赵博璇,刘明,李建伟
(河北工业大学 人工智能与数据科学学院,天津 300401)
摘要:
利用The Cancer Genome Atlas和Genotype-Tissue Expression公共数据检索收集胃癌(Gastric cancer,GC)基因表达数据集,筛选与早期胃癌密切相关的基因并构建胃癌早期诊断预测模型。运用Deseq2软件包筛选早期胃癌差异基因,并对差异基因进行GO和KEGG富集分析。通过STRING数据库建立其蛋白质相互作用网络并利用Cytoscape软件提取关键子网得到候选关键基因,进一步利用MedCalc软件确认胃癌早期诊断关键基因。根据筛选得到的10个关键基因构建基于支持向量机、随机森林、朴素贝叶斯、K-近邻、极限梯度提升和自适应提升等六种算法的胃癌早期诊断预测模型,依据ROC曲线和准确率等评价指标对各个分类器模型进行评估,通过独立测试集验证得到极致梯度提升诊断预测模型为最优模型。本研究成果为提高结胃癌早期诊断的研究提供了新的思路和方法。
关键词: 胃癌 关键基因 生物信息学 诊断预测模型 极限梯度提升
DOI:10.12113/202108017
分类号:Q344+.13
文献标识码:A
基金项目:国家自然科学基金项目(No.62072154).
Research on an early diagnosis and prediction model of gastriccancer based on bioinformatics
ZHAO Boxuan, LIU Ming, LI Jianwei
(School of Artificial Intelligence,Hebei University of Technology,Tianjin 300401, China)
Abstract:
Gastric cancer (GC) gene expression data were retrieved from the Cancer Genome Atlas and Genotype-Tissue Expression public databases. Genes closely related to early gastric cancer were screened and utilized to construct an early diagnosis and prediction model for gastric cancer. The differential genes of early gastric cancer were screened with Deseq2 software package, and GO and KEGG enrichment analyses were performed on the differential genes. The protein-protein interaction network of the differential genes was established with STRING database. The key subnetworks were extracted from the network to obtain candidate key genes by Cytoscape, and ten key genes were identified by MedCalc software. According to the ten key genes, six early diagnosis and prediction models of gastric cancer were constructed based on the algorithms of Support Vector Machine, Random Forest, Naive Bayes, K-nearest neighbor, XGBoost, and Adaptive Boosting. Each model was evaluated by ROC curve, accuracy rate, and other indicators. The diagnosis and prediction model based on XGBoost was verified as the optimal model by independent test set validation. The results of this study provide new ideas and methods for researchers to improve the efficiency of early diagnosis and prediction for gastric cancer.
Key words: Gastric cancer Key genes Bioinformatics Diagnosis and prediction model XGBoost
赵博璇, 刘明, 李建伟. 基于生物信息学的胃癌早期诊断预测模型研究[J]. 生物信息学, 2022, 20(4): 274-283. DOI: 10.12113/202108017.

ZHAO Boxuan, LIU Ming, LI Jianwei. Research on an early diagnosis and prediction model of gastric cancer based on bioinformatics[J]. Chinese Journal of Bioinformatics, 2022, 20(4): 274-283. DOI: 10.12113/202108017.

基金项目 国家自然科学基金项目(No.62072154) 通信作者 李建伟, 男, 教授, 研究方向: 生物信息学.E-mail: lijianwei@hebut.edu.cn 作者简介 赵博璇, 女, 硕士研究生, 研究方向: 生物信息学.E-mail: 15227107521@163.com 文章历史 收稿日期: 2021-08-26 修回日期: 2021-10-11
Contents Abstract Full text Figures/Tables PDF
基于生物信息学的胃癌早期诊断预测模型研究
赵博璇



河北工业大学 人工智能与数据科学学院,天津 300401
收稿日期: 2021-08-26; 修回日期: 2021-10-11
基金项目: 国家自然科学基金项目(No.62072154)
作者简介:赵博璇, 女, 硕士研究生, 研究方向: 生物信息学.E-mail: 15227107521@163.com
通信作者: 李建伟, 男, 教授, 研究方向: 生物信息学.E-mail: lijianwei@hebut.edu.cn
摘要: 利用The Cancer Genome Atlas和Genotype-Tissue Expression公共数据检索收集胃癌(Gastric cancer,GC)基因表达数据集,筛选与早期胃癌密切相关的基因并构建胃癌早期诊断预测模型。运用Deseq2软件包筛选早期胃癌差异基因,并对差异基因进行GO和KEGG富集分析。通过STRING数据库建立其蛋白质相互作用网络并利用Cytoscape软件提取关键子网得到候选关键基因,进一步利用MedCalc软件确认胃癌早期诊断关键基因。根据筛选得到的10个关键基因构建基于支持向量机、随机森林、朴素贝叶斯、K-近邻、极限梯度提升和自适应提升等六种算法的胃癌早期诊断预测模型,依据ROC曲线和准确率等评价指标对各个分类器模型进行评估,通过独立测试集验证得到极致梯度提升诊断预测模型为最优模型。本研究成果为提高结胃癌早期诊断的研究提供了新的思路和方法。
关键词: 胃癌 关键基因 生物信息学 诊断预测模型 极限梯度提升
Research on an early diagnosis and prediction model of gastric cancer based on bioinformatics
ZHAO Boxuan



