HTML
--> --> -->An important category of instruments measuring the upwelling microwave radiance from the earth and atmosphere in various frequency bands is the passive microwave (PMW) sounder. Examples of PMW sounding instruments placed on-board presently flying satellites are the Microwave Humidity Sounder (MHS) and the Advanced Microwave Sounding Unit-A (AMSU-A), both on-board the Metop spacecrafts (Saunders, 1993; Bonsignori, 2007; Klaes et al., 2007), the Advanced Technology Microwave Sounder (ATMS) on-board the Joint Polar Satellite System (JPSS) satellites (Goldberg and Weng, 2006), and the Micro-Wave Humidity Sounder-2 (MWHS-2) on-board the Fengyun-3 (FY-3) C and D satellites (Zhang et al., 2019). These PMW instruments are all so-called cross-track scanners and placed on various sun-synchronous polar orbiting satellites, each with different Equator crossing times (ECT), so over a particular area of the globe the data coverage and distribution in time is dependent of the satellite ECTs and the geographical location of the area. The horizontal resolution of individual observations in terms of their instantaneous field of view (IFOV) vary with viewing angle, but is typically around 20–50 km at nadir.
In addition to existing PMW sounding instruments on-board current orbiting satellites, there are instruments planned to be part of future satellite programs. One such example is a small cross-track PMW sounder planned on-board the Arctic Weather Satellite (AWS), a Swedish led initiative① for a prototype satellite under the Earth Watch Programme of the European Space Agency (ESA). The launch for the prototype AWS is planned for the first half of 2024, and is conceived as a demonstrator for a possible follow-on constellation of up to 20 small ( ≈ 120 kg) AWS satellites in various different orbital planes (ESA, 2021). Also a new polar orbiting FY-3 satellite, FY-3E, placed in an early morning orbit and carrying a MWHS-2 instrument, is scheduled for launch in 2021 (Zhang et al., 2019).
Satellite data is an important source of information in NWP DA for accurately describing structures of atmospheric temperature, moisture, wind fields, surface pressure, and clouds. In particular, PMW radiances sensitive to atmospheric temperature and moisture have been demonstrated to be important observation types for global (Li and Liu, 2016; Geer et al., 2017; Lawrence et al., 2018; Carminati et al., 2020; Jiang et al., 2020), as well as for regional NWP (Storto and Randriamampianina, 2010; Schwartz et al., 2012; Xu et al., 2016; Zou et al., 2017). The PMW radiances demonstrated to be useful in global NWP reside from a wide range of instruments, including AMSU-A and MHS (Geer et al., 2017; Lawrence et al., 2018) as well as MWHS-2 (Li and Liu, 2016; Lawrence et al., 2018; Carminati et al., 2020; Jiang et al., 2020). Geer et al. (2017) have reported on a growing impact from humidity sensitive PMW radiances. For regional models the importance of PMW radiances has been demonstrated using various approaches, including an energy-norm based method (Storto and Randriamampianina, 2010), case studies (Li and Liu, 2016; Jiang et al., 2020), and a data denial procedure (Schwartz et al., 2012).
PMW radiances are influenced by clouds and precipitation and until recently have been used for NWP DA only in clear-sky conditions. However, today all-sky PMW radiances are also assimilated by several operational centers (Geer et al., 2018). These improvements have been facilitated by enhanced radiative transfer models and representation of moist and cloud processes in the observation operators and have been obtained in global as well as regional model frameworks. In addition, research is being conducted aiming at an improved use of microwave sounding channels peaking low in the atmosphere and thus more sensitive to surface conditions (Karbou et al., 2005; English, 2008; Frolov et al., 2020). Key challenges are to handle surface emissivities and temperatures in combination with sometimes highly heterogeneous surface properties within the IFOV.
Here, we use a km-scale limited-area NWP system over a northern European domain (see Fig. 1). At high latitudes, radiances from geostationary satellites are in general much less useful than over areas closer to the Equator. This is due to the oblique viewing and larger IFOVs at high latitudes. Radiance observations from sensors on board polar orbiting satellites, on the other hand, are crucial over northern Europe. These observations become more and more frequent with increasing latitude, and can achieve higher horizontal resolution with their lower orbit and more nadir view.

In this paper, the focus is on the current use of PMW radiances and on the effect of an increased use of PMW radiance sounding data by extending the usage to also include radiances from PMW instruments on board the European Metop-C and the Chinese FY-3C and FY-3D satellites. The components of the limited-area forecasting system are the subject of section 2, with special focus on the handling of PMW radiances. In section 3, the experimental design is described, followed by a presentation of results in section 4. Concluding remarks are presented in section 5 together with a future outlook.
2.1. General description
The national meteorological weather services of Sweden, Norway, Finland, and Estonia have joined forces in a collaboration, named MetCoOp, around a common operational km-scale forecasting system (Müller et al., 2017). It is a configuration of the shared Aire Limitée Adaptation dynamique Developpement InterNational (ALADIN)-High Resolution Limited Area Model (HIRLAM) NWP system. This system can be run with different configurations and in MetCoOp the HIRLAM-ALADIN Regional Meso-scale Operational NWP In the Europe Application of Research to Operations at Mesoscale (HARMONIE-AROME) is used (Bengtsson et al., 2017) and is run as an ensemble forecasting system. In our study we use the cy43 version of the MetCoOp forecasting system. The northern European model domain is illustrated in Fig. 1. It has 900
The three main components of this forecasting system are surface DA, upper-air DA, and the forecast model. The system is run with a 3-hourly assimilation cycle and launching forecasts at 0000, 0300, 0600, 0900, 1200, 1500, 1800, and 2100 UTC. Only the forecasts launched at the synoptic hours 0000, 0600, 1200, and 1800 UTC are being used by the duty forecasters. Therefore, these have a relatively strict observation cut-off time of 1 h and 15 min compared to the 3 h and 20 min for the asynoptic cycles 0300, 0900, 1500, and 2100 UTC. For the asynoptic cycles, the only time-constraint is to produce a 3 h forecasts serving as background state for the DA at the following synoptic cycle. Thus, this 3 h forecast can be produced just prior to the synoptic cycle DA. Due to the operational cut-off constraints mentioned above, in practice only observations within a time-range from ?1 h and 30 min to +1 h and 15 min are used for the cycles at 0000, 0600, 1200, and 18 UTC. For the asynoptic cycles observations within the entire time-range ?1 h and 30 min to + 1 h and 29 min are used.
