
Received:2020-03-01Revised:2020-05-30Accepted:2020-06-02Online:2020-09-16

Abstract
Keywords:
PDF (2365KB)MetadataMetricsRelated articlesExportEndNote|Ris|BibtexFavorite
Cite this article
H A M Ali, D M Habashy. The electrical impedance, AC conductivity and dielectric properties of phenol red compound investigated and modeled by an artificial neural network. Communications in Theoretical Physics, 2020, 72(10): 105701- doi:10.1088/1572-9494/aba24d
1. Introduction
Organic electronic devices have gained noticeable interest in the scientific community owing to the influence of the special properties of organics, their capabilities for device fabrication and the cheapness of the final product. Organic functional materials exhibit ease of processing and also of improving their properties. The incorporation of functional groups has endowed molecular materials with unique and interesting optoelectronic properties [1]. Organic semiconductors are performing an increasingly important role in a large area of applications, i.e. low cost and flexible electronics [2]. They are used in various fields of technology from electronics, medical and environmental diagnostics to medicine [3]. As a result, many advances have been made that recognize the crucial physical and chemical properties of these materials [4]. The optical and electrical properties of organic molecules are identified by the existence of delocalized π-electrons [5] and this has made for a quick development in the attention given to π-conjugated materials [6].In addition the properties of organic molecules are extremely sensitive to molecular packing and the electrostatic medium [5]. Moreover, charge carrier mobility, crystallinity and interface properties are essential for the performance of organic semiconductors [2]. The mechanisms of charge transport in various organic materials are under debate, whereby various models for these mechanisms are used to characterize conduction in organic materials [7]. Several computational models, such as artificial neural networks (ANNs) have been utilized as a part of materials science research for estimating the experimental results of various research [8, 9] and have established complex nonlinear relationships between the input and output data [9-11].
The present work aims to investigate the behavior of the electrical properties of bulk phenol red as an organic dye (by experimental and theoretical study). The impedance spectroscopy, electrical conductivity and electric modulus for bulk phenol red in the form of Au/phenol red/Au pellets were studied under the influence of changing frequency (42-105 Hz) and temperature (303-423 K). The conduction mechanism of the electrical transport process was deduced for AC conduction. Nyquist diagrams for real and imaginary impedances are presented for phenol red at various temperatures. Additionally, ANN modeling was used to model the impedance and the electrical properties of bulk phenol red and create acceptable outputs for the inputs that were not found during training.
2. Experimental measurements
Phenol red compound was obtained from Sigma-Aldrich and utilized for the investigations without further purification. A differential thermal analysis (DTA) for phenol red in powder form carried out using a DTA-50 Shimadzu differential thermal analyzer with a scan rate of ten °C min−1. For electrical measurements, a finely ground powder of phenol red was compressed into pellets under suitable pressure (two× 108 N m−2). The pellets had a thickness (d) of 0.72 mm and a diameter of one cm. Au electrodes were thermally deposited on both faces of the pellet by a conventional thermal evaporation technique in a vacuum of 10−4 Pa, using a high vacuum coating unit (Edwards type E 306 A, England). The electrical properties of Au/phenol red/Au were measured by a computer-controlled Hioki 3532 Hi-tester automatic LCR bridge. The data for impedance (Z), capacitance (C), and the tangent loss (tanδ), were measured directly. The frequency was varied from 42 Hz to 0.5 MHz at different temperatures ranging from 303 to 423 K. The temperature of the sample was measured by a Chromel-Alumel thermocouple over a temperature range of 303 to 423 K. The consequent calculations of the dielectric measurements were determined, as presented elsewhere [12].3. Artificial neural networks (ANN)
An artificial neural network [13, 14] was utilized to model the impedance and electrical conductivity of phenol red compound. The design of the neural system has a number of layers (input, output, and hidden layers) that involve a number of neurons (see figure 1). The treatment units of the system are the neurons. These neurons are connected to each other in various layers through links called weights, then an activation function is applied to the total of the weighted inputs of each neuron to obtain the neuron's output, see figure 2. An artificial neural network is made using many stages: first, definition of the input and output parameters for training the network; second, validation of the neural network performance to select the best structure; and finally, testing the information which was not already utilized to train the system. A learning algorithm is characterized as a method for modifying the weights and biases of a network to decrease the error function between the network output and the right output already known. The mean squared error (MSE) was determined as [15]:Figure 1.
