

2Nonlinear Systems and Applications, Faculty of Electrical and Electronics Engineering,
3Department of Biomedical Engineering,
4Health Technology Research Institute,
5Department of Mathematics, Statistics and Physics,
Received:2020-03-01Revised:2020-06-29Accepted:2020-06-30Online:2020-10-02

Abstract
Keywords:
PDF (2193KB)MetadataMetricsRelated articlesExportEndNote|Ris|BibtexFavorite
Cite this article
Biqun Chen, Karthikeyan Rajagopal, Fatemeh Parastesh, Hamed Azarnoush, Sajad Jafari, Iqtadar Hussain. Observation of chimera patterns in a network of symmetric chaotic finance systems. Communications in Theoretical Physics, 2020, 72(10): 105003- doi:10.1088/1572-9494/aba261
1. Introduction
In 1986 Grandmont and Malgrange [1] discovered the chaotic behavior in economics. After that many nonlinear continuous models have been proposed for explaining the complex economical dynamics, such as the forced van der Pol model [2], the IS-LM model [3] and the Kaldorian model [4]. Financial system has some factors including interest rate, the price of goods, investment demand and stock, which make its behavior nonlinear and complex. Therefore, chaotic behavior can occur in financial systems, especially during the economic crisis [5, 6].In recent years, methods of statistical physics have been applied successfully and prolifically to study various aspects of social and financial systems [7, 8]. Furthermore, financial systems are well-known to be complex systems [9]. Complex systems which usually consist of large numbers of agents, are time-varying and have interactions in transferring the information. The economic systems are unceasingly influenced by the environment, such as the political system, the stability of the society and international relationships. On the other hand, an economic agent interacts with other agents either in the vicinity like local shops, or distant parties through intermediaries [10]. Motivated by these concepts and after the 2008 crisis, there has been growing attention in using complexity theory notions like tipping points, contagion and networks in financial researches [11].
In this paper, we consider a network of newly presented 3D nonlinear finance chaotic system in order to study the synchronization patterns especially chimera state which has not been studied earlier. Chimera state is a self-organized spatiotemporal pattern displaying coexistence of synchronized and asynchronized regions [12-14]. Starting from the pioneering work of Kuramoto and Battogtokh [15] that discovered this phenomenon in non-locally coupled phase oscillators, the chimera state has attracted many interests in different fields [16-20]. Kapitaniak et al [21] showed chimeras in a network of coupled pendulum like oscillators and also experimentally in mechanical oscillators named Huygens clock. Larger et al [22] reported chimera patterns experimentally in delayed feedback optoelectronic systems. Chimeras have also been investigated in various neuronal networks such as coupled FitzHugh-Nagumo oscillators and HindMarsh-Rose models with different coupling schemes and connection topologies [23-29]. Very recently, Majhi et al [30] presented a comprehensive review of the studies of chimera states in neuronal networks.
For our investigation, we use a finance system which has symmetric coexisting chaotic attractors, as the blocks of the network. The parameters of the coupling are changed and the spatiotemporal patterns and the oscillators' attractors are considered. In the following section, the mathematical model of the system and the coupled network is described. Section
2. Mathematical model
In 2001 Ma and Chen [31] introduced a nonlinear finance chaotic system as:The variables are described as the main system. The new system is rotation symmetric around the y-axis, since it is invariant under the change of coordinates $\left(x,y,z\right)\,\to \,\left(-x,y,-z\right).$ For different values of parameters, the system shows different periodic and chaotic behaviors. Here, we choose the parameters as $a\,=\,1,$ $b\,=\,0.15$ and $c\,=\,1,$ in which the system has chaotic behavior. Figure 1 shows the symmetric coexisting attractors of the system in different planes.
Figure 1.