A detailed description of the forecast model setup is given in Seity et al. (2011) and Bengtsson et al. (2017). It is a non-hydrostatic model formulation with a spectral representation of the model state (Bubnová et al., 1995; Bénard et al., 2010). Sub-grid scale parameterization of clouds, including shallow convection is handled by the EDMF (Eddy Diffusitivity Mass Flux) originating from de Rooy and Siebesma (2008) and Neggers et al. (2009). Deep convection is resolved by the model. Turbulence and vertical diffusion is represented using the so-called HARATU scheme, which is based on a Turbulent Kinetic Energy scheme by Lenderink and Holtslag (2004). The radiative transfer is modelled as described by Fouquart and Bonnel (1980) and Mlawer et al. (1997) for short- and long-wave radiation processes, respectively. Surface processes are modeled using the SURFEX (Surface Externalisée) scheme (Masson et al., 2013). Global forecasts provided by the European Centre for Medium-Range Weather Forecasts (ECMWF) are used as lateral boundary conditions. These forecasts are launched every 6 h with a 1 h output frequency. Global model information is also used to replace larger-scale information in the background state with lateral boundary information (Müller et al., 2017). The idea is to make use of high-quality large-scale information from the ECMWF global fields, in the MetCoOp analysis.
In the surface DA synoptic observations of two-meter temperature, two-meter relative humidity and snow cover are used to estimate the initial state of the soil temperature, soil moisture, and snow field. The DA is comprised of a horizontal optimal interpolation (Taillefer, 2002), which for soil moisture and temperature is followed by a vertical optimal interpolation procedure (Giard and Bazile, 2000). The upper-air DA is based on a 3-dimensional variational (3D-Var) approach (Fischer et al., 2005). Many types of observations are assimilated including conventional in-situ measurements (pilot–balloon wind, radiosonde, aircraft, buoy, ship, and synop), Global Navigation Satellite System (GNSS) Zenith Total Delay (ZTD) data, weather radar reflectivity information, as well as infrared (IR) and PMW radiances from satellite-based instruments. The IR radiances are sensed by the Infrared Atmospheric Sounding Interferometer (IASI) placed on board the Metop satellites. PMW radiances are traditionally provided by the Advanced TIROS Operational Vertical Sounder (ATOVS) instrument family, including AMSU-A and MHS. Recently the DA system has been prepared to also utilize data from the MWHS-2 instrument on board the FY-3C and FY-3D polar orbiting satellites. Background error covariances are based on a climatological assumption and their representation is based on a multivariate formulation under the assumptions of horizontal homogeneity and isotrophy. They are calculated from an ensemble of forecast differences (Berre, 2000; Brousseau et al., 2012). These are produced by Ensemble Data Assimilation (EDA) experiments carried out with the HARMONIE-AROME system. The HARMONIE-AROME EDA uses perturbed observations and ECMWF global EDA (Bonavita et al., 2012) forecasts as lateral boundary conditions. Scaling is applied to the derived statistics in order to be in agreement with the amplitude of HARMONIE-AROME + 3 h forecast errors (Brousseau et al., 2012).
2
2.2. Handling of microwave radiances
The NWP system uses atmospheric temperature and moisture information extracted from the PMW radiances sensed by satellite instruments summarized in Table 1. The AMSU-A instrument is primarily used to retrieve information on the vertical distribution of atmospheric temperature. The MHS instrument, on the other hand, provides information on the vertical structure of water vapour. Similarly to MHS, the MWHS-2 instrument has capability to retrieve information on atmospheric moisture. In addition, it has some capacity to retrieve information on temperature. To produce the model counterparts of the observed PMW radiances an observation operator,





Instrument | Satellites |
AMSU-A | Metop-A, Metop-B, Metop-C, NOAA-18, NOAA-19 |
MHS | Metop-A, Metop-B, Metop-C, NOAA-19 |
MWHS-2 | FY-3C, FY-3D |
Table1. PMW radiance observation usage.
Channel number | Channel Frequency (GHz) | |||||
AMSU-A | MWHS-2 | MHS | AMSU-A | MWHS-2 | MHS | |
6 | ? | ? | 54.400 (H) | ? | ? | |
7 | ? | ? | 54.940 (V) | ? | ? | |
8 | ? | ? | 55.500 (H) | ? | ? | |
9 | ? | ? | 57.290344 (H) | ? | ? | |
? | 5 | ? | ? | 118.75 ± 0.8 (H) | ? | |
? | 6 | ? | ? | 118.75 ± 1.1 (H) | ? | |
? | 11 | 3 | ? | 183 ± 1.0 (H) | 183 ± 1.0 (H) | |
? | 12 | ? | ? | 183 ± 1.8 (H) | ? | |
? | 13 | 4 | ? | 183 ± 3.0 (H) | 183 ± 3.0 (H) | |
? | 14 | ? | ? | 183 ± 4.5 (H) | ? | |
? | 15 | 5 | ? | 183 ± 7.0 (H) | 190.31 (V) |
Table2. PMW radiance channels used in the DA (window channels used in the data filtering are not included).