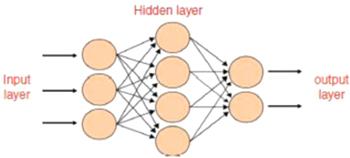
Figure 1.The architecture of a multilayer network with one hidden layer of four neurons.
Figure 2.
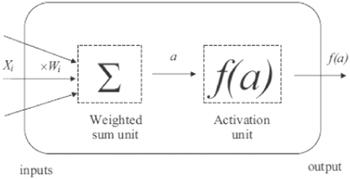
Figure 2.A simple neuron model: each input Xi is weighted by a factor Wi. The total of all inputs is calculated ${\sum }_{\mathrm{all\; inputs}}{W}_{i}{X}_{i},$ then an activation function f is applied to the product a. The neural result is taken to be f (a); and f (a)=activation function (${\sum }_{\mathrm{all\; inputs}}{W}_{i}{X}_{i}$).
4. Results and discussion
Figure 3 represents the DTA curve for the phenol red in powder form. A sharp endothermic peak was observed at 563 K. Thus, the range of temperatures between room temperature and the determined 563 K is consistent with the electrical measurements of bulk phenol red.Figure 3.
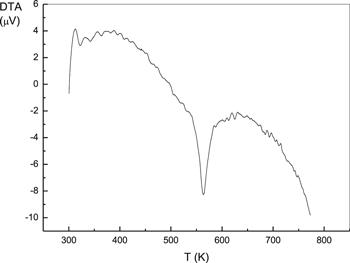
Figure 3.DTA for powdered phenol red.
4.1. Impedance spectroscopy
Complex impedance spectroscopy has been extensively applied as a useful tool for examining the frequency-dependent electrical properties of materials [16]. This procedure allows for the separation of the real and imaginary components of the complex impedance and related parameters. It also affords information about the structural properties of the material [17]. Complex impedance (Z*) has two components; Z/ and Z//, which are the real and imaginary components of Z*, respectively [17, 18]. The frequency dependence of the real part of the complex impedance (Z/) for bulk phenol red is shown in figure 4(a) for different temperatures. It can be seen that Z/ decreases with an increase of frequency, pointing to an increase in conductivity of the sample of phenol red [19]. At lower frequencies and temperatures, the Z/ curves show a high dispersion with larger values, that indicate larger effects of polarization in the sample. The Z/ values for different temperatures are then followed by a further decrease until they merge together at high frequencies. This behavior of Z/ suggests a slow dynamic relaxation process in the phenol red and may be due to the release of space charges [20]. Moreover, at a specific frequency the value of Z/ decreases with an increase of temperature, indicating a negative temperature coefficient of resistance (NTCR) type of behavior [21]. Figure 4(b) shows the frequency dependence of the imaginary part of the impedance (Z//) for bulk phenol red at different temperatures. The value of Z// increases over a range of low frequencies showing a characteristic peak at each temperature. Z// then decreases with a further increase in frequency. In this way, the nature of Z// displays the presence a relaxation process in the material. The broadening of the peaks of the Z// curves signifies the existence of temperature-dependent relaxation phenomena in phenol red [22]. In the high-frequency range, Z// reduced and amalgamated at all of the temperatures in the study, as a result of the accumulation of space charge in the material [23].Figure 4.
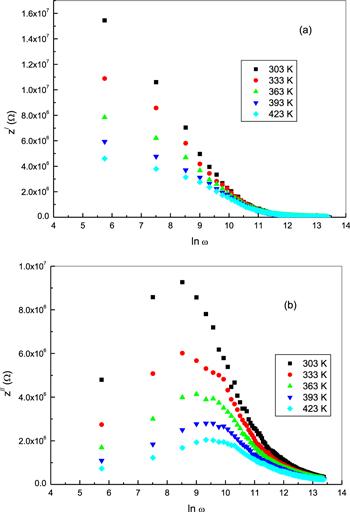
Figure 4.The frequency dependence of the parts of complex impedance for bulk phenol red at different temperatures: (a) the real part of Z/, (b) the imaginary part of Z//.