School of Artificial Intelligence, Hebei University of Technology, Tianjin 300401, China)
Abstract: Gastric cancer (GC) gene expression data were retrieved from the Cancer Genome Atlas and Genotype-Tissue Expression public databases. Genes closely related to early gastric cancer were screened and utilized to construct an early diagnosis and prediction model for gastric cancer. The differential genes of early gastric cancer were screened with Deseq2 software package, and GO and KEGG enrichment analyses were performed on the differential genes. The protein-protein interaction network of the differential genes was established with STRING database. The key subnetworks were extracted from the network to obtain candidate key genes by Cytoscape, and ten key genes were identified by MedCalc software. According to the ten key genes, six early diagnosis and prediction models of gastric cancer were constructed based on the algorithms of Support Vector Machine, Random Forest, Naive Bayes, K-nearest neighbor, XGBoost, and Adaptive Boosting. Each model was evaluated by ROC curve, accuracy rate, and other indicators. The diagnosis and prediction model based on XGBoost was verified as the optimal model by independent test set validation. The results of this study provide new ideas and methods for researchers to improve the efficiency of early diagnosis and prediction for gastric cancer.
Key Words: Gastric cancer Key genes Bioinformatics Diagnosis and prediction model XGBoost
胃癌是一种极为常见的恶性肿瘤,其发生于胃粘膜上皮细胞,在全球癌症死亡率排名中位居第二[1]。在我国,胃癌拥有较高的发病率和死亡率,位列我国恶性肿瘤的第三位,且全球新发胃癌病例中约有一半来自中国[2-3]。胃癌患者的早期症状不显著,难以引起人们重视,只有当肿瘤细胞增殖影响胃部正常功能时,患者才出现较为明显的症状。根据胃癌早期发病机制建立诊断预测模型,及早发现胃癌患者,可使患者避免错过早期治疗的最佳时机,辅以有效治疗可以极大提升胃癌患者的五年生存率。本研究通过生物信息学技术对胃癌基因表达数据进行特征处理,采用机器学习算法构建胃癌早期诊断预测模型,为胃癌早期诊断的研究提供了新思路和新方法。
随着高通量生物技术和生物信息学的迅猛发展,不断有学者根据人类基因表达谱数据对胃癌开展各种层面的研究。JIANG K等通过对GEO(Gene Expression Omnibus,https://www.ncbi.nlm.nih.gov/geo)数据库中的GSE29272数据集进行研究,发现了5个可能代表胃癌的新型预后生物标志物(ASPN、COL1A1、FN1、VCAN和MUC5AC)[4]。Chen J等人根据TCGA(The Cancer Genome Atlas,TCGA,https://portal.gdc.cancer.gov)数据库中胃癌患者的遗传和临床数据,通过构建加权基因共表达网络分析,得到7个影响胃癌患者生存的基因(PDGFRB、COL8A1、EFEMP2、FBN1、EMILIN1、FSTL1 和KIRREL)[5]。对人类胃癌组学数据的探索可为胃癌的预防、治疗和诊断提供强有力的帮助。本研究的工作流程主要包括数据下载与处理、胃癌早期诊断关键基因的筛选和诊断预测模型的构建3个部分。其中关键基因的筛选通过差异基因分析、PPI网络分析和诊断效能分析等3个步骤完成,并对差异基因进行GO和KEGG富集分析。
1 数据与处理1.1 数据描述与下载TCGA即癌症基因组图谱数据库,它从创建至今已收录了30多种类型癌症的基因组学数据,存储了丰富的与癌症相关的各类信息[6]。TCGA数据库中胃癌基因表达数据由二代测序技术(RNA-seq)获得,用户利用官方下载工具gdc-client,可下载基因表达丰度为read count值形式的原始表达数据,并可同时获得相关的临床数据。GTEx(Genotype-Tissue Expression,GTEx,https://gtexportal.org/home)名为基因型-组织表达数据库。截至2015年底,它已包括大约900名尸体捐赠者的大量尸检样本数据,涵盖50多个组织[7]。
在本研究中,从TCGA数据库中筛选得到201个胃癌样本,其中正常组织32个,早期胃癌组织样本为169个(56例癌症Ⅰ期,113例癌症Ⅲ期)。TCGA数据库记录的正常组织测序结果较少,大量病人的正常组织测序数据并未包含在内,如胃癌正常组织样本量与癌组织早期样本量相差近5倍。为增加正常组织样本量,本研究通过GTEx数据库官网下载原始表达矩阵文件和样本信息文件,根据样本信息从表达矩阵中提取出174个正常胃部组织的基因表达数据。
1.2 数据预处理对获得的TCGA和GTEx的胃癌原始表达数据集进行预处理,通过筛选同时存在于两个数据库的基因,最终得到二者的联合数据集。该数据集共包含375个样本,正常组织和胃癌早期组织样本分别为206个和169个(见表 1)。
表1(Table 1)