To indicate whether radiance data from particular instruments and channels are affected by clouds, radiances in associated window channels within the instrument, capable of identifying clouds, are compared with the corresponding model state equivalents. If the window channel departures are larger than a particular threshold value, the corresponding non-window channel radiances are considered to be affected by clouds, and are therefore rejected from use in the DA. The window channel for AMSU-A channels 6 and 7 is AMSU-A channel 4 (




Systematic errors that might be present in the clear-sky radiances that have passed the cloud detection are handled by applying an adaptive Variational Bias Correction (VARBC) as proposed by Dee (2005) and further adapted by Auligné et al. (2007). A linear model of the following form is applied to describe the bias

Here




A quality control procedure is applied to remove radiance observations that are considered to be of poor quality. Based on the operational monitoring experiences, we do not use AMSU-A and MHS radiances from field of views close to scan-line edges. Channels from instruments on specific satellite platforms might be temporarily or permanently blacklisted in the DA system. This blacklisting may occur due to known problems reported by the satellite agencies (NOAA-18 MHS channels 3-5, NOAA-19 MHS channel 3), by other collaborating NWP partners assimilating the same satellite radiances (NOAA-19 AMSU-A channels 7–8 and Metop-B channel 7), or due to quality limitations found during observation monitoring (i.e., where observed radiances are compared with corresponding model state equivalents over a longer period). It should be mentioned that the Metop-B AMSU-A channel 7 blacklisting is based on experiences with noisy radiances starting already in 2017. However, this noise is not presently observed, and the plan is to re-activate the assimilation of this channel. Further, as part of the quality control, a filtering is applied to get rid of radiance observations affected by gross errors. In this gross error check, a radiance observation,

where






The bias corrected radiances that have passed the data selection and quality control are assumed to have a Gaussian error distribution. The associated observation error standard deviations are derived from long-term observation monitoring. The observation errors are comprised of instrument errors, representativity errors, persistence errors, and errors in the observation operator, and they are slightly inflated to account for the lack of representation of observation error correlations (Bormann and Bauer, 2010). For the AMSU-A channels, the estimated error standard deviations are approximately






The rejections in the data selection and quality control are dominated by the cloud detection and the thinning procedure. Roughly 3% of the observations that have passed the data selection (except thinning, since it is applied after the quality control) are identified as gross errors and rejected by the gross error check described by Eq. 2.


The parallel experiments REF and SAT were performed for a period extending from 21 September 2019 to 27 October 2019. During this period, forecasts up to a range of 36 h were launched four times a day, at 0000, 0600, 1200 and 1800 UTC. The first six days were excluded from the verification, giving some time for systems to do potential minor adjustments to the VARBC predictor coefficients, which was particularly important regarding the SAT experiment due to active assimilation of the newly introduced additional PMW radiances.
4.1. Data coverage
The REF satellite-based PMW radiance observation usage results in a rather uneven distribution of microwave observations between the assimilation cycles. Some cycles are, not at all, or only by a small fraction, covered by PMW satellite radiances. This is illustrated by the left part of Fig. 2, which shows, for REF, the fraction of the model domain covered by satellite-based PMW radiances, assuming a 20 min latency (or timeliness, time from observation is made until being available for use in model), which is considered realistic. The right part of Fig. 2 shows the corresponding fractions covered for SAT experiment, when satellite-based PMW radiances from Metop-C, FY-3C, and FY-3D are also being used. With these additional PMW observations included there is a more even distribution, between different assimilation cycles, of the fraction of the area covered by PMW radiances. In SAT, almost 80% of the domain is covered by PMW radiance observations for all assimilation cycles. In particular, for the 0000 UTC assimilation cycle, a large part of the domain is covered by PMW data in SAT, whereas there are no PMW observations at all in REF. The additional PMW satellite radiances thus have the potential to improve forecast quality by filling existing data gaps.
2
4.2. Observation monitoring
One way to evaluate the quality of the observations in the DA is to compare the observed values with the corresponding model state equivalents. This was done for all the PMW instruments and channels used. Results are shown for AMSU-A in Fig. 3, MHS in Fig. 4, and MWHS-2 in Fig. 5. Channels for which statistics are lacking are permanently rejected by our DA system since they are considered to be of poor quality. Note that the innovation (observation minus background equivalent departure) statistics do basically have a Gaussian distribution so that the standard deviation of the innovations can be estimated from where the distribution has dropped to roughly one third of its maximum value. For unbiased data with observation errors uncorrelated with background errors, the square of the innovation standard deviations for a particular channel is further built up by the sum of the square of the observation error standard deviation and the square of the standard deviation of the error for the background observation equivalent. Taking into account the slight inflation of the observation error standard deviations, to compensate for the lack of representation of observation error correlations, the width of the innovations are consistent with the estimated observation error standard deviations (Figs. 3, 4 and 5). Innovations are smaller for temperature sensitive channels (Fig. 3) than for moisture sensitive channels (Figs. 4 and 5). Statistics from AMSU-A on Metop satellites look very similar to that of AMSU-A statistics from NOAA-18 and NOAA-19 satellites (not shown). For MWHS-2, only the moisture sensitive channels are shown. From Fig. 3 it is clear that the newly introduced Metop-C AMSU-A radiances are of at least similar quality as the corresponding AMSU-A measurements from instruments on-board the other Metop satellites already used in the system.