The peak position in the phenol red Z// curves moved to high-frequency values with increasing temperature. The shift seen in the peak frequency (ωz) is attributed to the existence of electrical relaxation in phenol red material [24]. The dependence of the frequency (ωz), of each peak on temperature follows the Arrhenius relation ([25]):
Figure 5.
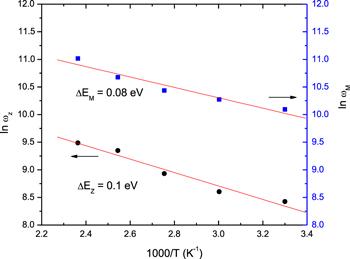
Figure 5.The temperature dependence of lnωz and lnωM.
Figure 6 represents the scaling behavior of Z// at different temperatures for bulk phenol red. All of the values of Z///Z//max for phenol red at different temperatures nearly overlap in one main curve. This is an outcome of the dynamic process of the charge, which, occurring at different time scales, displayed the same activation energy [26]. Thus, the distribution function of the relaxation times is nearly temperature-independent, with non-exponential conductivity relaxation [27].
Figure 6.
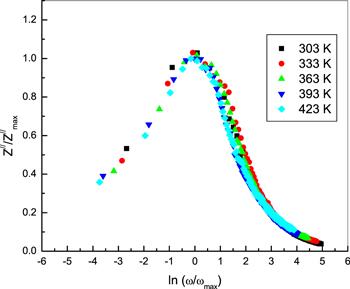
Figure 6.The scaling of Z// spectra for bulk phenol red at different temperatures.
Figure 7 shows Nyquist plots (Z/ versus Z//) of bulk phenol red at different temperatures. The plots exhibit semicircle arcs at different temperatures. The effect of temperature on the impedance behavior is much more notable at higher temperatures, as seen from the figure. The single semicircular arc suggests the presence of a granular interior (bulk) property of the material [28]. The area of the semicircle decreases with rising temperature owing to the associated decrease of the impedance. This suggests a temperature dependence on relaxation [22]. Also, the semicircles are shifted to higher frequencies, indicating that the bulk resistance of the Au/phenol red/Au sample decreases with an increase in temperature. This behavior is an indication of a thermally-activated conduction mechanism [29]. Each semicircle arc in the Nyquist plots of phenol red can be represented by an equivalent electrical circuit that consists of a parallel resistor and a capacitor [24], as shown in figure 7. The intercept of the semicircle arc on the Z/-axis as ω→zero gives the bulk resistance (R) value of the sample [30]. As can be seen from table 1, the values of R decrease with an increase in temperature. This behavior may be attributed to thermal agitation of the charge [31].
Figure 7.
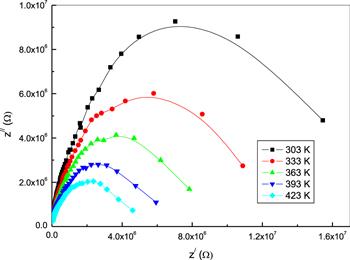
Figure 7.A Nyquist plot of Z/ versus Z// for bulk phenol red at different temperatures.
Table 1.
Table 1.Values of resistance for bulk phenol red at different temperatures.
T (K) | 303 | 333 | 363 | 393 | 423 |
---|---|---|---|---|---|
R (MΩ) | 18.87 | 12.97 | 9.24 | 6.57 | 5.35 |
New window|CSV
4.2. Electrical conductivity
For most solids, the total conductivity (σt) can be expressed as [19, 32, 33]:Figure 8.
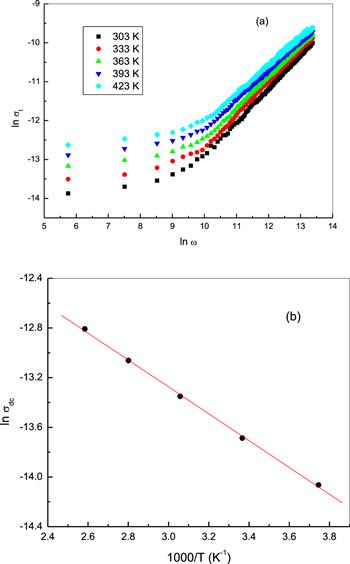
Figure 8.(a) The frequency dependence of the total electrical conductivity σt, for bulk phenol red at different temperatures and (b) the temperature dependence of DC conductivity σdc.