数据库 正常组织样本数 早期癌组织样本数 样本总数
TCGA 32 169 201
GTEx 174 0 174
样本总数 206 169 375
表 1 基因表达数据集描述信息Table 1 Description of gene expression dataset?
2 方法2.1 差异表达分析TCGA和GTEx为不同平台的测序数据,其数据因获取的方式不同而存在批次差异,在进行差异分析前先进行批次效应处理[8]。本研究使用R平台(R 4.0.3,https://www.r-project.org)中自带去批次效益函数的Deseq2软件包对TCGA和GTEx联合数据集进行批次效益去除和差异表达基因(Differentially expressed genes, DEGs)筛选。Deseq2软件包仅支持未经标准化的read count形式的数据类型[9],设置|log2FC|>2,Benjamini Hochberg校正后的差异显著性阈值P.adj < 0.05。
2.2 富集分析基因本体论(Gene Oncology, GO)分析被广泛应用于降低复杂性和全基因组的表达研究,其包括分子功能(Molecular Function,MF)、细胞组分(Cellular Component,CC)和生物过程(Biological process,BP)3部分。KEGG通路富集分析采用的是京都基因与基因组百科全书数据库((Kyoto Encyclopedia of Genes and Genomes,KEGG),它是一个基因功能系统分析库,包括基因组、化学和系统功能等信息。本研究利用R语言的clusterProfiler软件包实现差异基因的GO和KEGG富集分析,富集筛选阈值设定为经Benjamini Hochberg校正后的P < 0.05。
2.3 PPI网络分析STRING数据库(https://string-db.org)整合了蛋白质间所有已知关联和预测关联,包括物理相互作用和功能关联,从多个数据源收集评分证据,收录了千万种蛋白质间的相互作用[10]。通过STRING数据库构建蛋白质间的相互作用(Protein-protein interaction,PPI)网络,可得到关系密切的蛋白基因集,有助于筛选关键基因。利用Cytoscape(Cytoscape 3.7.0,https://cytoscape.org)软件中的MCODE插件搜索提取PPI网络中的关键子网,关键子网中的基因即可被认为是候选关键基因。
2.4 诊断效能分析通过MedCalc(MedCalc 19.1,https://www.medcalc.org)软件对候选关键基因的诊断能力进行评价分析。基于受试者工作特征曲线(Receiver Operating Characteristic,ROC)[11]、曲线下面积(AUC)、敏感性和特异性等指标可以评估关键基因的识别能力。随着ROC曲线下面积的增大,关键基因对胃癌早期识别能力逐渐增大,本研究设置AUC值大于0.9的基因可作为早期诊断关键基因。
2.5 诊断预测模型构建使用Python(Python 3.7.4,https://www.python.org) 机器学习扩展包scikit-learn开发实现分别基于支持向量机(Support Vector Machines,SVM)[12]、随机森林(Random Forest,RF)[13]、朴素贝叶斯(Naive Bayes Model,NBM)[14]、K近邻(K-Nearest Neighbor,KNN)[15]、极致梯度提升(eXtreme Gradient Boosting,XGBoost)[16]和自适应提升(Adaptive Boosting,AdaBoost)[17]的胃癌早期诊断预测模型。
2.6 模型验证与评估不同算法训练得到的分类器模型在训练集上具有不同的表现,广泛应用的评价指标有:准确率(Accuracy)、精确率(Precision)、召回率(Recall)[18]、F1_score[19]、ROC曲线和AUC值等。AUC定义为ROC曲线下面积值,AUC作为一个数值,其越大说明分类模型越好[20]。混淆矩阵常被用作二分类模型的评判指标[21]。
3 结果分析3.1 差异表达分析对于TCGA和GTEx联合数据集,通过Deseq2软件包进行批次效益去除并筛选差异表达基因,得到1 524个DEGs,包含735个上调基因和789个下调基因,其火山图(见图 1)。
图 1(Figure 1)
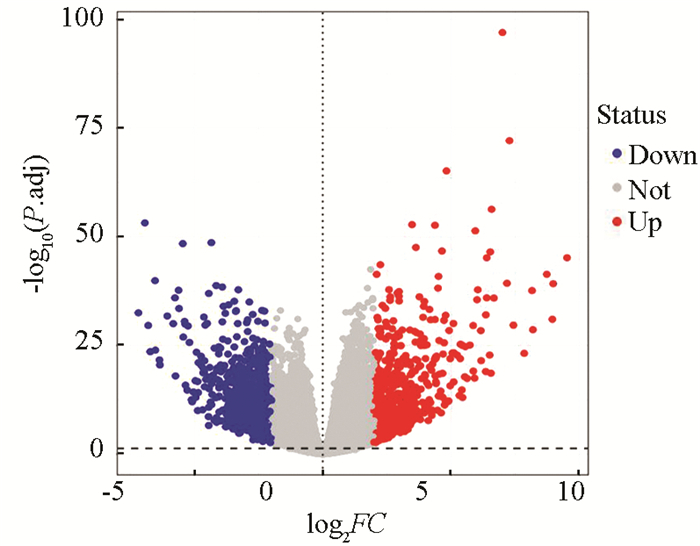
3.2 GO富集分析通过clusterProfiler软件包对差异基因进行GO和KEGG功能富集分析。GO富集分析结果中共包含501个条目,其中细胞组分条目48条,分子功能条目125条,生物过程条目328条。将P.adjust值按照升序排列,分别选取三部分前10条目进行展示(见图 2)。分析表明差异基因主要富集于生物过程上,包括表皮细胞分化、肌肉系统过程和皮肤发育等;细胞组分功能主要富集于细胞外基质、细胞顶端和转运复合体;分子功能主要富集于受体配体活性、信号受体及内肽酶活性,主要结果(见表 2)。
图 2(Figure 2)
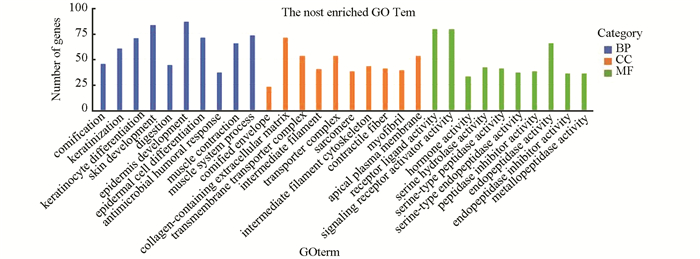
表2(Table 2)