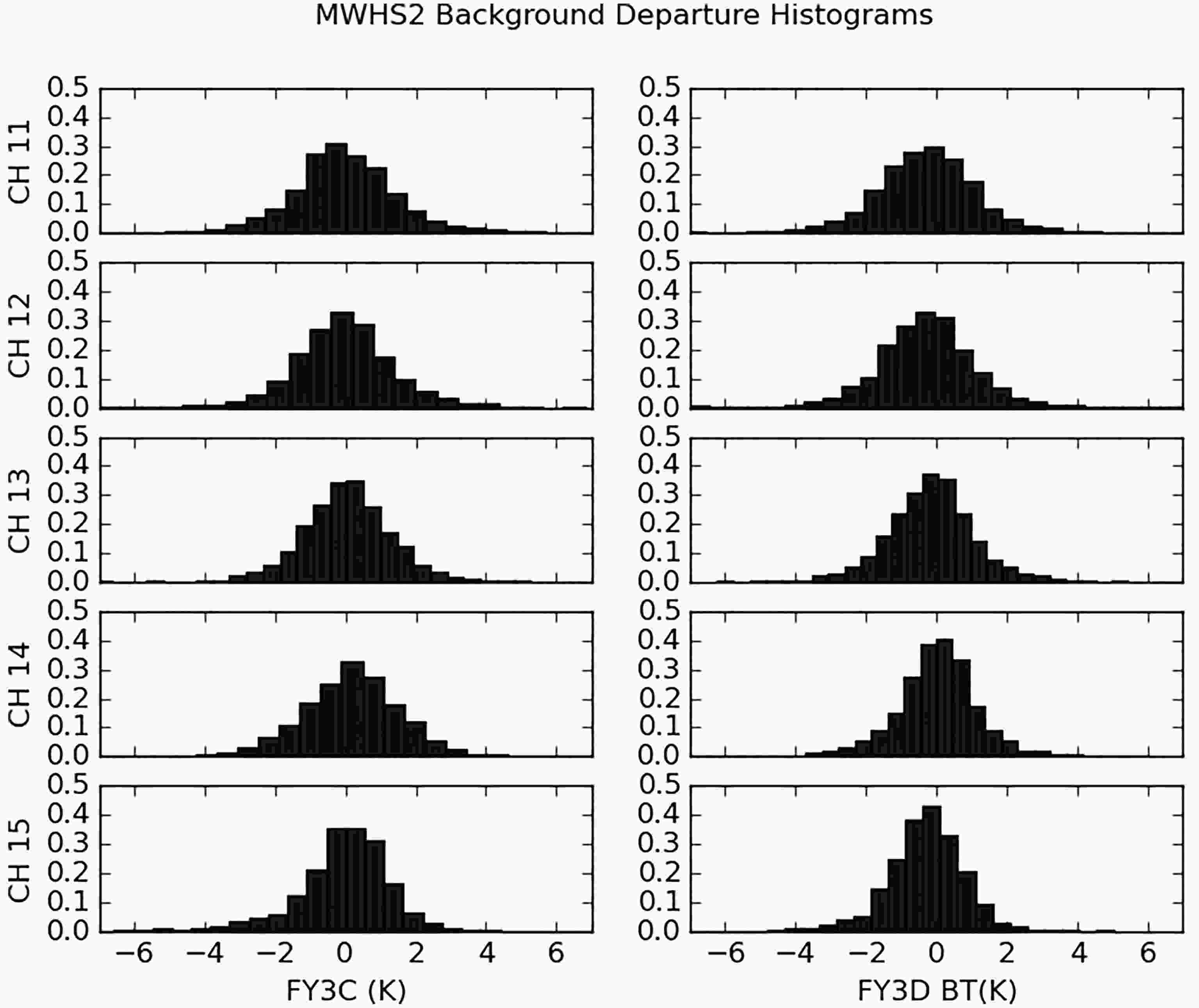
The MHS Metop-C channel 3 and 4 radiances are of slightly worse quality than the corresponding radiances from the other Metop satellites and NOAA-19. It is clear from the width of the histograms that the observation error standard deviations for Metop-C MHS channels 3 and 4 should be increased by a few tenths of a Kelvin in the future due to larger instrument errors. As a starting point, however, the same observation error standard deviations are applied for Metop-C as for the other satellites. The MHS channel 5 distributions are skewed for all satellites. The reason is that only observations over sea are assimilated, but close to the coast, they are likely influenced by land surface, which causes an undesirable deviation between observation and model counterpart in monitoring and DA. This will be improved by refined data selection in future versions of the system, taking the satellite footprint into account. The MWHS-2 instrument channels 11?15 are demonstrated to be of a similar quality to those of MHS. Again, skewed distributions appear for channel 15, for the same reason as for MHS channel 5.