Figure 9 represents the frequency dependence of the AC conductivity, σac(ω) for bulk phenol red pellets at different temperatures. The conductivity showed an increase with a rise in frequency. The exponent frequency η was calculated from the slope of the linear segments of the plots and is shown in figure 10. It diminished as temperature increased. As reported by the models which describe the temperature dependence of the frequency exponent [39], the obtained values for η are in agreement with the correlated barrier hopping (CBH) model. Consequently, the mechanism for AC conduction in bulk phenol red follows the CBH model. According to this model, the frequency exponent, η is expressed as [40]:
Figure 9.
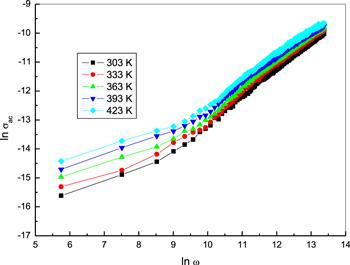
Figure 9.The frequency dependence of AC electrical conductivity σac, for bulk phenol at different temperatures.
Figure 10.
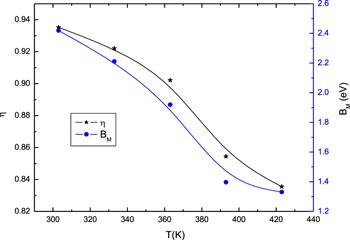
Figure 10.Temperature dependence of the frequency exponent (η) and the maximum barrier height (Bm).
The values of BM were calculated using equation (
The temperature dependence of the AC conductivity (σac) of bulk phenol red is shown in figure 11(a) at certain frequencies. As can be noticed from the figure, the σac of phenol red increases with increasing temperature. This suggests that σac is a thermally-activated process of an activation energy (ΔEac), which was determined using an Arrhenius temperature dependence of σac: σac=σo exp (−ΔEac/kT). The activation energy for AC conduction was calculated at different frequencies and plotted as a function of frequency, as shown in figure 11(b). The ΔEac of phenol red decreases with increasing frequency. A rise of the applied field frequency promotes an electronic jump between the localized states, consequently ΔEac decreases with increasing frequency [44]. The smaller values of AC activation energy as compared with that of DC activation energy affirm that hopping conduction is the dominant mechanism for carrier transport between localized states in phenol red [35, 45].
Figure 11.
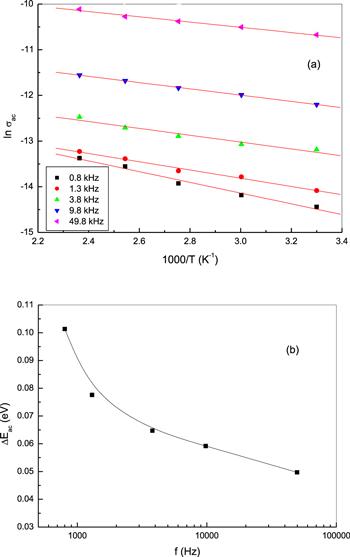
Figure 11.(a) The temperature dependence of AC electrical conductivity σac, at different frequencies and (b) the variation in ΔEac as a function of frequency for bulk phenol.
4.3. Modeling the real and imaginary parts of impedance and total electrical conductivity using ANN
Artificial neural network-based methods have been used in the fields of materials science and engineering research prediction, modeling, control, recognition design, and optimization. The reason for using artificial neural networks for materials science research is their ability to identify and learn the underlying nonlinear relationships between the input and output data. Furthermore, there is a need to recognize the properties of the materials used in design and manufacture, to maximize their capabilities for different applications, both experimentally and theoretically. The application of artificial neural network models in materials science research is becoming increasingly popular and is widely used in various branches of advanced materials science research by many authors [46]. Wang et al [47] established the use of a neural network model to predict the refractive index of ionic liquids and alcohols at different temperatures. Li et al [48] reported on using ANN models to predict the polarizability and the absolute permittivity of hydrocarbon compounds.4.3.1. The structure of the ANN model
In the present study, an artificial neural network model was used to simulate and predict the impedance and the total electrical conductivity. Using this technique, we attempted to mathematically model the real and imaginary impedance and the total electrical conductivity using ANNs. In this regard, three different ANN models were designed to achieve this goal. The proposed ANN models are presented in figure 12. The first model calculates and predicts the real part of the impedance. The second model simulates and predicts the imaginary part of the impedance and third model calculates and predicts the total electrical conductivity. The parameters used in all three networks are found in table 2.Figure 12.
