
Corresponding authors: * E-mail:zhaomingmingsp@163.com
Received:2019-04-9Revised:2019-04-29Online:2019-09-1

Abstract
Keywords:
PDF (3023KB)MetadataMetricsRelated articlesExportEndNote|Ris|BibtexFavorite
Cite this article
Ze Zhao. Comparison Between $\chi^2$ and Bayesian Statistics with Considering the Redshift Dependence of Stretch and Color from JLA Data. [J], 2019, 71(9): 1097-1108 doi:10.1088/0253-6102/71/9/1097
1 Introduction
Type Ia supernova (SN Ia) is one type of supernova, which occurs from a terrific explosion of white dwarf star in binary systems.[1] SN Ia is named as the cosmological standard candle which can measure the history of the expansive universe.[2]-[3] For this reason, SN Ia has become one of the most effective observation to explore the essence of dark energy (DE).[4]-[12] Several supernova (SN) datasets have been released in recent years, such as “SNLS”,[13] “Union”,[14] “Constitution”,[15]-[16] “SDSS”,[17] “Union2”,[18] “SNLS3”,[19] and “Union2.1”.[20] “Joint Light-curve Analysis” (JLA) dataset is the latest SN sample,[21] which consists of 740 supernovae (SNe). And in the process of cosmology-fits, stretch-luminosity parameter $\alpha$ and color-luminosity parameter $\beta$ are two important quantities of SN Ia which are treated as model-free parameters.[19]-[21] The aim of considering $\alpha$ and $\beta$ is to reduce systematic uncertainties of SN Ia.The possibility of the evolution of $\alpha$ and $\beta$ with redshift $z$ is one of the most important factors for controlling of the systematic uncertainties. So far, there is still no credible evidence in the past study for the evolution of $\alpha(z)$. On the other hand, the redshift-dependence of $\beta$ has been supported by several SN datasets including Union2.1,[22] SNLS3,[23] and JLA.[24] Reference [23] states that $\beta$ deviates from a constant at 6$\sigma$ confidence level (CL) based on the SNLS3 samples. And by applying a linear $\beta$ on studying various DE and modified gravity models,[25]-[28] the evolution of $\beta$ has effects on parameter estimation, and assuming $\beta$ as a time-varying form depress the tension between SN Ia and other cosmological observations. And also, Reference [29] found $\beta$ at high redshift has a clear decreasing trend, at about $3.5\sigma$ CL.
Recently, Reference [30] have performed a Bayesian inference method for the JLA dataset using Bayesian graphical models to derive the full posterior distribution of fitting model parameters. Compared with the old $\chi^2$ statistics, Bayesian statistics is a different statistical approach, which gives a statistically significant shift of the SN standard-candle corrections toward lower (absolute value). $\chi^2$ fit does not fully propagate the parameter dependence of the covariance. In contrast, Bayesian inference gives a fully account for the standard-candle parameter dependence of the data covariance matrix.
In this work, we adopt Bayesian statistics on the analysis of redshift dependence of stretch and color by using redshift tomography. Redshift tomography method has been widely applied in the study of numerical cosmology.[31]-[33] The basic thought of this method is dividing the SN data into several redshift bins, and assuming $\alpha$ and $\beta$ as piecewise constants in each bin. By using this method, we constrain $\Lambda$-cold-dark-matter ($\Lambda$CDM) model. And also, in each bin we check the reliability of cosmology-fit results. One of advantages by adopting the redshift tomography method is a reduction of statistical significance. Reference [30] adopt Bayesian statistical method on JLA data, and they only assume that $\alpha$ and $\beta$ are constants. But we find from the results given by Bayesian statistics, $\alpha$ and $\beta$ show a tendency of linear evolution with redshift $z$. Therefore, we further explore the possible evolution of $\alpha$ and $\beta$ by taking linear parametrization of $\alpha$ and $\beta$. And we make a comparison between results of Bayesian statistics and $\chi^2$ statistics. Furthermore, we consider the impacts of linear parametrization of $\alpha$ and $\beta$ for CPL, JBP, BA and Wang models. For further study on model-independent parameterizations, see Ref. [34]. Figure of merit (FoM) is also calculated for these 4 models based on the combined JLA+BAO+GC data. And based on the JLA data only and the combined JLA+CMB+GC data, we also compare the values of fractional matter density $\Omega_{m0}$ given by three cases: (i) $\chi^2$ statistics with constant $\alpha$ and $\beta$. (ii) Bayesian statistics with constant $\alpha$ and $\beta$. (iii) Bayesian statistics with linear $\alpha$ and $\beta$. Moreover, to discuss the fate of the universe, we present the evolution of deceleration parameter $q(z)$ for four models given by two statistical methods.
We state our method in Sec. 2, show our results in Sec. 3, and summarize in Sec. 4.
2 Methodology
2.1 Theoretical Model
The comoving distance $r(z)$ in a flat universe satisfiesiswhere $H_0$ is the current Hubble constant and $c$ is the speed of light. $E(z)$ represents the reduced Hubble parameter given by
Here $\Omega_{r0}$, $\Omega_{m0}$ and $\Omega_{de0}$ are the present fractional densities of radiation, matter and dark energy, respectively. And $X(z)$ is the DE density function, which is given by
where $w$ represents the equation of state (EoS) of DE, that is one of determinative elements for the properties of DE.And specially, $X=1$, for the standard $\Lambda$-cold-dark-matter ($\Lambda$CDM) model.
To analyse the evolution of $\alpha(z)$ and $\beta(z)$ for different models, we consider four important parametrization models:
(i) CPL model[35]-[36] has a dynamical EoS $w(z) = w_{0} + w_{a}{z}/({1+z})$, thus $X(z)$ satisfies
(ii) JBP model[37]-[38] has a dynamical EoS $w(z) = w_{0} + w_{a}{z}/{(1+z)^2}$, thus $X(z)$ satisfies
(iii) BA model[39] has a dynamical EoS $w(z) = w_{0} + w_{a}{z(1+z)}/[{1+z^2}]$, thus $X(z)$ satisfies
(iv) Wang model[40] has a dynamical EoS $w(z) = w_{0}({1-2z})/({1+z}) + w_{a}{z}/{(1+z)^2}$, thus $X(z)$ satisfies
Parameter $w_0$ describes the current value of EoS, and $w_a$ reflects the evolution of EoS for each model. We also make use of the deceleration parameter $q\equiv -{\ddot{a}}/{aH^{2}}$ to investigate the evolutionary behavior of cosmic acceleration (CA).