2
4.3. Observation impact on analyses
The impact of observations on the analysis system can be evaluated using the degrees of freedom for signal (DFS) (Randriamampianina et al., 2011). DFS is the derivative of the analysis increments in observation space with respect to the observations used in the analysis system. As proposed by Chapnik et al. (2006), DFS can be computed through a randomization technique, as follows:Here,







The DFS values vary depending on the assimilation cycles due to differences in observation coverage and also from day to day due to variations in the meteorological situation. We calculate the DFS subdivided into various observation types based on data from the three selected dates 17, 20, and 24 October 2019. The days are separated well in time to provide independent weather situations. The DFS was calculated for each type of observation assimilated in REF and SAT. The calculation was done based on all eight assimilation cycles within each of the three days, as presented in Fig. 6. In terms of absolute DFS, the contribution from satellite PMW radiances has clearly increased in SAT as compared to REF. The satellite PMW radiances can be seen to be the fourth most influential observation category on the analysis, after radar reflectivities, satellite IR radiances, and aircraft reports. One should keep in mind that at 0000 UTC there are very few aircraft observations available and no Metop satellite overpasses, and hence, no IR satellite data in neither of the parallel experiments. Satellite PMW data at 0000 UTC are available only in SAT, and together with radiosondes and radar reflectivities are the largest contribution to absolute DFS (not shown). Thus, there is an enhanced effect on the initial state of the additional observations, in particular at 0000 UTC. By including the additional PMW observations, the DFS of almost all the other observation types increases, indicating a consistency between the additional PMW radiances and the other observation types. It seems that the additional PMW observations also contribute by increasing the impact on the analysis of the other observation types. The exception is satellite IR radiances, for which the DFS slightly decreases when including additional PMW radiances. One potential explanation for the reduced impact of IR radiances when introducing additional PMW radiances is interactions through VARBC in combination with a relatively small number of anchoring observations. The reduction in DFS for IR radiances might also indicate some kind of deficiency in the handling of IR radiances, such as sub-optimal cloud-detection procedures. This is something that will be studied in more detail in the future.