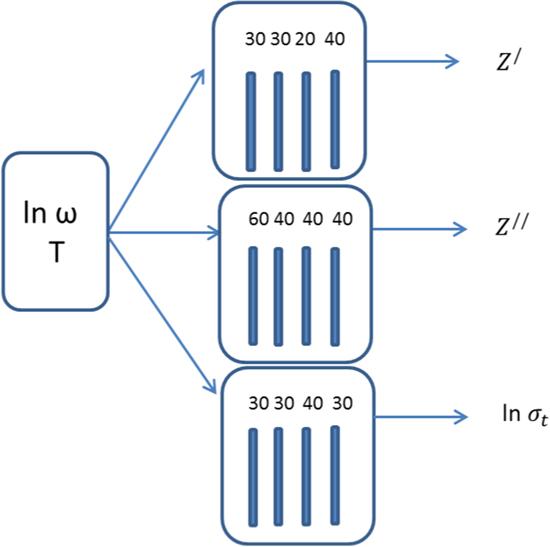
Figure 12.A block diagram of ANN-based modeling of Z/, Z// and ln σt.
Table 2.
Table 2.Overview of all the parameters in the ANNs for Z/, Z// and ln σt for bulk phenol red.
No. | Structural part of the ANN | ANN1 | ANN2 | ANN3 |
---|---|---|---|---|
1 | Inputs | lnω, T | lnω, T | lnω, T |
2 | Outputs | Z/ | Z// | ln σt |
3 | No. of hidden layers | 4 | 4 | 4 |
4 | No. of neurons | 30, 30, 20, 40 | 60, 40, 40, 40 | 30, 30, 40, 30 |
5 | No. of epochs=no. of training | 1000 | 800 | 1000 |
6 | Training algorithm | trainrp | trainrp | trainrp |
7 | Performance | 0.005 | 0.026 | 0.0002 |
8 | Transfer function | logsig | logsig | logsig |
9 | Output function | purelin | purelin | purelin |
New window|CSV
4.3.2. Simulation results
The model was trained using experimental data. The ANN was trained for the measured datasets. The network was exercised to get a better MSE and the best execution for the network. The training techniques are found in figures 13(a)-(c), which reveal the mean squared error of the system beginning at a higher value and decreasing to the smallest one. It appears the network is learning. Training was terminated after an average mean square error of (0.005, 0.026 and 0.0002) was reached for the three (1000, 800 and 1000 Epochs) ANNs, respectively. The performance of the trained network was examined by the relation between the simulated results from the trained neural system and the experimental data (target), see figures 14(a)-(c). A very good coincidence between the model results and the validation data was obtained, which shows that the prepared system has optimal generalization performance. Also, the prediction of the impedance and the total electrical conductivity (at 393 K) which were not included in our training of our model, were found to be in good coincidence with the target. In addition, the equation obtained from our model predicts the impedance and the total electrical conductivity at 443 K, which was not found in training and was predicted by our model alone. Thus, ANN can predict the results of any other temperature, this gives the ANN the possibility for wide utilization in modeling the real and imaginary parts of the impedance and the total electrical conductivity of bulk phenol red.Figure 13.
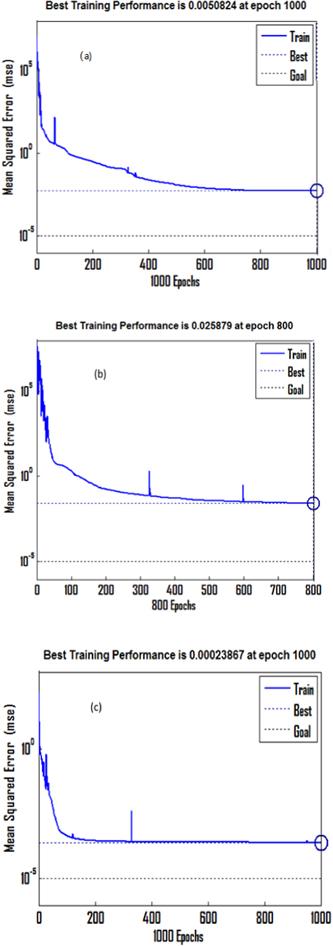
Figure 13.The training procedure for ANN models for (a) Z/, (b) Z// and (c) ln σt at different temperatures.