BP GO: 0008544 epidermis development 1.32×10-16 1.14×10-13 86
BP GO: 0043588 skin development 3.23×10-18 4.19×10-15 83
BP GO: 0003012 muscle system process 3.75×10-11 1.95×10-08 73
BP GO: 0009913 epidermal cell differentiation 1.16×10-15 8.62×10-13 71
CC GO: 0062023 collagen-containing extracellular matrix 1.30×10-11 3.41×10-09 71
CC GO: 0045177 apical part of cell 4.53×10-06 1.49×10-04 57
CC GO: 1902495 transmembrane transporter complex 7.46×10-09 1.30×10-06 53
CC GO: 1990351 transporter complex 1.76×10-08 1.85×10-06 53
MF GO: 0048018 receptor ligand activity 6.58×10-12 4.99×10-09 79
MF GO: 0030546 signaling receptor activator activity 1.11×10-11 4.99×10-09 79
MF GO: 0004175 endopeptidase activity 2.28×10-08 2.56×10-06 65
MF GO: 0015267 channel activity 9.65×10-06 3.39×10-04 61
表 2 GO功能富集分析部分结果Table 2 Partial results of GO function enrichment analysis
KEGG通路富集分析结果中共包含32个条目,差异基因主要富集在神经活性配体-受体相互作用、细胞因子-细胞因子受体相互作用和cAMP信号等通路。将经Benjamini Hochberg校正后的P值按升序排列,选择前10条目进行气泡图绘制(见图 3)。表 3全面地展示了将通路包含基因数量按照降序排列的前10条目结果。
图 3(Figure 3)
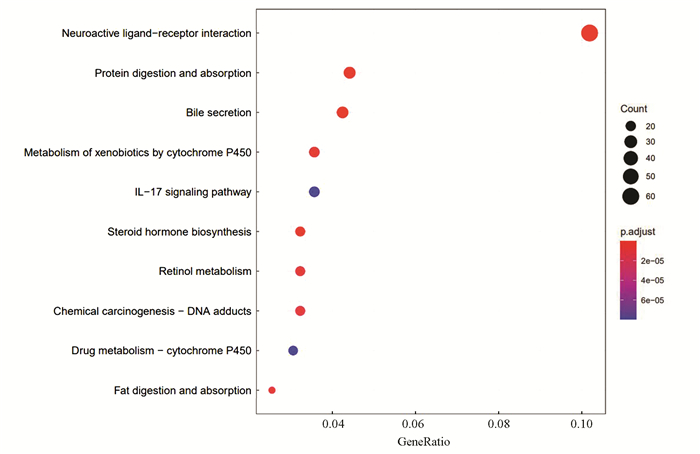
表3(Table 3)

