经网络结合遗传算法采用完整的“黑甲子”方法模拟数据,具有通用逼近能力,即其适用于所有的非线性多变量模型[12,14]。BP神经网络结合遗传算法不需要事先说明适合的拟合函数,建立的模型可以给出预测值与试验值的准确度[12,13,14,15,16,17,18]。有研究表明,在使用相同试验设计时,BP神经网络结合遗传算法寻优的效果远高于响应面法[19,20,21,22]。【本研究切入点】基于上述分析,本试验以前人确定的库尔勒香梨汁中特征风味物质[2,3]为导向,在考察SPME萃取各个因素分析的基础上,采用BP神经网络结合遗传算法对库尔勒香梨汁中香气物质萃取条件进行预测优化。【拟解决的关键问题】建立一套完善且相对较准确的库尔勒香梨汁挥发性物质固相微萃取分析方法,同时为研究食品中非线性模型工艺优化问题提供新思路。1 材料与方法
试验于2017年6—7月在石河子大学食品学院营养与风味实验室进行。1.1 材料与设备
于新疆石河子市农产品交易市场中的库尔勒香梨交易行随机购买6箱(每箱大概35—40个香梨)库尔勒香梨(Pyrus bretschneideri Rehd),存于4℃冷库中待用。正构烷烃混合标准品(C7—C40)购自美国Sigma公司;1, 2-二氯苯(内标),购自德国Dr. Ehrenstorfer公司;其他试剂均为国产分析纯,购自国药集团化学试剂有限公司。85 μm PA、50/30 μm DVB/CAR/PDMS、65 μm PDMS/DVB、100 μm PDMS和75 μm CAR/PDMS 5种萃取头购自美国Supelco公司;气相色谱质谱联用仪(GC-MS)5977A-7890B,美国Agilent公司;SJYZ11-200原汁机浙江苏泊尔股份有限公司。
1.2 试验方法
1.2.1 库尔勒香梨汁样本的制备 从4℃冷库中取出无损伤的库尔勒香梨置于室温下放置24 h,将恢复至室温的库尔勒香梨用纯净水洗净,去果梗、果核后,迅速采用原汁机榨汁,将香梨汁放于4℃下备用。1.2.2 库尔勒香梨汁香气物质萃取 取8.0 g的鲜榨库尔勒香梨汁于15 mL顶空瓶中,加入0.8 g的NaCl和0.2 μL的1,2-二氯苯溶液(1 μL?mL-1,内标)加盖封口。将封口的顶空瓶置于45℃温度下的水浴锅中平衡20 min后,将老化好的萃取头插入样品瓶的顶空部分,同时推出纤维头且使纤维头与香梨汁液面始终保持2 cm的距离,于平衡温度吸附30 min后,迅速移至GC进样口,解析7 min,同时启动GC-MS采集数据,每次试验均进行3次平行。
1.2.3 GC-MS分析 GC条件:HP-5 ms毛细管柱(30 m×250 μm,0.25 μm),升温程序:初始温度40℃,保持5 min,以4℃/min的速率升温至220℃,保持3 min,再以10℃/min的速率升温250℃,保持2min;进样口温度为250℃,载气(高纯He)流速1 mL?min-1,无分流进样。
MS条件:EI电离源,离子源温度200℃,传输线温度280℃,四级杆温度150℃,电子能量为70 eV,灯丝电流为80 μA,电子倍增器电压为1 000 V;质量扫面范围35—450 m/z。
定性分析:分离出的未知物与NIST/Wiley14相匹配(仅选匹配度大于80%的鉴定结果[2]),并与参考文献中的相关化合物的相对保留指数(RI值)进行对比分析。化合物保留指数[23]计算公式如下:
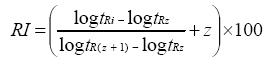
式中,RI为待测组分的保留指数;tRz和tR(z+1)为紧邻香气化合物i前后的2个正构烷烃的保留时间;z为正构烷烃的碳原子数;tRi为待测组分i的保留时间。
定量方法:采用1,2-二氯苯作为内标物质对香梨汁样品中的挥发性化合物进行定量分析,根据待测化合物和内标物相应的色谱峰面积之比,计算待测组分的相对含量,公式[24]如下:
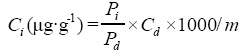
式中,Ci为待测组分i的相对含量;Pi是待测组分i的峰面积;Pd是内标物质的峰面积;Cd是内标物质的含量(mg);m是样品质量(g)。
1.3 SPME单因素试验
按照方法1.2.2中库尔勒香梨汁香气物质萃取方法制备样品,根据试验目的分别研究各单因素,包括萃取头(85 μm PA、50/30 μm DVB/CAR/PDMS、65 μm PDMS/DVB、100 μm PDMS和75 μm CAR/PDMS),样品量(4、6、8、10和12 g),萃取温度(35、40、45、50和55℃)、萃取时间(10、20、30、40和50 min)和NaCl添加量(0、0.4、0.8、1.2和1.6 g)对库尔勒香梨汁挥发性物质萃取效果的影响。1.4 BP神经网络与遗传算法结合优化SPME萃取条件
1.4.1 BP神经网络训练拟合 采用MATLAB 2016b软件,首先进行BP神经网络的训练拟合。训练网络时隐含层采用tansig函数作为激活函数;输出层采用线性函数purelin作为激活函数;输入层与隐含层间的传递函数使用S型函数。根据中央复合设计表1,确定BP神经网络的输入层神经元个数为4:分别是样品量、萃取温度、萃取时间和NaCl添加量;输出层神经元为库尔勒香梨汁中9种关键挥发性物质的含量;根据试错法[25]多次尝试训练选取最佳隐含层神经元个数为15。中央复合设计试验中总样本数为30(表1),运用随机函数从中抽取18个样本为训练样本,6个样本为测试样本,6个样本为验证样本[13]。所有输入和输出数据在网络训练前均进行归一化处理,避免训练前出现数值溢出。基于变化的输入/输出数据集进行BP神经网络模型的训练。使用相对误差(relative error)和均方误差(root mean square error,MSE)评价BP神经网络建模的能力,通过描述建模变量差异度的系数R2来确定模型的拟合优度。均方误差按如下公式计算: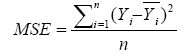
式中,