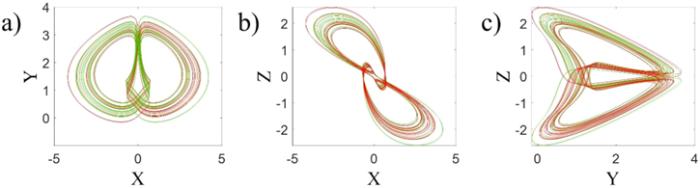
Figure 1.The symmetric coexisting attractors of the system equation (
To construct the network, we couple $N$ numbers of the above systems non-locally by using the nearest neighbor method. It is supposed that the interaction is through the price index variables, so the coupling is made on the z variables. Therefore, the equations of the network can be described as:
3. The results
In order to solve the network equations, we use the fourth order Runge-Kutta method with time step $0.01.$ The network size is fixed at $N\,=\,100$ and all the initial conditions are chosen randomly in the interval $x,\,y,\,z\,\in \,\left[-10,\,10\right].$ It should be noted that multiple sets of initial conditions have been considered and the similar results were obtained. The property of coherence and incoherence in the network can be established by using the real-valued local order parameter [34]3.1. The effect of coupling strength variation
For very small coupling strength with $r\,=\,0.4,$ the network is incoherent. When coupling strength gets higher than $0.005,$ chimera states appear. Figure 2 shows the spatiotemporal patterns and local order parameters for small coupling strengths. By increasing the coupling strength, firstly chimeras are non-stationary and unstable as can be seen in figures 2(a), (b) for $d\,=\,0.006$ and $d\,=\,0.008.$ Then the chimeras become stable, which is shown in figure 2(c) for $d\,=\,0.01.$ But, further increase of coupling strength makes the synchronous clusters to be narrower and thus the network becomes asynchronous at $d\,\gt \,0.02$ (figure 2(d)). For the small values of the coupling strength ($d$), the symmetric coexisting attractors of the single system are not seen any more. In this case, some of the coupled oscillators have one symmetric periodic attractor and some of them have one symmetric chaotic attractor, as shown in figure 3.Figure 2.
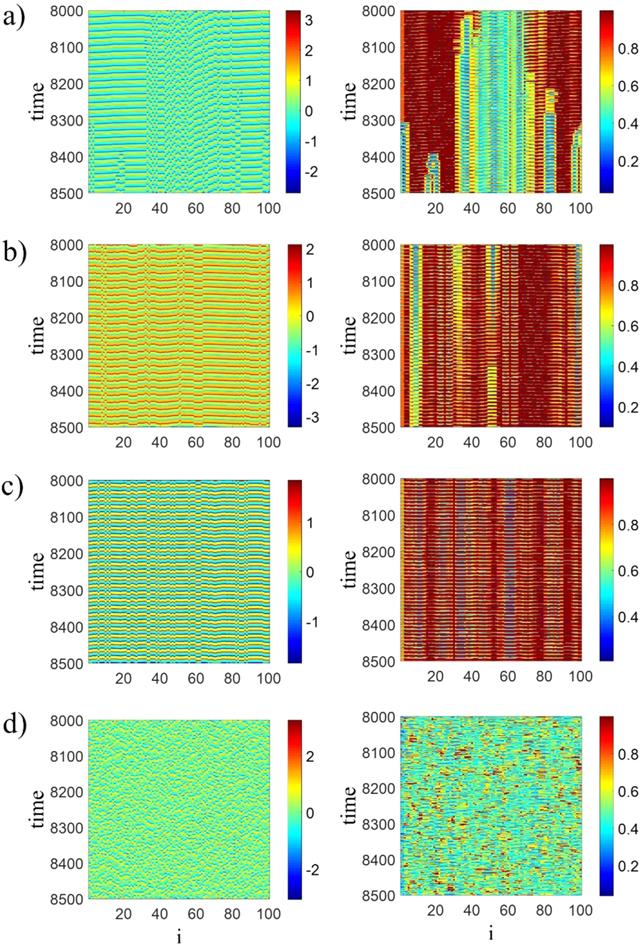
Figure 2.Spatiotemporal patterns (left panel) and local order parameters (right panel) of the network for $r\,=\,0.4,$ (a) non-stationary chimera at $d\,=\,0.006,$ (b) chimera at $d\,=\,0.008,$ (c) chimera at $d\,=\,0.01,$ (d) asynchronization at $d\,=\,0.03.$ The initial conditions are randomly selected in the interval, $y,\,z\,\in \,\left[-10,\,10\right].$ It is observed that for lower coupling strengths, the emerged chimera state is non-stationary, while increasing the coupling strength changes it to stationary one. However, further increasing of $d$ results in asynchronization.
Figure 3.