Figure of merit (FoM) was firstly proposed for the purpose of comparing different DE experiments. Making use of the CPL model, Dark Energy Task Force (DETF) constructs a quantitative expression of FoM, which is inversely proportional to the area enclosed by the $95%$ CL contour in the $(w_0, w_a)$ plane:[41]
Here
$$ \sigma(w_p)=w_0-w_a{\langle\delta w_0\delta w_a\rangle/}\langle{\delta w_a}^2\rangle\,, \quad \sigma(w_i)=\sqrt{\langle{\delta w_i}^2\rangle}\,. $$
Due to the relation $\sigma(w_a)\sigma(w_p)=\sqrt{\rm {detCov}(w_0, w_a)}$, there is no need to calculate the conversion of $w_p$ for the FoM. Evidently, larger $\rm FoM$ demonstrates better accuracy. In this paper, we apply the DETF version of FoM on CPL, JBP, BA and Wang models to compare $\chi^2$ statistic with Bayesian statistic.
2.2 Observational Data
In this section, we firstly show the process of calculating the $\chi^2$ function of JLA data for both $\chi^2$ and Bayesian statistics. Then, we describe the details of the redshift tomography method.In a flat universe, the distance modulus ${\upsilon}_{\rm th}$ can be calculated as
here $z_{\rm cmb}$ and $z_{\rm hel}$ represent the cosmic microwave background (CMB) restframe and heliocentric redshifts of SN.The luminosity distance ${d}_{L}$ can be written as
The observation of distance modulus ${\upsilon}_{\rm obs}$ can be calculated by a empirical linear expression:
in which, $m_{B}^{\star}$ represents the observed peak magnitude of the $B$ band rest-frame, $X_1$ and ${\cal C}$ determine the time stretching of light-curve and the supernova color at maximum brightness respectively. $M_B$ represents the absolute B-band magnitude, that depends on properties of the host galaxy.[42]-[43] It should be pointed out that $M_B$ has relation with the host stellar mass ($M_{\rm stellar}$) given by a step function[21]
$M_{\odot}$ is the mass of sun.
The $\chi^2$ of JLA data is calculated by the expression
where $\Delta \upsilon\equiv \upsilon_{\rm obs}-\upsilon_{\rm th}$ is the data vector. $C$ is the total covariance matrix, which is given by
${ D}_{\rm stat}$ is the diagonal part of the statistical uncertainty, which is given by[21]
where $\sigma^2_{z,i}$ describes the uncertainty for peculiar velocities in redshift, $\sigma^2_{\rm int}$ denotes the intrinsic variation in SN magnitude and $\sigma^2_{\rm lensing}$ accounts for the variation of magnitudes induced by gravitational lensing. $\sigma^2_{m_{B},i}$, $\sigma^2_{X_1,i}$, and $\sigma^2_{{\cal C},i}$ represent the uncertainties of $m_{B}$, $X_1$ and ${\cal C}$ for the $i$-th SN. Moreover, $C_{m_{B} X_1,i}$, $C_{m_{B} {\cal C},i}$, and $C_{X_1 {\cal C},i}$ are the covariances among $m_{B}$, $X_1$ and ${\cal C}$ for the $i$-th SN. ${ C}_{\rm stat}$ and ${ C}_{\rm sys}$ represent the statistical and the systematic covariance matrices respectively, which satisfies
JLA group (http://supernovae.in2p3.fr/sdss$_{-}$snls$_{-}$ jla/Re adMe.html) introduces matrices $V_0$, $V_{a}$, $V_{b}$, $V_{0a}$, $V_{0b}$ and $V_{ab}$.And also Ref. [21] gives a detailed discussions towards to JLA SN sample.
For the methodology of Bayesian statistics,[30] the posterior probability is defined in Bayesian statistics as
where ${\upsilon}$ is a distance modulus data vector with covariance matrix ${C}$, ${\vartheta}$ is a parameter vector for a specific theoretical model, distance modulus ${\upsilon}_t = f_{{t}}({\vartheta})$ is a deterministic function predicted by ${\vartheta}$. The term $P({\vartheta}, {\upsilon}_t, {\upsilon}, {C})$ represents the joint probability distribution that can be written as:
by applying the chain rule of conditional probabilities.
Marginal probability is the integral of $ P({\vartheta}, {\upsilon}_1, {\upsilon}, {C})$ with respect to ${\upsilon}_t$:
$Z({\upsilon}, {C})$ is a normalization constant defined by $ Z({\upsilon}, {C})\equiv P({\upsilon}, {C}) / P({C}) = P({{\upsilon}}|{{C}})$. $Z({\upsilon}, {C})$ is deterministic in model selection which is named by Bayesian evidence. Gaussian likelihood function $\mathcal{L}({\vartheta}; {\upsilon}, {C})$ can be written as
Take the logarithm of Eq. (19) and we have
here
is the chi-square of the $\chi^2$ statistics. Evidence variables ${C}$ and ${\upsilon}$ in Eq. (19) is fixed by the dataset. After this consideration, Eq. (19) turns to a function of ${\vartheta}$ which is easily evaluated. For brevity, in this paper we will not make notational distinction of conditioning variables and their realized values in the equations.
The likelihood up to a normalization for $\chi^2$ and Bayesian statistics can be calculated respectively as
As pointed out in Ref. [30], compared with the Bayesian statistics, $\chi^2$ approach shifts the standardization parameters away from the ideal standard candle case markedly. The standardization parameter given by $\chi^2$ statistics also has larger values. As a matter of fact, $\ln \mathcal{L} \to \ln \mathcal{L}_{\rm Bayesian} = \ln \mathcal{L} - ({1}/{2}) \ln \det { C}$ results in a distortion to the uniform prior of $\alpha$ and $\beta$ to a certain extent .