Table 1
表1
表1中央复合试验设计及结果
Table 1
序列 Sequence | 样品量 Sample amount (g) | 萃取温度 Extraction temperature (℃) | 萃取时间 Extraction time (min) | 盐添加量 Salt addition (g) | 试验值 Experimental value (μg?g-1) |
---|---|---|---|---|---|
1 | 4 | 40 | 20 | 0.4 | 2.24 |
2 | 8 | 40 | 20 | 0.4 | 2.02 |
3 | 4 | 50 | 20 | 0.4 | 2.25 |
4 | 8 | 50 | 20 | 0.4 | 1.20 |
5 | 4 | 40 | 40 | 0.4 | 2.75 |
6 | 8 | 40 | 40 | 0.4 | 2.54 |
7 | 4 | 50 | 40 | 0.4 | 2.52 |
8 | 8 | 50 | 40 | 0.4 | 2.21 |
9 | 4 | 40 | 20 | 1.2 | 1.87 |
10 | 8 | 40 | 20 | 1.2 | 2.18 |
11 | 4 | 50 | 20 | 1.2 | 2.52 |
12 | 8 | 50 | 20 | 1.2 | 1.10 |
13 | 4 | 40 | 40 | 1.2 | 1.45 |
14 | 8 | 40 | 40 | 1.2 | 2.46 |
15 | 4 | 50 | 40 | 1.2 | 2.15 |
16 | 8 | 50 | 40 | 1.2 | 1.41 |
17 | 6 | 45 | 30 | 0.8 | 2.30 |
18 | 6 | 45 | 30 | 0.8 | 2.26 |
19 | 6 | 45 | 30 | 0.8 | 2.12 |
20 | 6 | 45 | 30 | 0.8 | 1.60 |
21 | 2 | 45 | 30 | 0.8 | 1.16 |
22 | 10 | 45 | 30 | 0.8 | 2.05 |
23 | 6 | 35 | 30 | 0.8 | 2.49 |
24 | 6 | 55 | 30 | 0.8 | 1.69 |
25 | 6 | 45 | 10 | 0.8 | 3.00 |
26 | 6 | 45 | 50 | 0.8 | 3.19 |
27 | 6 | 45 | 30 | 0 | 3.34 |
28 | 6 | 45 | 30 | 1.6 | 3.22 |
29 | 6 | 45 | 30 | 0.8 | 3.57 |
30 | 6 | 45 | 30 | 0.8 | 3.27 |
新窗口打开|下载CSV
1.4.2 遗传算法寻优 遗传算法(genetic algorithm,GA)是基于达尔文进化论和孟德尔遗传学来实现随机、自适应、并行性全局搜索寻优的方法。遗传算法寻优[26,27]步骤如图1所示。
图1
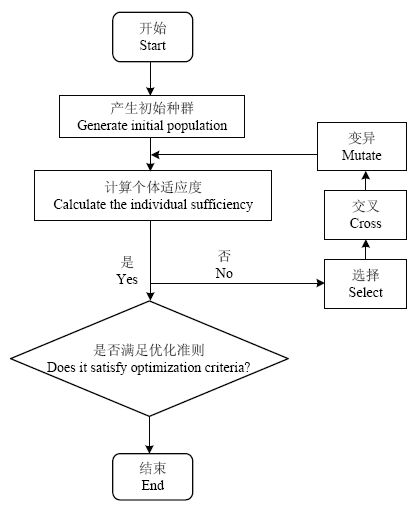
图1BP神经网络结合遗传算法优化的流程图
Fig. 1The flow chart of the neural network coupled genetic algorithm optimization
1.4.3 数据统计分析利用Microsoft Office Excel 2007 进行数据统计和计算,不同样本物质含量的方差分析和邓肯多重比较均使用SPSS 23.0软件(SPSS,Chicago,IL,USA)。采用统计软件包(Design Expert 7.0.1.0,Statease,USA)进行统计分析,生成试验设计(DOE)。用于构建神经网络的数据使用MATLAB 2016b(The Mathworks Inc.)进行处理。图采用Origin 2016软件进行绘制。所有数据均采用3次平行试验的平均值。
2 结果
2.1 SPME单因素试验结果
2.1.1 萃取头的选择 萃取纤维头的涂层材料决定了萃取头的极性和对不同挥发性物质的吸附能力[28]。由图2可知,不同萃取头萃取出的库尔勒香梨汁的物质含量具有显著差异(P<0.05),表明萃取头对SPME萃取效果影响较大。其中强极性萃取头PA对库尔勒香梨汁中除己醛以外的其他物质的萃取效果明显低于其他萃取头(P<0.05);中极性萃取头PDMS对于(Z)-2-己烯醛、壬醛和丙酸乙酯萃取效果显著高于其他4种萃取头(P<0.05),而对于己醇和(E)-2-己烯醛则显著优于CAR/PDMS、CAR/DVB/PDMS和PA(P<0.05);DVB/PDMS对除己酸乙酯、丙酸乙酯、壬醛和(Z)-2-己烯醛以外其他所有的香气物质均具有较好的萃取效果,各种物质的含量均显著优于其他萃取头(P<0.05);CAR/PDMS和CAR/DVB/PDMS虽然对于库尔勒香梨汁中的挥发性物质的吸附能力优于PA和PDMS,但低于DVB/PDMS萃取头。因此,最终选定DVB/PDMS做为香梨汁挥发性物质最佳萃取头进行后续试验。图2
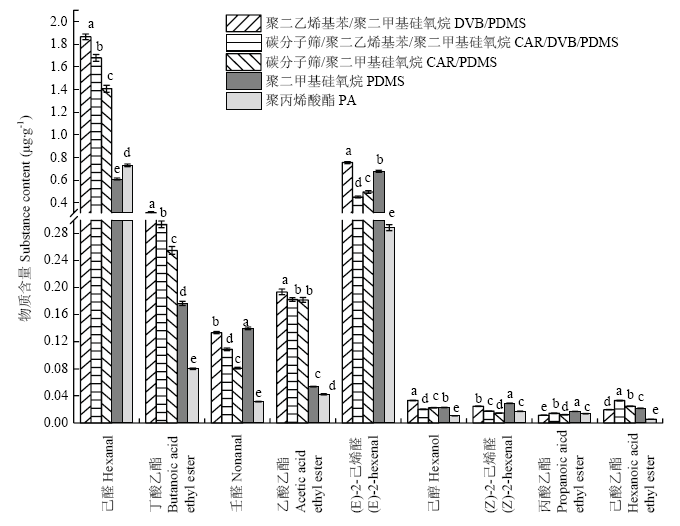
图2不同萃取头对库尔勒香梨汁主要香气物质含量的影响
不同小写字母表示同一香气物质上存在显著性差异(P<0.05)。下同
Fig. 2Effects of different extraction fibers on major aroma substance of Koral pear juice
Different lowercase values represent there are significant differences in the same aroma substances among treatments (P<0.05). The same as below
2.1.2 样品量对萃取效果的影响 样品量对库尔勒香梨汁中挥发性物质的影响如图3,从图3可知,不同样品量中的挥发性物质含量存在显著性差异(P<0.05),表明样品量对库尔勒香梨汁中的挥发性物质含量影响较大。随着样品量的增加,挥发性物质己醛、丁酸乙酯、壬醛、己酸乙酯、(E)-2-己烯醛、己醇、(Z)-2-己烯醛和丙酸乙酯的含量先增加后减少;乙酸乙酯的含量随着样品量的增加而减少。样品量为6 g时,己醛、丁酸乙酯、壬醛、己酸乙酯、(E)-2-己烯醛、(Z)-2-己烯醛和丙酸乙酯的含量达到最大值;乙酸乙酯的含量在样品量为4 g时达到最大;样品量为10 g时,己醇的含量达到最大值,因此,在单因素试验中选择6 g为最佳萃取温度。
图3
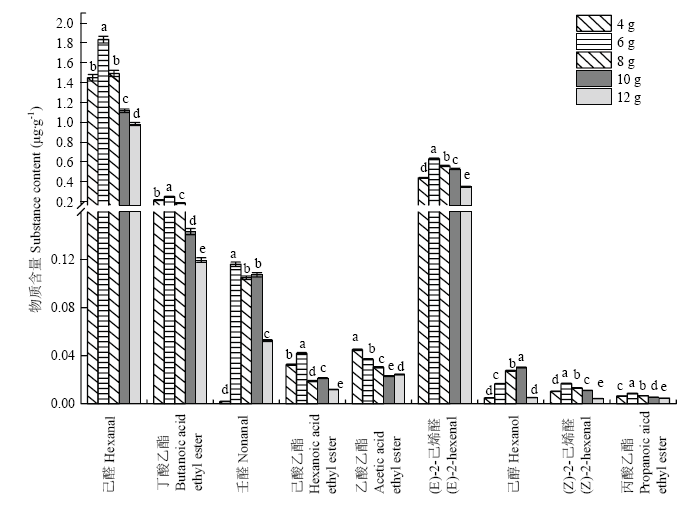
图3不同样品量对库尔勒香梨汁挥发性物质含量的影响
Fig. 3Effects of different sample amount on volatile substance in Koral pear juice
2.1.3 萃取温度对萃取效果的影响 从图4可知,不同萃取温度下的挥发性物质含量存在显著性差异(P<0.05),表明萃取温度对库尔勒香梨汁中的挥发性物质含量影响较大。己醛、丁酸乙酯、壬醛、己酸乙酯、乙酸乙酯、(E)-2-己烯醛、己醇、(Z)-2-己烯醛和丙酸乙酯的含量随着温度的升高先增加后降低。在萃取温度为45℃时,己醛、丁酸乙酯、壬醛、己酸乙酯、乙酸乙酯、(E)-2-己烯醛和丙酸乙酯的含量达到最大值;己醇含量在40℃时达到最大,这可能是由于高温导致己醇从萃取纤维头上解析到顶空瓶[29];(Z)-2-己烯醛含量在50℃时达到最大,因此,在单因素试验中选择45℃为最佳萃取温度。
图4
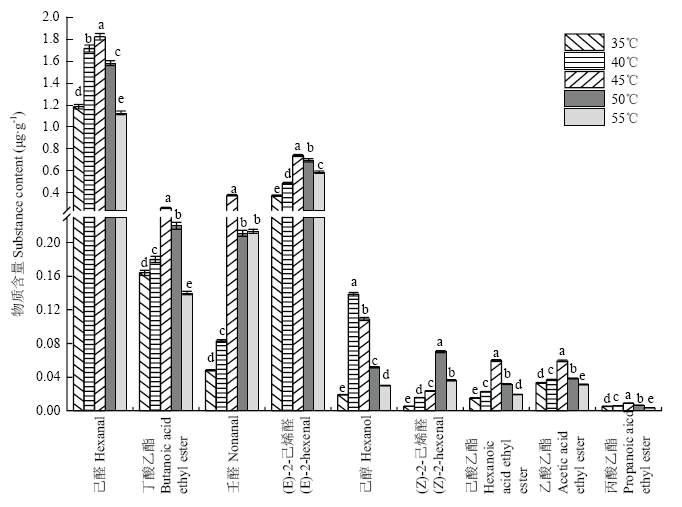
图4不同萃取温度对库尔勒香梨汁挥发性物质含量的影响
Fig. 4Effects of different extraction temperature on volatile substance in Koral pear juice
2.1.4 萃取时间对萃取效果的影响 由图5可知,萃取时间不同时,库尔勒香梨汁中的挥发性物质含量存在显著性差异(P<0.05),表明萃取时间对库尔勒香梨汁中的挥发性物质含量影响较大。随着萃取时间的增长,己醛、丁酸乙酯、壬醛、己酸乙酯、(E)-2-己烯醛、己醇、(Z)-2-己烯醛和丙酸乙酯的含量升高,但当萃取时间增加到一定值后,挥发性物质含量降低,这可能是由于其较低的扩散系数和较高的沸点造成[30]。乙酸乙酯的含量随着时间的增加减少,在10 min时的含量显著高于其他时间的物质含量(P<0.05),这可能是由于其需要较短的平衡时间[31]。己醛在20 min 时含量达到最大值;丁酸乙酯、壬醛、己酸乙酯、(E)-2-己烯醛、(Z)-2-己烯醛和丙酸乙酯的含量在30 min时达到最大值;在40 min时,己醇含量达到最大值。因此在上述分析的基础上,单因素试验中选择30 min为最佳萃取时间。
图5
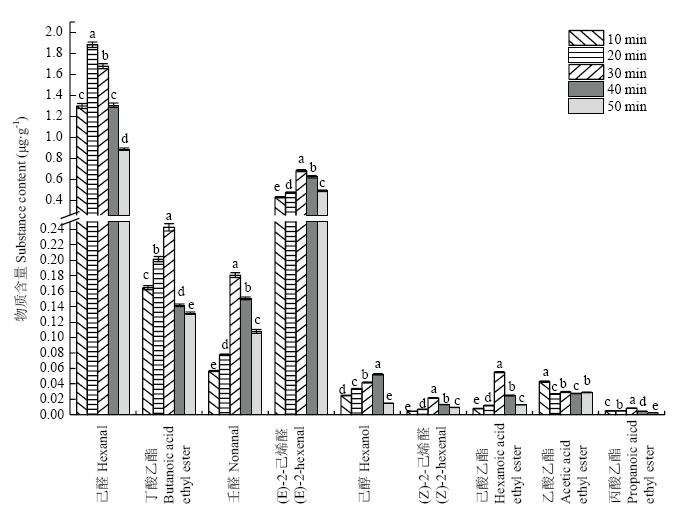
图5不同萃取时间对库尔勒香梨汁挥发性物质含量的影响
Fig. 5Effects of different extraction time on volatile substance in Koral pear juice