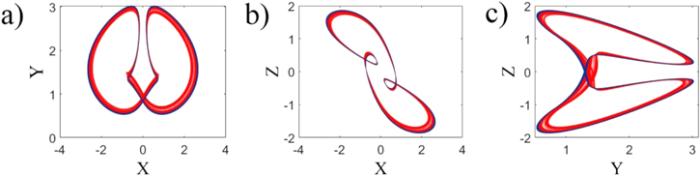
Figure 3.The symmetric attractors of the coupled oscillators (red: chaotic, blue: periodic). (a) x-y plane, (b) x-z plane and (c) y-z plane. The attractors of the coupled oscillators are different from the attractors of single oscillator (shown in figure
The asynchronous state continues until the coupling strength reaches to $d\,=\,0.77.$ Then the multi-cluster chimeras start to emerge, which seem very regular. Figure 4(a) shows the network pattern for $d\,=\,0.77,$ at which some synchronous regions are formed. In this case, the asynchronous oscillators have the same attractor as the single oscillator (shown in figure 1). While, the oscillators in the synchronous clusters have different attractors. The attractors of the synchronized oscillators (blue and green attractors) and one asynchronous oscillator (yellow attractor) are shown in figure 5. It should be noted that the attractors of the synchronized clusters are symmetric. Figure 4(b) illustrates the pattern of the network for $d\,=\,0.8,$ at which the synchronous region is more than the asynchronous one. In this case, the synchronous clusters have two different symmetric attractors which are shown in figure 5(b) (blue and green attractors). The attractor of the asynchronous oscillators is still similar to the single oscillator (yellow attractor). Raising the coupling strength causes the asynchronous clusters disappear gradually. Figure 4(c) relates to $d\,=\,0.9,$ in which just four solitaries exist and the pattern is imperfect cluster synchronization. Figure 5(c) depicts the attractors of the synchronous systems (blue and green attractors), and the attractor of the solitaries (yellow attractor). As $d$ approaches unity and more than unity, all the systems are attracted with two fixed-points. Figure 6 illustrates the network pattern for $d\,=\,1.2$ in which the systems are attracted by $\left(0.78,\,1.44,\,-0.35\right)$ and $\left(-0.78,\,1.44,\,0.35\right).$
Figure 4.
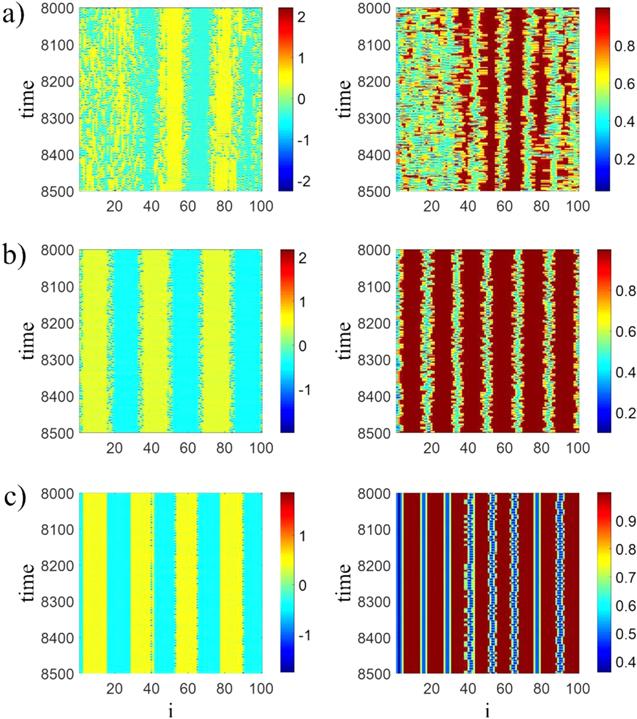
Figure 4.Spatiotemporal patterns (left panel) and local order parameters (middle panel) of network for $P\,=\,20,$ (a) multi-chimera at $d\,=\,0.77,$ (b) multi-chimera at $d\,=\,0.8,$ (c) imperfect cluster synchronization at $d\,=\,0.9.$ The initial conditions are randomly selected in the interval $x,\,y,\,z\,\in \,\left[-10,\,10\right].$ For larger coupling strengths, multi-chimera states which have multiple synchronized clusters are emerged.
Figure 5.