It should be pointed out that, we marginalize the Hubble constant $H_0$ and the absolute B-band magnitude $M_B$ according during the process of calculating $\ln \mathcal{L}$. Readers can check the details of marginalized process and the code related to JLA likelihood from Ref. [21] As stated above, we aim at studying the possible evolution of $\alpha$ and $\beta$ SN by adopting a model-independent method. Therefore we apply the redshift tomography method in this paper. The essential thought of redshift tomography is to split whole SN sample in several redshift bins. assuming that both $\alpha$ and $\beta$ are are piecewise constants. By constraining the $\Lambda$CDM model, we check the consistency of cosmology-fit results given by the SN sample of each redshift bin. Furthermore, in order to make results insensitive to the details of redshift to mography, in this work we divide the JLA data sample at redshift region [0,1] uniformly into 3 bins, 4 bins, and 5 bins. In each bin, $\alpha$ and $\beta$ are assumed as piecewise constants in the fitting process. Based on different statistical methods including $\chi^2$ and Bayesian statistics, We consider both $\alpha$ and $\beta$ are constants and also adopting linear parametrization of $\alpha$ and $\beta$. We plot these related results together in order to compare the fitting results from different cases and data. In this work we use Markov Chain Monte Carlo (MCMC) methods to constrain parameters and analysis data by the assist of the CosmoMC package. For the details of this package, see Ref. [44]
3 Results
We compare the Bayesian and $\chi^2$ statistics for the evolution of JLA luminosity parameters $\alpha$ and $\beta$. In Subsec. 3.1, for the $\Lambda$CDM model, we revisit in the evolution of $\alpha$ and $\beta$ using redshift tomography. In Subsec. {3.2}, we investigate the impacts of linear parametrization of $\alpha$ and $\beta$ on the parameter estimation, and compare with the results of Subsec. 3.1. And we further verify impacts of linear parametrization of $\alpha$ and $\beta$ on different models including CPL, BA, JBP and Wang models. In Subsec. 3.3, we also compare the values of fractional matter density $\Omega_{m0}$ given by three cases: (i) $\chi^2$ statistics with constant $\alpha$ and $\beta$. (ii) Bayesian statistics with constant $\alpha$ and $\beta$. (iii) Bayesian statistics with linear $\alpha$ and $\beta$. And results in this section are based on the JLA data only and the combined JLA+CMB+GC data. In Subsec. 3.4, we further discuss the fate of universe by investigate the evolution of the deceleration parameter for both statistical methods by using JLA and JLA+CMB+GC data.3.1 Revisit in the Evolution of $\alpha$ and $\beta$ Using Redshift Tomography
The major results in this section is comparing the evolution behaviors of JLA luminosity parameters $\alpha$ and $\beta$ given by different statistical methods.In Fig. 1, we present the 1$\sigma$ confidence regions of stretch-luminosity parameter $\alpha$ obtained from $\chi^2$ statistics (a)—(c) and Bayesian statistics (d)—(f). From left panels given by $\chi^2$ statistics, apparently for the SN samples of 3 bin the 1$\sigma$ regions of $\alpha$ consist with a constant. This conclusion is compliant with all the cases included 3 bins, 4 bins and 5 bins, which is also in compliance with the results in other paper.[22]-[24],[33] In contrast, for right panels given by Bayesian statistics, the results from SN samples of 3, 4 and 5 bins have a significant trend of decreasing at high redshift. Therefore, by using Bayesian statistics, we find the evidence for the evolution of $\alpha$ with $z$, which is significantly different with previous value obtained from $\chi^2$ statistics (see e.g. Ref. [29]). although it is consistent with a constant at low redshift. And meanwhile, Bayesian statistics reduce the error bar of $\alpha$ compare to $\chi^2$ statistics. Specifically, $\alpha$ For the case of 3 bins, the 1$\sigma$ upper frontier of $\alpha$ in the third bin deviates 1.5$\sigma$ CL from the results obtained from the full JLA sample. For the case of 4 bins, the 1$\sigma$ upper frontier of $\alpha$ in the forth bin deviates 3.8$\sigma$ CL from the value obtained from full JLA sample. For the case of 5 bins, the 1$\sigma$ upper frontier of $\alpha$ in the fifth bin deviates 4.1$\sigma$ CL from the value obtained from the full JLA sample. (see Table 1). These deviations imply at high redshift, there is more than $1.5\sigma$ CL evidence for the decline of $\alpha$. It should be pointed out this conclusion is not sensitive to the methods of redshift tomography.[29]
Fig. 1
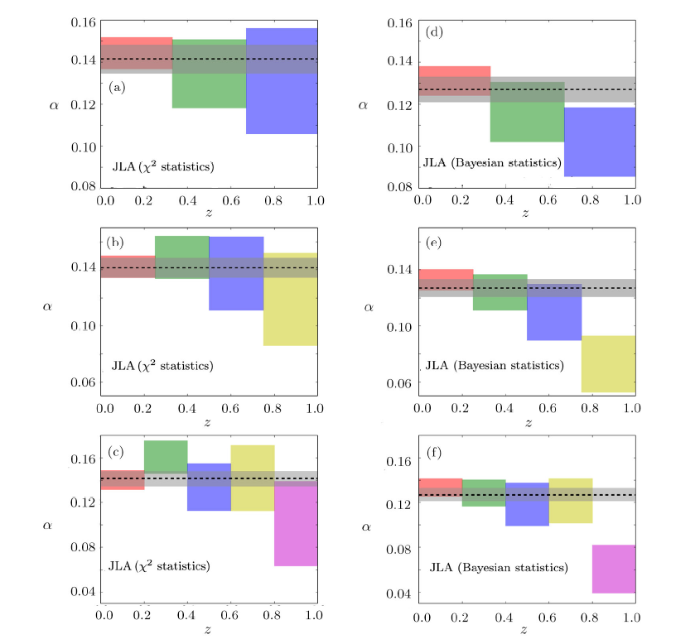
Fig. 1(Color online) The 1$\sigma$ confidence regions of stretch-luminosity parameter $\alpha(z)$ obtained from $\chi^2$ statistics (a)—(c) and Bayesian statistics (d)—(f). for $\Lambda$CDM The results only consider the $\Lambda$CDM model, based on by using the full JLA samples at redshift zone [0,1]. And 3 bins, 4 bins, and 5 bins are shown in the upper, middle and lower panel. which are divided into 3 bins (upper panels), 4 bins (middle panels), and 5 bins (lower panels). in which the absolute B-band magnitude $M_B$ are marginalized. The gray zone represents the 1$\sigma$ region and the gray dashed line denotes the best-fit result given by the full JLA data.