2.1.5 盐离子添加量对萃取效果的影响 由图6可知,盐添加量不同时,库尔勒香梨汁中的挥发性物质含量存在显著性差异(P<0.05),表明盐添加量对对库尔勒香梨汁中的挥发性物质含量影响也较大。随着盐离子添加量由0 g增加到0.8 g,己醛、丁酸乙酯、壬醛、己酸乙酯和丙酸乙酯的含量逐渐增加,在0.8 g时达到最大值;(E)-2-己烯醛、己醇和(Z)-2-己烯醛在0.6 g时达到最大值,这是由于盐离子能减少被分析物在水溶液中的可溶性[32]。盐离子添加量由0.8 g增加到1.6 g时,各个物质含量逐渐减少,这可能与盐离子表面的阻碍吸附有关[29]。因此,在单因素试验中选择0.8 g 为最佳盐离子添加量。
图6
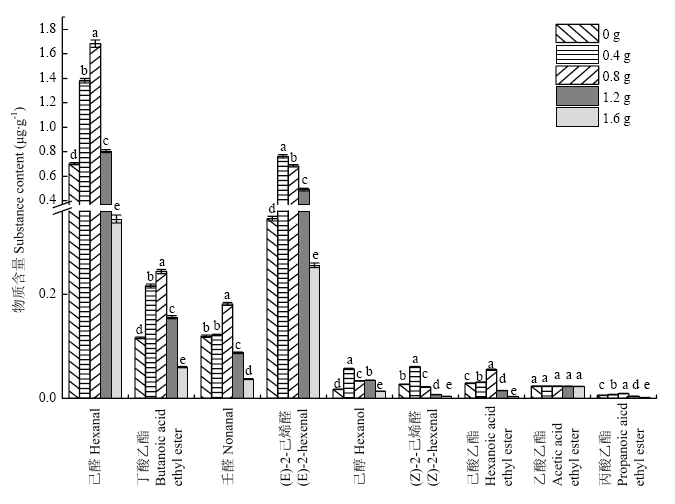
图6不同盐离子添加量对库尔勒香梨汁挥发性物质含量的影响
Fig. 6Effects of different salt addition on volatile substance in Koral pear juice
2.2 BP神经网络模型分析
2.2.1 BP网络模型性能分析 图7-a为神经网络误差的下降曲线图。训练、测试和验证网络中比较试验结果和神经网络的预测结果的散点图如图7-b所示。数据在45°附近散射时,表明试验结果和网络预测值间有很好的兼容性。由图7-a可知,随着训练步数的增加,模型误差曲线趋近于误差最优值。开发的BP神经网络在第3次迭代时,训练误差低于误差最优值0.017,验证误差趋近于误差最优值0.017;在第4次迭代时,网络训练较稳定且收敛迅速,模型能够满足试验需求;构建的BP神经网络的最好验证误差是0.0173,表明该神经网络模型可用。且在验证曲线增加之前,测试曲线未显著增加,因此网络没有过度训练[33]。由图7-b可知,在验证误差为0.017时,BP神经网络模型的训练、测试、验证的相关系数分别为0.990、0.951和0.973(相关系数均大于0.95),表明开发的BP网络模型对于训练样本、测试样本和验证样本都有很好的逼近能力,能很好的描述试验因素(样品量、萃取温度、萃取时间和盐添加量)和物质含量间的关系,可以用于SPME萃取条件的优化。图7
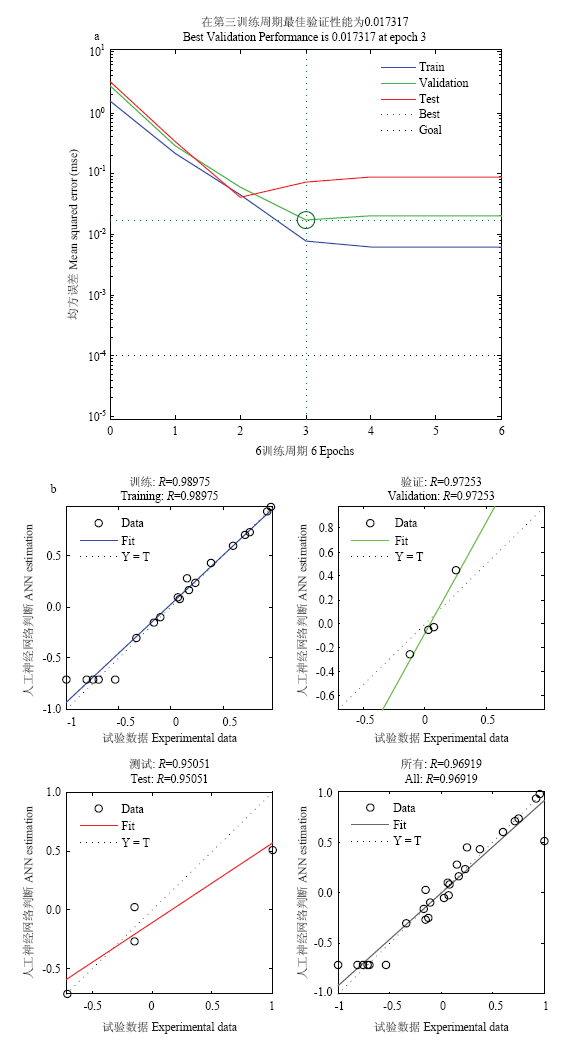
图7神经网络均方误差曲线(a)、试验值与预测值间的相关性(b)
Fig. 7MSE curve of BP neural network (a), the correlation between experimental and predicted value (b)
2.2.2 BP神经网络泛化能力的验证 BP神经网络模型泛化能力只有在完全看不见的数据集中才能被判断[12]。神经网络模型的泛化能力是指训练好的BP网络模型对不在训练集中的数据的预测能力。在中央复合设计因素表范围内重新随机组合试验因素水平(表2)进行BP神经网络泛化能力的验证。模型的拟合度用决定系数R2表示。由图8可知,BP神经网络模型的拟合度R2为0.992,试验值变异性的99.2%可以通过此模型来解释,表明建立的BP神经网络模型具有很好的泛化能力,模型能够准确预测不在训练集内的数据集的输出结果。
Table 2
表2
表2验证试验数据集
Table 2
序列 Sequence | 样品量 Sample amount (g) | 萃取温度 Extraction temperature (℃) | 萃取时间 Extraction time (min) | 盐添加量 Salt addition (g) | 实验值 Observed value (μg?g-1) | 预测值 Predictive value (μg?g-1) | 相对误差 Relative error (%) |
---|---|---|---|---|---|---|---|
1 | 7 | 50 | 30 | 0.8 | 1.52 | 1.54 | -1.60 |
2 | 4 | 45 | 15 | 0.5 | 2.88 | 2.81 | 2.43 |
3 | 6 | 40 | 15 | 0.7 | 2.24 | 2.27 | -1.34 |
4 | 5 | 45 | 25 | 0.9 | 3.10 | 3.00 | 3.23 |
5 | 6 | 35 | 20 | 1.0 | 2.05 | 2.11 | -2.93 |
6 | 5 | 50 | 25 | 0.5 | 1.96 | 2.02 | -3.06 |
7 | 3 | 35 | 25 | 0.8 | 1.64 | 1.59 | 1.24 |
新窗口打开|下载CSV
图8
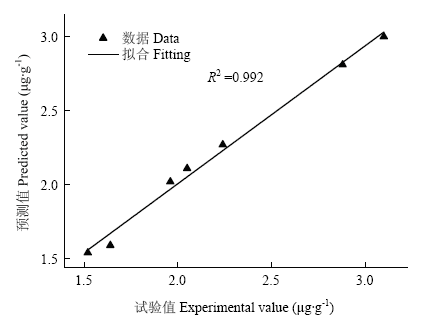
图8试验值与预测值拟合度
Fig. 8The fitting degree between experimental value and predicted value
2.2.3 SPME萃取条件的优化 利用遗传算法对已建立的BP神经网络模型进行全局寻优,以库尔勒香梨汁中的9种关键挥发性物质(己醛、丁酸乙酯、壬醛、己酸乙酯、乙酸乙酯、(E)-2己烯醛、己醇、(Z)-2-己烯醛和丙酸乙酯)含量最大为优化目标。遗传算法参数设置为:最大进化代数100、初始种群数20、变异概率0.2、交叉概率0.4。在此条件下进行寻优,得到每代种群适应度变化,结果如图9所示。
图9
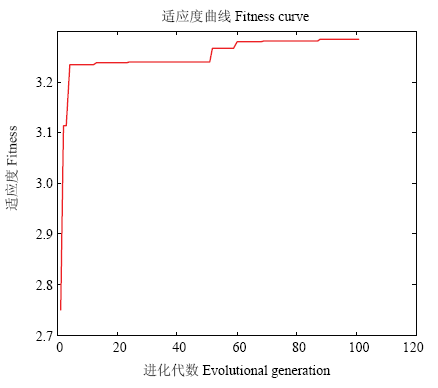
图9遗传算法的最优适应度曲线
Fig. 9Optimized fitness curve of genetic algorithm
从图9可知,随着迭代次数的增加,种群的适应度先呈现曲折上升的趋势,后逐渐趋于平稳状态。表明此时群体中对应于最大适应度的个体已经达到该优化算法下的最优解[34]。最优个体为3.41 μg?g-1,此时SPME萃取条件为:样品量5.33 g、萃取温度44.70℃、萃取时间25.22 min和盐添加量0.63 g。
3 讨论
香气成分是构成鲜食果品及加工品品质的主要因素,对果实品质起着非常重要的作用。果实的香气是由各种挥发性成分(酯类、醛类、醇类、酮类以及萜类化合物等)共同作用形成的,对果实风味品质以及市场前景起着重要作用,也是吸引消费者和增强市场竞争力的重要因素之一。因此对于果品香气成分分析及其影响因素方面的研究日益受到关注。SPME技术将萃取、浓缩和进样集成单个无溶剂步骤和装置,大大简化了样品的制备过程[35],其优点在于即使采用较低的萃取温度也可以获得较高的灵敏度,为此更为真实的呈现了样品原有风味[36]。CHEN等[37]采用了SSDE(Simultaneous Steam Distillation Extraction)和SPME对杏中香气成分进行分析,相较于SSDE技术,SPME萃取效果显著提升,特别是对于一些杏中有助于鲜杏香气的关键物质,如乙酸己酯、β-紫罗兰酮、乙酸丁酯和柠檬烯。周志等[38]在萃取分析野生刺梨汁挥发性物质的研究中同样发现SPME更适用于野生刺梨汁中挥发性成分的分析。
然而在具体研究SPME各因素对样品中挥发性成分萃取效果评价时,多数研究以检出物质的总峰面积或数量作为评价指标[8-9,39]。与上述研究不同,本研究借鉴课题组前期试验结果,初步筛选己醛、丁酸乙酯、壬醛、乙酸乙酯、(E)-2-己烯醛、己醇、(Z)-2-己烯醛、丙酸乙酯和己酸乙酯9种物质作为库尔勒香梨特征香气物质,并以其含量为指标进行相关研究;较前者评价指标相比,采用库尔勒香梨特征香气物质评价体系更有针对性,且省去了对样品中全部香气成分分析鉴定的繁琐步骤,极大降低了分析研究工作量。
优化SPME萃取条件,建立一套完善且相对较准确的水果果实挥发性物质萃取分析方法,可为后续相关产品风味的自然呈现及调控提供理论依据。目前,针对库尔勒香梨汁SPME萃取条件的优化,LI等[39]通过单因素逐级分析法研究了萃取头种类、萃取时间和萃取温度对库尔勒香梨汁挥发性物质萃取效果的影响,发现最佳萃取头为65 μm PDMS/ DVB,这一结论与本试验一致。然而,本研究通过BP神经网络结合遗传算法优化获得的最佳萃取温度(45℃)和萃取时间(25 min)与LI等[39]研究结果存在较大偏差(其萃取温度和萃取时间分别是40℃和45 min)。此外,与陈计峦等[3]通过正交试验优化获得的SPME最佳萃取温度(40℃)和萃取时间(30 min)相比,在本试验条件下实施SPME萃取,可大幅度提升库尔勒香梨特征香气物质己醛、(E)-2-己烯醛和丙酸乙酯的含量,这可能是基于试验构建的预测能力较好的BP神经网络模型能够充分模拟SPME萃取过程,同时借助遗传算法强大的寻优功能所致。
研究表明BP神经网络结合遗传算法的优化方法均优于传统试验优化方法[12,13,14,15,16,17,18],然而目前该优化方法在食品方面的应用相对较少[40,41,42]。在未来可以采用BP神经网络结合遗传算法优化食品加工、食品原料天然物质提取和果蔬贮藏等工艺的相关参数。
4 结论
萃取头种类、样品量、萃取温度、萃取时间和盐添加量对固相微萃取具有显著影响。基于BP神经网络建立的模型具有很好的逼近能力,可以用于固相微萃取过程的预测。在本试验选择的水平范围内,通过遗传算法成功优化了SPME萃取条件。在尽可能接近此条件下(样品量5.33 g、萃取温度45℃、萃取时间 25 min和NaCl添加量0.63 g)测出的挥发性物质含量为(3.37±0.23)μg?g-1,与最优个体的相对误差为-1.19%,表明BP神经网络结合遗传算法能准确地寻找到固相微萃取的最优参数。参考文献 原文顺序
文献年度倒序
文中引用次数倒序
被引期刊影响因子
DOI:10.1016/j.lwt.2015.08.011URL [本文引用: 1]