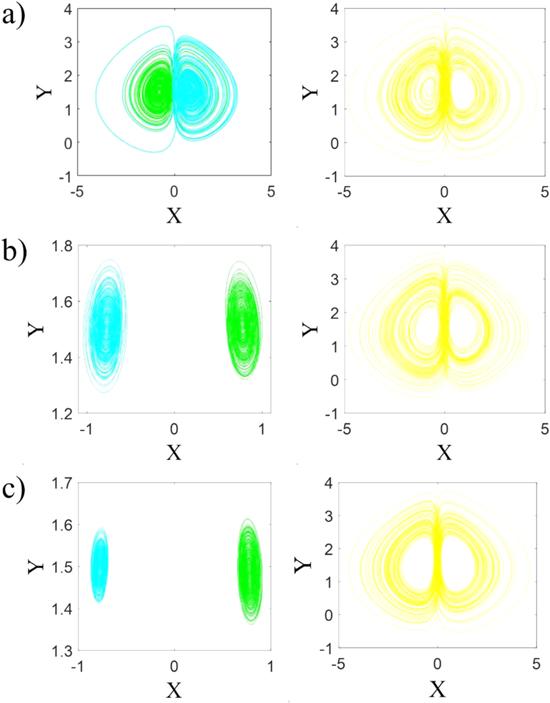
Figure 5.Attractors of the synchronized oscillators (blue and green) and the asynchronized oscillators (yellow) corresponding to the patterns of figure
Figure 6.
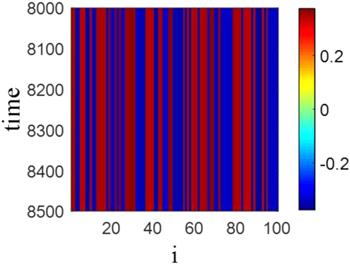
Figure 6.Spatiotemporal pattern of the network for $r\,=\,0.4$ and $d\,=\,1.2.$ For $d\,\gt \,1,$ all of the systems are attracted by two fixed-points (0.78, 1.44, −0.35) and (−0.78, 1.44, 0.35).
3.2. The effect of coupling range variation
Now we investigate the network patterns by changing the nearest neighbor numbers in different coupling strengths, which effects on the patterns significantly. When coupling strength is smaller than $0.2,$ changing the value of $r$ causes multiple transitions between asynchronization, synchronization and chimera states. As an illustration, figure 7 shows network patterns for $d\,=\,0.008$ and different coupling ranges. In this case, when the coupling range is very small, the network is asynchronous as in figure 7(a) for $r\,=\,0.1$ and by increasing $r$ until $r\,=\,0.9,$ chimera states appear as is shown in figure 7(c) for $r\,=\,0.6.$ But in this range, an exception occurs such that for $r$ values near $0.5,$ the oscillators tend to be all synchronized (figure 7(b)). Further increasing of $r$ from $0.9,$ makes the network asynchronous again.Figure 7.
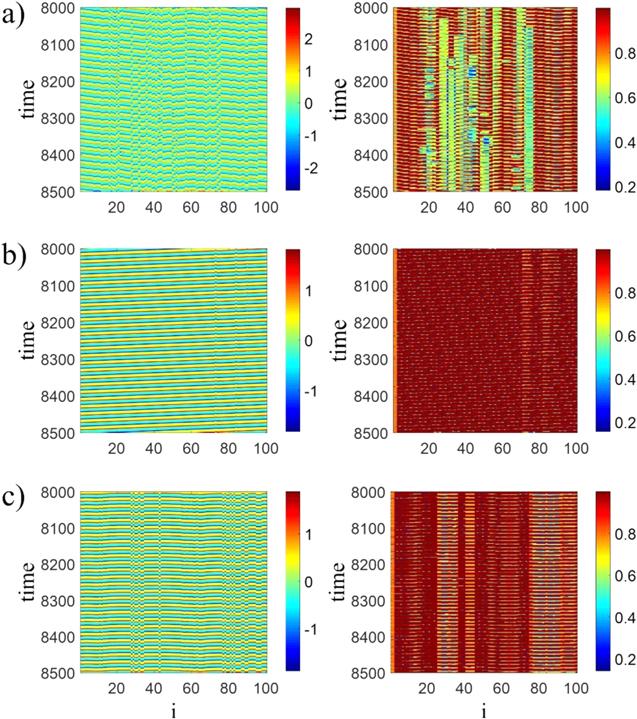
Figure 7.Spatiotemporal patterns (left panel) and local order parameters (right panel) of network for $d\,=\,0.008,$ (a) asynchronization at $r\,=\,0.1,$ (b) synchronization at $r\,=\,0.5,$ (c) chimera at $r\,=\,0.6.$ The initial conditions are randomly selected in the interval $x,\,y,\,z\,\in \,\left[-10,\,10\right].$ Similar to the coupling strength, the variation of the coupling range can change the network behavior significantly.