Table 1
Table 1
![]() |
New window|CSV
In Fig. 2, we present the 1$\sigma$ confidence regions of stretch-luminosity parameter $\beta$ given by $\chi^2$ statistics (a)—(c) and Bayesian statistics (d)—(f). For all left panels given by $\chi^2$ statistics, there is a clear deviation from the results of full JLA sample at high redshift between the results obtained from SN samples of several bins Specifically, $\beta$ has a significant trend of decline at high redshift. Although at low redshift, it consists with a constant. For all right panels given by Bayesian statistics, the decreasing tendency of $\beta$ is more clear than the results given by $\chi^2$ statistics. And meanwhile, Bayesian statistics reduce the error bar of $\beta$ compare to $\chi^2$ statistics. In details, There is a clear deviation at high redshift between the results given by SN samples of several bins from the results of full JLA sample. for the case of 3 bins, the 1$\sigma$ upper frontier of $\beta$ in the third bin deviates 19$\sigma$ CL from the value obtained from the full JLA sample. For the case of 4 bins, the 1$\sigma$ upper frontier of $\beta$ in the forth bin deviates 24.4$\sigma$ CL from the value obtained from the full JLA sample. For the case of 5 bins, the 1$\sigma$ upper frontier of $\beta$ in the fifth bin deviates 25.9$\sigma$ CL from the value obtained from the full JLA sample. (see Table 1). These deviations imply at high redshift, there is more than $19\sigma$ CL evidence for the decline of $\beta$. And it is also not sensitive to the methods of redshift tomography.
Fig. 2
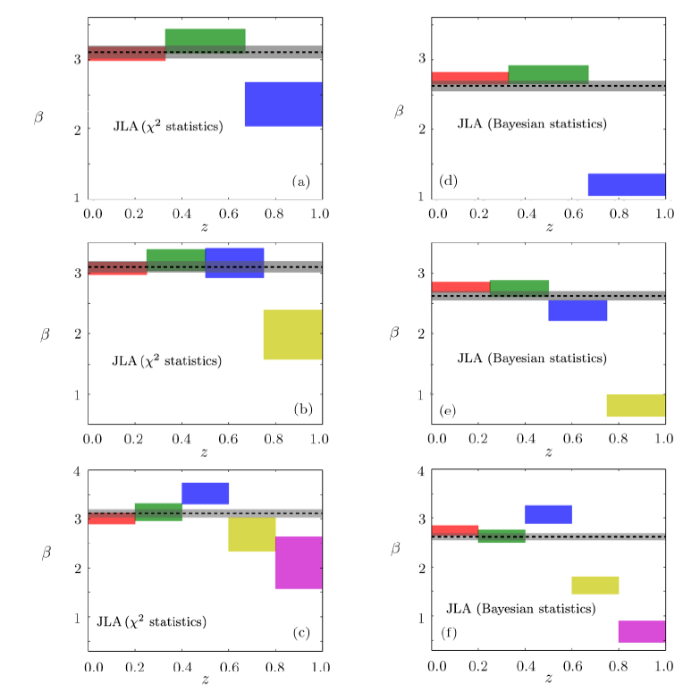
Fig. 2(Color online) The 1$\sigma$ confidence regions of stretch-luminosity parameter $\beta(z)$ obtained from $\chi^2$ statistics (a)—(c) and Bayesian statistics (d)—(f).for $\Lambda$CDM The results only consider the $\Lambda$CDM model by using the full JLA samples at redshift region [0,1]. And 3 bins, 4 bins and 5 bins are shown in the upper, middle, and lower panel. The gray zone represents the 1$\sigma$ region and the gray dashed line denotes the best-fit result given by the full JLA data.
For both the results of $\alpha$ and $\beta$, Bayesian statistics effectively reduce the error bar. This is because the $\chi^2$ fit does not fully propagate the parameter dependence of the covariance which contributes with a $\ln \det { C}$ term in the parameter posterior. From the decreasing tendency for the results given Bayesian statistics, it is necessary to consider the linear parametrization of $\alpha$ and $\beta$ (see Subsec. {3.2}).
3.2 A Closer Investigation on the Impacts of Linear Parametrization for $\alpha$ and $\beta$
In this section, we consider introducing linear parametrization of $\alpha$ and $\beta$: $\alpha{(z)}=\alpha_0+\alpha_1z$ and $\beta{(z)}=\beta_0+\beta_1z$.Table 1 presents the cosmology-fits results for the $\Lambda$CDM model obtained from $\chi^2$ statistics with constant $\alpha$, $\beta$, $\chi^2$ statistics with linear $\alpha$ and $\beta$, Bayesian statistics with constant $\alpha$, $\beta$ and Bayesian statistics with linear $\alpha$ and $\beta$. From this table we can see that, the best-fit of the $\alpha_1$ and $\beta_1$ from $\chi^2$ statistics is much closer to zero than Bayesian results, which is also can be seen in Fig. 3. For each statistical methods, taking linear parametrization of $\alpha$ and $\beta$ leads to a larger $\Omega_{m0}$ than the case of constant $\alpha$ and $\beta$. For the case of constant $\alpha$, $\beta$ and the case of linear $\alpha$ and $\beta$, adopting Bayesian statistics will also yield a larger $\Omega_{m0}$ compared with $\chi^2$ statistics.