hsa04080 Neuroactive ligand-receptor interaction 8.26×10-11 2.45×10-08 60
hsa04060 Cytokine-cytokine receptor interaction 1.77×10-04 2.86×10-03 39
hsa04024 cAMP signaling pathway 4.45×10-05 9.40×10-04 33
hsa04020 Calcium signaling pathway 2.37×10-03 2.60×10-02 30
hsa05207 Chemical carcinogenesis- receptor activation 2.94×10-03 3.11×10-02 27
hsa04974 Protein digestion and absorption 1.21×10-08 1.19×10-06 26
hsa04976 Bile secretion 2.12×10-09 3.14×10-07 25
hsa00980 Metabolism of xenobiotics by cytochrome P450 9.36×10-08 5.54×10-06 21
hsa04657 IL-17 signaling pathway 2.69×10-06 7.98×10-05 21
hsa04972 Pancreatic secretion 3.62×10-05 8.24×10-04 20
表 3 KEGG通路富集分析部分结果Table 3 Partial results of KEGG pathway enrichment analysis
3.3 PPI网络分析利用STRING数据库对1 524个DEGs构建其PPI网络,并通过Cytoscape软件中的MCODE插件获得每个蛋白质相互作用子网的评分,按照得分递减顺序提取前两名的子网为关键子网(见图 4)。两个关键子网中共包含的58个基因作为胃癌早期诊断候选关键基因。
图 4(Figure 4)
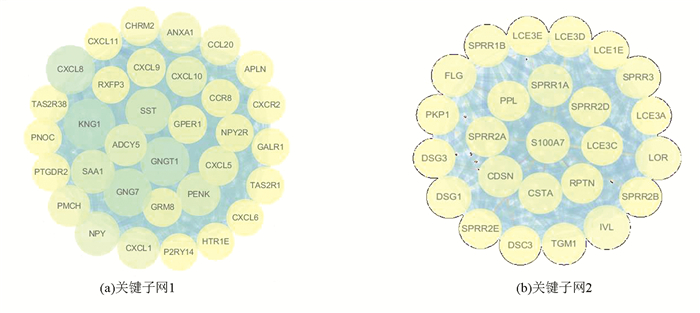
分别对两个关键子网中包含的基因进行GO功能富集分析,富集分析结果表明关键子网1所包含的33个基因主要富集在生物过程上,包括粒细胞趋化、趋化因子介导信号通路和G蛋白耦联受体信号通路等;关键子网2所包含的25个基因主要富集于生物过程的角质细胞分化和交联肽。
3.4 诊断效能分析基于基因表达数据,利用MedCalc软件对58个候选关键基因进行诊断效能分析,结果分别在图 5中进行展示。提取AUC值大于0.9的基因,最终得到10个胃癌早期诊断关键基因,它们分别为CXCL11、CCR8、CXCL9、CXCL10、CXCL1、CCL20、CXCL8、CXCL6、APLN、HTR1E。关键基因的诊断效能结果如表 4所示,其敏感性和特异性均高于70%。
图 5(Figure 5)
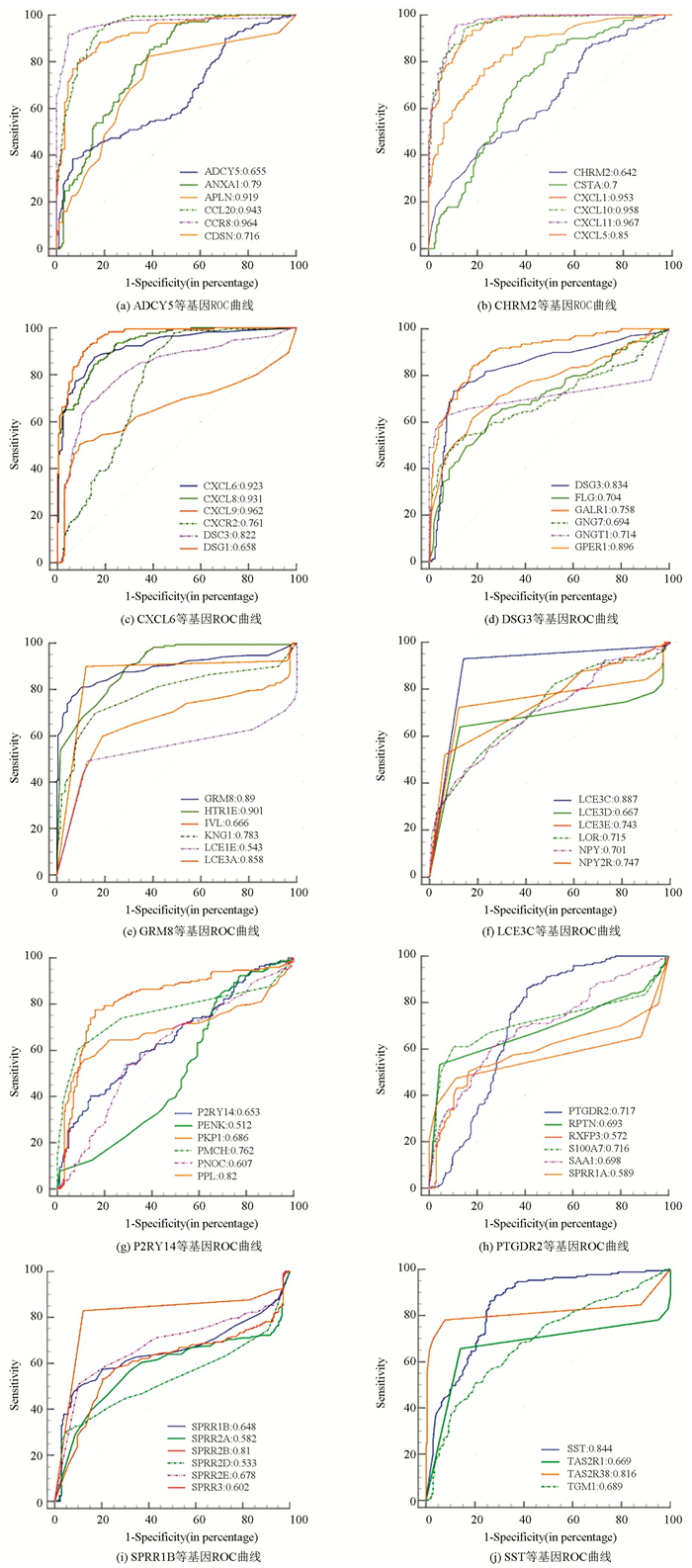
表4(Table 4)

CXCL11 0.967 95.86 88.35 < 0.001
CCR8 0.964 91.72 95.15 < 0.001
CXCL9 0.962 92.9 86.41 < 0.001
CXCL10 0.958 94.67 84.47 < 0.001
CXCL1 0.953 91.12 84.47 < 0.001
CCL20 0.943 93.49 82.52 < 0.001
CXCL8 0.931 84.02 84.95 < 0.001
CXCL6 0.923 97.57 84.47 < 0.001
APLN 0.919 79.29 91.26 < 0.001
HTR1E 0.901 88.76 71.84 < 0.001
表 4 基于关键基因的早期胃癌分类效果Table 4 Classification effect of early gastric cancer based on key genes
3.5 诊断预测模型构建利用10个胃癌早期诊断关键基因构建胃癌的早期诊断模型,具体步骤如下:
1) 提取出10个关键基因在TCGA联合GTEx数据集的表达值形成新的表达谱矩阵。
2) 将来源于TCGA联合GTEx数据集的169个早期胃癌样本和206个正常样本分别随机提取1/11组成独立测试集,用于验证诊断预测模型的鲁棒性和泛化能力。独立测试集共包括33个样本,胃癌早期样本和正常样本数量分别为15个和18个,余下的342个样本用作训练集,流程(见图 6)。
图 6(Figure 6)
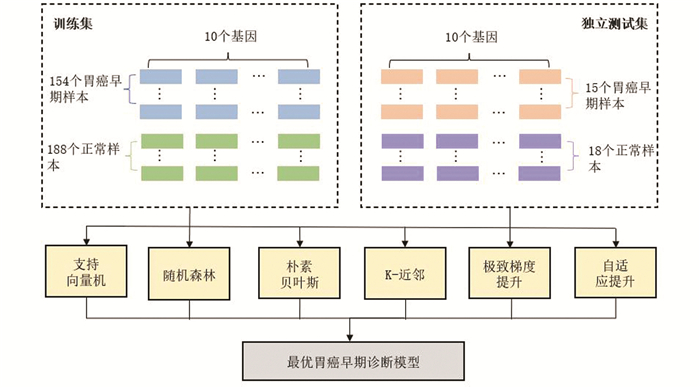
在含有342个样本的训练集上采用十折交叉验证法构建基于SVM、RF、NBM、KNN、XGBoost、AdaBoost 6种算法的诊断预测模型。在训练集中,SVM、RF、NBM、XGBoost、AdaBoost 5种模型均具有十分优秀的表现,各个指标得分均高于0.9,KNN模型表现略微逊色(见表 5)。根据图 7的ROC曲线图可知,各个模型均具有极高的AUC值。
表5(Table 5)