Figure 14.
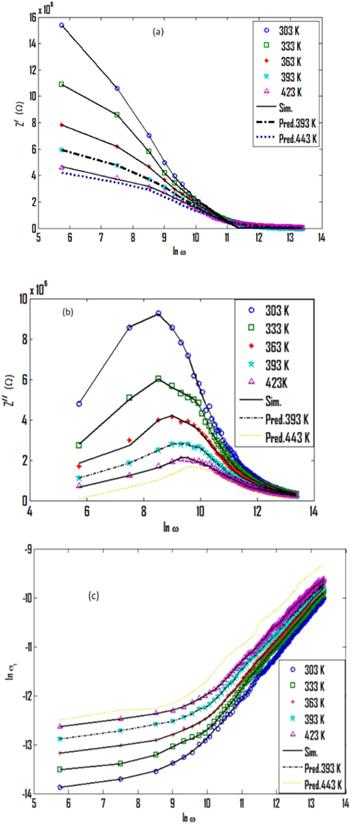
Figure 14.The ANN simulation and prediction results for (a) Z/, (b) Z// and (c) ln σt at different temperatures.
4.4. The electric modulus formalism
Modulus spectroscopy expounds the bulk dielectric behavior of materials and this formalism diminishes the effects of electrode polarization [49, 50]. The electric modulus (M*) of a material is calculated as [51, 52]:Figure 15.
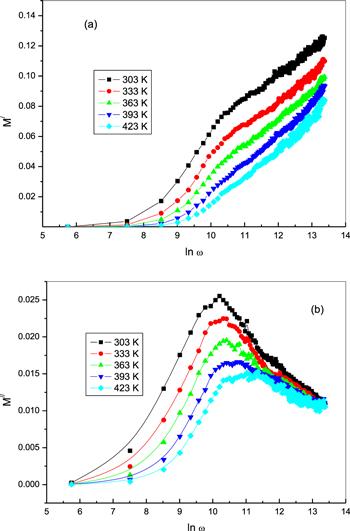
Figure 15.The frequency dependence of (a) the real part of the electric modulus M/, and (b) the imaginary part of the electric modulus M// for bulk phenol at different temperatures.
Figure 16.
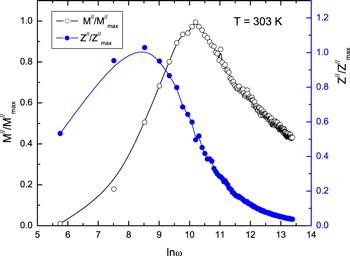
Figure 16.The normalized spectra of Z///Z//max and M///M//max as a function of frequency at 303 K.
5. Conclusions
The electrical and dielectric behavior of bulk phenol red were investigated by studying complex impedance and modulus spectroscopy. Z/ (the real part of the complex impedance) of phenol red decreases with an increase of temperature, expressing a negative temperature coefficient of resistance type of behavior. The behavior of Z// (the imaginary part of the complex impedance) of phenol red exhibited the existence of a relaxation process in the material with an activation energy of 0.1 eV. The peaks of the Z// spectra were shifted to a higher frequency on increasing temperature. The scaling behavior of Z// at specific temperatures for bulk phenol red suggests that the dynamic charge processes occurring at different time scales displayed the same activation energy. Nyquist plots (Z/ versus Z//) of bulk phenol red exhibited semicircle arcs. The variation of the DC conductivity with temperature showed an Arrhenius relation dependence with an activation energy of 0.094 eV. The AC electrical conductivity obeyed a universal power law with a frequency exponent decreasing with a rising temperature and satisfied the correlated barrier hopping model. The barrier height displayed a similar nature for the frequency exponent in its dependence on temperature. In the imaginary part of the spectra of the electric modulus, a single relaxation peak was noticed and its position shifted to a high frequency with rising temperature. The activation energy estimated for M// was close to the activation energy obtained for Z//. The frequency reliance of the normalized functions Z///Z//max and M///M//max and suggests a non-Debye-type relaxation process for the phenol red material. Moreover, a four-layer feed neural network was optimized to predict the real and imaginary parts of the impedance and the electrical conductivity of bulk phenol red at certain temperatures. The lowest MSE values were found (0.005, 0.026 and 0.0002) with four hidden layers. A regression analysis was performed between the model's predicted values and the experimental results. The ANN-predicted values are in close agreement with those determined from experimental results in the simulation and prediction process. Thus, the ANN model can effectively simulate and predict the electrical properties of bulk phenol red.Acknowledgments