Korla pear juice was treated by ultrafiltration (UF) followed by high pressure processing (HPP, 500MPaat ambient temperature for 10min) or high temperature short time (HTST, 110°C for 8.6s). Total plate count (TPC) and yeast & mold (Y&M) were reduced by 3.05 and 3.36 log 10 CFU/mL after UF, and further reduced below the detection level (≤10CFU/mL) after the following HPP or HTST. Clarity of UF-HPP and UF-HTST juices were 99.66% and 95.89%. Ascorbic acid and total phenols were greater in UF-HPP juice compared to UF-HTST juice, whilst Δ E of UF-HPP juice was smaller than that of UF-HTST juice with the corresponding values of 0.04 and 1.15. During 56 days of storage at 4°C, all samples showed microbial safety. Minor changes of clarity had been found in UF-HPP juice with the value from 99.66% to 99.11%, while it was significantly decreased from 95.89% to 94.00% in UF-HTST juice during storage. Less decrease in total phenols and antioxidant capacity during storage were observed in UF-HPP juice compared to UF-HTST juice. UF-HPP process can be used as an alternative approach to produce high quality korla pear juice with a shelf life of more than 56 days at 4°C.
[本文引用: 3]
DOI:10.3321/j.issn:0253-990X.2007.03.028URL [本文引用: 3]