SVM 0.935 6 0.944 1 0.915 8 0.927 5
RF 0.956 1 0.946 5 0.960 8 0.951 5
NBM 0.961 9 0.938 9 0.980 4 0.958 6
KNN 0.894 6 0.965 9 0.797 1 0.865 3
XGBoost 0.967 8 0.969 5 0.960 8 0.963 7
AdaBoost 0.953 2 0.946 5 0.954 2 0.948 1
表 5 6个模型在训练集中的评价指标Table 5 Evaluation indicators of six models on training set
图 7(Figure 7)
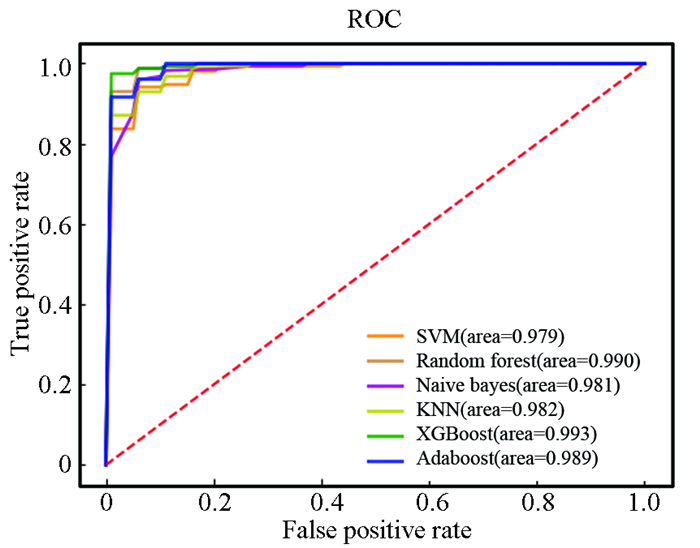
在含有33个样本的独立测试集上对6个模型的预测性能进行验证。据表 6可知各个模型性能均有一定程度的下降。图 8的ROC曲线表明在独立测试集上各个模型仍然具有较高的AUC值。综合6个模型在训练集和独立测试集上的表现,在本研究中,研究性能最出色、鲁棒性最高和泛化能力最好的模型是基于极致梯度提升算法构建的胃癌诊断预测模型。
表6(Table 6)