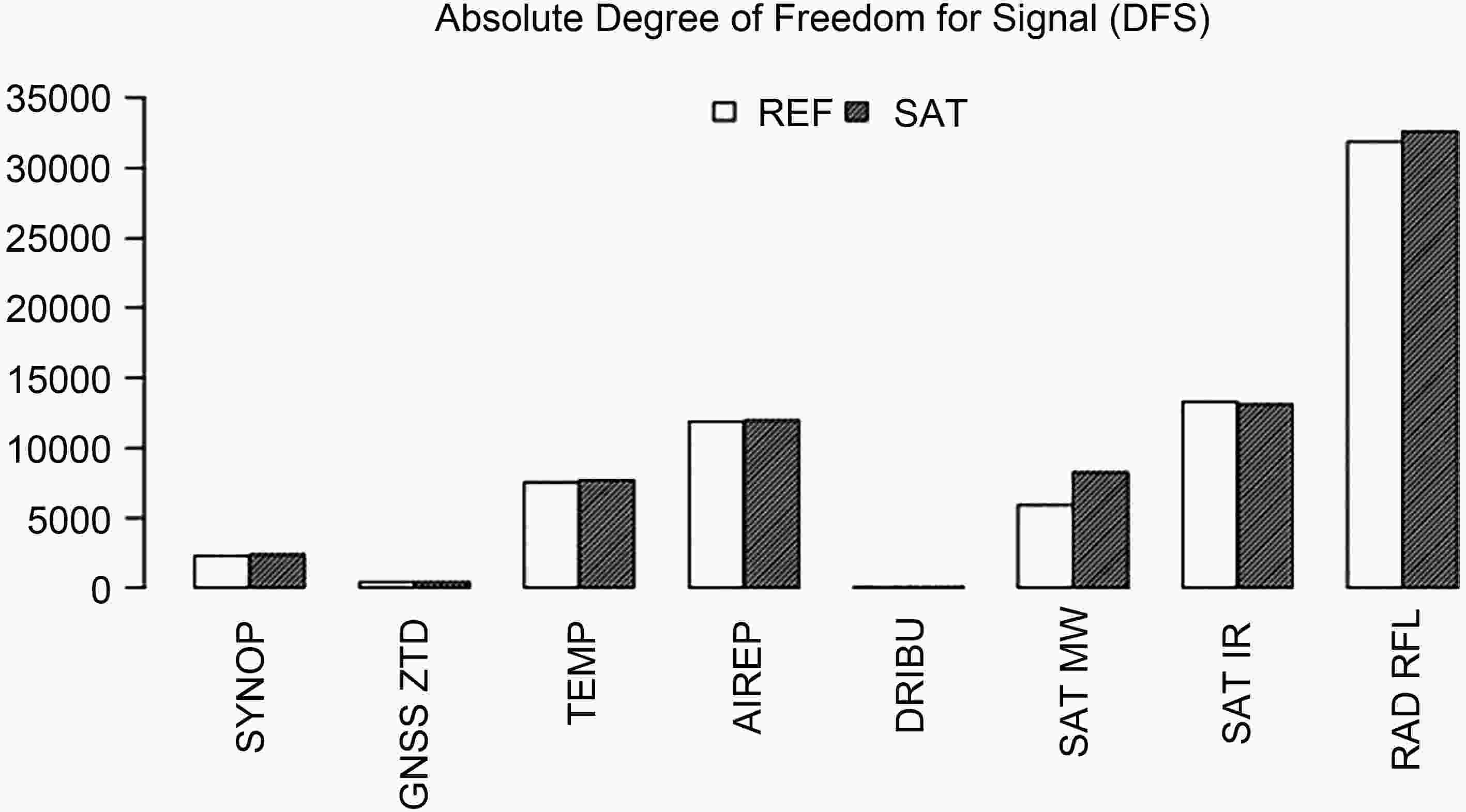
2
4.4. Observation impact on the forecasts
To evaluate the quality of the forecasts from the parallel experiment, we verified them against radiosonde and synoptic weather observations within the model domain. The verification was carried out for surface and upper-air model variables. Special emphasis was put on verification of humidity, clouds, precipitation, and temperature. Results revealed that the largest differences in verification statistics between the two parallel experiments were found for forecast ranges up to +18 h. In Fig. 7 the verification statistics for +12 h temperature and specific humidity forecasts are shown as a function of vertical level, for verification against radiosondes.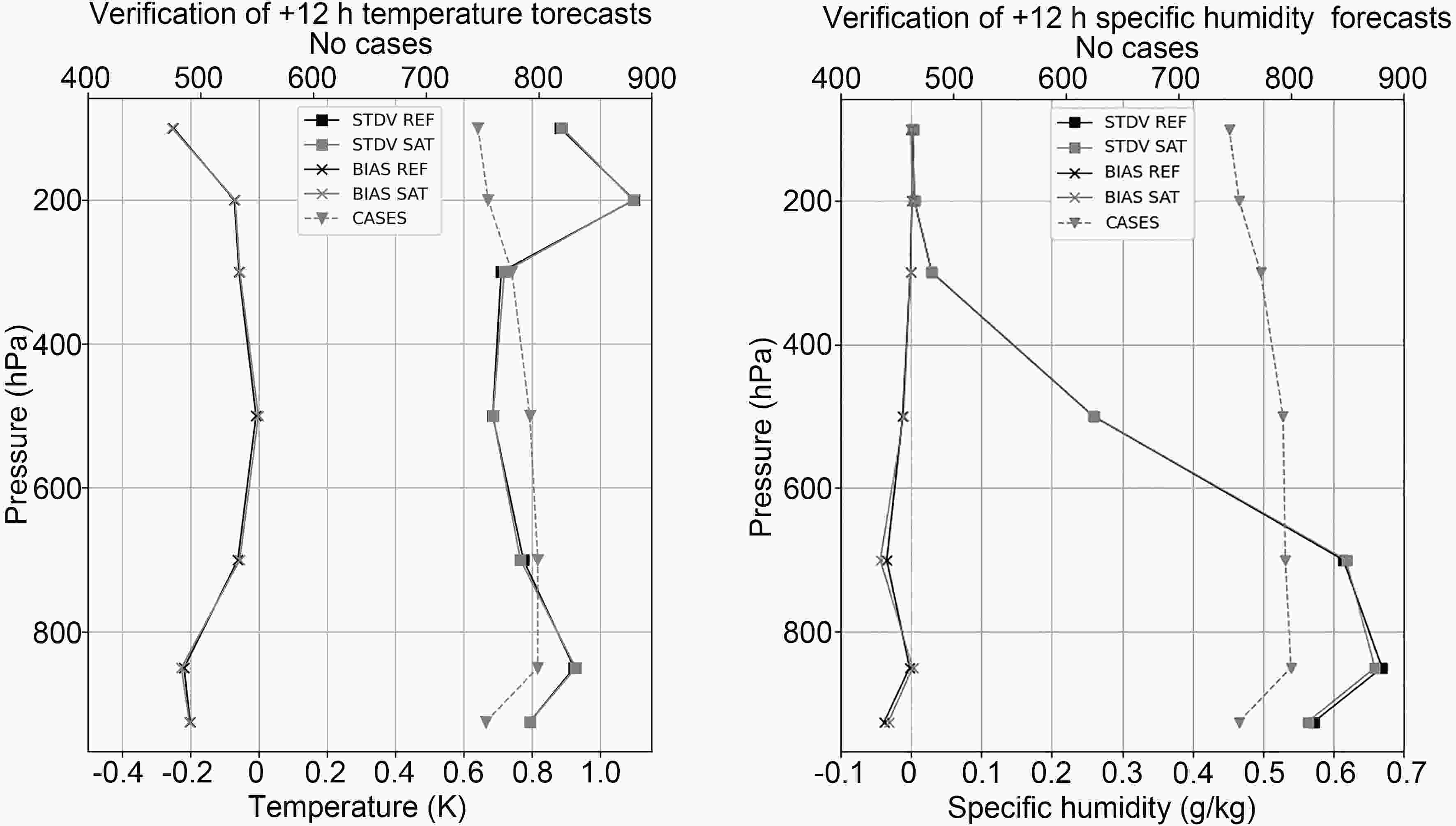
In terms of both bias and standard deviation, the temperature forecasts of SAT and REF are of a similar quality. On the other hand, with respect to standard deviations below 700 hPa, SAT forecasts are better than REF forecasts. Above 700 hPa humidity forecast quality is rather similar for SAT and REF, in terms of bias as well as standard deviation. The largest impact on low-level humidity forecasts can be explained by most of the humidity sensitive Jacobians peaking between 600 and 800 hPa in combination with background error humidity standard deviation profiles (not shown) having the largest values between roughly 700 and 900 hPa. At 700 hPa the bias is negative for both SAT and REF but the magnitude (absolute value) is larger for SAT than for REF. Thus, compared to radiosonde observations, both SAT and REF are slightly drier around 700 hPa, and SAT is the driest. One reason for the slightly more dry SAT forecast as compared to REF could be the too high model background equivalents close to coastlines for low-peaking moisture sensitive channels (Figs. 4 and 5), causing negative moisture increments. However, the magnitude of the bias actually seems to be smaller for SAT below the 800 hPa level.
Verification scores for forecasts of +12 h total cloud cover and 12 h accumulated precipitation between +6 h and +18 h are better for SAT than for REF, as shown in Fig. 8, in terms of Kuiper skill score, when verifying against synop land weather stations in the domain over the one month period. Both the cloud cover and accumulated precipitation forecasts are slightly better for SAT than for REF for almost all thresholds. These results are consistent for cloud and precipitation and with better low-level specific humidity forecasts in SAT as compared to REF.