用固相微萃取技术(SPME)提取梨果实中香气成分,对影响萃取效果的因素及实验参数进行探讨、优化,确定了最佳萃取条件,同时结合GC/MS对提取的成分进行分析鉴定,为梨果实中香气成分的快速测定提供技术保证。
DOI:10.3321/j.issn:0253-990X.2007.03.028URL [本文引用: 3]

用固相微萃取技术(SPME)提取梨果实中香气成分,对影响萃取效果的因素及实验参数进行探讨、优化,确定了最佳萃取条件,同时结合GC/MS对提取的成分进行分析鉴定,为梨果实中香气成分的快速测定提供技术保证。
DOI:10.1007/s11947-009-0299-3URL [本文引用: 1]

AbstractSolid-phase microextraction (SPME) is a relatively new sampling technique wherein sample extraction and pre-concentration could be achieved in a single step. The handling of an SPME device is simple, and the analysis of volatiles could be easy. However, the process becomes complex while analyzing sample matrices of heterogeneous nature. The complexity also increases depending upon the nature of compounds to be extracted. Careful selection and optimization of extraction parameters like fiber coating selection, extraction time, agitation, addition of salt, and extraction temperature have to be undertaken to improve the sensitivity and the reproducibility of this method. This paper reviews the principles associated with SPME technique from a general application viewpoint. Also, a comprehensive review of prior research related to characterization of food quality has been reported. SPME-related solutions for environmental applications have also been analyzed to be applied for new food-related applications.
DOI:10.1016/j.foodchem.2008.01.040URLPMID:26050188 [本文引用: 1]

The volatile compounds of Chinese traditional smoke-cured bacon (CSCB) were studied using SPMS-GC/MS. There were 48 volatile compounds identified and quantified, which belonged to several classes of chemical: 1 alkane, 16 aldehydes, 5 ketones, 9 alcohols, 4 thioethers and thiols, 3 furans and 10 phenols compounds. All the volatile compounds except for alkane was responsible for CSCB characteristic flavor. The major volatile compounds of CSCB came from smoking, oxidation and Maillard reaction, etc. Many volatile compounds were not reported in previous paper isolated by steam distillation method or nitrogen purge-and-steam distillation method on CSCB. It should be because of different method of isolating volatile substances from CSCB. Among the fibers tested, CAR/PDMS (carboxen/polydimethylsiloxane) fiber coating showed the highest area counts for most volatile compounds. CAR/PDMS coating extracted better those compounds whose linear retention indices (LRI) was lower than 926 (on average) and DVB/CAR/PDMS (divinylbenzene/carboxen/polydimethylsiloxane) those with higher LRI.
DOI:10.1021/ac00062a008URL [本文引用: 1]
URL [本文引用: 1]

运用顶空固相微萃取结合气相色 谱-质谱联用技术分析番木瓜香气成分,考察萃取头类型、萃取时间、样品量和氯化钠添加量4个单因素对萃取成分的影响。结果表明最佳萃取条件为萃取 头:50/30μm DVB/CAR/PDMS;样品量:6g番木瓜浆液;氯化钠添加量:3g;萃取时间:30min。将此条件下所得谱图与标准谱图比对分析,共鉴定出28种 化合物,占总峰面积的90.82%,番木瓜主要香气化合物为甲苯(36.68%)、芳樟醇(33.55%)、异硫氰酸苄酯(7.04%)、顺式芳樟醇氧化 物(2.56%)和氧化芳樟醇(1.46%)。
URL [本文引用: 1]

运用顶空固相微萃取结合气相色 谱-质谱联用技术分析番木瓜香气成分,考察萃取头类型、萃取时间、样品量和氯化钠添加量4个单因素对萃取成分的影响。结果表明最佳萃取条件为萃取 头:50/30μm DVB/CAR/PDMS;样品量:6g番木瓜浆液;氯化钠添加量:3g;萃取时间:30min。将此条件下所得谱图与标准谱图比对分析,共鉴定出28种 化合物,占总峰面积的90.82%,番木瓜主要香气化合物为甲苯(36.68%)、芳樟醇(33.55%)、异硫氰酸苄酯(7.04%)、顺式芳樟醇氧化 物(2.56%)和氧化芳樟醇(1.46%)。
[本文引用: 2]
[本文引用: 2]
DOI:10.16693/j.cnki.1671-9646(X).2017.04.037URL [本文引用: 2]

以油条为研究对象,采用单因素试验和正交试验优化了顶空固相微萃取油条风味物质的操作条件,并结合气 相色谱- 质谱对油条的挥发性风味物质组成进行了分析.结果表明,最佳的萃取条件为CAR/PDMS萃取头,70 ℃水 浴萃取40 min,解析时间5 min.油条皮中共检测出8 2种风味物质,其中醛类2 3种、酮类 5 种、醇 类7种、酸类 2 种、酯类 3 种、碳氢类化合物16种、杂环类12种、烷基吡嗪类1 2种、含氮和硫类化合物2 种 ;醛类为油条的主 要风味成分.
.
DOI:10.16693/j.cnki.1671-9646(X).2017.04.037URL [本文引用: 2]

以油条为研究对象,采用单因素试验和正交试验优化了顶空固相微萃取油条风味物质的操作条件,并结合气 相色谱- 质谱对油条的挥发性风味物质组成进行了分析.结果表明,最佳的萃取条件为CAR/PDMS萃取头,70 ℃水 浴萃取40 min,解析时间5 min.油条皮中共检测出8 2种风味物质,其中醛类2 3种、酮类 5 种、醇 类7种、酸类 2 种、酯类 3 种、碳氢类化合物16种、杂环类12种、烷基吡嗪类1 2种、含氮和硫类化合物2 种 ;醛类为油条的主 要风味成分.
DOI:10.1016/j.carbpol.2007.12.026URLPMID:26048221 [本文引用: 1]

Yield of polysaccharides from (3)) were investigated to get the best extraction conditions. The results showed that extraction temperature, ratio of phosphate buffer to raw material, extraction time, and ratio of trypsinase to raw material were the main four variables that influenced the yields of extracts. The highest yield was obtained when extraction temperature, ratio of phosphate buffer to raw material, extraction time and ratio of trypsinase to raw material were 4002°C, 2, 5.502h and 1.6, respectively. The immunity-stimulating method showed that polysaccharides from P. esulenta could significantly raise liver, spleen and thymus index of mice and enhance Con A-stimulated mouse spleen cells proliferation. These results indicate that polysaccharides from P. esulenta had significantly higher immunity-stimulating activities.
DOI:10.1016/j.talanta.2013.08.037URLPMID:24209315 [本文引用: 1]

61Heterocyclic compounds could be implicated in wine ageing named Bouquet of wines.61HS-SPME optimization by central composite design and the response surface methodology.61The developed method allows the simultaneous determination of 24 heterocyclic compounds.
DOI:10.1016/j.bej.2008.05.009URL [本文引用: 5]

Response surface methodology (RSM) is the most preferred method for fermentation media optimization so far. In last two decades, artificial neural network-genetic algorithm (ANN-GA) has come up as one of the most efficient method for empirical modeling and optimization, especially for non-linear systems. This paper presents the comparative studies between ANN-GA and RSM in fermentation media optimization. Fermentative production of biopolymer scleroglucan has been chosen as case study. The yield of scleroglucan was modeled and optimized as a function of four independent variables (media components) using ANN-GA and RSM. The optimized media produced 16.22 0.44 g/l scleroglucan as compared to 7.8 0.54 g/l with unoptimized medium. Two methodologies were compared for their modeling, sensitivity analysis and optimization abilities. The predictive and generalization ability of both ANN and RSM were compared using separate dataset of 17 experiments from earlier published work. The average % error for ANN and RSM models were 6.5 and 20 and the CC was 0.89 and 0.99, respectively, indicating the superiority of ANN in capturing the non-linear behavior of the system. The sensitivity analysis performed by both methods has given comparative results. The prediction error in optimum yield by hybrid ANN-GA and RSM were 2% and 8%, respectively.
DOI:10.1016/j.ultsonch.2014.10.019URLPMID:25457517 [本文引用: 4]