The network exhibits asynchronous patterns for $0.2\,\lt \,d\,\lt \,0.7$ for any value of $r.$ In $d\,=\,0.7,$ just for small coupling range, near $r\,=\,0.1,$ chimeras are observed and for more $r$ values, the oscillators oscillate asynchronously. But when $0.7\,\lt \,d\,\lt \,1,$ multi-chimera patterns are observed for most values of $r$ and raising the value of $r$ makes the clusters to be wider. Figure 8 demonstrates this claim for $d\,=\,0.8.$ When $r\,=\,0.1,$ asynchronization occurs in the network as is shown in figure 8(a), but more increase of $r,$ causes multi-chimera states. Comparing figures 8(b) and (c) shows that when more numbers of nearest neighbors are encountered in the coupling, more oscillators are involved in the same clusters. If the coupling range gets more than $0.9,$ the network becomes asynchronous.
Figure 8.
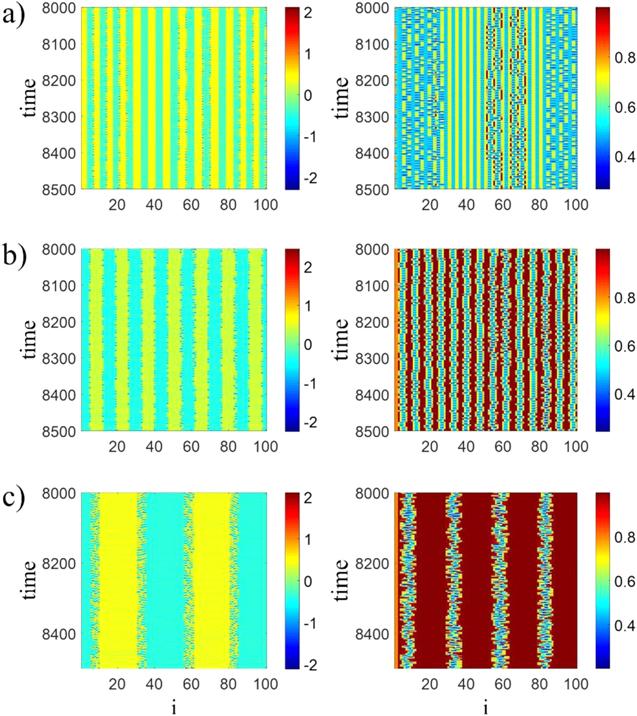
Figure 8.Spatiotemporal patterns (left panel) and local order parameters (right panel) of network for $d\,=\,0.8,$ (a) asynchronization at $r\,=\,0.1,$ (b) multi-chimera at $r\,=\,0.2,$ (c) multi-chimera at $r\,=\,0.6.$ The initial conditions are randomly selected in the interval $x,\,y,\,z\,\in \,\left[-10,\,10\right].$ It is observed that by increasing the coupling range, more oscillators are involved in the clusters and the clusters become wider.
If the coupling strength becomes equal to $1,$ variations of $r$ causes many transitions, and $d\,=\,1$ is a very sensitive point. When $r\,=\,0.1,$ asynchronization is observed and when it approaches to $r\,=\,0.2$ chimeras appear. As $r$ reaches to $0.3,$ all oscillators are attracted by two fixed-points. At $r\,=\,0.4,$ cluster synchronization state appears and in $0.5\,\lt \,r\,\lt \,0.8,$ the attractors change into some near fixed-points. For $r\,=\,0.9,$ the chimera state returns and for global coupling it alters to two fixed-points. The spatiotemporal patterns for all of these cases are shown in figure 9, and their corresponding local order parameters are illustrated in figure 10. If the coupling strength gets higher than unity, the coupling range has no effect and the all the oscillators are attracted by two fixed-points.
Figure 9.
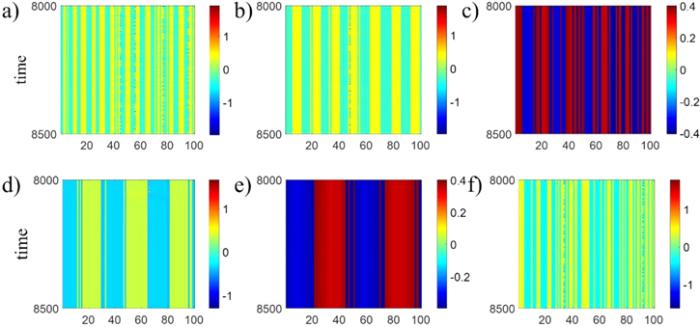
Figure 9.Spatiotemporal patterns of network for $d\,=\,1,$ (a) asynchronization at $r\,=\,0.1,$ (b) chimera at $r\,=\,0.2,$ (c) two fixed-points attractors at $r\,=\,0.3,$ (d) cluster synchronization at $r\,=\,0.4,$ (e) some near fixed-points attractors at $r\,=\,0.7,$ (f) chimera at $r\,=\,0.9.$ The initial conditions are randomly selected in the interval $x,\,y,\,z\,\in \,\left[-10,\,10\right].$ This figure shows at d=1, the value of the coupling range remarkably effects on the network behavior and the oscillators attractors.