Fig. 3
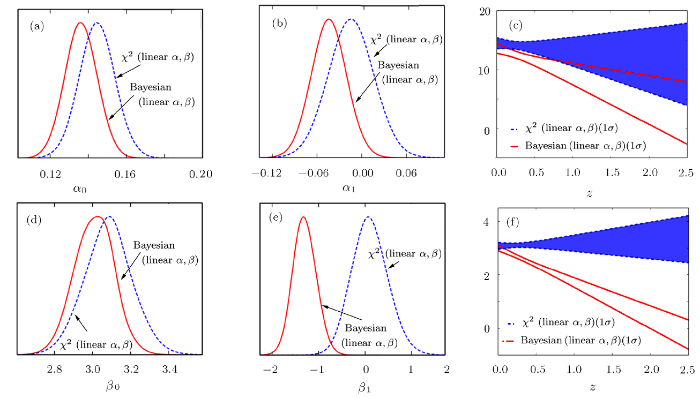
Fig. 3(Color online) The 1D marginalized probability distributions of $\alpha_0$, $\alpha_1$, $\beta_0$, $\beta_1$ and the evolution of $\alpha(z)$, $\beta(z)$ given by $\chi^2$ statistics (blue dashed line) and Bayesian statistics (red solid line). Note that, we consider linear parametrization: $\alpha{(z)}=\alpha_0+\alpha_1z$ and $\beta{(z)}=\beta_0+\beta_1z$ for $\Lambda$CDM based on the full JLA sample.
In Fig. 3, we present the 1D marginalized probability distributions of $\alpha_0$, $\alpha_1$, $\beta_0$, $\beta_1$ and the evolution of $\alpha(z)$, $\beta(z)$ for the two statistical methods. Based on the full JLA sample, we consider the linear parametrization of $\alpha$ and $\beta$ for the $\Lambda$CDM model. For $\chi^2$ statistics, the center of the probability distributions for $\alpha_1$ and $\beta_1$ is close to zero (see Table 1), which means, $\chi^2$ statistics tends to the case that $\alpha$ and $\beta$ are constant: $\alpha(z)=\alpha_0$ and $\beta(z)=\beta_0$. As for the Bayesian statistics, the center of the probability distributions for both $\alpha_1$ and $\beta_1$ show clear deviation from zero compared to results from $\chi^2$ statistics. Which means, Bayesian statistics show much tendency of linear $\alpha$ and $\beta$ with respect to redshift $z$ than $\chi^2$ statistics. And the evolution of $\alpha(z)$, $\beta(z)$ Figs. 3(e) and 3(f) also confirm the same evolutionary trend: the result of $\chi^2$ statistics with linear $\alpha$ and $\beta$ is consist with a constant; the result of Bayesian statistics with linear $\alpha$ and $\beta$ show clear decreasing tendency which is not consist with a constant.
Figure 4 is the evolution of linear $\alpha(z)$ for CPL, JBP, BA and Wang models given by $\chi^2$ statistics (a)—(d) and Bayesian statistics (e)—(h).Once adopting $\chi^2$ statistics, the 1$\sigma$ region of $\alpha$ for the case of constant $\alpha$ and $\beta$ locates entirely in the 1$\sigma$ region corresponds to linear $\alpha$ and $\beta$. For the Bayesian statistics, the 1$\sigma$ region for the case of linear $\alpha$ and $\beta$ show clear deviation from the region corresponds to the constant $\alpha$ and $\beta$ at high redshift region. The decreasing tendency consists with the results of the $\Lambda$CDM model (lower panels of Figs. 3).
Fig. 4
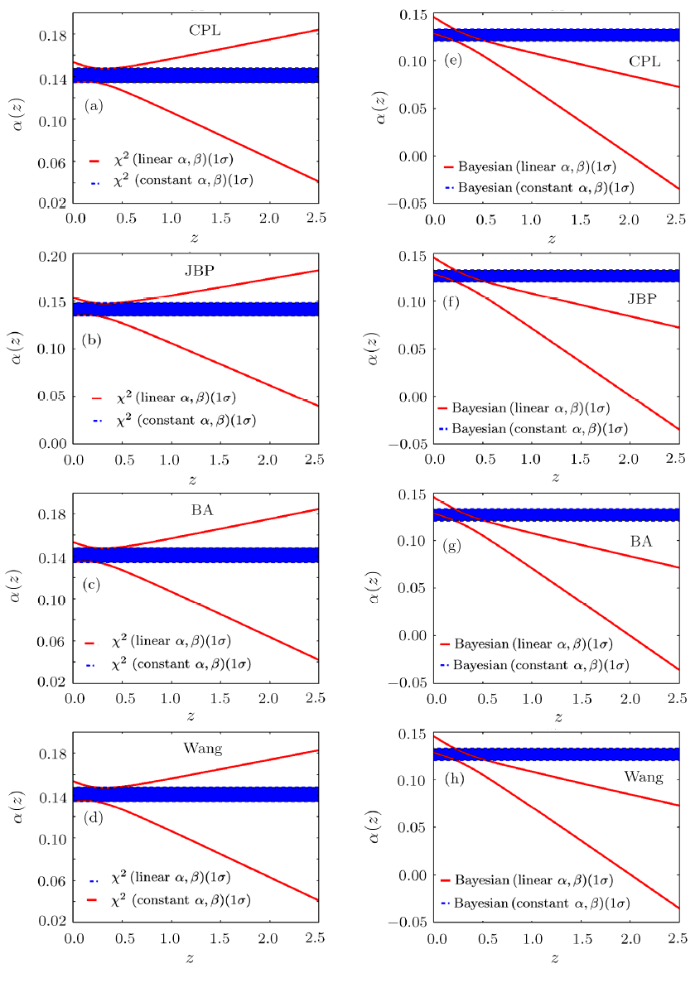
Fig. 4(Color online) The evolution of $\beta(z)$ for CPL, JBP, BA and Wang models obtained from $\chi^2$ statistics (a)—(d) and Bayesian statistics (e)—(h).The results based on the full JLA samples at redshift region [0,1]. The blue region denotes the 1$\sigma$ confidence region of $\alpha$ for the case of constant $\alpha$ and $\beta$. The red solid line corresponds to the 1$\sigma$ boundary for the case of linear $\alpha$ and $\beta$.