The present study is to evaluate and compare the prediction and simulating efficiencies of response surface methodology (RSM) and artificial neural network (ANN) based models on fatty acid methyl esters (FAME) yield achieved from muskmelon oil (MMO) under ultrasonication by two step in situ process. In first in situ process, free fatty acid content of MMO was reduced from 6.43% to 0.91% using H2SO4 as acid catalyst and organic phase in the first step was subjected to second reaction by adding KOH in methanol as basic catalyst. The influence of process variables (methanol to oil molar ratio, catalyst concentration, reaction temperature and reaction time) on conversion of FAME (second step) was investigated by central composite rotatable design (CCRD) of RSM and Multi-Layer Perceptron (MLP) neural network with the topology of 4-7-1. Both (RSM and ANN) were statistically compared by the coefficient of determination, root mean square error and absolute average deviation, based on the validation data set. The coefficient of determination (R2) calculated from the validation data for RSM and ANN models were 0.869 and 0.991 respectively. While both models showed good predictions in this study. But, the ANN model was more precise compared to the RSM model and it showed that, ANN is to be a powerful tool for modeling and optimizing FAME production.
DOI:10.1007/s10295-004-0171-4URLPMID:15480940 [本文引用: 3]

Abstract Many variables and their interactions can affect a biotechnological process. Testing a large number of variables and all their possible interactions is a cumbersome task and its cost can be prohibitive. Several screening strategies, with a relatively low number of experiments, can be used to find which variables have the largest impact on the process and estimate the magnitude of their effect. One approach for process screening is the use of experimental designs, among which fractional factorial and Plackett-Burman designs are frequent choices. Other screening strategies involve the use of artificial neural networks (ANNs). The advantage of ANNs is that they have fewer assumptions than experimental designs, but they render black-box models (i.e., little information can be extracted about the process mechanics). In this paper, we simulate a biotechnological process (fed-batch growth of baker's yeast) to analyze and compare the effect of random experimental errors of different magnitudes and statistical distributions on experimental designs and ANNs. Except for the situation in which the error has a normal distribution and the standard deviation is constant, it was not possible to determine a clear-cut rule for favoring one screening strategy over the other. Instead, we found that the data can be better analyzed using both strategies simultaneously.
[本文引用: 2]
DOI:10.1007/s40242-014-3410-xURL [本文引用: 2]

A novel method for rapid, accurate and nondestructive determination of trimethoprim in complex matrix was presented. Near-infrared spectroscopy coupled with multivariate calibration(partial least-squares and artificial neural networks) was applied in the experiment. The variable selection process based on a modified genetic algorithm with fixed number of selected variables was proceeded, which can reduce the training time and enhance the predictive ability when coupled with artificial neural network model.
[本文引用: 2]
DOI:10.1016/j.jfoodeng.2005.11.025URL [本文引用: 2]

In this study, estimation capabilities of response surface methodology (RSM) and artificial neural networks (ANN) in a biochemical process were investigated. An enzymatic reaction catalyzed by amyloglucosidase was selected as the model biochemical process. The initial reaction rate of enzymatic reaction was investigated as a function of two independent variables, substrate concentration and reaction pH with two different experimental designs, face-centered design (FCD) and modified face-centered design (MFCD) by means of two different estimation techniques, RSM and ANNs. After prediction of the model equation in RSM and training of the artificial neurons in ANNs, using the data of 13 experimental points, the products were used for estimation of the response of the 35 experimental points. Estimated responses were compared with the experimentally determined responses and prediction capabilities of RSM and ANNs were determined. The best estimation was done by ANNs, which was trained with the data of MFCD. The coefficient of determination (R2) and average absolute deviation (AAD) values between actual and estimated responses were determined as 0.98 and 5.35%, respectively. The actual and estimated data were used for the determination of kinetic constants of enzymatic reaction, maximum reaction rate (Vmax) and Michaelis鈥揗enten constant (Km), at five different pH levels. The closest kinetic constants to actual values were obtained when the data of ANNs-MFCD were used. The R2 and AAD values were determined as 0.97 and 5.15% for Vmax and 0.96 and 7.18% for Km, respectively.
DOI:10.1016/S0731-7085(97)00170-2URLPMID:9608428 [本文引用: 1]

Abstract The use of artificial neural networks (ANNs) for response surface modelling in HPLC method development for amiloride and methychlothiazide separation is reported. The independent input variables were pH and methanol percentage in mobile phase. The outputs were capacity factors. The results were compared with a statistical method (multiple nonlinear regression analysis). Networks were able to predict the experimental responses more accurately than the regression analysis.
DOI:10.1016/S0963-9969(01)00074-6URL [本文引用: 1]

An artificial neural networks (ANNs) prediction is proposed based on effects of temperature ( T), pH and water activity ( a w) on the thermal inactivation of Escherichia coli. Using the data of Reichart [Reichart, O. (1994). Modelling the destruction of Listeria monocytogenes on the base (sic) of reaction kinetics. International Journal of Food Microbiology, 23, 449-465)], root-mean-squares error and determination coefficient ( R 2) of prediction were 0.144 and 0.949, 0.232 and 0.868 and 0.234 and 0.815 for ANN, response surface methodology and Cerf's model [Cerf, O., Davey, K.R. & Sadoudi, A.K. (1996). Thermal inactivation of bacteria02— a new predictive model for the combined effect of three environmental factors: temperature, pH and water activity. Food Research International, 29, 219–226.], respectively. This result demonstrates higher accuracy of ANN models than those of other two models. Of these three environmental factors, temperature was most important for thermal inactivation of E. coli. The other two factors were less but equally important. The effects of thermal inactivation of bacteria were neither linear nor second-order form of T, pH and a w, instead being in more complicated nonlinear form. The superiority of the ANN-based approach is due to high prediction accuracy and the ability to compute combined effects of environmental factors on the thermal inactivation rate of the bacteria.
DOI:10.1007/s12161-013-9643-3URL [本文引用: 1]
DOI:10.1007/s13203-014-0077-7URL [本文引用: 1]

In this study, poly ethyleneimine (PEI)/Titania (TiO 2 ) multilayer film on quartz tubes have been successfully fabricated via a layer-by-layer (LbL) self-assembly method. Scanning electron microscopy (SEM) and Brunauer-Emmett-Teller (BET) surface area analysis were carried out for characterization of the layers on quartz tube. The SEM pictures showed that the film surface is smooth and uniform. The BET characterization verified the formation of multilayer thin film. The photocatalytic activity of the PEI/TiO 2 multilayer deposited on the quartz tubes was evaluated in the treatment of raw petroleum refinery wastewater (PRW) under UV light irradiation in three annular photocatalytic reactors. This study examined the impact of initial chemical oxygen demand (COD) concentration, H 2 O 2 concentration, pH and reaction time on the PRW treatment and the results were used to generate both a response surface methodology (RSM) model and an artificial neural network (ANN) model. Maximum COD removal (9802%) was achieved at the optimum conditions (initial COD concentration of 30002mg/l, hydrogen peroxide concentration of 8.802mM, pH of 5 and reaction time of 12002min). A comparison between the model results and experimental data gave a high correlation coefficient ( R ANN 2 02=020.9632, R RSM 2 02=020.943) and showed that two models were able to predict COD removal from PRW by PEI/TiO 2 /UV process. However, ANN model was superior to RSM model with higher value of coefficient of determination (0.9632 ANN 02>020.94 RSM ) and the lower root mean square error (RMSE) (3.377 AAN 02<023.569 RSM ). The average percentage error for ANN and RSM models was 0.18 and 0.73, respectively, indicating the superiority of ANN in capturing the nonlinear behavior of the system. It was clear that the best networks were able to predict the experimental responses more accurately than the multiple regression analysis.
DOI:10.7506/spkx1002-6630-201714021URL [本文引用: 1]