The authors are grateful to Prof. M M El-Nahass, Ain Shams University, for his fruitful discussions.Reference By original order
By published year
By cited within times
By Impact factor
[Cited within: 1]
DOI:10.1038/srep41171 [Cited within: 2]
[Cited within: 1]
[Cited within: 1]
[Cited within: 2]
DOI:10.1021/cr050140x [Cited within: 1]
DOI:10.1063/1.3246160 [Cited within: 1]
[Cited within: 1]
DOI:10.1016/j.ceramint.2014.04.095 [Cited within: 2]
DOI:10.1016/j.eswa.2007.10.005
[Cited within: 1]
DOI:10.1140/epjp/i2017-11595-4 [Cited within: 1]
DOI:10.1007/s10973-014-4002-1 [Cited within: 1]
DOI:10.1088/1757-899X/100/1/012023 [Cited within: 1]
DOI:10.1016/j.icheatmasstransfer.2015.08.015 [Cited within: 1]
DOI:10.1063/1.3273319 [Cited within: 1]
DOI:10.5185/amlett.2011.2228 [Cited within: 2]
DOI:10.1039/C5TA01660F [Cited within: 1]
DOI:10.1016/j.jascer.2017.01.002 [Cited within: 2]
DOI:10.1016/j.jallcom.2006.11.081 [Cited within: 1]
DOI:10.1016/j.ceramint.2014.03.038 [Cited within: 1]
DOI:10.1007/s40145-014-0087-z [Cited within: 2]
DOI:10.4236/jmp.2012.38114 [Cited within: 1]
DOI:10.1142/S201019451301060X [Cited within: 2]
DOI:10.1016/j.physb.2005.10.104 [Cited within: 2]
DOI:10.1038/srep13645 [Cited within: 2]
DOI:10.2298/PAC1403145B [Cited within: 1]
DOI:10.1155/2008/746256 [Cited within: 1]
DOI:10.1016/j.ssi.2005.12.019 [Cited within: 1]
DOI:10.1016/j.kijoms.2016.10.001 [Cited within: 1]
DOI:10.3938/jkps.56.462 [Cited within: 1]
DOI:10.1080/00018738700101971 [Cited within: 1]
DOI:10.1038/267673a0 [Cited within: 1]
DOI:10.1007/s10853-011-5344-8 [Cited within: 1]
DOI:10.1016/S0925-3467(00)00035-5 [Cited within: 2]
DOI:10.1016/j.ssc.2012.03.002 [Cited within: 1]
DOI:10.1007/s12034-011-0103-7 [Cited within: 1]
DOI:10.1039/C4RA11323C [Cited within: 1]
DOI:10.1016/j.physb.2007.12.015 [Cited within: 1]
DOI:10.1063/1.4749414 [Cited within: 1]
DOI:10.1103/PhysRevB.6.1572 [Cited within: 1]
DOI:10.1007/s003390201306
DOI:10.1142/S021797920703587X [Cited within: 1]
DOI:10.1016/j.mssp.2013.11.006 [Cited within: 1]
DOI:10.1016/j.mseb.2010.03.029 [Cited within: 1]
[Cited within: 1]
DOI:10.1080/15567036.2019.1648605 [Cited within: 1]
[Cited within: 1]
DOI:10.1016/j.matchemphys.2011.07.006 [Cited within: 1]
DOI:10.1063/1.3676254 [Cited within: 1]
DOI:10.4236/msa.2011.210192 [Cited within: 1]
DOI:10.1007/s12034-011-0163-8 [Cited within: 1]
DOI:10.1063/1.4991484 [Cited within: 1]
DOI:10.1007/s40145-015-0152-2 [Cited within: 2]