In Fig. 5, we plot the evolution of linear $\beta(z)$ for CPL, JBP, BA, and Wang models obtained from $\chi^2$ statistics (a)—(d) and Bayesian statistics (e)—(h). For $\chi^2$ statistics, the 1$\sigma$ region of $\beta$ for the case of constant $\alpha$ and $\beta$ locates entirely in the 1$\sigma$ region corresponds to linear $\alpha$ and $\beta$. Based on the Bayesian statistics, the 1$\sigma$ region for the case of linear $\alpha$ and $\beta$ show clear deviation from the region corresponds to the constant $\alpha$ and $\beta$ at high redshift region. Compared with the evolution of $\alpha$ in Figs. 4(a)—4(d), the deviation of $\beta$ is more evident and the overlapping region at low redshift is more tiny. The decreasing tendency consists with the results of the $\Lambda$CDM model Figs. 3(e) and 3(f).
Fig. 5
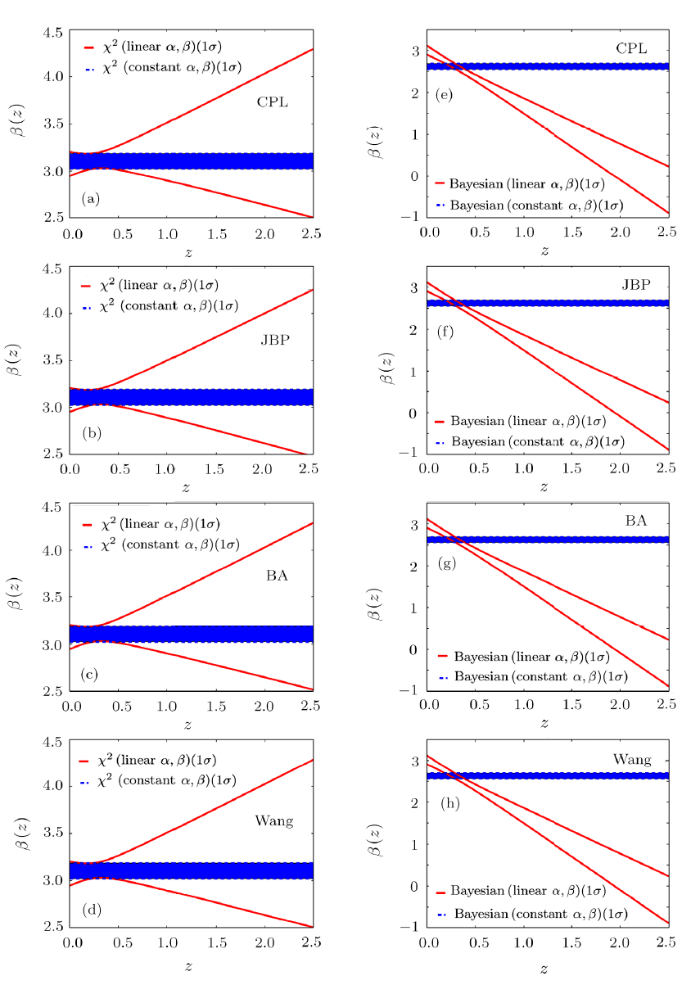
Fig. 5(Color online) The evolution of $\alpha(z)$ for CPL, JBP, BA and Wang models obtained from $\chi^2$ statistics (a)—(d) and Bayesian statistics (e)—(h). The results based on the full JLA samples at redshift region [0,1]. The blue region denotes the 1$\sigma$ confidence region of $\alpha$ for the case of constant $\alpha$ and $\beta$. The red solid line corresponds to the 1$\sigma$ boundary for the case of linear $\alpha$ and $\beta$.
It should be mentioned that, for these two statistical methods, the results of $\alpha(z)$ and $\beta(z)$ for CPL, JBP, BA and Wang models are very close, which is consistent with the model-independent methods we used. which means, the evolution of $\alpha(z)$ and $\beta(z)$ are not depend on models.
3.3 Comparison of $\Omega_{m0}$ Between $\chi^2$ and Bayesian Statistics for CPL, JBP, BA and Wang Models
As shown in Fig. 6, we present the 1D marginalized probability distributions of $\Omega_{m0}$ for CPL, JBP, BA and Wang models given by different statistical methods. We apply $\chi^2$ statistics with constant $\alpha$, $\beta$ (red solid line), Bayesian statistics with constant $\alpha$, $\beta$ (green dotted line) and Bayesian statistics with linear $\alpha$, $\beta$ (blue dashed line) based on full JLA data. For all 4 models, the best-fit of $\Omega_{m0}$ given by Bayesian statistics with linear $\alpha$, $\beta$ is around $0.45$, which is larger than the results given by the other case. $\Omega_{m0}$ from $\chi^2$ statistics with constant $\alpha$, $\beta$ is the minimum value among this three cases. This cosmological fitting is not ideal, because this results is based on the JLA data only, which is not capable to constrain the parameter $\Omega_{m0}$. Therefore, it is necessary to consider using combined observational data to constrain these parameters.Fig. 6
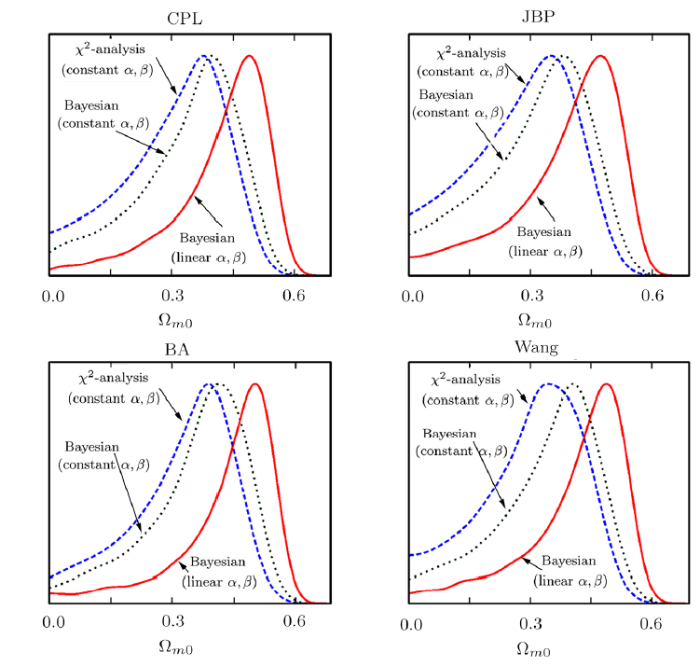
Fig. 6(Color online) The 1D marginalized probability distributions of $\Omega_{m0}$ for CPL, JBP, BA and Wang models given by different statistical methods. We apply $\chi^2$ statistics with constant $\alpha$, $\beta$ (red solid line), Bayesian statistics with constant $\alpha$, $\beta$ (black dotted line) and Bayesian statistics with linear $\alpha$, $\beta$ (blue dashed line) based on full JLA data.