采用固相微萃取-气相色谱-质谱对市售3种梨酒香气物质进行分离鉴定,共检出43种挥发性成分,其中醇类16种、酯类15种、醛类4种、酮类2种、酚类1种、酸类3种和其他化合物2种。结合香气活度值(odoractivityvalue,OAV)和偏最小二乘回归(partialleastsquaresregression,PLSR)确定梨酒特征香气物质并推断其对梨酒香气的贡献程度。OAV结果表明:梨酒特征香气物质主要为异丁醇、1-辛醇、1-壬醇、苯乙醇、丁酸乙酯、3-甲基丁酸乙酯、乙酸异戊酯、己酸乙酯、辛酸乙酯、β-大马士酮、丁香酚。建立6个感官属性(发酵香、酸香、果香、花香、甜香、清香)与43种香气物质的PLSR模型表明,苯甲醇、正丁醇、丁二酸二乙酯的OAV小于1,但对梨酒的香气有贡献,经OAV确定的梨酒特征香气物质与发酵香和甜香属性具有很好的相关性,而在清香、酸香、果香和花香上的相关性不明显。
DOI:10.7506/spkx1002-6630-201714021URL [本文引用: 1]

采用固相微萃取-气相色谱-质谱对市售3种梨酒香气物质进行分离鉴定,共检出43种挥发性成分,其中醇类16种、酯类15种、醛类4种、酮类2种、酚类1种、酸类3种和其他化合物2种。结合香气活度值(odoractivityvalue,OAV)和偏最小二乘回归(partialleastsquaresregression,PLSR)确定梨酒特征香气物质并推断其对梨酒香气的贡献程度。OAV结果表明:梨酒特征香气物质主要为异丁醇、1-辛醇、1-壬醇、苯乙醇、丁酸乙酯、3-甲基丁酸乙酯、乙酸异戊酯、己酸乙酯、辛酸乙酯、β-大马士酮、丁香酚。建立6个感官属性(发酵香、酸香、果香、花香、甜香、清香)与43种香气物质的PLSR模型表明,苯甲醇、正丁醇、丁二酸二乙酯的OAV小于1,但对梨酒的香气有贡献,经OAV确定的梨酒特征香气物质与发酵香和甜香属性具有很好的相关性,而在清香、酸香、果香和花香上的相关性不明显。
[本文引用: 1]
.
[本文引用: 1]
DOI:10.11975/j.issn.1002-6819.2016.16.019URL [本文引用: 1]

为了进一步提高玉米叶绿素含量的高光谱估算精度,该文测定了西北地区玉米乳熟期叶片的光谱反射率及其对应的叶绿素相对含量(soil and plant analyzer development,SPAD)值,分析了一阶微分光谱、高光谱特征参数与SPAD的相关关系,构建了基于一阶微分光谱、高光谱特征参数和BP神经网络的SPAD估算模型,并对模型进行验证;再结合主成分回归(principal component regression,PCR)、偏最小二乘回归(partial least squares regression,PLSR)以及传统回归模型与BP神经网络模型进行比较。结果表明:SPAD值与一阶微分光谱在763nm处具有最大相关系数(R=0.901);以763 nm处的一阶微分值、蓝边内最大一阶微分为自变量建立的传统回归模型可用于玉米叶片SPAD估算;将构建传统回归模型时筛选到的光谱参数作为输入,实测SPAD值作为输出,构建BP神经网络模型,其建模与验模R2分别为0.887和0.896,RMSE为2.782,RE为4.59%,与其他回归模型相比,BP神经网络模型预测精度最高,研究表明BP神经网络对叶绿素具有较好的预测能力,是估算玉米叶片SPAD值的一种实时高效的方法。
DOI:10.11975/j.issn.1002-6819.2016.16.019URL [本文引用: 1]

为了进一步提高玉米叶绿素含量的高光谱估算精度,该文测定了西北地区玉米乳熟期叶片的光谱反射率及其对应的叶绿素相对含量(soil and plant analyzer development,SPAD)值,分析了一阶微分光谱、高光谱特征参数与SPAD的相关关系,构建了基于一阶微分光谱、高光谱特征参数和BP神经网络的SPAD估算模型,并对模型进行验证;再结合主成分回归(principal component regression,PCR)、偏最小二乘回归(partial least squares regression,PLSR)以及传统回归模型与BP神经网络模型进行比较。结果表明:SPAD值与一阶微分光谱在763nm处具有最大相关系数(R=0.901);以763 nm处的一阶微分值、蓝边内最大一阶微分为自变量建立的传统回归模型可用于玉米叶片SPAD估算;将构建传统回归模型时筛选到的光谱参数作为输入,实测SPAD值作为输出,构建BP神经网络模型,其建模与验模R2分别为0.887和0.896,RMSE为2.782,RE为4.59%,与其他回归模型相比,BP神经网络模型预测精度最高,研究表明BP神经网络对叶绿素具有较好的预测能力,是估算玉米叶片SPAD值的一种实时高效的方法。
DOI:10.1016/B978-0-08-094832-4.50009-XURL [本文引用: 1]

A set of executable equations are defined which model the ideal behavior of a simple genetic algorithm. The equations assume an infinitely large population and require the enumeration of all points in the search space. When implemented as a computer program, the equations can be used to study problems of up to approximately 15 bits. These equations represent an extension of earlier work by Bridges and Goldberg. At the same time these equations are a special case of a model introduced by Vose and Liepins. The various models are reviewed and the predictive behavior of the executable equations is examined. Then the executable equations are extended by quantifying the behavior of a reduced surrogate operator and a uniform crossover operator. In addition, these equations are used to study the computational behavior of a parallel island model implementation of the simple genetic algorithm.
[本文引用: 1]
[本文引用: 1]
DOI:10.1016/j.foodchem.2012.08.048URLPMID:23122097 [本文引用: 1]

Volatiles in Rabdosia serra were investigated by headspace solid phase microextraction (HS-SPME) and simultaneous-distillation extraction (SDE). The HS-SPME technique was previously evaluated to optimise sampling conditions. A total of 56 and 48 compounds including alcohols, aldehydes, hydrocarbons, ketones, carboxylic acid, ester, and aromatics were identified in leaf and stem by optimised HS-SPME method (CAR/PDMS fibre; incubation time, 10 min; extraction temperature, 50 degrees C; extraction time, 40 min), respectively. 1-Octen-3-ol and (2E)-hexenal had significant contribution to R. serra aroma. Cluster analysis indicated that leaf and stem exhibited different volatile diversity. Air drying was favourable for the retention of the volatiles, while freeze- and sun-drying led to the loss of volatiles. SDE method preferred to the analysis of compounds with low volatility including fatty acids and esters. HS-SPME was a useful technique for the analysis of readily volatile components for the characteristics of R. serra aroma. (C) 2012 Elsevier Ltd. All rights reserved.
DOI:10.1016/j.foodchem.2010.11.079URL [本文引用: 2]

The first part of the paper presents the detailed optimisation of all steps of the determination procedure of volatiles from Polish honeys by headspace solid-phase microextraction (HS-SPME) as the sample preparation method and gas chromatography/mass spectrometry (GC S) as the method of final determination. In the result, the following conditions were applied: CAR/PDMS/DVB SPME fibre, the sample composition (5:1w/w honey to water ratio), 3g sample size. The other procedure parameters, like the temperatures of individual stages of the process, were also included. The methodology of semi-quantative analysis allowed the isolation and identification of volatile fraction compounds from popular Polish honeys, rape, acacia, linden, buckwheat, heather, polyfloral and honey-dew. In the effect, it was possible to determine the series of compounds which may be the indicators of varieties and organoleptic quality of Polish honeys (almost 200 compounds were identified, aliphatic and aromatic acids, aldehydes, ketones, alcohols, phenols, terpenoids and derivatives of furan and pyran). Some of the compounds were found for the first time, but a significant number had already been reported in many other papers.
DOI:10.1016/j.microc.2012.10.010URL [本文引用: 1]
DOI:10.1016/j.foodchem.2015.06.100URLPMID:26304314 [本文引用: 1]

A headspace solid phase microextraction (HS-SPME) procedure followed by gas chromatography–flame ionisation detector (GC–FID) analysis was developed and validated for the simultaneous analysis of furan, 2-methylfuran and 2-pentylfuran from juice samples. Extraction at 3202°C for 2002min with stirring at 60002rpm and NaCl concentration 15% (W/V) was the optimal HS-SPME condition for all the three compounds by using a carboxen/polydimethylsiloxane fused silica fibre (7502μm). The extracted compounds were base line separated on a SPB-1 GC column within 1202min. The relative standard deviations of all analytes were less than 6.7%. The recovery rates were between 90.2% and 110.1%. The limits of detection and limits of quantification were 0.056–0.2302ng/mL and 0.14–0.7602ng/mL, respectively. The results showed that the developed method was sensitive, precise, accurate and robust for the determination of furan, 2-methylfuran and 2-pentylfuran in complex matrices without interferences from other components.
DOI:10.3969/j.issn.1004-7360.2006.03.001URL [本文引用: 1]