Figure 10.
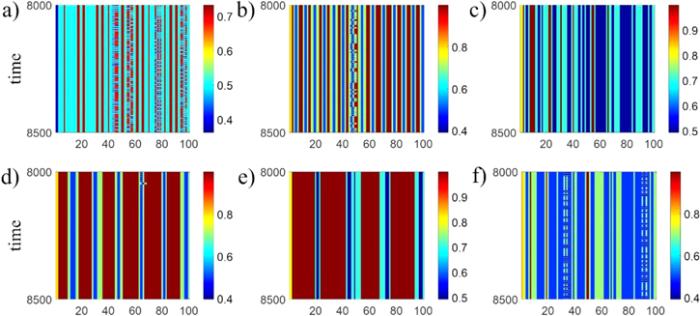
Figure 10.The local order parameters of the states shown in figure
4. Conclusion
In this paper, we investigated a ring network of symmetric finance systems connected via nearest neighbor method. The used finance system is a modification of an old system that can exhibit different symmetric attractors by varying its parameters. Here, to model the real complex behavior of financial networks, we used the parameters in which the system has two symmetric coexisting chaotic attractors. To study the dynamics of the network, the effect of both coupling strength and coupling range variations were considered. It was found that the network shows rich dynamics by changing the coupling parameters, and different behaviors such as asynchronization, synchronization, imperfect cluster synchronizations, chimera and multi-chimera states could be observed. Observing the attractors of the coupled oscillators represented that two different synchronized clusters with symmetric attractors were formed in the network which were different from the single oscillator's attractor. While the attractors of the asynchronized oscillators were similar to the main attractor.Reference By original order
By published year
By cited within times
By Impact factor
DOI:10.1016/0022-0531(86)90003-7 [Cited within: 1]
DOI:10.1016/j.chaos.2005.08.218 [Cited within: 1]
DOI:10.1016/j.chaos.2004.11.044 [Cited within: 1]
[Cited within: 1]
DOI:10.5755/j01.itc.44.2.7732 [Cited within: 1]
DOI:10.1007/s11071-011-0137-9 [Cited within: 2]
DOI:10.1038/s41598-019-53300-4 [Cited within: 1]
DOI:10.1016/j.chaos.2019.109521 [Cited within: 1]
DOI:10.1007/s100510050929 [Cited within: 1]
[Cited within: 1]
DOI:10.1126/science.aad0299 [Cited within: 1]
DOI:10.1209/0295-5075/118/10001 [Cited within: 1]
DOI:10.1063/1.4967386
DOI:10.1016/j.chaos.2018.03.025 [Cited within: 1]
[Cited within: 1]
DOI:10.1038/s41598-017-02409-5 [Cited within: 1]
DOI:10.1103/PhysRevE.95.042218
DOI:10.1016/j.cnsns.2016.06.024
DOI:10.1007/s11071-018-4664-5
DOI:10.1103/PhysRevE.100.012315 [Cited within: 1]
DOI:10.1038/srep06379 [Cited within: 1]
DOI:10.1038/ncomms8752 [Cited within: 1]
DOI:10.1063/1.5088833 [Cited within: 1]
DOI:10.1038/srep39033
DOI:10.1209/0295-5075/123/48003
DOI:10.1103/PhysRevE.93.012209
DOI:10.1103/PhysRevE.93.052223
DOI:10.1103/PhysRevE.93.012205
DOI:10.1063/1.4993836 [Cited within: 1]
DOI:10.1016/j.plrev.2018.09.003 [Cited within: 1]
DOI:10.1023/A:1022806003937 [Cited within: 1]
DOI:10.1016/j.chaos.2006.07.051 [Cited within: 1]
DOI:10.1016/j.amc.2015.12.015 [Cited within: 2]
DOI:10.1103/PhysRevE.94.012215 [Cited within: 1]