SVM 0.939 4 0.882 4 1.000 0 0.937 5
RF 0.939 4 0.882 4 1.000 0 0.937 5
NBM 0.909 1 0.833 3 1.000 0 0.909 1
KNN 0.909 1 0.875 0 0.933 3 0.933 2
XGBoost 0.939 4 0.933 3 0.933 3 0.933 3
Adaboost 0.939 4 0.882 4 1.000 0 0.937 5
表 6 6个模型在独立测试集中的评价指标Table 6 Evaluation indicators of six models on independent test set
图 8(Figure 8)
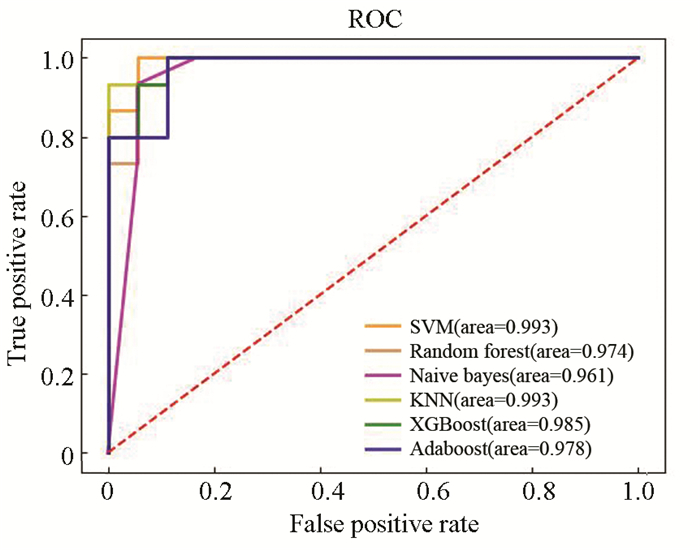
4 讨论通过检索公开数据库收集胃癌基因表达数据信息,利用生物信息学方法进行胃癌早期诊断关键基因的挖掘,最终得到10个关键基因(CXCL11、CCR8、CXCL9、CXCL10、CXCL1、CCL20、CXCL8、CXCL6、APLN、HTR1E)。
Wang H等[22]通过多种生物信息学分析方法发现CXCL11与胃癌肿瘤免疫浸润显著相关,其高表达可以作为胃癌预后和肿瘤浸润的潜在生物标志物,为EBVaGC的免疫治疗提供了新视角。Jie Yi等[23]对TCGA数据库中正常组织及胃癌组织数据进行统计分析,结果表明CCR8在胃癌组织中表达上调,并与胃癌患者的不良生存相关。Zhang C等[24]探索胃癌中程序性死亡配体1(PD-L1)相关基因,体外实验验证阐明CXCL9/10/11-CXCR3通过激活胃癌细胞中的STAT和PI3K-Akt信号通路上调PD-L1的表达。Chen X等[25]利用qPCR分析胃癌标本中CXCL1和CXCL8的表达,认为CXCL1和CXCL8通过与受体CXCR2结合协同参与胃癌细胞增殖、凋亡和迁移过程。相关临床数据表明CXCL1和CXCL8的低表达与胃癌不良预后的特征显著相关,包括AFP水平、肿瘤大小和TNM分期。Chen X等[26]还通过研究CXCL家族与胃癌发展的关系,结论表明CXCL6梯度与B细胞的绝对数相关,CXCL家族在胃癌的发病机制中具有重要作用,可以作为胃癌发展的标志物。
幽门螺杆菌感染相关的慢性炎症是胃癌的主要原因,Yin H等[27]利用TCGA和GEO数据库,分析识别到CCL20为幽门螺杆菌感染相关的胃癌关键差异表达基因。Feng M等[28]采集270名胃癌患者的肿瘤样本和匹配的相邻正常组织,其研究数据表明APLN的表达水平和肿瘤分化、淋巴结和远处转移密切相关,可以用作评估临床特征和预测胃癌患者的预后的标志。腹膜转移(PM)是胃癌治疗手术最常见的失败原因之一,Zhang J等[29]利用差异分析识别到HTR1E为高风险PM患者的关键基因。
Alberto等[30]通过研究从32名胃癌患者的冰冻肿瘤样本获得的基因表达谱数据,利用方差分析和差异表达分析等方法,得到了3个与淋巴结转移风险较高的胃癌关键基因(Bik、aurora kinase B和eIF5A2)。基于关键基因建立逻辑回归诊断预测模型用于预测淋巴结状态,该模型正确预测出32例胃癌患者中30例淋巴结状态,模型准确率为93.75%。该胃癌诊断预测模型为极致梯度提升诊断预测模型,其在训练集和独立测试集准确率分别为96.78%和93.94%,具有较好的预测效果。
5 结论通过生物信息学方法挖掘了胃癌早期诊断的10个关键基因,利用MedCalc软件分析可知,该10个关键基因对正常样本和胃癌早期样本具有较高的分类识别能力,可以作为早期胃癌诊断及研究的靶点。
本文特色之处在于基于关键基因的表达数据,通过分析多种机器学习算法,实现了诊断预测模型的构建,并最终选择了XGBoost诊断预测模型为最优模型。该模型在训练集和独立测试集上的具有最好的综合性能,可以作为一种无创性检查早期胃癌的手段,具有良好的应用前景。通过筛选关键基因构建了早期胃癌诊断预测模型,为提高胃癌早期诊断的研究提供了新的思路和方法。本研究不足之处在于对胃癌发生机制的研究不够深入全面,转录组学数据的分析并不能完全阐释机体总体变化;此外,本文研究内容仅为生物信息学诊断预测层面,缺少体内或体外实验支撑。在后续研究中,要加强与生物实验相结合,开发出更加实用、更加准确地胃癌早期诊断预测模型。
参考文献
[1] MALIHA S K, EMA R R, GHOSH S K, et al. Cancer disease prediction using naive bayes, k-nearest neighbor and J48 algorithm[C]//Proceedings of the 2019 10th International Conference on Computing, Communication and Networking Technologies (ICCCNT). Kanpur: IEEE, 2019: 1-7. DOI: 10.1109/ICCCNT45670.2019.8944686. (

[2] 樊祥山, 陈杰, 薛卫成. 基于癌组织的生物标志物病理学检测有助于进展期胃癌患者个体化精准诊治[J]. 中华病理学杂志, 2021, 50(2): 84-89.
FAN Xiangshan, CHEN Jie, XUE Weicheng. Accurate diagnosis and individualized treatment of advanced gastric cancer: pathological detection of biomarkers based on cancer tis sue samples[J]. Chinese Journal of Pathology, 2021, 50(2): 84-89. DOI:10.3760/cma.j.cn112151-20200701-00517 (

[3] 郑荣寿, 孙可欣, 张思维, 等. 2015年中国恶性肿瘤流行情况分析[J]. 中华肿瘤杂志, 2019(1): 19-28.
ZHENG Rongshou, SUN Kexin, ZHANG Siwei, et al. Report of cancer epidemiology in China, 2015[J]. Chinese Journal of Oncology, 2019(1): 19-28. DOI:10.3760/cma.j.issn.0253-3766.2019.01.005 (

[4] JIANG K, LIU H, XIE D, et al. Differentially expressed genes ASPN, COL1A1, FN1, VCAN and MUC5AC are potential prognostic biomarkers for gastric cancer[J]. Oncology Letters, 2019, 17(3): 3191-3202. DOI:10.3892/ol.2019.9952 (

[5] CHEN J, WANG X, HU B, et al. Candidate genes in gastric cancer identified by constructing a weighted gene co-expression network[J]. PeerJ, 2018, 6: e4692. DOI:10.7717/peerj.4692 (

[6] SETTINO M, CANNATARO M. Survey of main tools for querying and analyzing TCGA Data[C]//Proceedings of the 2018 IEEE International Conference on Bioinformatics and Biomedicine (BIBM). Madrid: IEEE, 2018 : 1711-1718. DOI: 10.1109/BIBM.2018.8621270. (

[7] CARITHERS L J, MOORE H M. The genotype-tissue expression (GTEx) project[J]. Biopreservation and Biobanking, 2015, 13(5): 580-585. DOI:10.1038/ng.2653 (

[8] GOH W W B, WANG W, WONG L. Why batch effects matter in omics data, and how to avoid them[J]. Trends in Biotechnology, 2017, 35(6): 498-507. DOI:10.1016/j.tibtech.2017.02.012 (