Based on the combined JLA+CMB+GC data, Fig. 7 presents the 1D marginalized probability distributions of $\Omega_{m0}$ obtained from different statistical methods. It should be pointed out that we use CMB data from Ref. [45] and other distance priors data from Refs. [46—48]. And we use updated Galaxy Cluster (GC) data extracted from SDSS samples.[49] We apply $\chi^2$ statistics with constant $\alpha$, $\beta$ (red solid line), Bayesian statistics with constant $\alpha$, $\beta$ (green dotted line) and Bayesian statistics with linear $\alpha$, $\beta$ (blue dashed line) on CPL, JBP, BA and Wang models. It is clear that $\Omega_{m0}$ given by JLA+CMB+GC data is less than the values from JLA data on the whole. The best-fit of $\Omega_{m0}$ given by Bayesian statistics with linear $\alpha$, $\beta$ is around 0.311 (see Table 2), which is much closer to results of Ref. [45] than other two cases. $\Omega_{m0}$ given by Bayesian statistics with linear $\alpha$, $\beta$ is less than the results from Bayesian statistics with linear $\alpha$, $\beta$. $\Omega_{m0}$ given by $\chi^2$ statistics with constant $\alpha$, $\beta$ is still minimal among three cases.
Fig. 7
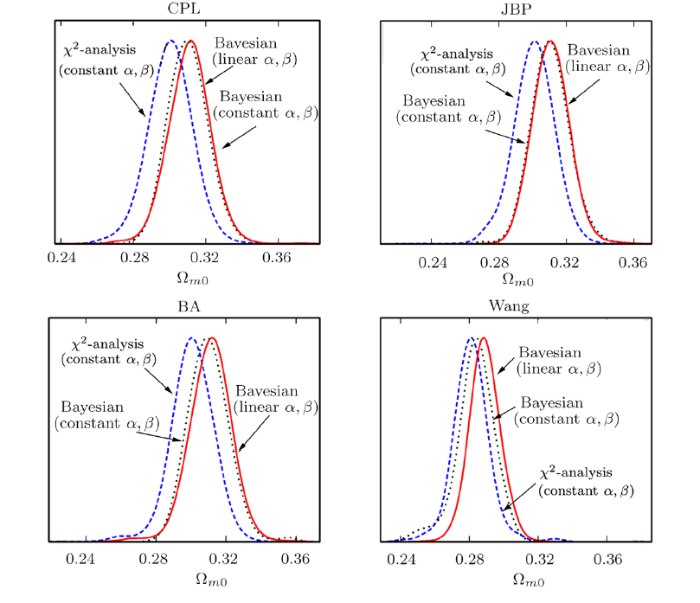
Fig. 7(Color online) The 1D marginalized probability distributions of $\Omega_{m0}$ by using $\chi^2$ statistics with constant $\alpha$, $\beta$ (red solid line) and Bayesian statistics with linear $\alpha$, $\beta$ (blue dashed line). We consider the CPL, JBP, BA and Wang models respectively, based on the combined JLA+CMB+GC data.
Table 2
Table 2
![]() |
New window|CSV
Furthermore, we extend this comparison with more details. Table 2 presents the cosmology-fits results for the CPL, the JBP, the BA and the Wang models, obtained from $\chi^2$ statistics with constant $\alpha$, $\beta$, Bayesian statistics with constant $\alpha$, $\beta$ and Bayesian statistics with linear $\alpha$, $\beta$. “$\chi^2$ const” denotes $\chi^2$ statistics with constant $\alpha$ and $\beta$. “Bayesian const” represents Bayesian statistics with constant $\alpha$ and $\beta$. “Bayesian linear” corresponds to Bayesian statistics with linear $\alpha$ and $\beta$. Both the best-fit result and the 1$\sigma$ errors of these parameters, as well as the corresponding results of FoM are listed in this table. The combined JLA+CMB+GC data are used in the analysis. From Table 2, it is apparent to see that, for all the four DE models, the case of applying “Bayesian linear" will yield a larger $\Omega_{m0}$, compared with the cases of “$\chi^2$ const” and “Bayesian const”. The comparison between these three cases is already shown in Fig. 7.
Moreover, FoM given by Bayesian statistics is apparently larger than the values given by $\chi^2$ statistics (constant $\alpha$, $\beta$). For instance, for CPL model, FoM of Bayesian statistics is about two times Fom of $\chi^2$ statistics. As we know, a larger value of FoM implies a better accuracy for constraining parameters. This means that we can obtain tighter DE constraints from Bayesian statistics. And it should be pointed out, since this conclusion is tenable for all these four DE parameterizations, our discussion is not sensitive to the specific model of DE.