Although low-level SAT moisture forecasts are better on average than REF forecasts during the month period, it should be noted that there is a considerable variation in verification scores between different days. In addition, one can identify some differences between forecasts launched from 0000 and 1200 UTC. Figure 9 illustrates a clear daily variation for +12 h 925 hPa relative humidity forecasts launched from 00 UTC (left) and 12 UTC (right). In terms of magnitude of standard deviations for forecasts launched at 00 UTC, SAT performs better than REF in 63% of the cases. For forecasts launched at 12 UTC, SAT forecasts are better than REF forecasts in 56% of the cases. The improved SAT forecast as compared with REF is particularly evident for forecasts launched at 00 UTC and with valid times between 17 and 24 October. One contributing factor for this difference between 00 and 12 UTC based forecasts is likely that at 00 UTC there are no humidity sensitive PMW observations assimilated in REF while there is a substantial amount of such observations available for assimilation in SAT (recall Fig. 2).

2
4.5. Case study
As an example of impact of assimilation of the additional PMW observations on forecast quality, one particular case has been selected. We illustrate in Fig. 10 the precipitation forecasts launched from 0000 UTC 18 October 2019 and valid at 19 October between 0000 and 0300 UTC. The forecasts are for 3 h accumulated precipitation (unit: mm). For comparison, also the corresponding weather radar derived 3 h accumulated precipitation is shown.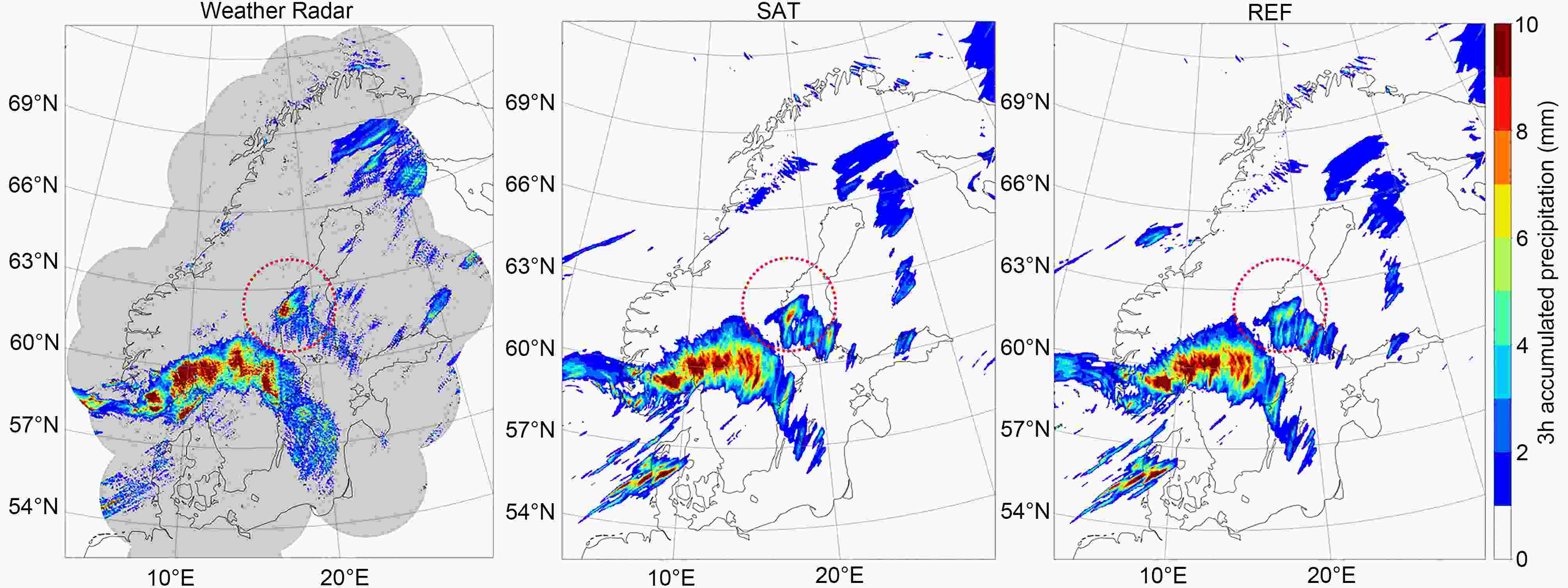
The weather situation is characterised by a synoptic scale cyclone, with associated frontal systems, over the southern part of the model domain and moving towards north-east. The event resulted in substantial precipitation amounts in the southern parts of Norway as well as in southern and central parts of Sweden. The main precipitation pattern was rather well predicted, both in terms of amount and pattern. In front of this main precipitation pattern there are also two other frontal structures, accompanied by precipitation. One of these is situated close to the eastern coast and over the Baltic sea in the middle part of Sweden, and one is situated over the northern parts of Sweden and Finland. Interestingly, the substantial precipitation amounts close to the coast in eastern Sweden to the north-east of the main frontal structure (and marked with a red dotted circle in Fig. 10) are better predicted with the SAT-based forecast than with the REF-based forecast. It can be argued that the exact position of such small-scale precipitation structures is in general not predictable at a forecast range of 24 h. Nevertheless, the assimilation of additional PMW radiances in SAT as compared to REF might have provided improved lower-level atmospheric moisture fields, enabling improved precipitation forecasts.
Extending the regional forecasting system with assimilation of MWHS-2 microwave radiance observations as well as Metop-C MHS and AMSU-A radiances was demonstrated to be beneficial in different ways. It was shown that the newly introduced observations fill the lack of PMW satellite data over the northern European domain during midnight and early morning, which leads to a more even availability of PMW radiances in DA over the course of the day. Observation monitoring results for humidity sensitive PMW channels point out that the quality of the MWHS-2 is comparable to the already assimilated MHS instruments, on board the NOAA-19, Metop-A, and Metop-B satellites. On the other hand, MHS channels 3 and 4 on Metop-C seem to be of slightly worse quality than the corresponding MHS observations from other satellites already used in operations. Through the DFS diagnostic we have shown that the additional PMW radiances also had a clear impact on the analyses. Finally, it was demonstrated through verification scores that the additional observations do have a positive impact on the short-range forecasts of humidity, clouds, and precipitation, in a statistical sense. The potential impact of the additional microwave radiances was demonstrated through a case study where an example of prediction of precipitation was discussed. Based on the work and findings presented here, radiances from MHS and AMSU-A from Metop-C as well as MWHS-2 on board FY-3C and FY-3D are now assimilated in a pre-operational version of the regional forecasting system.