[9] LOVE M I, HUBER W, ANDERS S. Moderated estimation of fold change and dispersion for RNA-seq data with DESeq2[J]. Genome Biology, 2014, 15(12): 550. DOI:10.1186/s13059-014-0550-8 (

[10] SZKLARCZYK D, GABLE A L, NASTOU K C, et al. The STRING database in 2021: customizable protein-protein networks, and functional characterization of user-uploaded gene/measurement sets[J]. Nucleic Acids Research, 2021, 49(D1): D605-D612. DOI:10.1093/nar/gkaa1074 (

[11] OBUCHOWSKI N A, BULLEN J A. Receiver operating characteristic (ROC) curves: review of methods with applications in diagnostic medicine[J]. Physics in Medicine and Biology, 2018, 63(7): 07TR01. DOI:10.1088/1361-6560/aab4b1 (

[12] GHADDAR B, NAOUM-SAWAYA J. High dimensional data classification and feature selection using support vector machines[J]. European Journal of Operational Research, 2018, 265(3): 993-1004. DOI:10.1016/j.ejor.2017.08.040 (

[13] QI Y. Random forest for bioinformatics[J]. Ensemble Machine Learning, 2012, 307-323. DOI:10.1007/978-1-4419-9326-7_11 (

[14] YU J, PING P, WANG L, et al. A novel probability model for lncRNA-disease association prediction based on the na?ve bayesian classifier[J]. Genes, 2018, 9(7): 345. DOI:10.3390/genes9070345 (

[15] OKFALISA, GAZALBA I, MUSTAKIM, et al. Comparative analysis of k-nearest neighbor and modified k-nearest neighbor algorithm for data classificatio[C]//Proceedings of the 2017 2nd International conferences on Information Technology, Information Systems and Electrical Engineering (ICITISEE). Yogyakarta: IEEE, 2017: 294-298. DOI: 10.1109/ICITISEE.2017.8285514. (

[16] OGUNLEYE A, WANG Q G. XGBoost model for chronic kidney disease diagnosis[J]. IEEE/ACM Transactions on Computational Biology and Bioinformatics, 2020, 17(6): 2131-2140. DOI:10.1109/tcbb.2019.2911071 (

[17] CHOKKA A, RANI K S. AdaBoost with feature selection using iot to bring the paths for somatic mutations evaluation in cancer[J]. Internet of Things and Personalized Healthcare Systems, 2019, 51-63. DOI:10.1007/978-981-13-0866-6_5 (

[18] JING X, PENG W, CHEN Y, et al. A novel deep flexible neural forest model for classification of cancer subtypes based on gene expression data[J]. IEEE Access, 2019, 7: 22086-22095. DOI:10.1109/ACCESS.2019.2898723 (

[19] LI Y, WU J, WU Q. Classification of breast cancer histology images using multi-size and discriminative patches based on deep learning[J]. IEEE Access, 2019, 7: 21400-21408. DOI:10.1109/ACCESS.2019.2898044 (

[20] JIANG X, LI J, KAN Y, et al. MRI based radiomics approach with deep learning for prediction of vessel invasion in early-stage cervical cancer[J]. IEEE/ACM Transactions on Computational Biology and Bioinformatics, 2021, 18(3): 995-1002. DOI:10.1109/tcbb.2019.2963867 (

[21] CAELEN O. A Bayesian interpretation of the confusion matrix[J]. Annals of Mathematics and Artificial Intelligence, 2017, 81(3/4): 1-22. DOI:10.1007/s10472-017-9564-8 (

[22] WANG H, ZHOU L, YANG Y, et al. Screening and identification of key genes in EBV-associated gastric cancer based on bioinformatics analysis[J]. Pathology Research and Practice, 2021, 222(4): 153439. DOI:10.1016/j.prp.2021.153439 (

[23] YI J, JIANG S J. Dysregulation of CCL18/CCR8 axis predicts poor prognosis in patients with gastric cancer[J]. European Journal of Inflammation, 2018, 16. DOI:10.1177/2058739218796887 (

[24] ZHANG C, LI Z, XU L, et al. CXCL9/10/11, a regulator of PD-L1 expression in gastric cancer[J]. BMC Cancer, 2018, 18(1): 462. DOI:10.1186/s12885-018-4384-8 (

[25] CHEN X, JIN R, CHEN R, et al. Complementary action of CXCL1 and CXCL8 in pathogenesis of gastric carcinoma[J]. International Journal of Clinical and Experimental Pathology, 2018, 11(2): 1036-1045. (

[26] CHEN X, CHEN R, JIN R, et al. The role of CXCL chemokine family in the development and progression of gastric cancer[J]. International Journal of Clinical and Experimental Pathology, 2020, 13(3): 484-492. (

[27] YIN H, CHU A, LIU S, et al. Identification of DEGs and transcription factors involved in H. pylori-associated inflammation and their relevance with gastric cancer[J]. PERRJ, 2020, 8(S1): e9223. DOI:10.7717/peerj.9223 (

[28] FENG M, YAO G, YU H, et al. Tumor apelin, not serum apelin, is associated with the clinical features and prognosis of gastric cancer[J]. BMC Cancer, 2016, 16(1): 794. DOI:10.1186/s12885-016-2815-y (

[29] ZHANG J, HUANG J Y, CHEN Y N, et al. Whole genome and transcriptome sequencing of matched primary and peritoneal metastatic gastric carcinoma[J]. Scientific Reports, 2015, 5: 13750. DOI:10.1038/srep13750 (

[30] ALBERTO M, SIMONE M, CLAUDIO B, et al. Gene expression profile of primary gastric cancer: towards the prediction of lymph node status[J]. Annals of Surgical Oncology, 2006. DOI:10.1245/s10434-006-9090-0 (