利用固相微萃取和气质固相微萃取联用技术对赤霞珠干红葡萄酒中的香气成分进行分析,研究了萃取时间、萃取温度、加电解质的量等对红葡萄酒中香气物质萃取的影响,优化了提取条件,建立了快速测定葡萄酒中香气物质的方法。质谱图中共分离出70个峰,利用计算机检索,结合已有的报道鉴定出65种香气物质,根据面积归一法测其相对含量,共占其色谱流出组分总量的98.46%。
.
DOI:10.3969/j.issn.1004-7360.2006.03.001URL [本文引用: 1]

利用固相微萃取和气质固相微萃取联用技术对赤霞珠干红葡萄酒中的香气成分进行分析,研究了萃取时间、萃取温度、加电解质的量等对红葡萄酒中香气物质萃取的影响,优化了提取条件,建立了快速测定葡萄酒中香气物质的方法。质谱图中共分离出70个峰,利用计算机检索,结合已有的报道鉴定出65种香气物质,根据面积归一法测其相对含量,共占其色谱流出组分总量的98.46%。
DOI:10.1016/j.apenergy.2013.04.025URL [本文引用: 1]

For the first time, an efficient simultaneous trans/esterification process for biodiesel synthesis from waste goat tallow with considerable free fatty acids (FFAs) content has been explored employing an infrared radiation assisted reactor (IRAR). The impacts of methanol to tallow molar ratio, IRAR temperature and H2SO4 concentration on goat tallow conversion were evaluated by response surface methodology (RSM). Under optimal conditions, 96.7% FFA conversion was achieved within 2.5h at 59.93wt.% H2SO4, 69.97 C IRAR temperature and 31.88:1 methanol to tallow molar ratio. The experimental results were also modeled using artificial neural network (ANN) and marginal improvement in modeling efficiency was observed in comparison with RSM. The infrared radiation strategy could significantly accelerate the conversion process as demonstrated through a substantial reduction in reaction time compared to conventionally heated reactor while providing appreciably high biodiesel yield. Moreover, the in situ water removal using silica-gel adsorbent could also facilitate achieving higher FFA conversion to fatty acid methyl ester (FAME). Owing to the occurrence of simultaneous transesterification of triglycerides present in goat tallow, overall 98.5wt.% FAME content was determined at optimal conditions in the product biodiesel which conformed to ASTM and EN biodiesel specifications.
URL [本文引用: 1]

采用均匀设计法制定岗松油β-环糊精包合物制备的实验方案,应用人工神经网络对影响包合物制备的因素与考察指标之间的关系进行模型拟合,并结合遗传算法优化包合物的制备工艺参数。优化结果为:环糊精与岗松油的用量配比7.1、包合温度46.6℃、时间149.9 min、搅拌速度417.8 r/min。参照优化后的工艺条件所制备的包合物,含油率和包合率分别为11.89%和89.55%。结果可见,人工神经网络建模与遗传算法寻优相结合,为药物制剂工艺的多维非线性系统的优化提供了有效途径。
URL [本文引用: 1]

采用均匀设计法制定岗松油β-环糊精包合物制备的实验方案,应用人工神经网络对影响包合物制备的因素与考察指标之间的关系进行模型拟合,并结合遗传算法优化包合物的制备工艺参数。优化结果为:环糊精与岗松油的用量配比7.1、包合温度46.6℃、时间149.9 min、搅拌速度417.8 r/min。参照优化后的工艺条件所制备的包合物,含油率和包合率分别为11.89%和89.55%。结果可见,人工神经网络建模与遗传算法寻优相结合,为药物制剂工艺的多维非线性系统的优化提供了有效途径。
DOI:10.1002/(ISSN)1096-9888URL [本文引用: 1]
DOI:10.1111/j.1365-2621.2004.00839.xURL [本文引用: 1]

Summary Solid-phase microextraction (SPME) is a modern, solvent-free sample preparation technique, commonly used in trace analysis. This technique has been developed to combine sampling and sample preparation in one step. This paper reviews selected theoretical and practical aspects of the SPME method used for the isolation and preconcentration of impurities, food constituents, additives and flavour compounds in food samples. The main parameters affecting the extraction effectiveness are discussed and exemplified by selected chromatograms. The review is intended for readers who are either new to the field of SPME or its use in food analysis and many examples of its application for different food matrices are listed.
DOI:10.1016/S1671-2927(06)60139-9URL [本文引用: 1]

Volatile constituents in fully mature fruits of apricot ( Prunus armeniaca L.) cultivar Xinshiji were extracted using headspace solid-phase microextraction (HS-SPME) and simultaneous steam distillation extraction (SSDE) and then analyzed using capillary gas chromatography and gas chromatography-mass spectrometry. A total of 70 components were identified by HS-SPME, including 20 esters, 19 hydrocarbons, 5 alcohols, 5 ketones, 4 acids, 4 lactones, 3 aldehydes, and 10 miscellaneous components, with the esters being the dominant constituent. On the basis of the odor unit values, it is believed that the following compounds probably contributed to the fresh apricot odor: hexyl acetate, β-ionone, butyl acetate, (E)-2-hexcnal, linalool, limonene, γ-decalactone, and hexanal. A total of 49 components were also detected by SSDE, including 13 hydrocarbons, 9 alcohols, 7 aldehydes, 9 esters, 4 ketones, 4 lactones, 2 acids, and 1 miscellaneous component, of which the monoterpene alcohols were the dominant constituents. It could be judged from the odor unit values that the following compounds were the major contributors to boiled apricot aroma: β-ionone, linalool, hexyl acetate, γ-dodecalactone, γ-decalactone, (E)-2-hexenal, hexanal, γ-octalactone, phenylacetaldehyde, butyl acetate, limonene, α-terpineol, and δ-decalactone. The results show that HS-SPME is a simple, rapid, and solvent-free method, which is an alternative to the classical SSDE.
[本文引用: 1]
[本文引用: 1]
DOI:10.4028/www.scientific.net/AMR.1033-1034.762URL [本文引用: 3]

Solid-phase microextraction (SPME) is a rapid sample preparation in volatile analysis. The aromatic volatile compositions of ‘Kuerle’ were analyzed using SPME and gas chromatography-mass spectrometry (GC-MS). The effect of different types of fibers, extraction time, and extraction temperature on the volatile results were compared and discussed. The results were as followed. Results showed that 65μm PDMS/DVB fiber had the best effect of extraction. The optimum factors such as extraction time and temperature were 45 min and 40°C.
[本文引用: 1]
[本文引用: 1]
[本文引用: 1]
[本文引用: 1]
DOI:10.16429/j.1009-7848.2016.12.010URL [本文引用: 1]

本文通过神经网络和响应面法建模,研究温度、pH、氯化钠浓度和硒浓度对预测枯草芽孢杆菌的生长,碱性蛋白酶活和富硒的影响。将径向基(Radial Basis Function,RBF)神经网络和反向传播(Back Propagation,BP)神经网络这两种类型的网络,与响应面法作比较。同时,使用遗传算法优化神经网络的内部结构。通过灵敏度分析发现枯草芽孢杆菌的生物量以及碱性蛋白酶活性的变化与氯化钠浓度的变化密切相关,并且温度的变化也影响碱性蛋白酶的活性,而亚硒酸钠的浓度是影响富硒最重要的参数。通过比较实测值和预测值发现:用BP网络所预测的结果比RBF网络和响应面法更加准确。遗传算法-神经网络方法提供了一个可靠的软件工具来预测枯草芽孢杆菌的富硒过程,为实际的规模化生产奠定理论基础。
DOI:10.16429/j.1009-7848.2016.12.010URL [本文引用: 1]

本文通过神经网络和响应面法建模,研究温度、pH、氯化钠浓度和硒浓度对预测枯草芽孢杆菌的生长,碱性蛋白酶活和富硒的影响。将径向基(Radial Basis Function,RBF)神经网络和反向传播(Back Propagation,BP)神经网络这两种类型的网络,与响应面法作比较。同时,使用遗传算法优化神经网络的内部结构。通过灵敏度分析发现枯草芽孢杆菌的生物量以及碱性蛋白酶活性的变化与氯化钠浓度的变化密切相关,并且温度的变化也影响碱性蛋白酶的活性,而亚硒酸钠的浓度是影响富硒最重要的参数。通过比较实测值和预测值发现:用BP网络所预测的结果比RBF网络和响应面法更加准确。遗传算法-神经网络方法提供了一个可靠的软件工具来预测枯草芽孢杆菌的富硒过程,为实际的规模化生产奠定理论基础。