Despite the encouraging results obtained, there is room for further improvements regarding the handling of satellite-based humidity-sensitive PMW observations in our regional NWP model. As a first step we plan to investigate the horizontal correlations of observation errors for the MWHS-2 radiances using a posteriori diagnosis of observation error correlations following Bormann and Bauer (2010). From such a study, we hope to justify the application of a shorter horizontal thinning distance than the currently used 160 km for MWHS-2. The thinning procedures, when rejecting radiances affected by land surface from low-peaking humidity sensitive channels, needs to be improved to better take the actual satellite footprint into account. In the long term, important future improvements are the assimilation of all-sky radiances and introduction of flow-dependent data assimilation techniques, such as 4D-Var (Gustafsson et al., 2012) or a hybrid ensemble-variational data assimilation (Gustafsson et al., 2014).
Within MetCoOp, the researchers in regional data assimilation are working towards the introduction of an NWP based nowcasting system, with data assimilation carried out each hour and with a 15 min observation cut-off time. Figure 11 shows the PMW radiance observation coverage and availability for such a nowcasting system. Clearly the extended observation usage will also benefit such a system, but there is still room for enhancements regarding the availability of PMW data. A system of many small polar satellites equipped with a PMW instrument would be sufficient for filling these many remaining gaps in a cost-effective way. The AWS concept has been born out of exactly such a need. The prototype AWS is expected to be launched in early 2024, and a follow-on constellation, of up to 20 small satellites providing observational coverage over the Arctic every 30 min, is considered for 2025 and onwards.
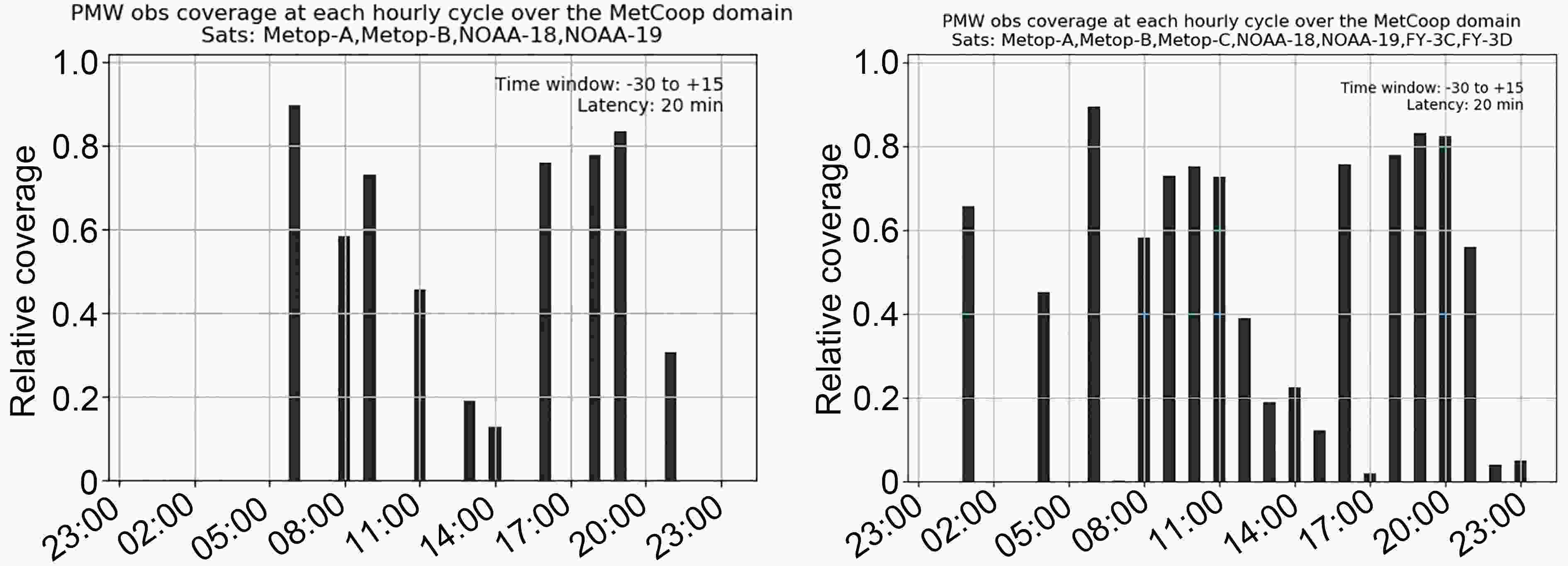
Acknowledgements. The work has been carried out within MetCoOp. We acknowledge Philippe Chambon for support and discussions regarding handling of PMW radiances in HARMONIE-AROME. We are grateful for technical assistance from Eoin WHELAN, Frank GUILLAUME, Ulf ANDR?, and Ole VIGNES. We are grateful to Susanna HAGELIN for support on improving the readability of the manuscript. We also thank the anonymous reviewers for useful comments.
Funding: Open access funding provided by Norwegian Meteorological Institute.
Open Access This article is distributed under the terms of the Creative Commons Attribution 4.0 International License (