3.4 An Extended Comparison of the Deceleration Parameter $q(z)$ Given by JLA and JLA+CMB+GC
Figure 8 is the evolution of the deceleration parameter $q(z)$ for CPL, JBP, BA and Wang model given by $\chi^2$ and Bayesian statistics.Fig. 8
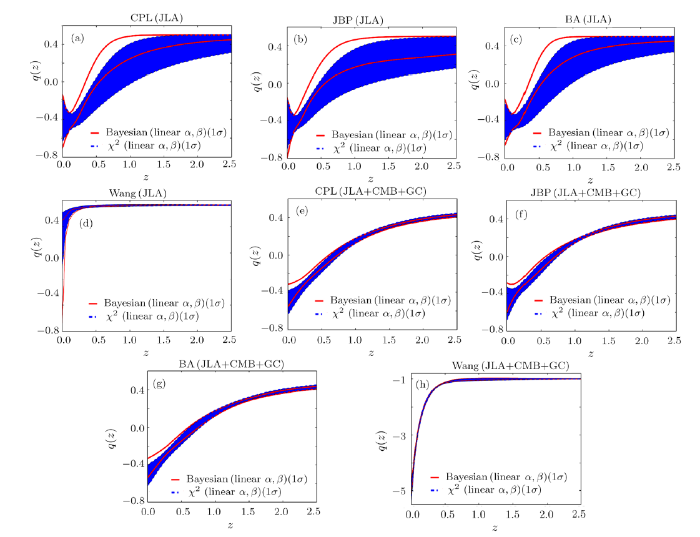
Fig. 8(Color online) The evolution of the deceleration parameter $q(z)$ for CPL, JBP, BA and Wang model given by $\chi^2$ and Bayesian statistics. The results based on the JLA only (a)—(d) and combined JLA+CMB+GC data (e)—(h). in which the absolute B-band magnitude $M_B$ are marginalized. The blue region denotes the 1$\sigma$ region of $q(z)$ for the case of $\chi^2$ statistics with constant $\alpha$ and $\beta$. The red solid line corresponds to the 1$\sigma$ boundary for the case of Bayesian statistics with linear $\alpha$ and $\beta$.
The results based on the JLA only (a)—(d) and combined JLA+CMB+GC data (e)—(h). From left panels, based on the JLA only, the 1$\sigma$ region of $q(z)$ for the case of Bayesian statistics (linear $\alpha$, $\beta$) and $\chi^2$ statistics (constant $\alpha$, $\beta$) are partly overlapped. For four models given by two statistics, $q(z)$ is consistent with an decreasing function at 1$\sigma$ CL, which favor an eternal CA. Note that this results is also consistent with Ref. [50] From right panels, based on the combined JLA+CMB+GC, the 1$\sigma$ region for the case of $\chi^2$ statistics (constant $\alpha$, $\beta$) almost locates entirely in the 1$\sigma$ region corresponds to Bayesian statistics (linear $\alpha$, $\beta$). Four models given by two statistics favor an eternal CA. It should be mentioned that, the overlapped region given by JLA+CMB+GC is larger than the results given by JLA only. This because using combined data reduce the difference between $\chi^2$ and Bayesian statistics
4 Summary
SN Ia is one of the most effective observation to study the current accelerating universe. The constrain on the systematic uncertainties of SN Ia is also seen as an important research area from precision cosmology. SN stretch and color parameter $\alpha$ and $\beta$ are considered as two free parameters in order to shrink systematic uncertainties of SN Ia. In recent years, exploring the possible evolution of $\alpha$ and $\beta$ has catch lots of attentions and yield some interesting work.[22]-[24],[33] Recently, Ref. [30] has performed a Bayesian inference method for the JLA dataset, which gives another statistical methods to derive the full posterior distribution of fitting model parameters of JLA.In this work, we adopt $\chi^2$ and Bayesian statistics on the analysis of redshift dependence of stretch and color, and make a comparison between this two statistical methods. By constraining the $\Lambda$CDM model, we check the consistency of cosmology-fit results given by the SN sample of each redshift bin. We also adopt the linear parametrization to explore the possible evolution of $\alpha$, $\beta$ and the deceleration parameter $q(z)$ for CPL, JBP, BA and Wang models.
Our conclusions are as follows:
(i) Using the full JLA data, at high redshift $\alpha$ has a trend of decreasing at more than $1.5\sigma$ CL, and $\beta$ has a significant trend of decreasing at more than $19\sigma$ CL.
(ii) Compared with $\chi^2$ statistics (constant $\alpha$, $\beta$) and Bayesian statistics (constant $\alpha$, $\beta$), Bayesian statistics (linear $\alpha$ and $\beta$) yields a larger best-fit value of fractional matter density $\Omega_{m0}$ from JLA+CMB+GC data, which is much closer to slightly deviates from the best-fit result given by other cosmological observations; However, for both these three cases, the $1\sigma$ regions of $\Omega_{m0}$ are still consistent with the result given by other observations.
(iii) The figure of merit (FoM) given by JLA +CMB+GC data from Bayesian statistics is also larger than the FoM from $\chi^2$ statistics, which indicates that former statistics has a better accuracy.
(iv) $q(z)$ given by both statistical methods favor an eternal cosmic acceleration at 1$\sigma$ CL.
Reference By original order
By published year
By cited within times
By Impact factor
[Cited within: 6]
[Cited within: 1]
[Cited within: 1]
[Cited within: 1]
[Cited within: 1]
[Cited within: 1]
[Cited within: 1]
[Cited within: 1]
[Cited within: 1]
[Cited within: 1]
[Cited within: 1]
[Cited within: 2]
[Cited within: 1]
[Cited within: 6]
[Cited within: 3]
[Cited within: 2]
[Cited within: 3]
[Cited within: 1]
[Cited within: 1]
[Cited within: 3]
[Cited within: 5]
[Cited within: 1]
[Cited within: 3]
[Cited within: 1]
[Cited within: 1]
[Cited within: 1]
[Cited within: 1]
[Cited within: 1]
[Cited within: 1]
[Cited within: 1]
[Cited within: 1]
[Cited within: 1]
[Cited within: 1]
[Cited within: 1]
[Cited within: 2]
[Cited within: 1]
[Cited within: 1]
[Cited within: 1]
[Cited within: 1]